续
上集说到语义搜索,这集接着玩一下图搜图,这种场景在电商中很常见------拍照搜商品。图搜图实现非常类似语义搜索,代码逻辑结构都很类似...
开搞
还是老地方modelscope找个Vision Transformer模型,这里选用vit-base-patch16-224
,如果还想玩玩文搜图,可以选用支持多模态的multi-modal_clip-vit-base-patch16_zh
powershell
D:\python2023>modelscope download --model AI-ModelScope/vit-base-patch16-224
准备测试数据
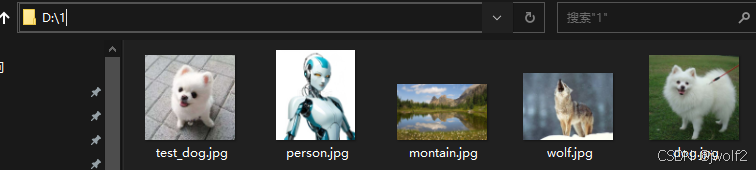
运行代码
python
from PIL import Image
from transformers import ViTFeatureExtractor, ViTModel
import torch
import time
from elasticsearch import Elasticsearch
# 初始化模型和图片特征提取器
MODEL_PATH = 'C:\\Users\\Administrator\\.cache\\modelscope\\hub\\AI-ModelScope\\vit-base-patch16-224'
feature_extractor = ViTFeatureExtractor.from_pretrained(MODEL_PATH)
model = ViTModel.from_pretrained(MODEL_PATH)
def extract_features(image_path):
image = Image.open(image_path)
inputs = feature_extractor(images=image, return_tensors="pt")
with torch.no_grad():
outputs = model(**inputs)
last_hidden_states = outputs.last_hidden_state
# 取出CLS token的输出作为图片的特征向量
features = last_hidden_states[:, 0].squeeze()
return features.numpy()
def store_image_features(image_id, features):
doc = {
'image_id': image_id,
'features': features.tolist()
}
res = es.index(index=index_name, id=image_id, body=doc)
print(res['result'])
def search_similar_images(query_image_path, top_k=5):
query_features = extract_features(query_image_path)
body = {
"size": top_k,
"query": {
"script_score": {
"query": {"match_all": {}},
"script": {
"source": "cosineSimilarity(params.query_vector, 'features') + 1.0",
"params": {"query_vector": query_features.tolist()}
}
}
}
}
response = es.search(index=index_name, body=body)
similar_images = [hit['_id'] for hit in response['hits']['hits']]
return similar_images
# 假设有一个图片ID和路径的字典
images = {
'img_dog': 'D:\\1\\dog.jpg',
'img_wolf': 'D:\\1\\wolf.jpg',
'img_person': 'D:\\1\\person.jpg',
'img_montain': 'D:\\1\\montain.jpg',
}
# 调用ES api创建索引
es = Elasticsearch([{'scheme':'http','host':'192.168.72.128','port':9200}])
index_name = 'image_search'
body = {
"mappings": {
"properties": {
"features": {
"type": "dense_vector",
"dims": 768 # ViT base模型的特征向量维度
}
}
}
}
if not es.indices.exists(index=index_name):
es.indices.create(index=index_name, body=body)
# 存储图片特征到ES
for img_id, img_path in images.items():
img_features = extract_features(img_path)
store_image_features(img_id, img_features)
# ES向量搜索找到某张图片最相似的图片集
time.sleep(3)
similar_ids = search_similar_images("D:\\1\\test_dog.jpg")
print(f"找到的相似图片ID: {similar_ids}")
可以看到最相似的还是狗,狼也像狗所以次之,然后是人,其实相关度已经很低了,最不相关的是风景图
看看索引情况
图片向量ES内存使用还不大,和文本数据基本一样,主要是因为图片特征向量维度都使用了768,如果不搜索不不准确,可以调高维度,但ES内存使用会增加。反之图片干扰特征少一点,特征向量维度小一些也会有较好的搜索效果。