这些年从网上的各位大牛那学到很多,本着开源开放的精神,今天我决定开源我量化交易代码。输入股票代码,和训练的数据时间,自动预测股票未来的走势。。。。。。。。。。。。。。。。。。
#!/usr/bin/env python3
# -*- coding: utf-8 -*-
"""
Created on Tue May 7 17:55:28 2019
@author: lg
"""
from matplotlib.dates import DateFormatter, WeekdayLocator, DayLocator, MONDAY,YEARLY
#from matplotlib.finance import quotes_historical_yahoo_ohlc, candlestick_ohlc
#import matplotlib
import tushare as ts
import pandas as pd
import matplotlib.pyplot as plt
from matplotlib.pylab import date2num
import datetime
import numpy as np
from pandas import DataFrame
from numpy import row_stack,column_stack
from mpl_finance import candlestick_ochl
df=ts.get_hist_data('601857',start='2019-01-15',end='2019-05-07')
dd=df[['open','high','low','close']]
from mpl_finance import candlestick_ochl,candlestick_ohlc
#print(dd.values.shape[0])
dd1=dd .sort_index()
dd2=dd1.values.flatten()
g1=dd2[::-1]
g2=g1[0:120]
g3=g2[::-1]
gg=DataFrame(g3)
gg.T.to_excel('gg.xls')
#dd3=pd.DataFrame(dd2)
#dd3.T.to_excel('d8.xls')
g=dd2[0:140]
for i in range(dd.values.shape[0]-34):
s=dd2[i*4:i*4+140]
g=row_stack((g,s))
fg=DataFrame(g)
print(fg)
fg.to_excel('fg.xls')
#-*- coding: utf-8 -*-
#建立、训练多层神经网络,并完成模型的检验
#from __future__ import print_function
import pandas as pd
inputfile1='fg.xls' #训练数据
testoutputfile = 'test_output_data.xls' #测试数据模型输出文件
data_train = pd.read_excel(inputfile1) #读入训练数据(由日志标记事件是否为洗浴)
data_mean = data_train.mean()
data_std = data_train.std()
data_train1 = (data_train-data_mean)/5 #数据标准化
y_train = data_train1.iloc[:,120:140].as_matrix() #训练样本标签列
x_train = data_train1.iloc[:,0:120].as_matrix() #训练样本特征
#y_test = data_test.iloc[:,4].as_matrix() #测试样本标签列
from keras.models import Sequential
from keras.layers.core import Dense, Dropout, Activation
model = Sequential() #建立模型
model.add(Dense(input_dim = 120, output_dim = 240)) #添加输入层、隐藏层的连接
model.add(Activation('relu')) #以Relu函数为激活函数
model.add(Dense(input_dim = 240, output_dim = 120)) #添加隐藏层、隐藏层的连接
model.add(Activation('relu')) #以Relu函数为激活函数
model.add(Dense(input_dim = 120, output_dim = 120)) #添加隐藏层、隐藏层的连接
model.add(Activation('relu')) #以Relu函数为激活函数
model.add(Dense(input_dim = 120, output_dim = 20)) #添加隐藏层、输出层的连接
model.add(Activation('sigmoid')) #以sigmoid函数为激活函数
#编译模型,损失函数为binary_crossentropy,用adam法求解
model.compile(loss='mean_squared_error', optimizer='adam')
model.fit(x_train, y_train, nb_epoch = 100, batch_size = 8) #训练模型
model.save_weights('net.model') #保存模型参数
inputfile2='gg.xls' #预测数据
pre = pd.read_excel(inputfile2)
pre_mean = data_mean[0:120]
pre_std = pre.std()
pre1 = (pre-pre_mean)/10 #数据标准化
#pre1 = (pre-pre_mean)/pre.std() #数据标准化
pre2 = pre1.iloc[:,0:120].as_matrix() #预测样本特征
r = pd.DataFrame(model.predict(pre2))
rt=r*10+data_mean[120:140].as_matrix()
print(rt.round(2))
rt.to_excel('rt.xls')
#print(r.values@data_train.iloc[:,116:120].std().values+data_mean[116:120].as_matrix())
a=list(df.index[0:-1])
b=a[0]
c= datetime.datetime.strptime(b,'%Y-%m-%d')
d = date2num(c)
c1=[d+i+1 for i in range(5)]
c2=np.array([c1])
r1=rt.values.flatten()
r2=r1[0:4]
for i in range(4):
r3=r1[i*4+4:i*4+8]
r2=row_stack((r2,r3))
c3=column_stack((c2.T,r2))
r5=DataFrame(c3)
if len(c3) == 0:
raise SystemExit
fig, ax = plt.subplots()
fig.subplots_adjust(bottom=0.2)
#ax.xaxis.set_major_locator(mondays)
#ax.xaxis.set_minor_locator(alldays)
#ax.xaxis.set_major_formatter(mondayFormatter)
#ax.xaxis.set_minor_formatter(dayFormatter)
#plot_day_summary(ax, quotes, ticksize=3)
#candlestick_ochl(ax, c3, width=0.6, colorup='r', colordown='g')
candlestick_ohlc(ax, c3, width=0.5, colorup='r', colordown='g')
ax.xaxis_date()
ax.autoscale_view()
plt.setp(plt.gca().get_xticklabels(), rotation=45, horizontalalignment='right')
ax.grid(True)
#plt.title('000002')
plt.show()
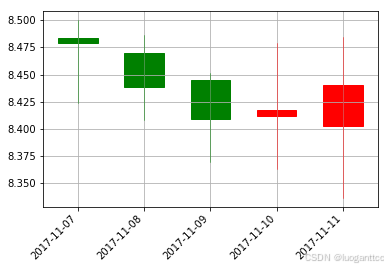