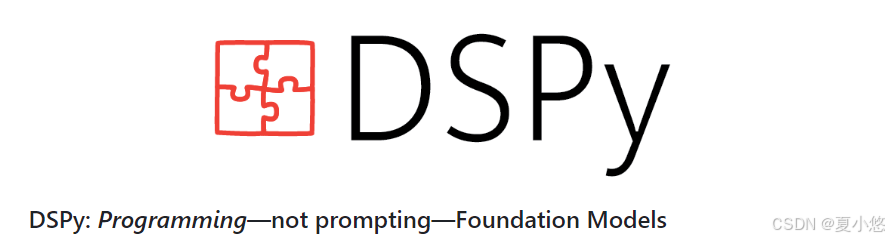
文章目录
-
- [1. 背景](#1. 背景)
- [2. 签名](#2. 签名)
- [3. 模块](#3. 模块)
-
- [3.1 预测模块](#3.1 预测模块)
- [3.2 其他内置模块](#3.2 其他内置模块)
- [4. 提词器](#4. 提词器)
- [5. 评估目标](#5. 评估目标)
- [6. 代码分析](#6. 代码分析)
-
- [6.1 _prepare_student_and_teacher](#6.1 _prepare_student_and_teacher)
- [6.2 _prepare_predictor_mappings](#6.2 _prepare_predictor_mappings)
- [6.3 _bootstrap](#6.3 _bootstrap)
-
- [6.3.1 构造prompt](#6.3.1 构造prompt)
- [6.3.2 送入LLM](#6.3.2 送入LLM)
- [6.3.3 解析响应结果](#6.3.3 解析响应结果)
- [6.4 _train](#6.4 _train)
- [7. 完整代码](#7. 完整代码)
- 预告
1. 背景
想让 LLM 完成一个任务,需要手动设计 prompt,但 LLM 对 prompt 比较敏感,稍有变动就会导致不同的结果,反复试验会将 prompt 变得越来越长,这对 LLM 在特定任务上的优化带来了极大的挑战。
DSPy 的目的就是根据任务的样例数据,自动优化 prompt,即任务数据+代码=适用该任务的 prompt。
DSPy 引入了三个抽象概念(编程模型范式):
-
Signature
(签名):抽象模块的输入/输出行为。
-
Module
(模块): 取代现有的手动提示技术,并且可以在任意管道中组合。
-
Teleprompter
(提词器): 优化管道中的所有模块以最大化指标。
注:本博客使用的
dspy-ai
版本为2.5.3
.
2. 签名
DSPy 签名是函数的自然语言类型声明,一个简短的声明性规范,告诉 DSPy 文本转换需要做什么(what)
,而不是提示 LLM 该如何做(how)
。
DSPy 签名是 input fields
和 output fields
以及一个可选的 instruction
组成的元组。一个字段由字段名称和可选的元数据组成。字段的角色由 DSPy 根据字段名称推断。例如,DSPy 编译器将使用上下文学习来解释不同地问题和答案,并会迭代地完善它对这些字段的使用。
python
class BasicQA(dspy.Signature):
"""Answer questions with short factoid answers."""
question = dspy.InputField(desc="question about something")
answer = dspy.OutputField(desc="often between 1 and 5 words")
签名比提示有两个好处:它们可以被编译成自适应和适应管道的提示或微调。这主要是通过为每个签名引导有用的示例来实现的。此外,它们处理结构化的格式和解析逻辑,以减少(或理想情况下,避免)用户程序中脆弱的字符串操作。
实际使用时,也可以使用简写方法(符号表示法):
python
qa = dspy.Predict("question -> answer")
qa(question="Where is Guaranı spoken?" )
# Out: Prediction(answer="Guaranı is spoken mainly in South America.")
在符号表示法中,每个字段的名称表明了输入(或输出)字段在转换中扮演的语义角色。DSPy 将解析这个符号表示法,并为 LM 扩展字段名称,以便english_document -> french_translation
会提示进行英语到法语的翻译。如果需要,DSPy 还提供了更高级的编程接口来表达对签名的更明确的约束。
当需要更多控制时,可以将签名表示为 Python 类,以提供转换的明确说明,并更直接地描述每个字段的格式或角色。例如,以下签名使用 context 和可选 question 生成搜索查询:
python
class GenerateSearchQuery(dspy.Signature):
"""Write a simple search query that will help answer a complex question."""
context = dspy.InputField(desc="may contain relevant facts")
question = dspy.InputField()
query = dspy.OutputField(dtype=dspy.SearchQuery)
使用上述签名,可以指定一个完整的系统,用于生成合成信息检索数据集,其中查询由 LLM 生成的中间问题产生:
python
query_gen = dspy.Predict(GenerateSearchQuery)
query_gen(context="Language typology")
# Out: Prediction(question="What are the main types of language classification?", query="`language classification` OR `language typology` -wikipedia")
如果提供了问题,可以像下面这样提供:
python
query_gen(context="Language typology", question="What are the primary language families of South America?")
用户可以可选地指定输出字段的类型为 bool、int、float、list 或 dict,而不是默认的字符串类型,如 contexts, question -> answer_found: bool
。
3. 模块
DSPy 签名简单地定义了一个接口,并提供了关于预期行为的类型提示。要使用一个签名,我们必须声明一个具有该签名的模块,就像我们在上面实例化了一个 Predict 模块一样。这样的模块声明返回一个具有该签名的函数。
3.1 预测模块
在 DSPy 中处理签名的核心模块是 Predict。在内部,Predict 存储了提供的签名、一个可选的 LM(最初为 None,但会覆盖此模块的默认 LM),以及用于提示的演示列表(最初为空)。像 PyTorch 中的层一样,实例化的模块表现为一个可调用的函数:它接受与签名输入字段相对应的关键字参数(例如 question),格式化一个实现签名的提示,包括适当的演示、调用 LM,并解析输出字段。当 Predict 检测到它在编译(compile)模式下使用时,它还将在内部跟踪输入/输出跟踪,以协助 teleprompter 进行引导。
3.2 其他内置模块
DSPy 模块将提示技术转换为支持任何签名的模块化函数,这与使用任务特定细节(例如,手写的少数示例)提示 LM 的标准方法形成鲜明对比。为此,DSPy 包括一些更复杂的模块,如ChainOfThought、ProgramOfThought、MultiChainComparison 和 ReAct,这些都可以互换使用,以实现 DSPy 签名。例如,简单地将上面的 Predict 更改为 ChainOfThought,就会得到一个逐步思考然后才允许其输出字段的系统。
重要的是,所有这些模块都以几行代码实现,通过扩展用户定义的签名并根据需要在新签名上多次调用 Predict。例如,下面展示了内置 ChainOfThought 的简化实现:
python
class ChainOfThought(dspy.Module):
def __init__(self, signature):
# Modify signature from `*inputs -> *outputs` to `*inputs -> rationale , *outputs`.
rationale_field = dspy.OutputField(prefix="Reasoning: Let's think step by step.")
signature = dspy.Signature(signature).prepend_output_field(rationale_field)
# Declare a sub-module with the modified signature.
self.predict = dspy.Predict(signature)
def forward(self, **kwargs):
# Just forward the inputs to the sub-module.
return self.predict(**kwargs)
4. 提词器
当编译 DSPy 程序时,我们通常调用一个 teleprompter,这是一个优化器,它接受程序、训练集和度量标准,并返回一个新的优化程序。不同的 teleprompters 采用不同的优化策略。
在 DSPy 中,训练集可能很小,可能只有几个例子,尽管更大的数据可以支持更强大的优化。训练示例可能是不完整的,即只需要输入值。除非它们需要在度量标准中使用,否则不需要标签。
度量标准可以是简单的概念,如精确匹配(EM)或 F1,但它们也可以是平衡多个关注点的完整 DSPy 程序。
例如,我们可以根据问答对 qa_trainset 数据集 和度量 EM 编译上面的 RAG 模块:
python
# Small training set with only questions and final answers.
qa_trainset = [dspy.Example(question="What is the capital of France?", answer="Paris")]
# The teleprompter will bootstrap missing labels: reasoning chains and retrieval contexts.
teleprompter = dspy.BootstrapFewShot(metric=dspy.evaluate.answer_exact_match)
compiled_rag = teleprompter.compile(RAG(), trainset=qa_trainset)
在这个例子中,BootstrapFewShot(teleprompter) 模拟了训练示例中的 RAG。它将收集每个模块的示范(demonstrations)(即其输入-输出行为的示例),这些示范共同导致有效输出(即尊重签名和度量标准)。
如果想根据检索到的上下文将编译后的程序推送为提取程序,则可以自定义一个指标来代替 dspy.evaluate.answer_exact_match
:
python
def answer_and_context_match(example , pred , trace=None):
answer_match = dspy.evaluate.answer_exact_match(example, pred)
# Is the prediction a substring of some passage?
context_match = any((pred.answer.lower() in c) for c in pred.context)
return answer_match and context_match
teleprompter也可以通过指定教师程序来组合,适用教师模型(LLM)来微调学生模型(小LLM),将 BootstrapFewShot 改为 BootstrapFinetune,这里不再介绍。
编译时会经历三个阶段:
-
候选生成:编译器会递归地找到程序中所有唯一的预测模块(预测器),包括那些嵌套在其他模块下的预测器。对于每个唯一的预测器 p p p,teleprompter 可以为 p p p的参数生成候选值:指令、字段描述或示例(即输入-输出对)。在当前版本中更专注于示例,并发现简单的拒绝采样方法可以帮助引导高效的多级系统。
-
参数优化:许多超参数调整算法(例如,随机搜索或 HyperOpt 和 Optuna 中的树结构 Parzen 估计器)可以应用于候选值的选择。另一种优化类型是使用 BootstrapFinetune 进行微调,其中示例用于更新每个预测器的 LM 权重。当应用此方法时,每个模块的 LM 参数将更新为新的 LM 权重。通常,我们使用度量标准和交叉验证来优化训练集或验证集上的平均质量。
-
更高阶程序优化:DSPy 编译器支持的另一种优化类型是修改程序的控制流。其中最简单的形式之一是集成,集成将引导同一程序的多个副本,然后将程序替换为一个新程序,该程序并行运行所有副本,并通过自定义函数(例如,多数投票)将它们的预测合并为一个。在未来的工作中,这个阶段可以轻松适应更多动态(即测试时)引导以及自动回溯逻辑的技术。
5. 评估目标
编程框架可以从多个维度进行评估:计算效率、开发者效率、代码和概念的直观性等等。在这篇论文中,作者专注于当前 LM 流水线可能面临的最紧迫问题:手工编写的任务特定提示在实现高性能系统中的作用。评估旨在测试以下假设:
-
使用DSPy,我们可以用简洁且定义良好的模块替换手工制作的提示字符串,而不会降低质量或表达能力。
-
通过将模块参数化并将提示视为优化问题,DSPy 能更好地适应不同的 LM,并且可能胜过专家编写的提示。
-
由此产生的模块化使得更彻底地探索具有有用性能特征或符合细微度量标准的复杂流水线成为可能。
作者的评估将使用多样的"task--program pairs"
来探索这些假设。希望这能从一个转变开始,即从 不同 LM 在任务 T 上的表现如何 这样的未明确指定的问题,转向 在程序 P 上使用策略 S 编译时,它们在任务 T 上的表现如何,这是一个定义明确且可复现的运行。最终,我们的目标是减少现代 AI 中巧妙提示构造的作用,转而支持新模块化、可组合程序和优化器的发展。
6. 代码分析
本文以地域识别任务为例,使用BootstrapFewShot.compile
进行编译优化,来分析一下 PSPy 的优化流程:
python
def compile(self, student, *, teacher=None, trainset):
self.trainset = trainset
self._prepare_student_and_teacher(student, teacher)
self._prepare_predictor_mappings()
self._bootstrap()
self.student = self._train()
self.student._compiled = True
# set assert_failures and suggest_failures as attributes of student w/ value 0
self.student._assert_failures = 0
self.student._suggest_failures = 0
return self.student
6.1 _prepare_student_and_teacher
在当前示例中,student 为 RAG 实例,teacher 默认设置为 None,在 _prepare_student_and_teacher
函数中,会将 student 复制一份作为teacher的初始值,然后对 teacher 进行编译优化,具体为:
python
teleprompter = LabeledFewShot(k=self.max_labeled_demos)
self.teacher = teleprompter.compile(self.teacher.reset_copy(), trainset=self.trainset)
这里的 compile 主要是对 teacher 随机采样k各训练数据:
python
predictor.demos = rng.sample(self.trainset, min(self.k, len(self.trainset)))
6.2 _prepare_predictor_mappings
这里主要是获得两个数据:name2predictor和predictor2name。
python
name2predictor, predictor2name = {}, {}
for (name1, predictor1), (name2, predictor2) in zip(student.named_predictors(), teacher.named_predictors()):
name2predictor[name1] = None # dict(student=predictor1, teacher=predictor2)
predictor2name[id(predictor1)] = name1
predictor2name[id(predictor2)] = name2
self.name2predictor = name2predictor
self.predictor2name = predictor2name
6.3 _bootstrap
在这个函数里面对数据进行采样,遍历 trainset 数据集中的每个数据,将这条数据送入到 LLM,如果 LLM 给出的答案与 ground truth 一致,则记录其 idx,然后从未预测正确的训练集中随机采样,作为 validation 数据集。
LLM 的预测及评估的流程在 _bootstrap_one_example
函数中,先来看下 LLM 的预测流程:
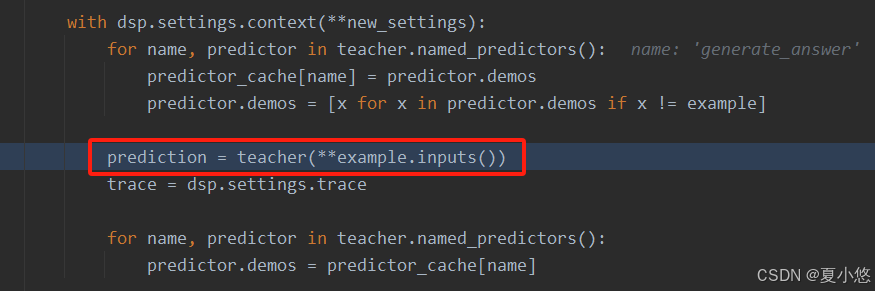
具体执行流程是:
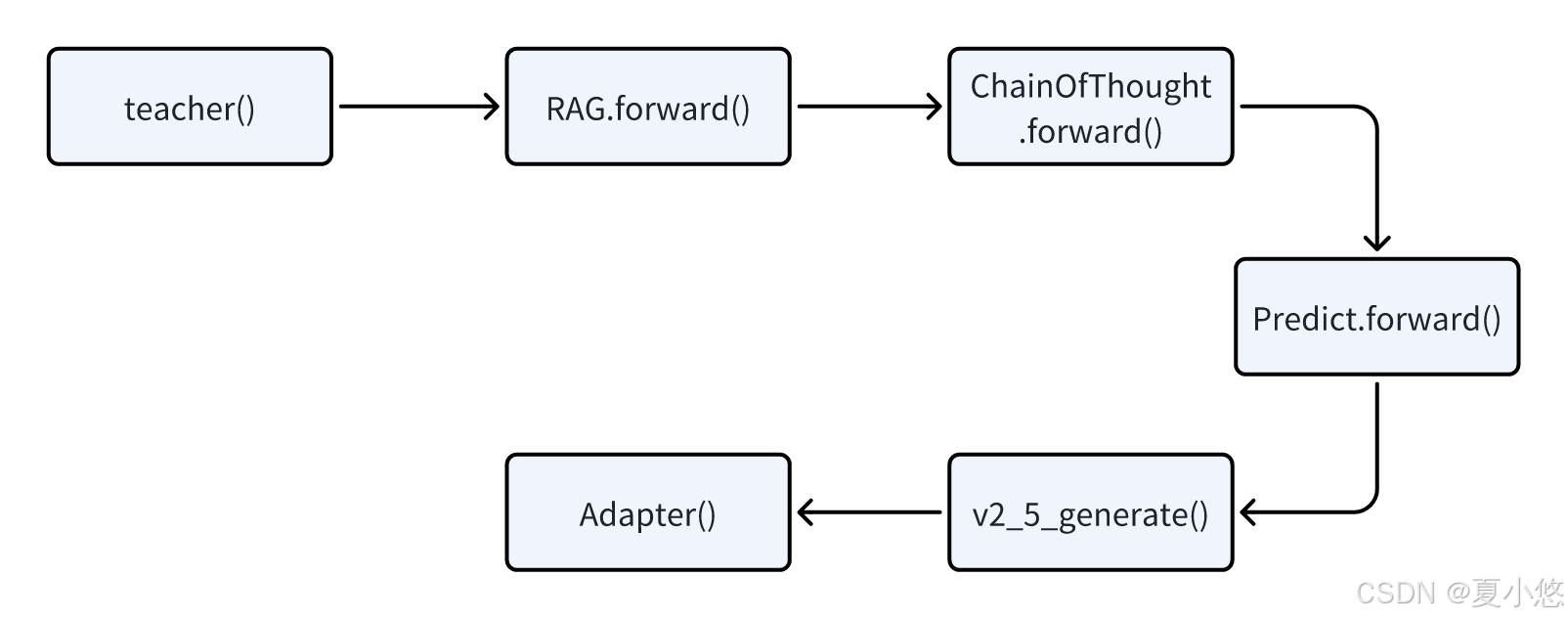
在Adapter中分为三部分:构造prompt、送入LLM、解析LLM的响应。
6.3.1 构造prompt
代码位置:ChatAdapter.format()
-
Step1:
根据签名来构建 system 指令:messages.append({"role": "system", "content": prepare_instructions(signature)})
python
# prepare_instructions()
parts = [
'Your input fields are:\n1. `question` (str): question about something',
'Your output fields are:\n1. `reasoning` (str)\n2. `answer` (str): default: none',
'All interactions will be structured in the following way, with the appropriate values filled in.',
'[[ ## question ## ]]\n{question}',
'[[ ## reasoning ## ]]\n{reasoning}\n\n[[ ## answer ## ]]\n{answer}',
'[[ ## completed ## ]]',
'In adhering to this structure, your objective is: \n Recognize location from question and give the reasoning for the same.'
]
return '\n\n'.join(parts).strip()
-
Step2:
根据签名和随机采样的数据(demos)来构建 user 指令:messages.append({"role": "user", "content": format_turn(signature, example)})
python
# format_turn()
content = [
'This is an example of the task, though some input or output fields are not supplied.',
'[[ ## question ## ]]\n美国留学回来',
'Respond with the corresponding output fields, starting with the field `reasoning`, then `answer`, and then ending with the marker for `completed`.'
]
return '\n\n'.join(content).strip()
-
Step3:
根据签名和随机采样的数据(demos)来构建 assistant 指令:messages.append({"role": "assistant", "content": format_turn(signature, example)})
python
# format_turn()
content = [
'[[ ## reasoning ## ]]\nNot supplied for this particular example.\n\n[[ ## answer ## ]]\n美国\n\n[[ ## completed ## ]]'
]
return '\n\n'.join(content).strip()
-
Step4:
根据签名和输入数据来构建 user 指令:messages.append({"role": "user", "content": format_turn(signature, inputs)})
python
# format_turn()
content = [
'[[ ## question ## ]]\n担任中职学校班级班主任,是否需要具备教师资格证?',
'Respond with the corresponding output fields, starting with the field `reasoning`, then `answer`, and then ending with the marker for `completed`.'
]
return '\n\n'.join(content).strip()
6.3.2 送入LLM
DSPy 使用litellm.completion
来完成 LLM-API 的调用。
6.3.3 解析响应结果
将 messages 数据传入 LLM,得到响应结果:
python
ModelResponse(
id='chat-51cf2a4062ef4801bd40e281d600b5ff',
choices=[Choices(
finish_reason='stop',
index=0,
message=Message(
content='[[ ## reasoning ## ]]\nNot supplied for this particular example.\n\n[[ ## answer ## ]]\nnone\n\n[[ ## completed ## ]]',
role='assistant',
tool_calls=None,
function_call=None))],
created=1728648483,
model='qwen2dot5-32b-4bit',
object='chat.completion',
system_fingerprint=None,
usage=Usage(completion_tokens=25, prompt_tokens=1613, total_tokens=1638, completion_tokens_details=None, prompt_tokens_details=None),
service_tier=None,
prompt_logprobs=None
)
从响应结果中解析出 reasoning 和 answer,然后在Predict.forward()
函数中将结果封装到一个 Prediction 实例中,并返回。
评估流程主要是验证预测结果是否正确,依据是自定义的validate_answer()
函数,这里采用的是精确匹配,即将预测的结果与 ground truth 进行比较,看看结果是否一致。
如果验证结果正确,则将这个训练数据存入到 name2traces 中,作为 augmented_demos:
python
if success:
for step in trace:
predictor, inputs, outputs = step
demo = Example(augmented=True, **inputs, **outputs)
try:
predictor_name = self.predictor2name[id(predictor)]
except KeyError:
continue
name2traces[predictor_name].append(demo)
6.4 _train
从 validation 数据集中随机采样数据,并与 augmented_demos 中的数据合并在一起,作为 student 的 demos 数据:
python
rng = random.Random(0)
raw_demos = self.validation
for name, predictor in self.student.named_predictors():
augmented_demos = self.name2traces[name][: self.max_bootstrapped_demos]
sample_size = min(self.max_labeled_demos - len(augmented_demos), len(raw_demos))
sample_size = max(0, sample_size)
raw_demos = rng.sample(raw_demos, sample_size)
predictor.demos = augmented_demos + raw_demos
分析到这,真是离谱他妈给离谱开门,离谱到家了,这训练了啥,这不妥妥的就是内置prompt
模板+fewshot
来构造prompt
吗???
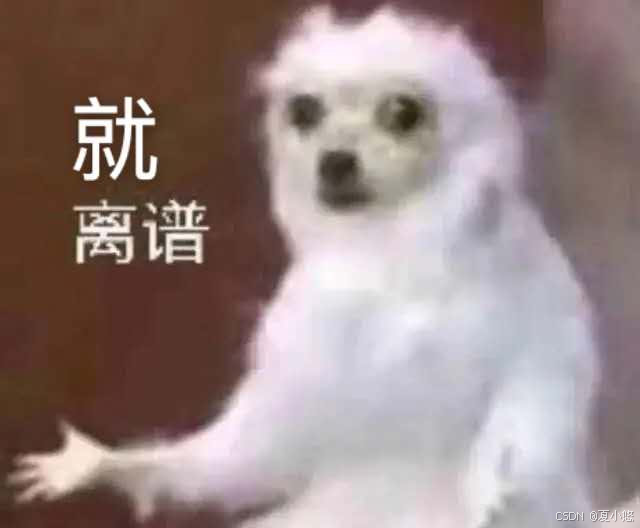
7. 完整代码
python
# -*- coding: utf-8 -*-
# Author : liyanpeng
# Email : [email protected]
# Datetime: 2024/9/29 15:03
# Filename: location.py
import dspy
from dspy.teleprompt import BootstrapFewShot, BootstrapFewShotWithRandomSearch, BootstrapFewShotWithOptuna, KNNFewShot
from dspy import Example
import pandas as pd
import random
class BuildDataset:
def __init__(self):
self.xlsx_path = 'data/location_data.xlsx'
random.seed(10086)
def build(self, val_ratio=0.2):
df = pd.read_excel(self.xlsx_path, dtype=str)
data_list = []
for row in df.values:
question, answer = row
data_list.append(Example(question=question, answer=answer).with_inputs('question'))
random.shuffle(data_list)
val_num = int(len(data_list) * val_ratio) + 1
return data_list[:-val_num], data_list[-val_num:]
class CoTSignature(dspy.Signature):
"""Recognize location from question and give the reasoning for the same."""
question = dspy.InputField(desc="question about something")
answer = dspy.OutputField(desc="default: none")
class CoTPipeline(dspy.Module):
def __init__(self, signature):
super().__init__()
self.signature = signature
self.predictor = dspy.ChainOfThought(self.signature)
def forward(self, question):
prediction = self.predictor(question=question)
return dspy.Prediction(reasoning=prediction.reasoning, answer=prediction.answer)
def validate_answer(example, pred, trace=None):
answer_EM = dspy.evaluate.answer_exact_match(example, pred)
return answer_EM
if __name__ == '__main__':
data_builder = BuildDataset()
trainset, valset = data_builder.build()
llm_model = dspy.LM(model='openai/qwen2dot5-32b-4bit', api_base='http://192.168.3.21:11026/v1', api_key='none',
cache=False)
dspy.settings.configure(lm=llm_model)
teleprompter = BootstrapFewShot(metric=validate_answer,
max_bootstrapped_demos=4,
max_rounds=1)
# teleprompter = BootstrapFewShotWithRandomSearch(metric=validate_answer,
# max_bootstrapped_demos=4,
# max_rounds=1)
compiled_cot = teleprompter.compile(CoTPipeline(CoTSignature), trainset=trainset)
test_example = '大洛阳有好玩的地方吗?'
cot = dspy.ChainOfThought(CoTSignature)
pred = cot(question=test_example)
print("[uncompiled]Question --> Reasoning --> Predicted Answer")
print(f"{test_example} --> {pred.reasoning} --> {pred.answer}")
pred = compiled_cot(question=test_example)
print("[compiled]Question --> Reasoning --> Predicted Answer")
print(f"{test_example} --> {pred.reasoning} --> {pred.answer}")
运行结果:
bash
30%|█████████████▏ | 6/20 [00:23<00:54, 3.86s/it]
Bootstrapped 4 full traces after 7 examples in round 0.
[uncompiled]Question --> Reasoning --> Predicted Answer
大洛阳有好玩的地方吗? --> 从问题中,我们可以看到关键词"大洛阳",这表明问题是关于洛阳这个城市。洛阳是中国河南省的一个城市,有着丰富的历史和文化遗迹,因此问题询问的是洛阳是否有好玩的地方。 --> 洛阳有很多好玩的地方,比如龙门石窟、白马寺等。
[compiled]Question --> Reasoning --> Predicted Answer
大洛阳有好玩的地方吗? --> 问题中提到的"大洛阳"暗示了地点是在中国河南省的洛阳市。 --> 洛阳
预告
其实啊,在这篇论文中没有提高,另外一篇 DSPy 的论文及项目中有介绍,就是 COPRO 和 MIPROv2 这两个优化器,他们会根据任务的不同动态提出(微调)指令,这些内容将在下一篇博客中介绍。