分类任务
- 网络基本构建与训练方法,常用函数解
- torch.nn.functional模块
- nn.Module模块
MNIST数据集下载
python
from pathlib import Path
import requests
DATA_PATH = Path("data")
PATH = DATA_PATH / "mnist"
PATH.mkdir(parents=True, exist_ok=True)
URL = "http://deeplearning.net/data/mnist/"
FILENAME = "mnist.pkl.gz"
if not (PATH / FILENAME).exists():
content = requests.get(URL + FILENAME).content
(PATH / FILENAME).open("wb").write(content)
解压数据集
python
import pickle
import gzip
with gzip.open((PATH / FILENAME).as_posix(), "rb") as f:
((x_train, y_train), (x_valid, y_valid), _) = pickle.load(f, encoding="latin-1")
查阅数据
python
from matplotlib import pyplot
import numpy as np
pyplot.imshow(x_train[0].reshape((28, 28)), cmap="gray")
print(x_train.shape)
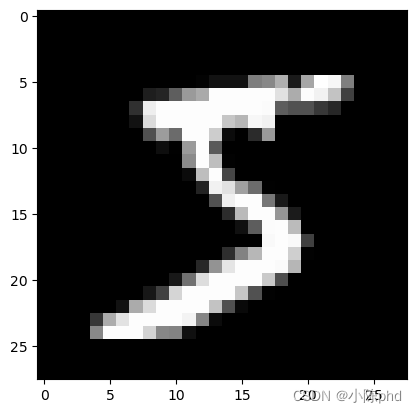
网络模型搭建
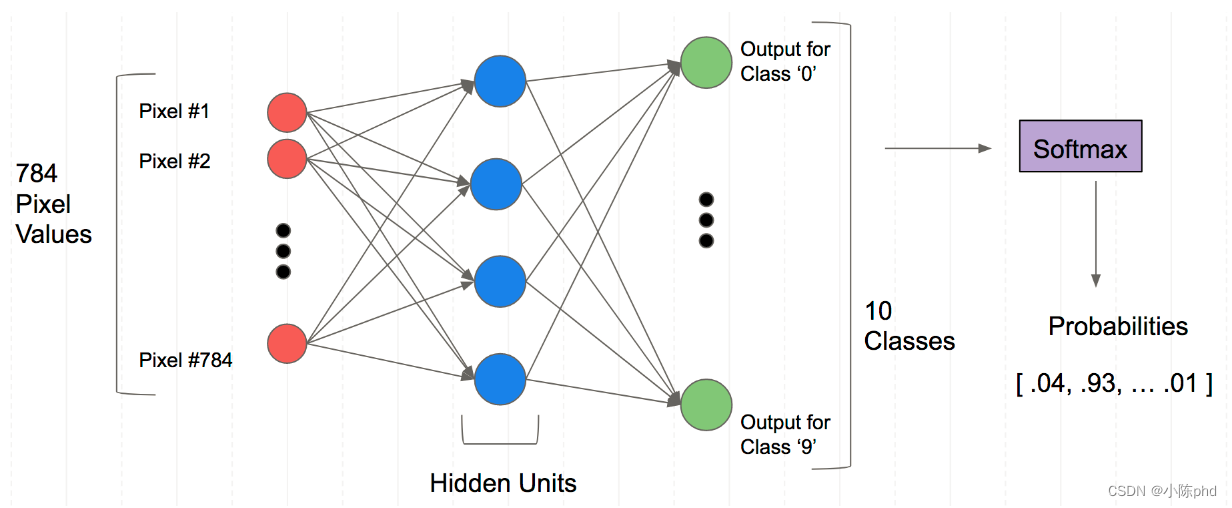
python
import torch
x_train, y_train, x_valid, y_valid = map(
torch.tensor, (x_train, y_train, x_valid, y_valid)
)
n, c = x_train.shape
x_train, x_train.shape, y_train.min(), y_train.max()
print(x_train, y_train)
print(x_train.shape)
print(y_train.min(), y_train.max())
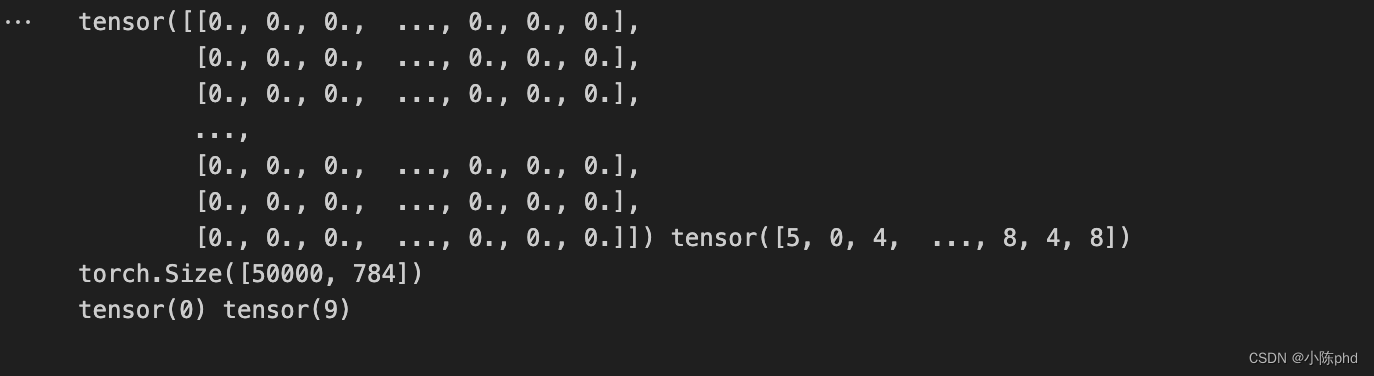
常用函数介绍
python
import torch.nn.functional as F
loss_func = F.cross_entropy
def model(xb):
return xb.mm(weights) + bias
bs = 64
xb = x_train[0:bs] # a mini-batch from x
yb = y_train[0:bs]
weights = torch.randn([784, 10], dtype = torch.float, requires_grad = True)
bs = 64
bias = torch.zeros(10, requires_grad=True)
print(loss_func(model(xb), yb))
模型搭建
python
from torch import nn
class Mnist_NN(nn.Module):
def __init__(self):
super().__init__()
self.hidden1 = nn.Linear(784, 128)
self.hidden2 = nn.Linear(128, 256)
self.out = nn.Linear(256, 10)
def forward(self, x):
x = F.relu(self.hidden1(x))
x = F.relu(self.hidden2(x))
x = self.out(x)
return x
net = Mnist_NN()
print(net)
Mnist_NN(
(hidden1): Linear(in_features=784, out_features=128, bias=True)
(hidden2): Linear(in_features=128, out_features=256, bias=True)
(out): Linear(in_features=256, out_features=10, bias=True)
)
python
for name, parameter in net.named_parameters():
print(name, parameter,parameter.size())
dataset数据接口
python
from torch.utils.data import TensorDataset
from torch.utils.data import DataLoader
train_ds = TensorDataset(x_train, y_train)
train_dl = DataLoader(train_ds, batch_size=bs, shuffle=True)
valid_ds = TensorDataset(x_valid, y_valid)
valid_dl = DataLoader(valid_ds, batch_size=bs * 2)
def get_data(train_ds, valid_ds, bs):
return (
DataLoader(train_ds, batch_size=bs, shuffle=True),
DataLoader(valid_ds, batch_size=bs * 2),
)
- 一般在训练模型时加上model.train(),这样会正常使用Batch Normalization和 Dropout
- 测试的时候一般选择model.eval(),这样就不会使用Batch Normalization和 Dropout
python
import numpy as np
from torch import optim
def fit(steps, model, loss_func, opt, train_dl, valid_dl):
for step in range(steps):
model.train()
for xb, yb in train_dl:
loss_batch(model, loss_func, xb, yb, opt)
model.eval()
with torch.no_grad():
losses, nums = zip(
*[loss_batch(model, loss_func, xb, yb) for xb, yb in valid_dl]
)
val_loss = np.sum(np.multiply(losses, nums)) / np.sum(nums)
print('当前step:'+str(step), '验证集损失:'+str(val_loss))
def get_model():
model = Mnist_NN()
return model, optim.SGD(model.parameters(), lr=0.001)
def loss_batch(model, loss_func, xb, yb, opt=None):
loss = loss_func(model(xb), yb)
if opt is not None:
loss.backward()
opt.step()
opt.zero_grad()
return loss.item(), len(xb)
train_dl, valid_dl = get_data(train_ds, valid_ds, bs)
model, opt = get_model()
fit(25, model, loss_func, opt, train_dl, valid_dl)