T11周:优化器对比实验
- 🍨 本文为🔗365天深度学习训练营 中的学习记录博客
- 🍖 原作者:K同学啊
🍺
本次主要是探究不同优化器、以及不同参数配置对模型的影响,在论文当中我们也可以进行优化器的比对,以增加论文工作量。·
⛽ 我的环境
- 语言环境:Python3.10.12
- 编译器:Google Colab
- 深度学习环境:
- TensorFlow2.17.0
一、前期工作
1.设置GPU,导入库
python
#os提供了一些与操作系统交互的功能,比如文件和目录操作
import os
#提供图像处理的功能,包括打开和显示、保存、裁剪等
import PIL
from PIL import Image
#pathlib提供了一个面向对象的接口来处理文件系统路径。路径被表示为Path对象,可以调用方法来进行各种文件和目录操作。
import pathlib
#用于绘制图形和可视化数据
import tensorflow as tf
import matplotlib.pyplot as plt
#用于数值计算的库,提供支持多维数组和矩阵运算
import numpy as np
#keras作为高层神经网络API,已被集成进tensorflow,使得训练更方便简单
from tensorflow import keras
#layers提供了神经网络的基本构建块,比如全连接层、卷积层、池化层等
#提供了构建和训练神经网络模型的功能,包括顺序模型(Sequential)和函数式模型(Functional API)
from tensorflow.keras import layers, models
#导入两个重要的回调函数:前者用于训练期间保存模型最佳版本;后者监测到模型性能不再提升时提前停止训练,避免过拟合
from tensorflow.keras.callbacks import ModelCheckpoint, EarlyStopping
python
import tensorflow as tf
gpus = tf.config.list_physical_devices("GPU")
if gpus:
gpu0 = gpus[0] #如果有多个GPU,仅使用第0个GPU
tf.config.experimental.set_memory_growth(gpu0, True) #设置GPU显存用量按需使用
tf.config.set_visible_devices([gpu0],"GPU")
from tensorflow import keras
import matplotlib.pyplot as plt
import pandas as pd
import numpy as np
import warnings,os,PIL,pathlib
warnings.filterwarnings("ignore") #忽略警告信息
#plt.rcParams['font.sans-serif'] = ['SimHei'] # 用来正常显示中文标签
plt.rcParams['axes.unicode_minus'] = False # 用来正常显示负号
二、数据预处理
1.导入数据
python
from google.colab import drive
drive.mount("/content/drive/")
%cd "/content/drive/My Drive/Colab Notebooks/jupyter notebook/data/"
Drive already mounted at /content/drive/; to attempt to forcibly remount, call drive.mount("/content/drive/", force_remount=True).
/content/drive/My Drive/Colab Notebooks/jupyter notebook/data
python
data_dir = "./T6"
data_dir = pathlib.Path(data_dir)
# 使用glob方法获取当前目录的子目录里所有以'.jpg'为结尾的文件
# '*/*.jpg' 是一個通配符模式
# 第一个星号表示当前目录
# 第二个星号表示子目录
image_count = len (list(data_dir.glob("*/*.jpg")))
print("图片总数:", image_count)
图片总数: 1800
python
#设置批量大小,即每次训练模型时输入图像数量
#每次训练迭代时,模型需处理32张图像
batch_size = 16
#图像的高度,加载图像数据时,将所有的图像调整为相同的高度
img_height = 336
#图像的宽度,加载图像数据时,将所有的图像调整为相同的宽度
img_width = 336
python
"""
关于image_dataset_from_directory()的详细介绍可以参考文章:https://mtyjkh.blog.csdn.net/article/details/117018789
"""
train_ds = tf.keras.preprocessing.image_dataset_from_directory(
data_dir,
validation_split=0.2,
#指定数据集中分割出多少比例数据当作验证集,0.1表示10%数据会被用来当验证集
subset="training",
#指定是用于训练还是验证的数据子集,这里设定为training
seed=12,
#用于设置随机数种子,以确保数据集划分的可重复性和一致性
image_size=(img_height, img_width),
batch_size=batch_size)
Found 1800 files belonging to 17 classes.
Using 1440 files for training.
python
"""
关于image_dataset_from_directory()的详细介绍可以参考文章:https://mtyjkh.blog.csdn.net/article/details/117018789
"""
val_ds = tf.keras.preprocessing.image_dataset_from_directory(
data_dir,
validation_split=0.2,
subset="validation",
seed=12,
image_size=(img_height, img_width),
batch_size=batch_size)
Found 1800 files belonging to 17 classes.
Using 360 files for validation.
python
class_names = train_ds.class_names
# 可以通过class_names输出数据集的标签。标签将按字母顺序对应于目录名称
class_names
['Angelina Jolie',
'Brad Pitt',
'Denzel Washington',
'Hugh Jackman',
'Jennifer Lawrence',
'Johnny Depp',
'Kate Winslet',
'Leonardo DiCaprio',
'Megan Fox',
'Natalie Portman',
'Nicole Kidman',
'Robert Downey Jr',
'Sandra Bullock',
'Scarlett Johansson',
'Tom Cruise',
'Tom Hanks',
'Will Smith']
2.检查数据
python
for image_batch, labels_batch in train_ds:
print(image_batch.shape)
print(labels_batch.shape)
break
(16, 336, 336, 3)
(16,)
3.配置数据集
python
#自动调整数据管道性能
AUTOTUNE = tf.data.AUTOTUNE
# 使用 tf.data.AUTOTUNE 具体的好处包括:
#自动调整并行度:自动决定并行处理数据的最佳线程数,以最大化数据吞吐量。
#减少等待时间:通过优化数据加载和预处理,减少模型训练时等待数据的时间。
#提升性能:自动优化数据管道的各个环节,使整个训练过程更高效。
#简化代码:不需要手动调整参数,代码更简洁且易于维护。
#使用cache()方法将训练集缓存到内存中,这样加快数据加载速度
#当多次迭代训练数据时,可以重复使用已经加载到内存的数据而不必重新从磁盘加载
#使用shuffle()对训练数据集进行洗牌操作,打乱数据集中的样本顺序
#参数1000指缓冲区大小,即每次从数据集中随机选择的样本数量
#prefetch()预取数据,节约在训练过程中数据加载时间
def train_preprocessing(image,label):
return(image/255.0,label)
train_ds = train_ds.cache().shuffle(1000).map(train_preprocessing).prefetch(buffer_size=AUTOTUNE)
val_ds = val_ds.cache().shuffle(1000).map(train_preprocessing).prefetch(buffer_size=AUTOTUNE)
4.数据可视化
python
plt.figure(figsize=(10, 8)) # 图形的宽为10高为5
plt.suptitle("数据展示")
for images, labels in train_ds.take(1):
for i in range(15):
plt.subplot(4, 5, i + 1)
plt.xticks([])
plt.yticks([])
plt.grid(False)
# 显示图片
plt.imshow(images[i])
# 显示标签
plt.xlabel(class_names[labels[i]-1])
plt.show()
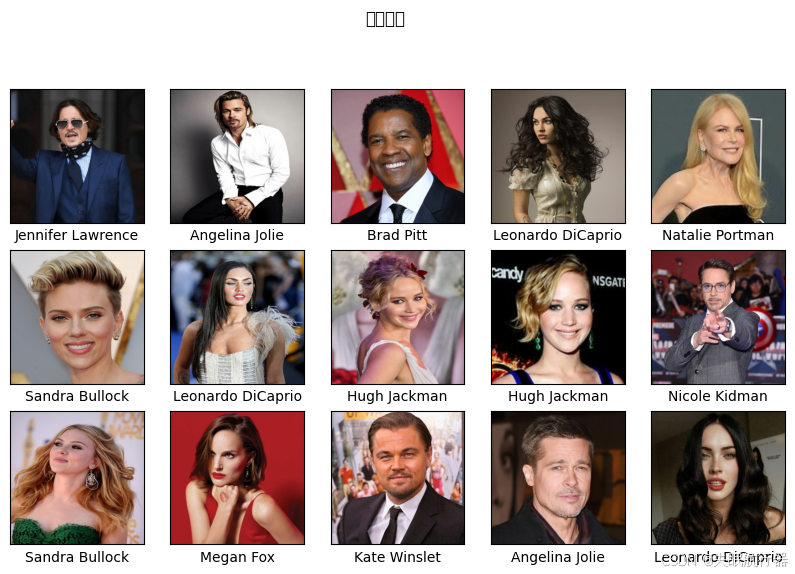
三、构建模型
python
from tensorflow.keras.layers import Dropout,Dense,BatchNormalization
from tensorflow.keras.models import Model
def create_model(optimizer):
# 加载预训练模型
vgg16_base_model = tf.keras.applications.vgg16.VGG16(
weights='imagenet',
include_top=False,
input_shape=(img_width, img_height, 3),
pooling='avg')
for layer in vgg16_base_model.layers:
layer.trainable = False
X = vgg16_base_model.output
X = Dense(170, activation='relu')(X)
X = BatchNormalization()(X)
X = Dropout(0.5)(X)
output = Dense(len(class_names), activation='softmax')(X)
vgg16_model = Model(inputs=vgg16_base_model.input, outputs=output)
vgg16_model.compile(optimizer=optimizer,
loss='sparse_categorical_crossentropy',
metrics=['accuracy'])
return vgg16_model
model1 = create_model(optimizer=tf.keras.optimizers.Adam())
model2 = create_model(optimizer=tf.keras.optimizers.SGD())
model2.summary()
四、训练模型
python
NO_epochs = 50
history_model1=model1.fit(train_ds,epochs=NO_epochs,validation_data=val_ds)
history_model2=model2.fit(train_ds,epochs=NO_epochs,validation_data=val_ds)
部分训练过程:
五、模型评估
python
from matplotlib.ticker import MultipleLocator
plt.rcParams['savefig.dpi'] = 300 #图片像素
plt.rcParams['figure.dpi'] = 300 #分辨率
acc1 = history_model1.history['accuracy']
acc2 = history_model2.history['accuracy']
val_acc1 = history_model1.history['val_accuracy']
val_acc2 = history_model2.history['val_accuracy']
loss1 = history_model1.history['loss']
loss2 = history_model2.history['loss']
val_loss1 = history_model1.history['val_loss']
val_loss2 = history_model2.history['val_loss']
epochs_range = range(len(acc1))
plt.figure(figsize=(16, 4))
plt.subplot(1, 2, 1)
plt.plot(epochs_range, acc1, label='Training Accuracy-Adam')
plt.plot(epochs_range, acc2, label='Training Accuracy-SGD')
plt.plot(epochs_range, val_acc1, label='Validation Accuracy-Adam')
plt.plot(epochs_range, val_acc2, label='Validation Accuracy-SGD')
plt.legend(loc='lower right')
plt.title('Training and Validation Accuracy')
# 设置刻度间隔,x轴每1一个刻度
ax = plt.gca()
ax.xaxis.set_major_locator(MultipleLocator(1))
plt.subplot(1, 2, 2)
plt.plot(epochs_range, loss1, label='Training Loss-Adam')
plt.plot(epochs_range, loss2, label='Training Loss-SGD')
plt.plot(epochs_range, val_loss1, label='Validation Loss-Adam')
plt.plot(epochs_range, val_loss2, label='Validation Loss-SGD')
plt.legend(loc='upper right')
plt.title('Training and Validation Loss')
# 设置刻度间隔,x轴每1一个刻度
ax = plt.gca()
ax.xaxis.set_major_locator(MultipleLocator(1))
plt.show()
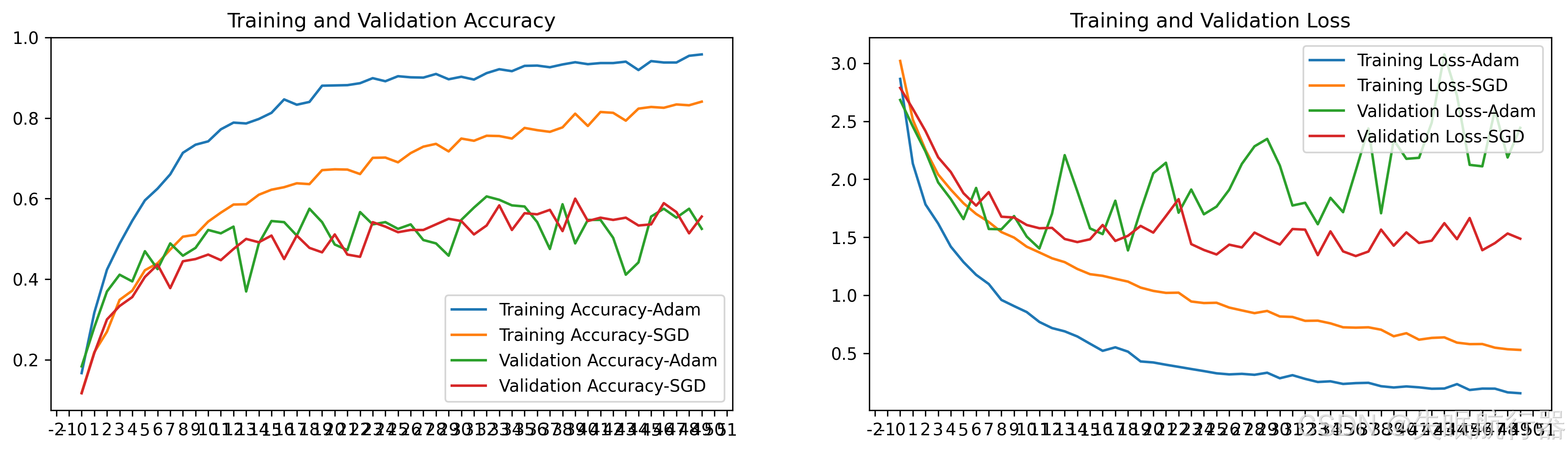
python
def test_accuracy_report(model):
score = model.evaluate(val_ds, verbose=1)
print('Loss function: %s, accuracy:' % score[0], score[1])
print("model1:")
test_accuracy_report(model1)
print("model2:")
test_accuracy_report(model2)
model1:
[1m23/23[0m [32m━━━━━━━━━━━━━━━━━━━━[0m[37m[0m [1m3s[0m 140ms/step - accuracy: 0.5283 - loss: 2.5871
Loss function: 2.443842649459839, accuracy: 0.5249999761581421
model2:
[1m23/23[0m [32m━━━━━━━━━━━━━━━━━━━━[0m[37m[0m [1m3s[0m 141ms/step - accuracy: 0.5863 - loss: 1.3692
Loss function: 1.4873623847961426, accuracy: 0.5555555820465088
六、总结
本周学习了调用vgg16并构建functional model来进行不同优化器的设置和训练,并在最后对训练参数过程进行可视化。