info_nce_loss
这个是clip作对比学习的损失函数
各个博客上都有详细介绍了,我这里就不赘述
python
def info_nce_loss(image_features, text_features,logit_scale,labels, temperature=0.07):
batch_size = image_features.shape[0]
image_features = image_features / image_features.norm(dim=-1, keepdim=True)
text_features = text_features / text_features.norm(dim=-1, keepdim=True)
similarity_matrix = torch.matmul(image_features, text_features.T) / temperature
logits_per_image = similarity_matrix
logits_per_text = similarity_matrix.T
# 构造标签,正样本对应的位置为1,其余为0,这里假设批次内第一个文本特征是对应图像的正样本文本特征
gen_labels = torch.arange(batch_size).long().to(image_features.device)
total_loss = (
F.cross_entropy(logits_per_image, gen_labels)+
F.cross_entropy(logits_per_text, gen_labels)
)/2
return total_loss, logits_per_image, logits_per_text
我踩的坑
我微调 c l i p clip clip 做分类任务类别数为3
:
- 数据集为图像-文本对数据集:即一个数据样本为一个图像和对应的文本在json文件里。
这里每个类别的图像的文本都是一样的,也就是a类别下图像可能会有细微不同,但是文本都是一样的
- 微调 c l i p clip clip 的结构同原始 c l i p clip clip 一致,输出的图像特征维度为 [ 输入图像数量 , 512 ] [输入图像数量,512] [输入图像数量,512],文本特征维度为 [ 输入的文本数量 , 512 ] [输入的文本数量,512] [输入的文本数量,512]。
这里选用不同的clip结构,输出维度可能有所不同
- 我微调过程输入 c l i p clip clip 的数据为 b a t c h _ s i z e batch\_size batch_size个图像、文本。输出的logit维度为 [ b a t c h _ s i z e , b a t c h _ s i z e ] [batch\_size,batch\_size] [batch_size,batch_size]
当使用 c l i p clip clip 去做分类任务假设类别为3
时,直接使用上面的损失函数并不合适
因为:
g e n _ l a b e l s gen\_labels gen_labels会产生一个 [ 0 ... ... b a t c h _ s i z e − 1 ] [0......batch\_size-1] [0......batch_size−1]的序列,接着和 l o g i t logit logit 做交叉熵。这里的 l o g i t logit logit 维度为 [ b a t c h _ s i z e , b a t c h _ s i z e ] [batch\_size,batch\_size] [batch_size,batch_size]
这意味着: l o g i t logit logit的对角线处的数据才会被 l o s s loss loss记录即第 i i i 个图像和第 i i i 个文本才是匹配的正样本,其余的为负样本。
这跟我实验设置下的分类任务有所冲突:因为我只有3个类别,而对于 l o g i t logit logit的第 i i i 行(即第 i i i 图像),只会跟第 i i i 列(即第 i i i 个文本)是正样本,而第 i i i 个图像应该和不止一个文本是正样本 。例如:第0行图像和第0列的文本是正样本,还会和第 0 + 3 i , i = 0 , 1 , 2 ... ... 0+3i,i=0,1,2...... 0+3i,i=0,1,2......列的文本是正样本,而 i n f o _ n c e _ l o s s info\_nce\_loss info_nce_loss会忽略掉后面的正样本
导致微调出来的 A C C ACC ACC 和 F 1 F1 F1 都比较低
clip选用这样的损失函数,是因为其并不是做分类任务,而是直接用海量的互联网数据去预训练(a类别下图像可能会有细微不同,但是文本都是一样的
这个情况存在的可能性小)
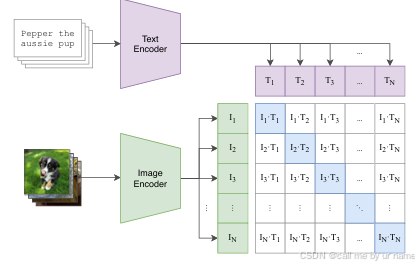
clip分类任务损失函数
python
def info_nce_loss(image_features, text_features,logit_scale,labels, temperature=0.07):
"""
计算InfoNCE损失函数,模拟CLIP中的对比学习损失计算
参数:
image_features (torch.Tensor): 图像特征表示,形状为 [batch_size, feature_dim]
text_features (torch.Tensor): 文本特征表示,形状为 [batch_size, feature_dim]
temperature (float): 用于缩放相似度得分的温度参数,控制分布的平滑程度
返回:
loss (torch.Tensor): InfoNCE损失值
"""
batch_size = image_features.shape[0]
image_features = image_features / image_features.norm(dim=-1, keepdim=True)
text_features = text_features / text_features.norm(dim=-1, keepdim=True)
similarity_matrix = torch.matmul(image_features, text_features.T) / temperature
logits_per_image = similarity_matrix
logits_per_text = similarity_matrix.T
gen_labels = labels
total_loss = F.cross_entropy(logits_per_image, gen_labels)
return total_loss, logits_per_image, logits_per_text
- 给每个图像-文本对记录类别 l a b e l label label
- 改变文本输入 ,每个 b a t c h _ s i z e batch\_size batch_size下输入的文本维度为 [ n _ c l a s s , ] [n\_class,] [n_class,],经过 c l i p _ e n c o d e r clip\_encoder clip_encoder 后维度为 [ n _ c l a s s , 512 ] [n\_class,512] [n_class,512]
- 接着做交叉熵计算