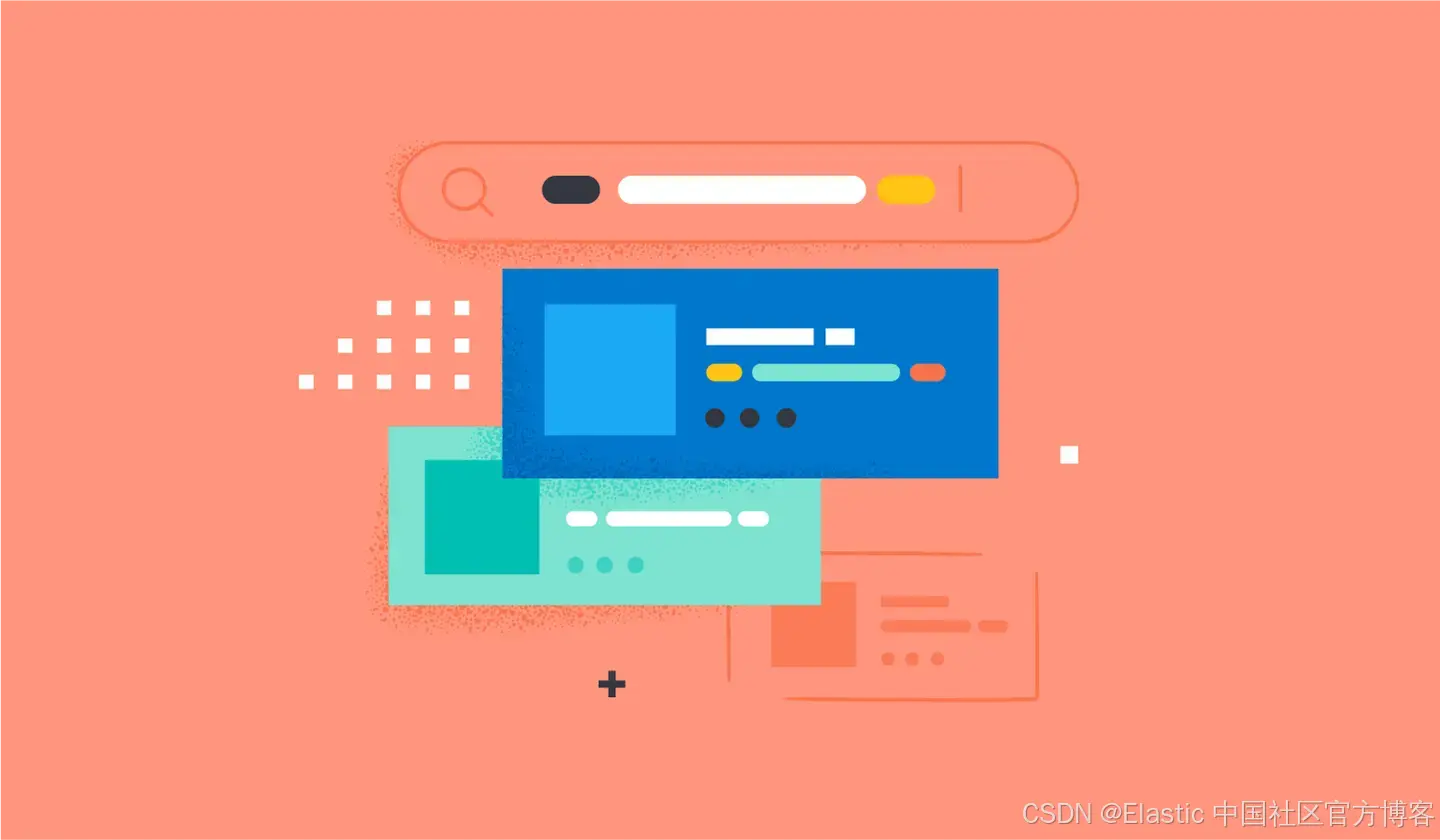
这篇文章是之前的文章 "使用 Elasticsearch 构建食谱搜索(一)" 的续篇。在这篇文章中,我将详述如何使用本地 Elasticsearch 部署来完成对示例代码的运行。该项目演示了如何使用 Elastic 的 ELSER 实现语义搜索并将其结果与传统的词汇搜索进行比较。项目目标
- 配置 Elasticsearch 基础架构以支持语义和词汇搜索索引。
- 数据提取:使用 Python 脚本用杂货产品数据填充索引。
- 比较搜索类型:执行搜索并显示结果以供比较。
准备工作
安装 Elasticsearch 及 Kibana
如果你还没有安装好自己的 Elasticsearch 及 Kibana,请参考如下的文章来进行安装:
在安装的时候,我们可以选择 Elastic Stack 8.x 的安装指南来进行安装。在本博文中,我将使用最新的 Elastic Stack 8.10.4 来进行展示。
在安装 Elasticsearch 的过程中,我们需要记下如下的信息:
我们记下上面的信息。它们将在如下的配置中进行使用。
克隆代码
为了方便大家学习,请在如下的地址克隆代码,并运行:
git clone https://github.com/liu-xiao-guo/elasticsearch-labs
$ pwd
/Users/liuxg/python/elasticsearch-labs
$ cd supporting-blog-content/building-a-recipe-search-with-elasticsearch/
$ ls
README.md infra.py ingestion_lexical_index.py
elasticsearch_connection.py infra_lexical_index.py requirements.txt
files ingestion.py search.py
我们进入大上面的目录中,并拷贝 Elasticsearch 的证书到该目录中:
$ cp ~/elastic/elasticsearch-8.15.3/config/certs/http_ca.crt .
$ ls
README.md infra.py requirements.txt
elasticsearch_connection.py infra_lexical_index.py search.py
files ingestion.py
http_ca.crt ingestion_lexical_index.py
安装 ELSER 模型
我们可以访问文章 "Elasticsearch:部署 ELSER - Elastic Learned Sparse EncoderR" 来安装 ELSER 模型。最终我们可以看到部署的 ELSER 模型:
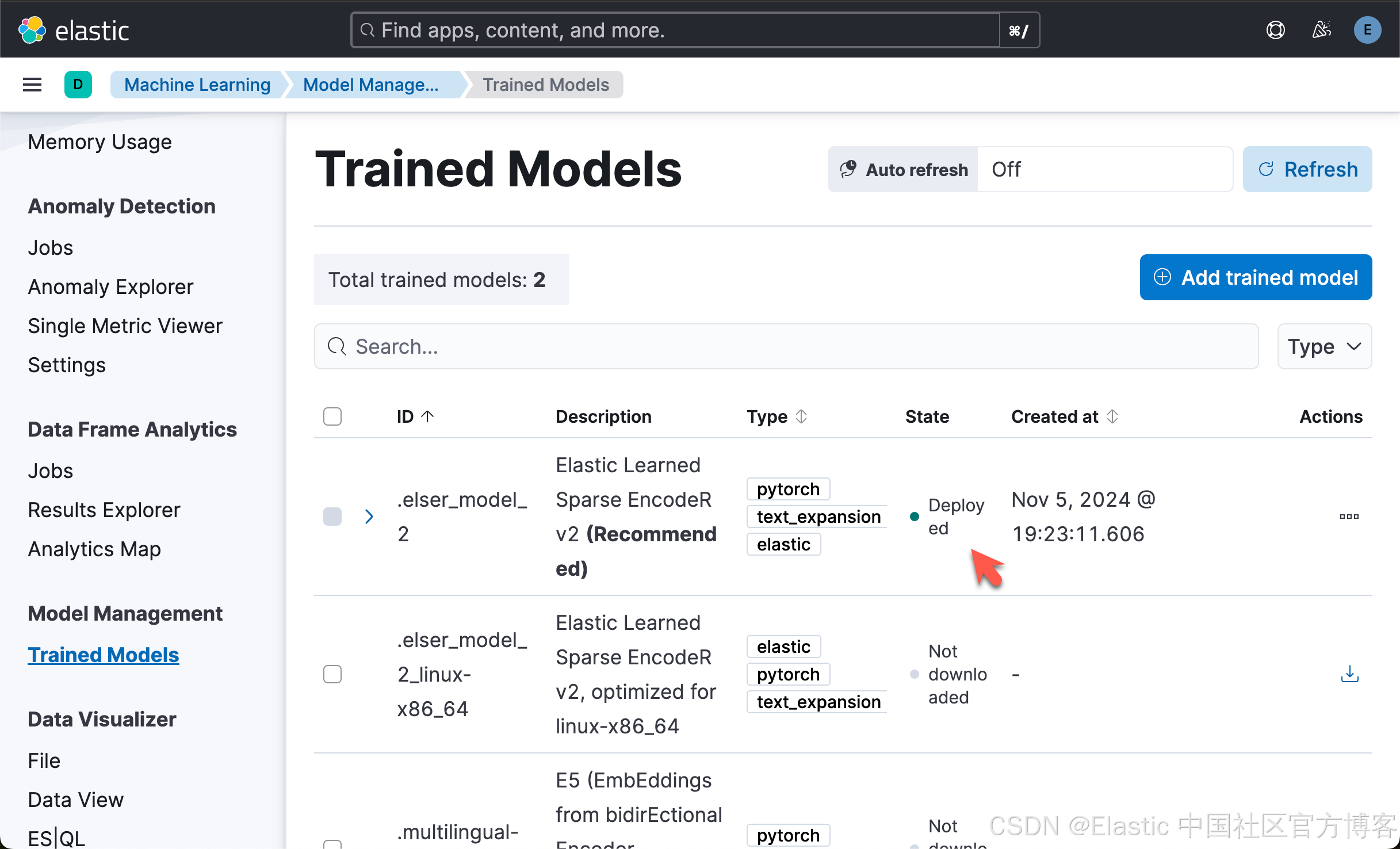
创建环境变量
我们在当前的目录中创建应用所需要的环境变量。我们创建一个文件 .env
.env
ES_USER="elastic"
ES_PASSWORD="DgmQkuRWG5RQcodxwGxH"
ES_ENDPOINT="localhost"
我们需要根据自己的 Elasticsearch 安装进行相应的修改。
$ pwd
/Users/liuxg/python/elasticsearch-labs/supporting-blog-content/building-a-recipe-search-with-elasticsearch
$ ls -al .env
-rw-r--r-- 1 liuxg staff 77 Nov 8 15:35 .env
运行代码
我们首先使用如下的命令来创建环境运行:
python3 -m venv .venv
$ pwd
/Users/liuxg/python/elasticsearch-labs/supporting-blog-content/building-a-recipe-search-with-elasticsearch
$ ls -al .env
-rw-r--r-- 1 liuxg staff 77 Nov 8 15:35 .env
$ python3 -m venv .venv
$ source .venv/bin/activate
(.venv) $
我们接着使用如下的命令来安装必须的库:
pip3 install -r requirements.txt
创建索引
要创建语义和词汇搜索索引,请运行以下脚本:
Semantic index
python infra.py
(.venv) $ python infra.py
{'name': 'liuxgm.local', 'cluster_name': 'elasticsearch', 'cluster_uuid': 'HF3DAYNQSnOq0D1NmsNubg', 'version': {'number': '8.16.0', 'build_flavor': 'default', 'build_type': 'tar', 'build_hash': '12ff76a92922609df4aba61a368e7adf65589749', 'build_date': '2024-11-08T10:05:56.292914697Z', 'build_snapshot': False, 'lucene_version': '9.12.0', 'minimum_wire_compatibility_version': '7.17.0', 'minimum_index_compatibility_version': '7.0.0'}, 'tagline': 'You Know, for Search'}
/Users/liuxg/python/elasticsearch-labs/supporting-blog-content/building-a-recipe-search-with-elasticsearch/infra.py:34: ElasticsearchWarning: Putting elasticsearch service inference endpoints (including elser service) without a model_id field is deprecated and will be removed in a future release. Please specify a model_id field.
response = client.inference.put(
/Users/liuxg/python/elasticsearch-labs/supporting-blog-content/building-a-recipe-search-with-elasticsearch/infra.py:34: ElasticsearchWarning: The [elser] service is deprecated and will be removed in a future release. Use the [elasticsearch] service instead, with [model_id] set to [.elser_model_2] in the [service_settings]
response = client.inference.put(
{'inference_id': 'elser_embeddings', 'task_type': 'sparse_embedding', 'service': 'elasticsearch', 'service_settings': {'num_allocations': 1, 'num_threads': 1, 'model_id': '.elser_model_2'}, 'chunking_settings': {'strategy': 'sentence', 'max_chunk_size': 250, 'sentence_overlap': 1}}
{'acknowledged': True, 'shards_acknowledged': True, 'index': 'grocery-catalog-elser'}
我们可以在 Kibana 中进行查看:
GET grocery-catalog-elser/_mapping
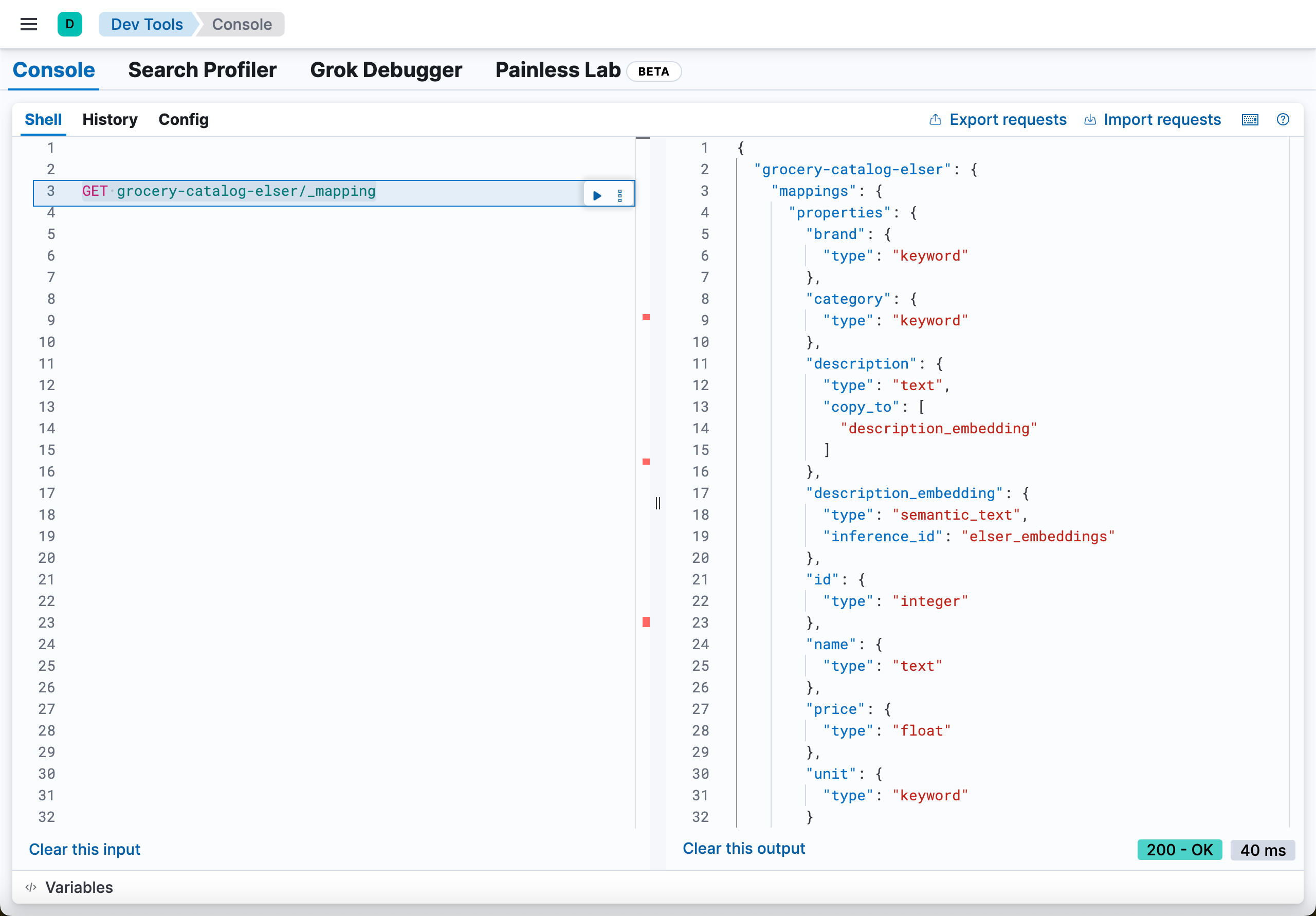
GET _inference/elser_embeddings
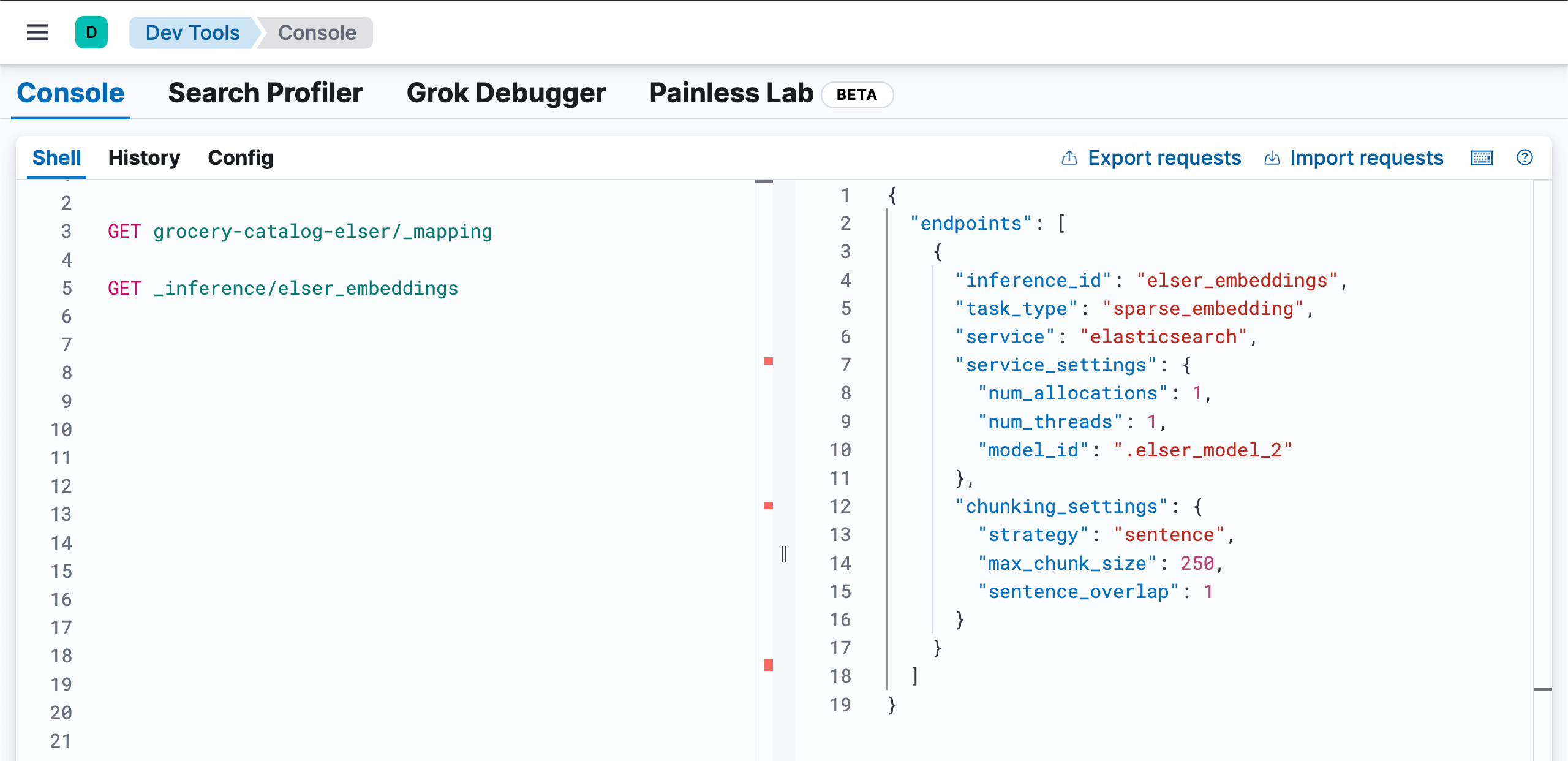
上面的代码生成索引及一个叫做 elser_embeddings 的 inference id。
词汇索引
我们运行如下的命令来生成词汇索引:
python infra_lexical_index.py
(.venv) $ python infra_lexical_index.py
{'name': 'liuxgm.local', 'cluster_name': 'elasticsearch', 'cluster_uuid': 'HF3DAYNQSnOq0D1NmsNubg', 'version': {'number': '8.16.0', 'build_flavor': 'default', 'build_type': 'tar', 'build_hash': '12ff76a92922609df4aba61a368e7adf65589749', 'build_date': '2024-11-08T10:05:56.292914697Z', 'build_snapshot': False, 'lucene_version': '9.12.0', 'minimum_wire_compatibility_version': '7.17.0', 'minimum_index_compatibility_version': '7.0.0'}, 'tagline': 'You Know, for Search'}
{'acknowledged': True, 'shards_acknowledged': True, 'index': 'grocery-catalog'}
上面的代码会创建一个叫做 grocery-catalog 的索引:
GET grocery-catalog/_mapping
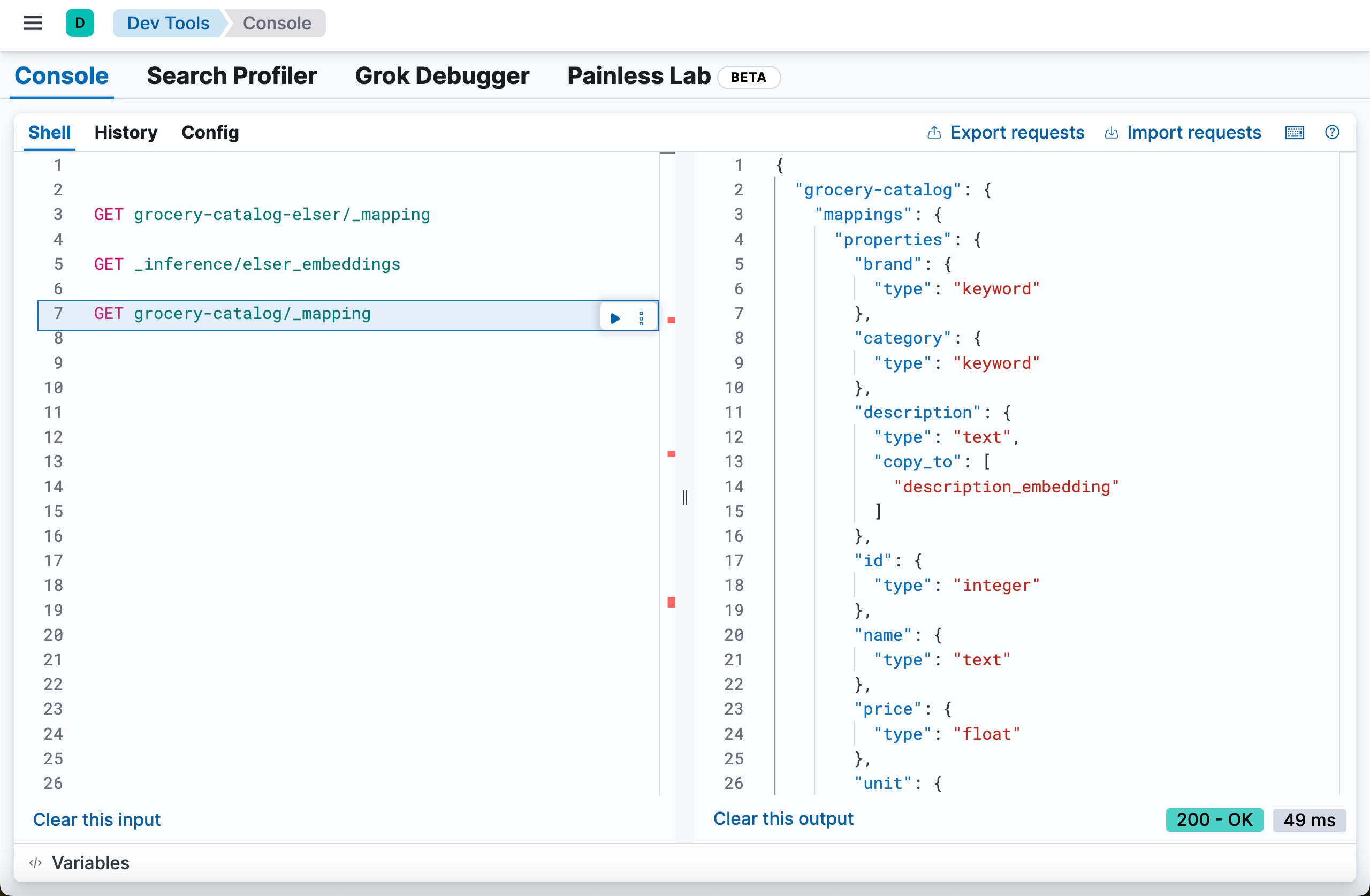
摄取数据
要将 recipe 数据纳入索引,请使用以下命令:
将数据导入语义索引
我们使用如下的命令:
python ingestion.py
(.venv) $ python ingestion.py
{'name': 'liuxgm.local', 'cluster_name': 'elasticsearch', 'cluster_uuid': 'HF3DAYNQSnOq0D1NmsNubg', 'version': {'number': '8.16.0', 'build_flavor': 'default', 'build_type': 'tar', 'build_hash': '12ff76a92922609df4aba61a368e7adf65589749', 'build_date': '2024-11-08T10:05:56.292914697Z', 'build_snapshot': False, 'lucene_version': '9.12.0', 'minimum_wire_compatibility_version': '7.17.0', 'minimum_index_compatibility_version': '7.0.0'}, 'tagline': 'You Know, for Search'}
partition 1
Successfully indexed 500 documents. Failed to index [] documents.
partition 2
Successfully indexed 500 documents. Failed to index [] documents.
partition 3
Successfully indexed 500 documents. Failed to index [] documents.
partition 4
Successfully indexed 257 documents. Failed to index [] documents.
我们可以通过如下的命令来进行查看已经写入的数据:
GET grocery-catalog-elser/_search
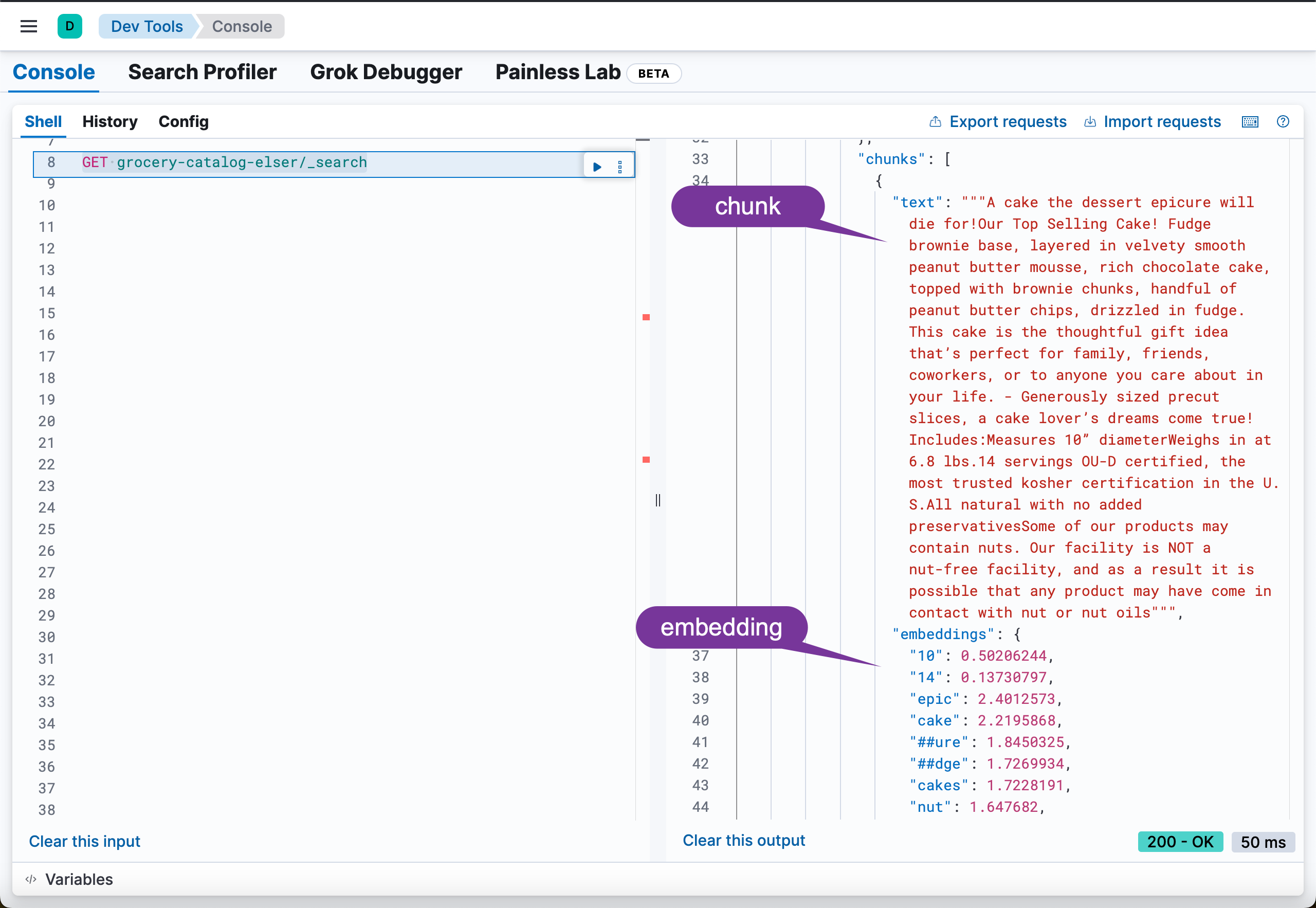
我们发现共有 2257 个文档:
GET grocery-catalog-elser/_count
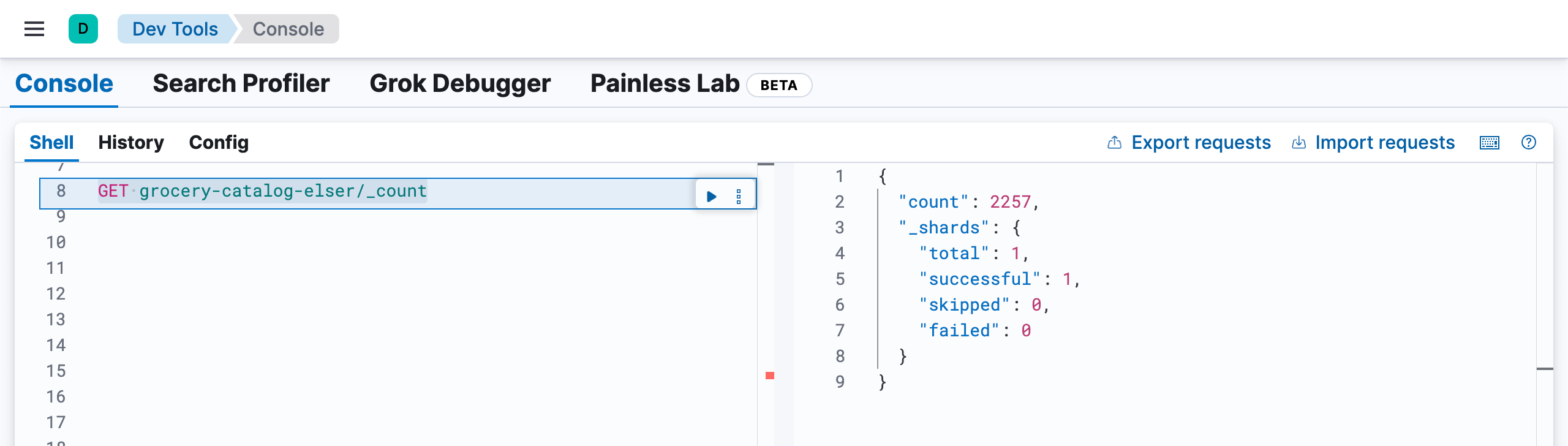
将数据导入词汇索引
我们使用如下的命令来写入数据:
python ingestion_lexical_index.py
(.venv) $ python ingestion_lexical_index.py
{'name': 'liuxgm.local', 'cluster_name': 'elasticsearch', 'cluster_uuid': 'HF3DAYNQSnOq0D1NmsNubg', 'version': {'number': '8.16.0', 'build_flavor': 'default', 'build_type': 'tar', 'build_hash': '12ff76a92922609df4aba61a368e7adf65589749', 'build_date': '2024-11-08T10:05:56.292914697Z', 'build_snapshot': False, 'lucene_version': '9.12.0', 'minimum_wire_compatibility_version': '7.17.0', 'minimum_index_compatibility_version': '7.0.0'}, 'tagline': 'You Know, for Search'}
partition 1
Successfully indexed 500 documents. Failed to index [] documents.
partition 2
Successfully indexed 500 documents. Failed to index [] documents.
partition 3
Successfully indexed 500 documents. Failed to index [] documents.
partition 4
Successfully indexed 257 documents. Failed to index [] documents.
我们通过如下的命令来查看文档数:
GET grocery-catalog/_count
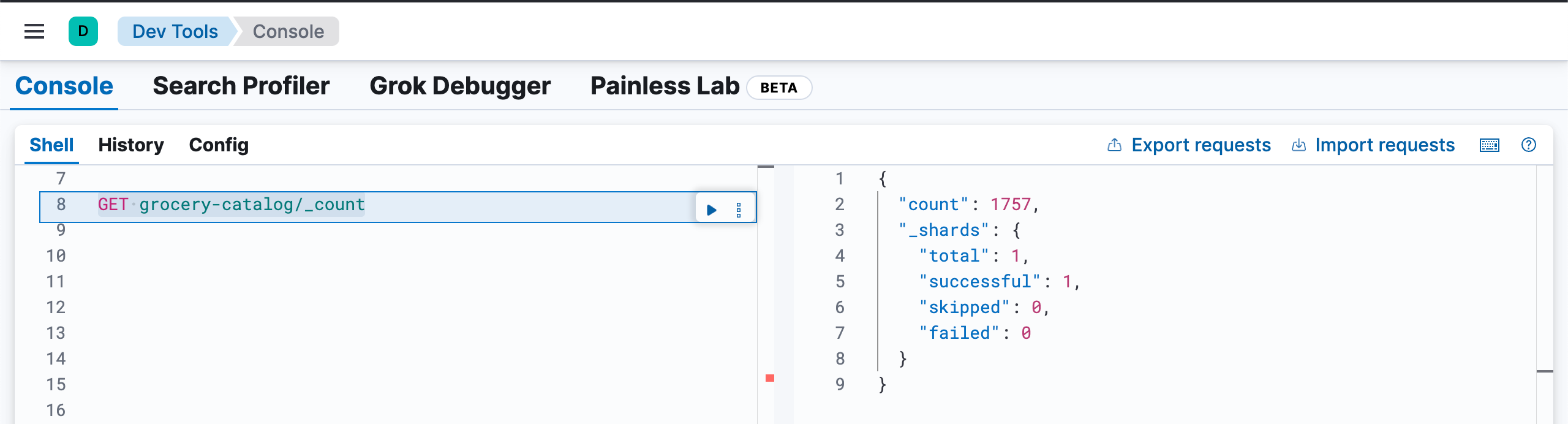
我们通过如下的命令来查看文档的内容:
GET grocery-catalog/_search
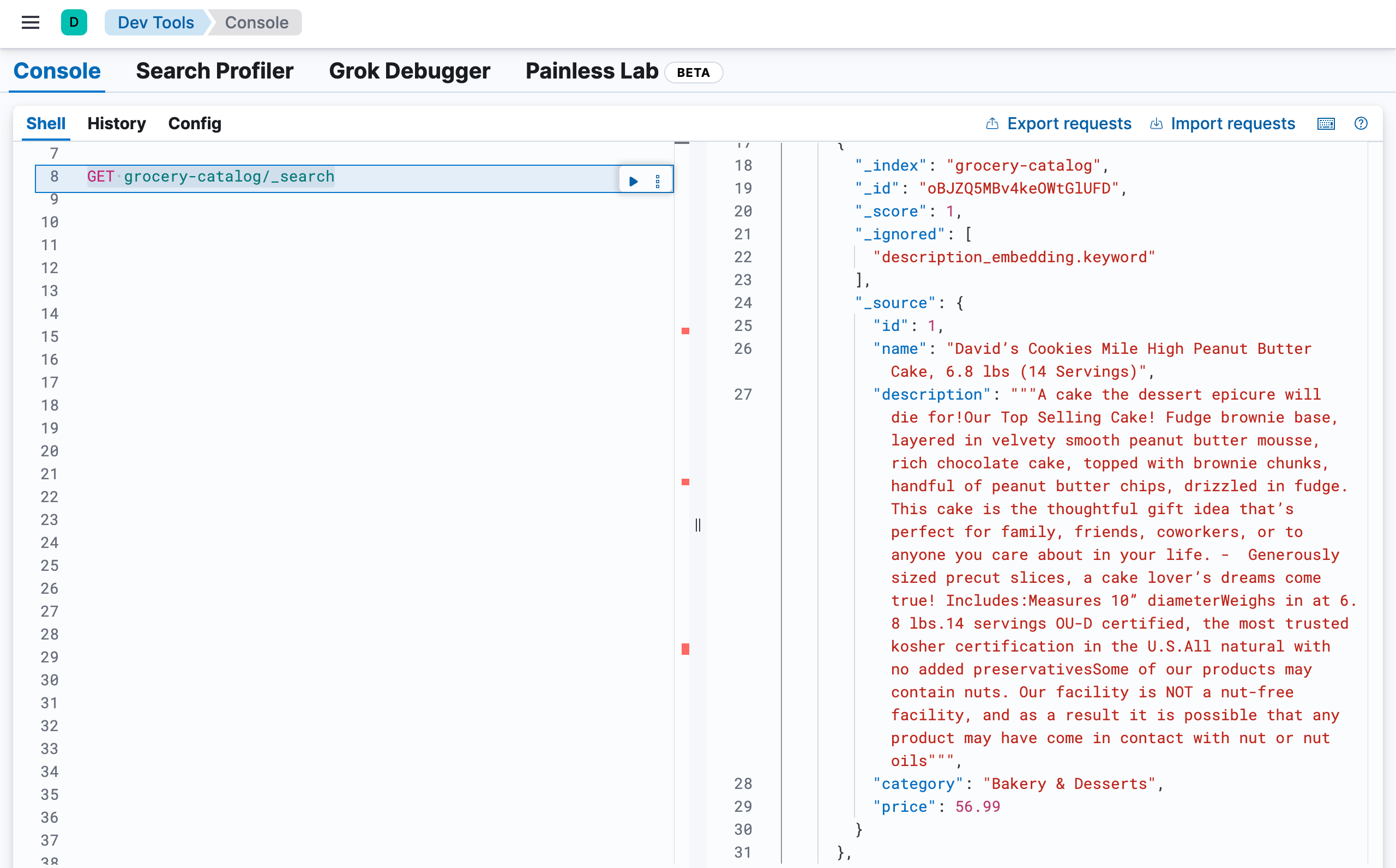
搜索数据
要执行搜索并获取语义和词汇搜索的结果,请运行以下命令:
python search.py
(.venv) $ python search.py
{'name': 'liuxgm.local', 'cluster_name': 'elasticsearch', 'cluster_uuid': 'HF3DAYNQSnOq0D1NmsNubg', 'version': {'number': '8.16.0', 'build_flavor': 'default', 'build_type': 'tar', 'build_hash': '12ff76a92922609df4aba61a368e7adf65589749', 'build_date': '2024-11-08T10:05:56.292914697Z', 'build_snapshot': False, 'lucene_version': '9.12.0', 'minimum_wire_compatibility_version': '7.17.0', 'minimum_index_compatibility_version': '7.0.0'}, 'tagline': 'You Know, for Search'}
Search Type Name Score
Semantic Coastal Seafood Live Maine Lobsters, (6/1.25 Lbs. Per Lobster) 6... 16.78093
Semantic Coastal Seafood Live Maine Lobsters, (6/1.25 Lbs. Per Lobster) 6... 16.78093
Semantic American Red Snapper - Whole, Head-On, Cleaned, (5-7 Individually Vacuum... 15.793174
Semantic American Red Snapper - Whole, Head-On, Cleaned, (5-7 Individually Vacuum... 15.793174
Semantic Whole Head On, Cleaned Branzino Fish (12-18 Oz. Per Fish),... 15.677712
Lexical Northwest Fish Alaskan Bairdi Snow Crab Sections, (10-14 / 13... 10.924439
Lexical Premium Seafood Variety Pack - 20 Total Packs, Total 12.5... 7.5987325
Lexical Premium Seafood Variety Pack - 20 Total Packs, Total 12.5... 7.5987325
Lexical Mr. Yoshida's, Sauce Original Gourmet, 86 oz 7.5274334
Lexical American Red Snapper - Whole, Head-On, Cleaned, (5-7 Individually Vacuum... 7.376876
上面是针对 "seafood for grilling" 进行搜索的结果。
我们可以根据文章 "使用 Elasticsearch 构建食谱搜索(一)" 里介绍的例子进行分别运行。这里就不再赘述了。