随机森林是一种集成学习方法,属于 决策树 的扩展。它通过构建多棵决策树并结合其结果进行预测,能够显著提升模型的准确性和鲁棒性。随机森林特别适用于分类和回归任务,广泛应用于许多实际问题中,如金融欺诈检测、疾病预测、图像识别等。
以下是随机森林分类模型在数学建模中的建模过程,涵盖其基本原理、数学公式和建模步骤。
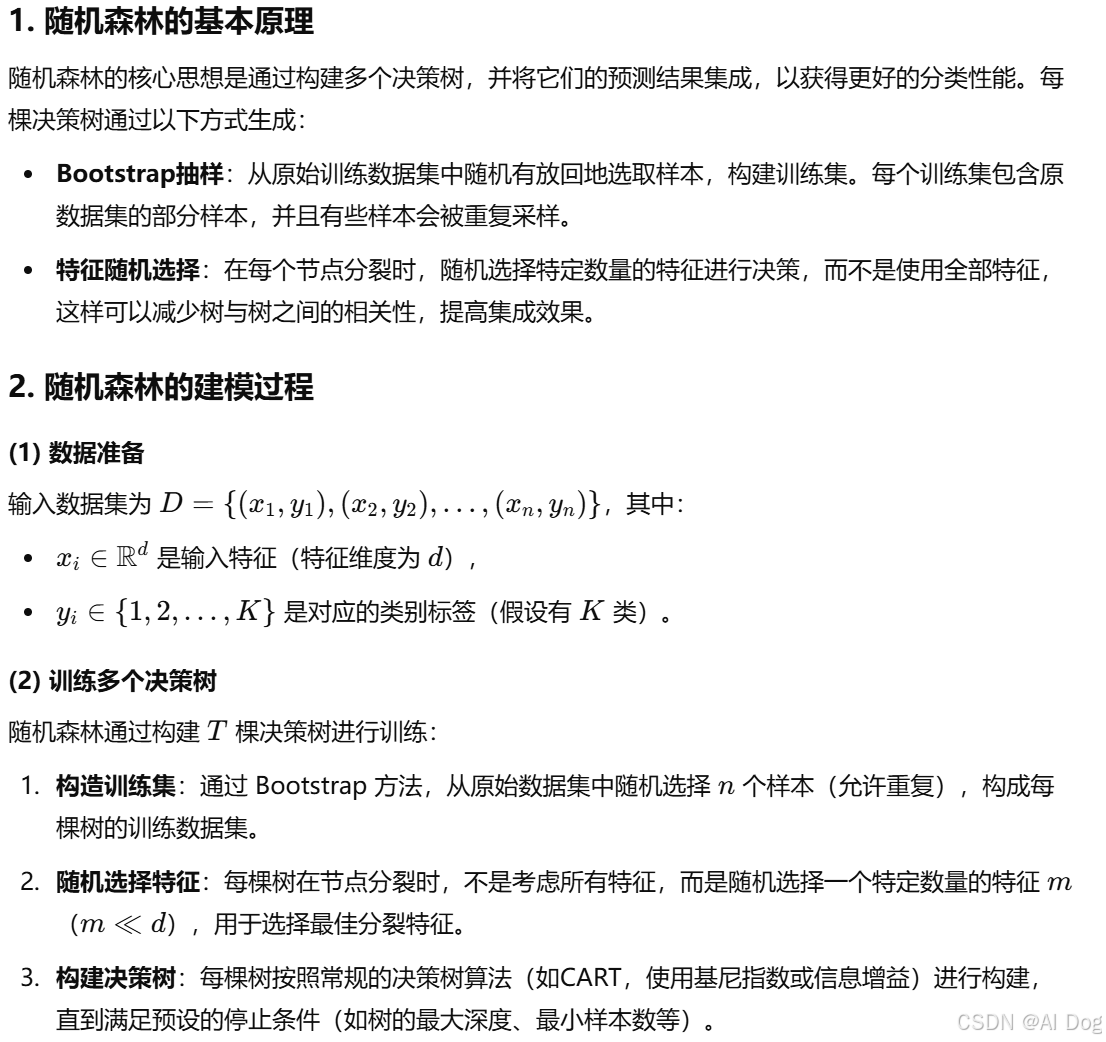
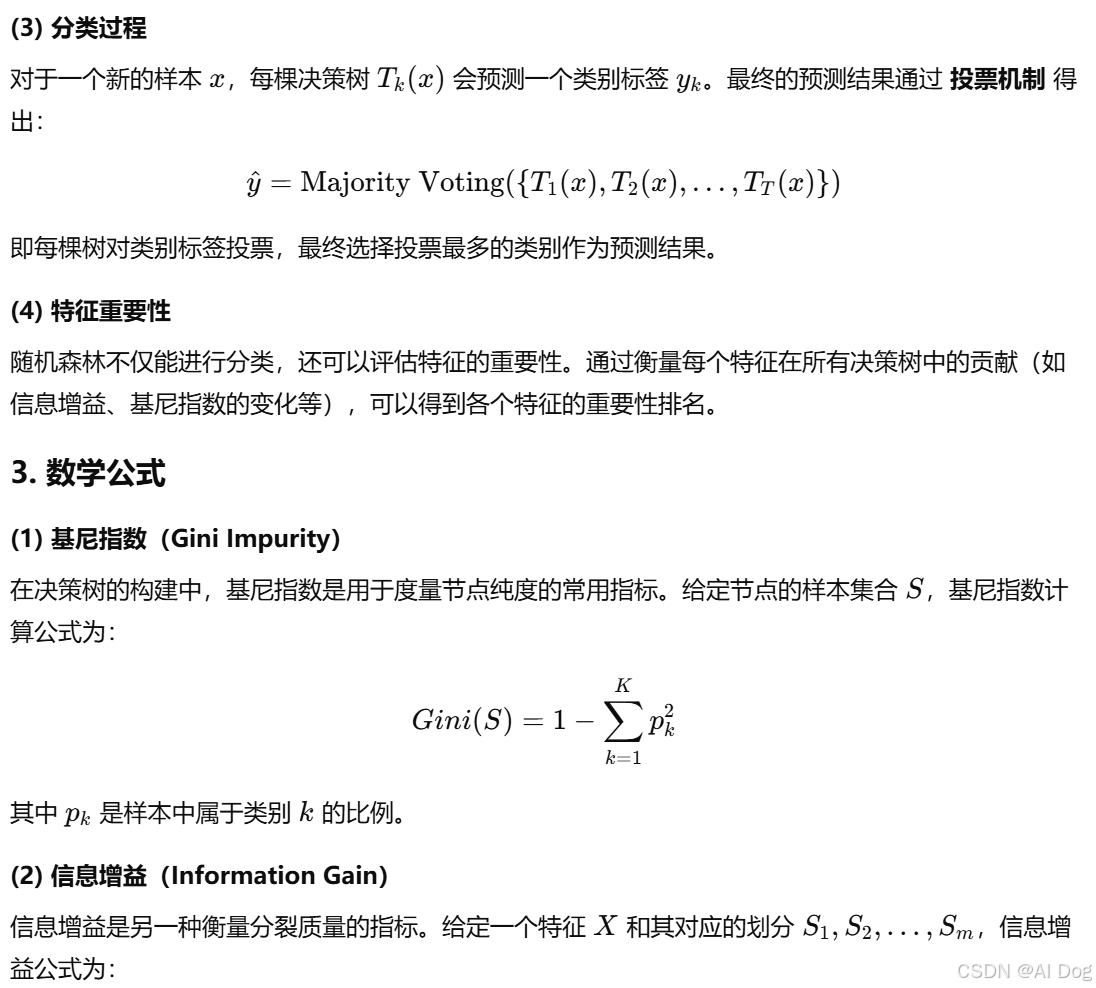
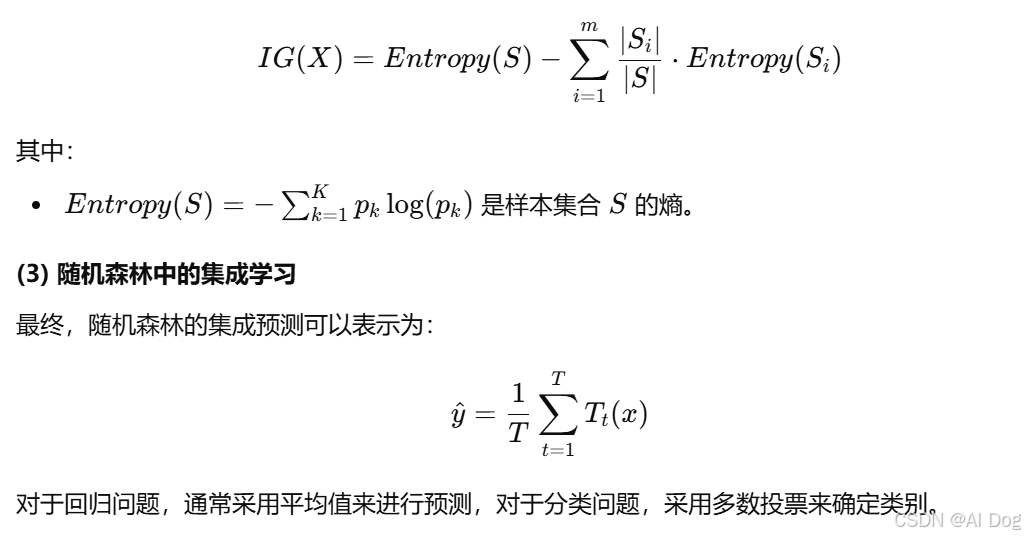
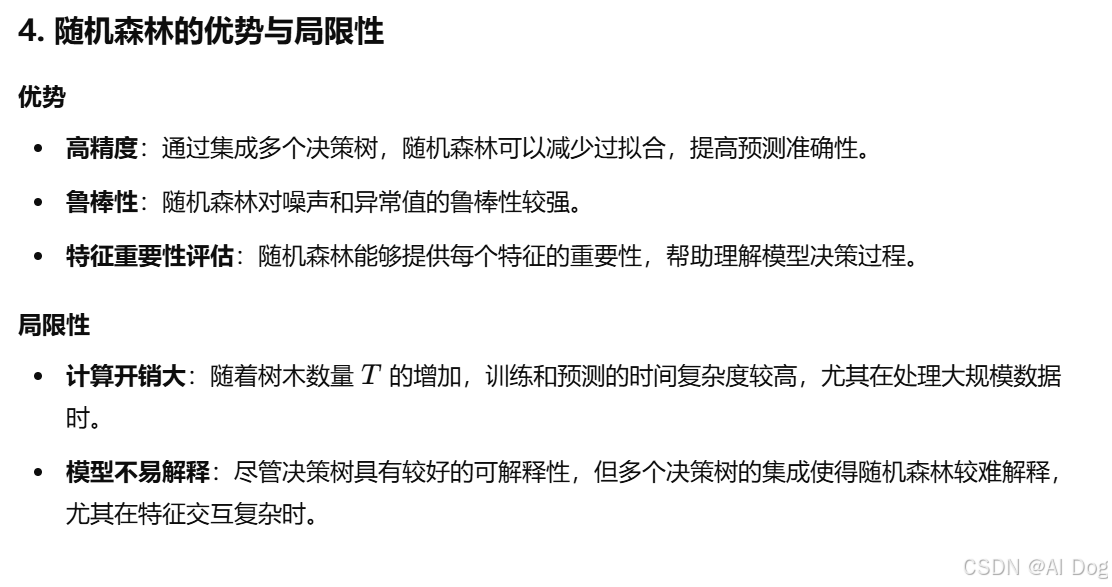
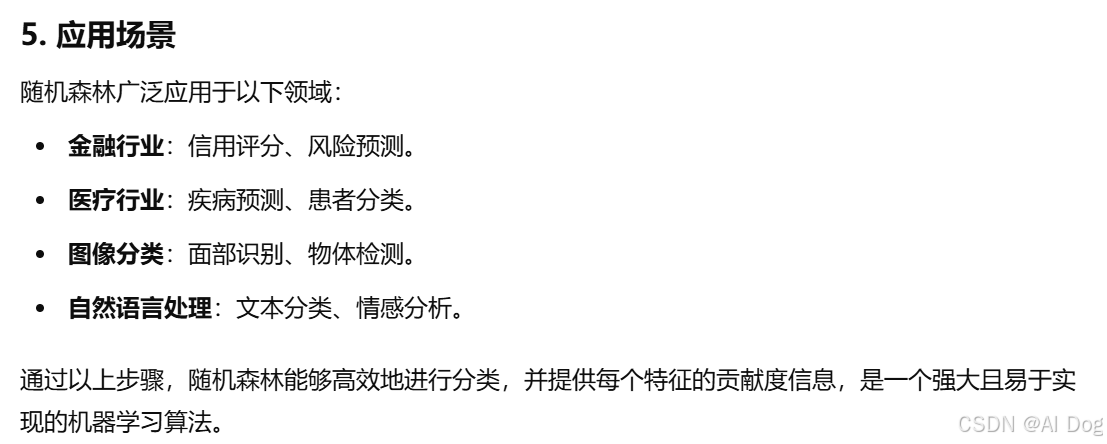
% 1. 加载数据集
% 使用MATLAB自带的鸢尾花数据集作为示例
load fisheriris; % 数据集包含iris数据,特征存储在meas中,类别标签存储在species中
% 2. 数据准备
X = meas; % 特征矩阵 (150 x 4)
y = species; % 类别标签 (150 x 1)
% 将数据分成训练集和测试集
cv = cvpartition(length(y), 'HoldOut', 0.3); % 70%用于训练,30%用于测试
X_train = X(training(cv), :); % 训练集特征
y_train = y(training(cv)); % 训练集标签
X_test = X(test(cv), :); % 测试集特征
y_test = y(test(cv)); % 测试集标签
% 3. 训练随机森林分类器
num_trees = 100; % 随机森林中树的数量
rf_model = TreeBagger(num_trees, X_train, y_train, 'OOBPrediction', 'On', 'Method', 'classification');
% 4. 模型评估
% 预测测试集
y_pred = predict(rf_model, X_test);
y_pred = categorical(y_pred); % 转换为类别数据
% 计算准确率
accuracy = sum(y_pred == y_test) / length(y_test);
fprintf('Test Accuracy: %.2f%%\n', accuracy * 100);
% 5. 特征重要性评估
% 获取特征重要性
feature_importance = rf_model.OOBPermutedPredictorDeltaError;
disp('Feature Importance:');
disp(feature_importance);
% 6. 绘制特征重要性条形图
figure;
bar(feature_importance);
title('Feature Importance');
xlabel('Features');
ylabel('Importance');
set(gca, 'XTickLabel', {'SepalLength', 'SepalWidth', 'PetalLength', 'PetalWidth'});
% 7. 交叉验证错误
% 使用袋外数据计算错误率
figure;
oobError = oobError(rf_model);
plot(oobError);
title('Out-of-Bag Error');
xlabel('Number of Grown Trees');
ylabel('Out-of-Bag Error Rate');