车
车辆种类分类识别数据集,可以识别7种汽车类型,已经按照7:2:1比 例划分数据集,训练集1488张、验证集507张,测试集31张, 共计2026张。 数据集分为一类客车(tinycar) ,=类客车(midcar) ,三类 客车(bigcar) , - -类货车(smalltruck) ,= _类货车(bigtru ck) ,油罐车(oil truck) 以及特殊车辆(specialcar) ,共计7 个种类。 nc:7. names: ['tinycar'midcar,'bigcar'smalltruck,'bigtruck,'oil truck',' specialcar']
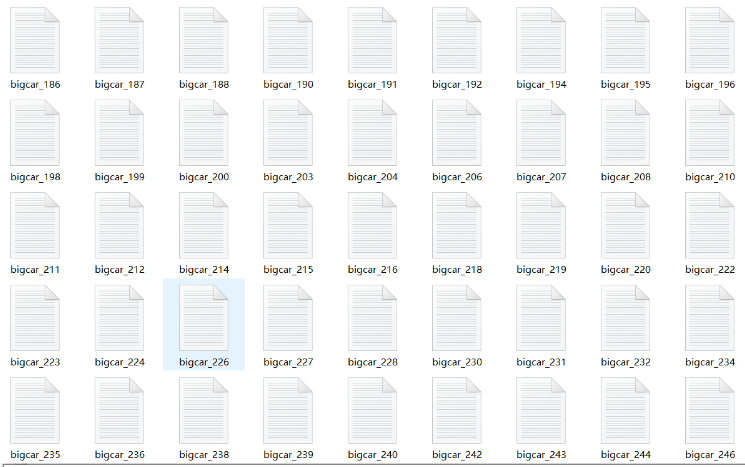
车辆种类识别数据集
规模
- 图像数量:2026张
- 类别数量:7种
- 数据量:未提供具体数据量,但通常这类数据集可能达到数百MB到几GB。
类别
- 一类客车 (Tiny Car, tinycar)
- 二类客车 (Mid Car, midcar)
- 三类客车 (Big Car, bigcar)
- 一类货车 (Small Truck, smalltruck)
- 二类货车 (Big Truck, bigtruck)
- 油罐车 (Oil Truck, oiltruck)
- 特殊车辆 (Special Car, specialcar)
每类车辆的样本数量根据比例划分:
- 训练集:1488张
- 验证集:507张
- 测试集:31张
数据特点
- 高质量图像:所有图像均为高分辨率,提供了丰富的细节信息,有助于提高检测精度。
- 多样化车辆类型:涵盖了七种常见的车辆类型,确保模型能够适应多种类型的车辆。
- 详细标注:每张图像都附有详细的边界框标注(txt格式),标明了车辆的位置和大小。
- 预处理数据:数据集已经按照7:2:1的比例划分好训练集、验证集和测试集,可以直接用于训练。
应用场景
- 智能交通系统:在城市交通监控中自动识别不同类型的车辆,提高交通管理效率。
- 自动驾驶:帮助自动驾驶汽车更好地理解和分类道路上的各种车辆,提升驾驶安全性。
- 停车场管理:在停车场管理系统中自动识别车辆类型,便于分类管理和收费。
- 物流管理:在物流运输过程中,自动识别货车类型,优化货物装载和运输方案。
- 研究与教育:用于科研机构的研究以及相关院校的教学,帮助学生和研究人员更好地了解车辆识别技术。
- 安全监控:集成到视频监控系统中,自动识别特定类型的车辆,支持安全监控和预警。
数据集结构
假设数据集的文件结构如下:
vehicle_classification_dataset/
├── images/
│ ├── train/
│ │ ├── 0001.jpg
│ │ ├── 0002.jpg
│ │ └── ...
│ ├── val/
│ │ ├── 0001.jpg
│ │ ├── 0002.jpg
│ │ └── ...
│ └── test/
│ ├── 0001.jpg
│ ├── 0002.jpg
│ └── ...
├── labels_txt/
│ ├── train/
│ │ ├── 0001.txt
│ │ ├── 0002.txt
│ │ └── ...
│ ├── val/
│ │ ├── 0001.txt
│ │ ├── 0002.txt
│ │ └── ...
│ └── test/
│ ├── 0001.txt
│ ├── 0002.txt
│ └── ...
└── metadata.csv
metadata.csv
文件内容示例:
深色版本
image_id, category, split
train/0001.jpg, tinycar, train
train/0002.jpg, midcar, train
val/0001.jpg, bigcar, val
val/0002.jpg, smalltruck, val
test/0001.jpg, bigtruck, test
...
labels_txt/0001.txt
示例(YOLO格式):
0 0.5 0.5 0.3 0.3 # 类别ID, 中心点x, 中心点y, 宽度, 高度
代码示例
下面是一个完整的Python脚本示例,展示如何加载数据集、使用预训练的YOLOv5模型进行车辆种类识别,并可视化检测结果。我们将使用PyTorch和YOLOv5的相关库。
1. 安装依赖库
首先,确保安装了必要的依赖库。可以在项目目录中的requirements.txt
文件中列出这些依赖库,然后运行以下命令进行安装:
pip install -r requirements.txt
requirements.txt
文件内容示例:
torch==1.10.0
torchvision==0.11.1
opencv-python-headless==4.5.4.60
yolov5 @ git+https://github.com/ultralytics/yolov5.git
2. 加载数据集和预训练模型
import os
import cv2
import torch
import numpy as np
from yolov5.models.common import DetectMultiBackend
from yolov5.utils.general import (check_img_size, non_max_suppression, scale_coords)
from yolov5.utils.torch_utils import select_device
from yolov5.utils.plots import Annotator, colors
# 设置设备
device = select_device('') # 使用默认设备(通常是GPU,如果没有则使用CPU)
# 加载预训练模型
model_path = 'path_to_your_model_directory/yolov5s_vehicle_detection.pt'
model = DetectMultiBackend(model_path, device=device, dnn=False, data=None, fp16=False)
imgsz = check_img_size(640, s=model.stride) # 检查图像尺寸
# 设置模型为评估模式
model.eval()
# 加载图像
def load_image(image_path):
img = cv2.imread(image_path)
if img is None:
print(f"Failed to load image: {image_path}")
return None
return img
# 进行推理
def detect_vehicles(img, model, imgsz, device):
# 转换图像
img = [letterbox(img, new_shape=imgsz, auto=True)[0]]
img = np.stack(img, 0)
img = img[..., ::-1].transpose((0, 3, 1, 2)) # BGR to RGB, BHWC to BCHW
img = np.ascontiguousarray(img)
# 将图像转换为Tensor
img = torch.from_numpy(img).to(device)
img = img.float()
img /= 255.0 # 0 - 255 to 0.0 - 1.0
if len(img.shape) == 3:
img = img[None] # 扩展批处理维度
# 推理
with torch.no_grad():
pred = model(img, augment=False, visualize=False)[0]
pred = non_max_suppression(pred, conf_thres=0.5, iou_thres=0.45, classes=None, agnostic=False, max_det=1000)
# 处理预测结果
for i, det in enumerate(pred): # 每张图像的检测结果
if len(det):
det[:, :4] = scale_coords(img.shape[2:], det[:, :4], img.shape[2:]).round()
annotator = Annotator(img[i].permute(1, 2, 0).cpu().numpy(), line_width=3, example=str('vehicle'))
for *xyxy, conf, cls in reversed(det):
label = f'{names[int(cls)]} {conf:.2f}'
annotator.box_label(xyxy, label, color=colors(int(cls), True))
return annotator.result()
return img[0].permute(1, 2, 0).cpu().numpy()
# 字母框调整
def letterbox(img, new_shape=(640, 640), color=(114, 114, 114), auto=True, scaleFill=False, scaleup=True, stride=32):
shape = img.shape[:2] # 当前形状 [height, width]
if isinstance(new_shape, int):
new_shape = (new_shape, new_shape)
r = min(new_shape[0] / shape[0], new_shape[1] / shape[1])
if not scaleup: # 只缩小,不放大
r = min(r, 1.0)
ratio = r, r # 宽高比
new_unpad = int(round(shape[1] * r)), int(round(shape[0] * r))
dw, dh = new_shape[1] - new_unpad[0], new_shape[0] - new_unpad[1] # wh padding
if auto: # 最小矩形
dw, dh = np.mod(dw, stride), np.mod(dh, stride) # wh padding
elif scaleFill: # 拉伸
dw, dh = 0.0, 0.0
new_unpad = (new_shape[1], new_shape[0])
ratio = new_shape[1] / shape[1], new_shape[0] / shape[0] # 宽高比
dw /= 2 # 分配到两边
dh /= 2
if shape[::-1] != new_unpad: # 缩放
img = cv2.resize(img, new_unpad, interpolation=cv2.INTER_LINEAR)
top, bottom = int(round(dh - 0.1)), int(round(dh + 0.1))
left, right = int(round(dw - 0.1)), int(round(dw + 0.1))
img = cv2.copyMakeBorder(img, top, bottom, left, right, cv2.BORDER_CONSTANT, value=color) # 添加边框
return img, ratio, (dw, dh)
# 主函数
def main(image_dir, model, imgsz, device):
names = ['tinycar', 'midcar', 'bigcar', 'smalltruck', 'bigtruck', 'oiltruck', 'specialcar']
for image_name in os.listdir(image_dir):
if image_name.endswith('.jpg'):
image_path = os.path.join(image_dir, image_name)
img = load_image(image_path)
if img is not None:
result = detect_vehicles(img, model, imgsz, device)
cv2.imshow('Vehicle Detection', result)
cv2.setWindowTitle('Vehicle Detection', f'Image: {image_name}')
if cv2.waitKey(0) & 0xFF == ord('q'):
break
cv2.destroyAllWindows()
# 假设图像存储在'image'目录下
image_dir = 'path_to_your_image_directory'
# 运行主函数
main(image_dir, model, imgsz, device)
说明
- 路径设置 :请根据实际的数据集路径调整
path_to_your_image_directory
和path_to_your_model_directory
。 - 文件命名 :假设图像文件名分别为
.jpg
。如果实际命名规则不同,请相应修改代码。 - 可视化:通过绘制边界框和标注置信度,可以直观地看到图像中的车辆位置和类型。
进一步的应用
- 训练深度学习模型:可以使用这个数据集来进一步训练或微调YOLOv5模型,以提高检测精度。
- 数据增强:为了增加数据集的多样性和鲁棒性,可以使用数据增强技术(如旋转、翻转、缩放等)生成更多的训练样本。
- 评估与优化:通过交叉验证和测试集评估模型性能,并不断优化模型参数,以提高检测准确率。
这个数据集对于车辆种类识别具有重要的实用价值,可以帮助相关部门及时发现和处理不同类型车辆,提升交通管理和安全水平。
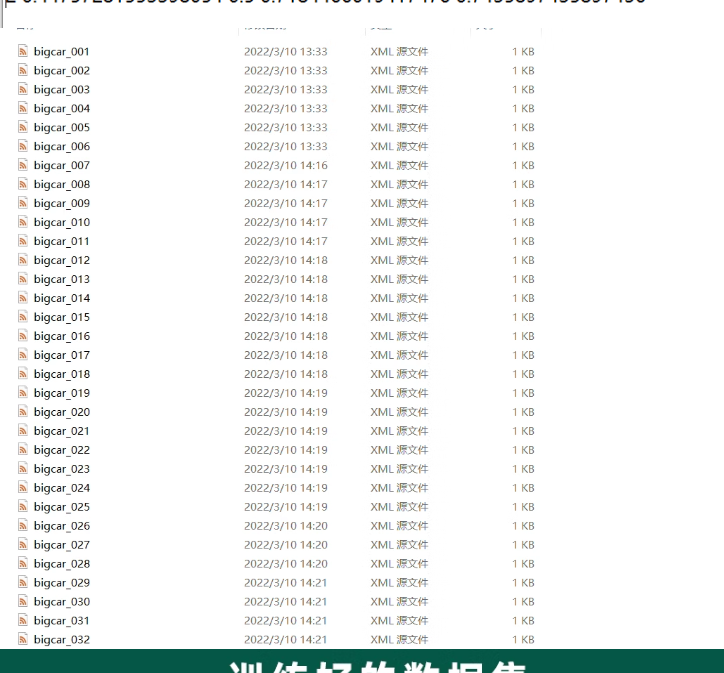