使用ultralytics 工程(https://github.com/ultralytics/ultralytics)导出onnx模型:
python
from ultralytics import RTDETR
# Load a model
model = RTDETR("rtdetr-l.pt")
# Export the model
model.export(format="onnx")
model.export(format="engine")
onnxruntime推理
python
import cv2
import math
import numpy as np
import onnxruntime
class_names = ['person', 'bicycle', 'car', 'motorcycle', 'airplane', 'bus', 'train', 'truck', 'boat', 'traffic light',
'fire hydrant', 'stop sign', 'parking meter', 'bench', 'bird', 'cat', 'dog', 'horse', 'sheep', 'cow',
'elephant', 'bear', 'zebra', 'giraffe', 'backpack', 'umbrella', 'handbag', 'tie', 'suitcase', 'frisbee',
'skis', 'snowboard', 'sports ball', 'kite', 'baseball bat', 'baseball glove', 'skateboard', 'surfboard',
'tennis racket', 'bottle', 'wine glass', 'cup', 'fork', 'knife', 'spoon', 'bowl', 'banana', 'apple',
'sandwich', 'orange', 'broccoli', 'carrot', 'hot dog', 'pizza', 'donut', 'cake', 'chair', 'couch',
'potted plant', 'bed', 'dining table', 'toilet', 'tv', 'laptop', 'mouse', 'remote', 'keyboard', 'cell phone',
'microwave', 'oven', 'toaster', 'sink', 'refrigerator', 'book', 'clock', 'vase', 'scissors', 'teddy bear',
'hair drier', 'toothbrush'] #coco80类别
input_shape = (640, 640)
score_threshold = 0.5
nms_threshold = 0.5
def nms(boxes, scores, score_threshold, nms_threshold):
x1 = boxes[:, 0]
y1 = boxes[:, 1]
x2 = boxes[:, 2]
y2 = boxes[:, 3]
areas = (y2 - y1 + 1) * (x2 - x1 + 1)
keep = []
index = scores.argsort()[::-1]
while index.size > 0:
i = index[0]
keep.append(i)
x11 = np.maximum(x1[i], x1[index[1:]])
y11 = np.maximum(y1[i], y1[index[1:]])
x22 = np.minimum(x2[i], x2[index[1:]])
y22 = np.minimum(y2[i], y2[index[1:]])
w = np.maximum(0, x22 - x11 + 1)
h = np.maximum(0, y22 - y11 + 1)
overlaps = w * h
ious = overlaps / (areas[i] + areas[index[1:]] - overlaps)
idx = np.where(ious <= nms_threshold)[0]
index = index[idx + 1]
return keep
def xywh2xyxy(x):
y = np.copy(x)
y[:, 0] = x[:, 0] - x[:, 2] / 2
y[:, 1] = x[:, 1] - x[:, 3] / 2
y[:, 2] = x[:, 0] + x[:, 2] / 2
y[:, 3] = x[:, 1] + x[:, 3] / 2
return y
def filter_box(output): #过滤掉无用的框
output = np.squeeze(output)
boxes = []
scores = []
class_ids = []
output = output[output[..., 4] > score_threshold]
for i in range(output.shape[0]):
boxes.append(output[i, :6])
scores.append(output[i][4])
class_ids.append(output[i][5])
boxes = np.array(boxes)
boxes = xywh2xyxy(boxes)
boxes[..., [0, 2]] *= input_shape[0]
boxes[..., [1, 3]] *= input_shape[1]
return boxes
def letterbox(im, new_shape=(416, 416), color=(114, 114, 114)):
# Resize and pad image while meeting stride-multiple constraints
shape = im.shape[:2] # current shape [height, width]
# Scale ratio (new / old)
r = min(new_shape[0] / shape[0], new_shape[1] / shape[1])
# Compute padding
new_unpad = int(round(shape[1] * r)), int(round(shape[0] * r))
dw, dh = (new_shape[1] - new_unpad[0])/2, (new_shape[0] - new_unpad[1])/2 # wh padding
top, bottom = int(round(dh - 0.1)), int(round(dh + 0.1))
left, right = int(round(dw - 0.1)), int(round(dw + 0.1))
if shape[::-1] != new_unpad: # resize
im = cv2.resize(im, new_unpad, interpolation=cv2.INTER_LINEAR)
im = cv2.copyMakeBorder(im, top, bottom, left, right, cv2.BORDER_CONSTANT, value=color) # add border
return im
def scale_boxes(boxes, output_shape):
# Rescale boxes (xyxy) from self.input_shape to shape
gain = min(input_shape[0] / output_shape[0], input_shape[1] / output_shape[1]) # gain = old / new
pad = (input_shape[1] - output_shape[1] * gain) / 2, (input_shape[0] - output_shape[0] * gain) / 2 # wh padding
boxes[..., [0, 2]] -= pad[0] # x padding
boxes[..., [1, 3]] -= pad[1] # y padding
boxes[..., :4] /= gain
boxes[..., [0, 2]] = boxes[..., [0, 2]].clip(0, output_shape[1]) # x1, x2
boxes[..., [1, 3]] = boxes[..., [1, 3]].clip(0, output_shape[0]) # y1, y2
return boxes
def draw(image, box_data):
box_data = scale_boxes(box_data, image.shape)
boxes = box_data[...,:4].astype(np.int32)
scores = box_data[...,4]
classes = box_data[...,5].astype(np.int32)
for box, score, cl in zip(boxes, scores, classes):
top, left, right, bottom = box
cv2.rectangle(image, (top, left), (right, bottom), (255, 0, 0), 1)
cv2.putText(image, '{0} {1:.2f}'.format(class_names[cl], score), (top, left), cv2.FONT_HERSHEY_SIMPLEX, 0.6, (0, 0, 255), 1)
if __name__=="__main__":
image = cv2.imread('bus.jpg', -1)
input = letterbox(image, input_shape)
input = input[:, :, ::-1].transpose(2, 0, 1).astype(dtype=np.float32) #BGR2RGB和HWC2CHW
input = input / 255.0
input_tensor = []
input_tensor.append(input)
onnx_session = onnxruntime.InferenceSession('rtdetr-l.onnx', providers=['CPUExecutionProvider', 'CUDAExecutionProvider'])
input_name = []
for node in onnx_session.get_inputs():
input_name.append(node.name)
output_name = []
for node in onnx_session.get_outputs():
output_name.append(node.name)
inputs = {}
for name in input_name:
inputs[name] = np.array(input_tensor)
outputs = onnx_session.run(None, inputs)[0]
boxes = filter_box(outputs)
draw(image, boxes)
cv2.imwrite('result.jpg', image)
tensorrt推理
python
import cv2
import math
import numpy as np
import tensorrt as trt
import pycuda.autoinit
import pycuda.driver as cuda
class_names = ['person', 'bicycle', 'car', 'motorcycle', 'airplane', 'bus', 'train', 'truck', 'boat', 'traffic light',
'fire hydrant', 'stop sign', 'parking meter', 'bench', 'bird', 'cat', 'dog', 'horse', 'sheep', 'cow',
'elephant', 'bear', 'zebra', 'giraffe', 'backpack', 'umbrella', 'handbag', 'tie', 'suitcase', 'frisbee',
'skis', 'snowboard', 'sports ball', 'kite', 'baseball bat', 'baseball glove', 'skateboard', 'surfboard',
'tennis racket', 'bottle', 'wine glass', 'cup', 'fork', 'knife', 'spoon', 'bowl', 'banana', 'apple',
'sandwich', 'orange', 'broccoli', 'carrot', 'hot dog', 'pizza', 'donut', 'cake', 'chair', 'couch',
'potted plant', 'bed', 'dining table', 'toilet', 'tv', 'laptop', 'mouse', 'remote', 'keyboard', 'cell phone',
'microwave', 'oven', 'toaster', 'sink', 'refrigerator', 'book', 'clock', 'vase', 'scissors', 'teddy bear',
'hair drier', 'toothbrush'] #coco80类别
input_shape = (640, 640)
score_threshold = 0.5
nms_threshold = 0.5
def nms(boxes, scores, score_threshold, nms_threshold):
x1 = boxes[:, 0]
y1 = boxes[:, 1]
x2 = boxes[:, 2]
y2 = boxes[:, 3]
areas = (y2 - y1 + 1) * (x2 - x1 + 1)
keep = []
index = scores.argsort()[::-1]
while index.size > 0:
i = index[0]
keep.append(i)
x11 = np.maximum(x1[i], x1[index[1:]])
y11 = np.maximum(y1[i], y1[index[1:]])
x22 = np.minimum(x2[i], x2[index[1:]])
y22 = np.minimum(y2[i], y2[index[1:]])
w = np.maximum(0, x22 - x11 + 1)
h = np.maximum(0, y22 - y11 + 1)
overlaps = w * h
ious = overlaps / (areas[i] + areas[index[1:]] - overlaps)
idx = np.where(ious <= nms_threshold)[0]
index = index[idx + 1]
return keep
def xywh2xyxy(x):
y = np.copy(x)
y[:, 0] = x[:, 0] - x[:, 2] / 2
y[:, 1] = x[:, 1] - x[:, 3] / 2
y[:, 2] = x[:, 0] + x[:, 2] / 2
y[:, 3] = x[:, 1] + x[:, 3] / 2
return y
def filter_box(output): #过滤掉无用的框
output = np.squeeze(output)
boxes = []
scores = []
class_ids = []
output = output[output[..., 4] > score_threshold]
for i in range(output.shape[0]):
boxes.append(output[i, :6])
scores.append(output[i][4])
class_ids.append(output[i][5])
boxes = np.array(boxes)
boxes = xywh2xyxy(boxes)
boxes[..., [0, 2]] *= input_shape[0]
boxes[..., [1, 3]] *= input_shape[1]
return boxes
def letterbox(im, new_shape=(416, 416), color=(114, 114, 114)):
# Resize and pad image while meeting stride-multiple constraints
shape = im.shape[:2] # current shape [height, width]
# Scale ratio (new / old)
r = min(new_shape[0] / shape[0], new_shape[1] / shape[1])
# Compute padding
new_unpad = int(round(shape[1] * r)), int(round(shape[0] * r))
dw, dh = (new_shape[1] - new_unpad[0])/2, (new_shape[0] - new_unpad[1])/2 # wh padding
top, bottom = int(round(dh - 0.1)), int(round(dh + 0.1))
left, right = int(round(dw - 0.1)), int(round(dw + 0.1))
if shape[::-1] != new_unpad: # resize
im = cv2.resize(im, new_unpad, interpolation=cv2.INTER_LINEAR)
im = cv2.copyMakeBorder(im, top, bottom, left, right, cv2.BORDER_CONSTANT, value=color) # add border
return im
def scale_boxes(boxes, output_shape):
# Rescale boxes (xyxy) from self.input_shape to shape
gain = min(input_shape[0] / output_shape[0], input_shape[1] / output_shape[1]) # gain = old / new
pad = (input_shape[1] - output_shape[1] * gain) / 2, (input_shape[0] - output_shape[0] * gain) / 2 # wh padding
boxes[..., [0, 2]] -= pad[0] # x padding
boxes[..., [1, 3]] -= pad[1] # y padding
boxes[..., :4] /= gain
boxes[..., [0, 2]] = boxes[..., [0, 2]].clip(0, output_shape[1]) # x1, x2
boxes[..., [1, 3]] = boxes[..., [1, 3]].clip(0, output_shape[0]) # y1, y2
return boxes
def draw(image, box_data):
box_data = scale_boxes(box_data, image.shape)
boxes = box_data[...,:4].astype(np.int32)
scores = box_data[...,4]
classes = box_data[...,5].astype(np.int32)
for box, score, cl in zip(boxes, scores, classes):
top, left, right, bottom = box
cv2.rectangle(image, (top, left), (right, bottom), (255, 0, 0), 1)
cv2.putText(image, '{0} {1:.2f}'.format(class_names[cl], score), (top, left), cv2.FONT_HERSHEY_SIMPLEX, 0.6, (0, 0, 255), 1)
if __name__=="__main__":
logger = trt.Logger(trt.Logger.WARNING)
with open("rtdetr-l.engine", "rb") as f, trt.Runtime(logger) as runtime:
engine = runtime.deserialize_cuda_engine(f.read())
context = engine.create_execution_context()
inputs_host = cuda.pagelocked_empty(trt.volume(context.get_binding_shape(0)), dtype=np.float32)
outputs_host = cuda.pagelocked_empty(trt.volume(context.get_binding_shape(1)), dtype=np.float32)
inputs_device = cuda.mem_alloc(inputs_host.nbytes)
outputs_device = cuda.mem_alloc(outputs_host.nbytes)
stream = cuda.Stream()
image = cv2.imread('bus.jpg', -1)
input = letterbox(image, input_shape)
input = input[:, :, ::-1].transpose(2, 0, 1).astype(dtype=np.float32) #BGR2RGB和HWC2CHW
input = input / 255.0
input = np.expand_dims(input, axis=0)
np.copyto(inputs_host, input.ravel())
with engine.create_execution_context() as context:
cuda.memcpy_htod_async(inputs_device, inputs_host, stream)
context.execute_async_v2(bindings=[int(inputs_device), int(outputs_device)], stream_handle=stream.handle)
cuda.memcpy_dtoh_async(outputs_host, outputs_device, stream)
stream.synchronize()
boxes = filter_box(outputs_host.reshape(context.get_binding_shape(1)))
draw(image, boxes)
cv2.imwrite('result.jpg', image)
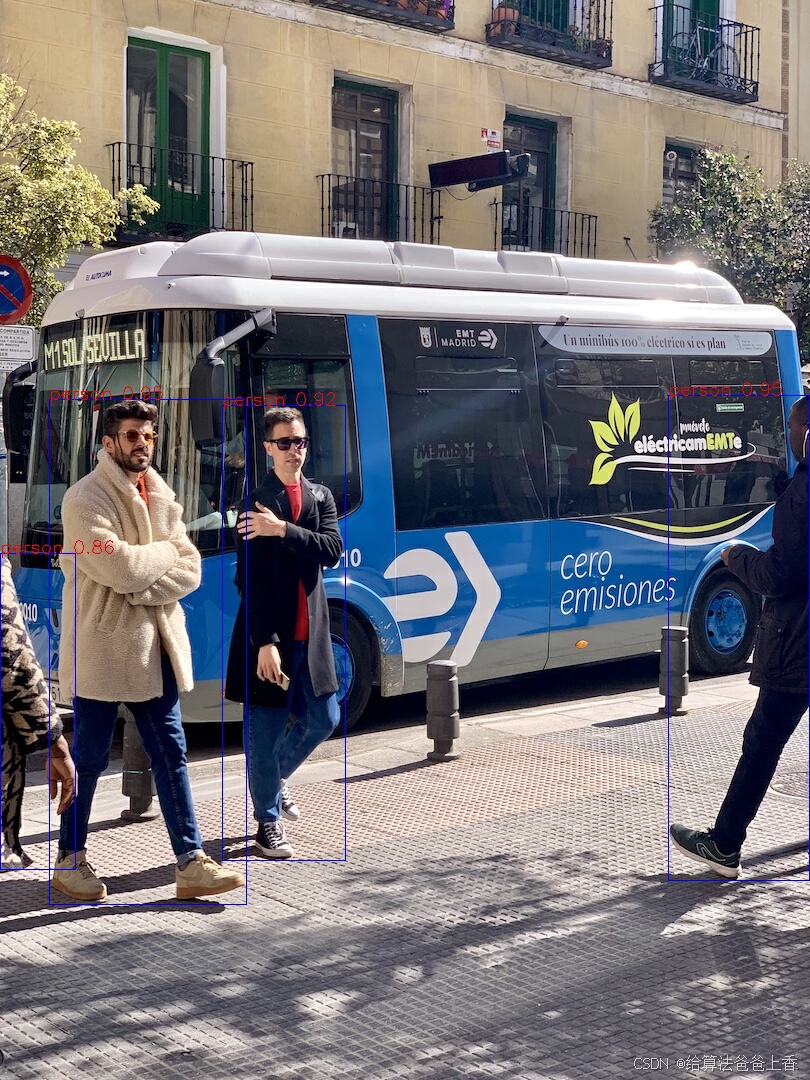