向量数据库
- vector storage engine
- 存储向量
- 关系型数据库:
- table
- id, name, age, school, address, ......
- 增删改查:字符串本身的比较
- table
- 文档型数据库:
- mongodb
- collection:
- document:
- json
- 每个json都是任意的结构
- json
- document:
- collection:
- mongodb
- 键值对数据库:
- redis:
- 内存型,做缓存
- key-value对
- redis:
- 存储向量 :
- 虽然叫向量数据库,但是向量部分只是索引,数据本身还是重点
- 数据查询时,使用向量进行语义化检索,而不是字符串匹配
- 结构:
- id, vector, text, metadata
- id:唯一标识,数据管理时使用
- vector:向量,用来做语义检索
- text:文本,信息本身
- metadata:元数据,用来做过滤
- 兼容文档型和关系型数据库的特点
- 常见的向量库:
- FAISS
- chromadb
- pinecone
- milvus
- 语义检索:
- 欧式距离(不开方)
- 余弦相似度
- 向量内积
ChromaDB
- 安装:
- pip install chromadb
- 增删改查
- 如何连接数据库?
- 如何插入数据?
- 如何检索数据?
- 如何删除数据?
- 如何更新数据?
- Chroma官方说明文档:https://docs.trychroma.com/docs/overview/introduction
- 本质:
- 数据存在那里?
- 语义检索式怎么实现的?
- 设计理念:
- 把数据存在 sqlite 中
- 自己设计一套语义检索逻辑
ChromaDB 基本操作
1. 安装
bash
pip install chromadb
2. 创建 Chroma 客户端
python
import chromadb
chroma_client = chromadb.Client()
3. 创建 Collection 集合
集合是存储嵌入、文档和任何附加元数据的地方
python
collection = chroma_client.create_collection(name="my_collection")
4. 添加文本到集合中
Chroma存储文本,并自动处理嵌入和索引
python
collection.add(
documents=[
"This is a document about pineapple",
"This is a document about oranges"
],
ids=["id1", "id2"]
)
5. 查询集合
可以使用查询文本列表查询该集合,Chroma 将返回最相似的 n 个结果。如果未提供 n_results,Chroma 将默认返回 10 个结果。这里我们只添加了 2 篇文档,因此将 n_results 设置为 2。
python
results = collection.query(
query_texts=["This is a query document about hawaii"], # Chroma will embed this for you
n_results=2 # how many results to return
)
print(results)
6. 检查结果
从上述查询中可以看到,我们关于夏威夷的查询在语义上与关于菠萝的文档最为相似。
python
{
'documents': [[
'This is a document about pineapple',
'This is a document about oranges'
]],
'ids': [['id1', 'id2']],
'distances': [[1.0404009819030762, 1.243080496788025]],
'uris': None,
'data': None,
'metadatas': [[None, None]],
'embeddings': None,
}
7. Demo
python
"""
ChromaDB基本操作
"""
from chromadb import Client
chroma_client = Client()
chroma_client
collection = chroma_client.create_collection(name="my_collection",get_or_create=True)
collection.database
collection.configuration_json
"""
增删改查
"""
import uuid
def get_uuid():
return str(uuid.uuid4())
ids = [get_uuid() for _ in range(2)]
documents = ["我今天去上学","天气很好"]
collection.add(ids=ids, documents=documents)
results["ids"]
collection.delete('b837983b-2911-4b15-9cc2-447975541a1e')
collection.get()
collection.update(ids=['3f850c2e-b772-4c99-bf19-2edbf5e3ae28'],
documents=["大家今天都很高兴"])
collection.get()
# update + insert
collection.upsert()
ChromaDB 服务端存储
参考 chromadb官方文档:https://docs.trychroma.com/production/chroma-server/client-server-mode
- 终端输入以下命令,启动本地 Chroma 服务端:
bash
chroma run --path "./chroma_data"
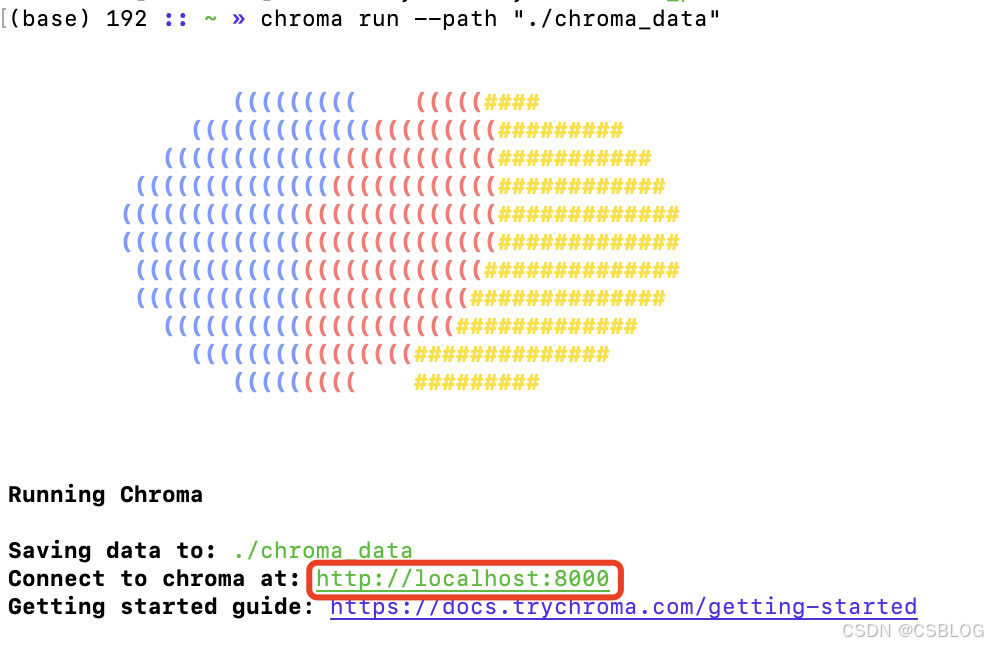
- 启动成功,可通过8000端口,连接到服务器
python
from chromadb import HttpClient
chroma_client = HttpClient(host='localhost',port=8000)
python
from dotenv import load_dotenv
load_dotenv()
from langchain_community.embeddings.dashscope import DashScopeEmbeddings
from langchain_community.chat_models import ChatTongyi
def get_embed():
"""
连接模型
"""
return DashScopeEmbeddings(model="text-embedding-v3")
def get_chat():
"""
连接模型
"""
return ChatTongyi(model="qwen-turbo", temperature=0.1, top_p=0.7)
- 语义检索
python
from models import get_embed
embed = get_embed()
# 语义检索
text = "外面天气怎么样?"
embedding = embed.embed_query(text=text)
collection.query(query_embeddings=embedding, n_results=1)
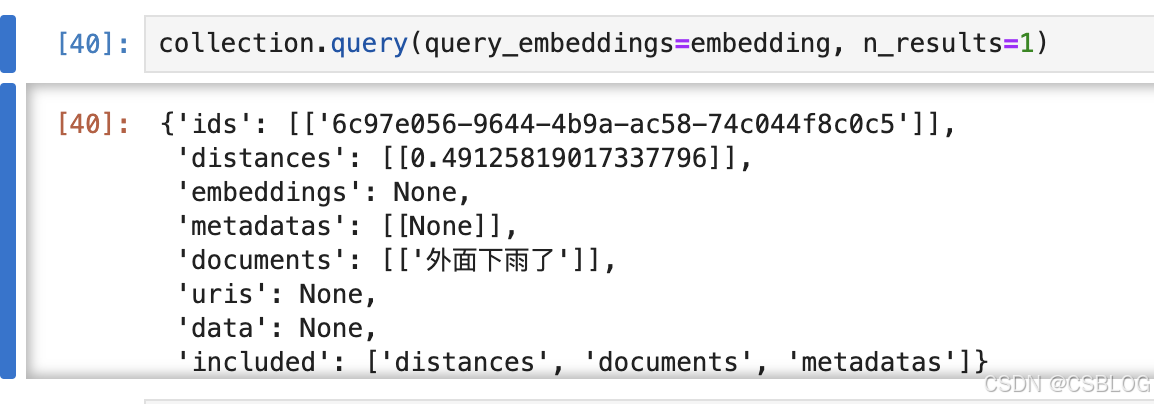
LangChain 和 Chroma 结合使用
python
from models import get_embed
from langchain_chroma import Chroma
from chromadb import HttpClient
embed = get_embed()
# 配置连接服务器的信息
client = HttpClient(host="localhost", port=8000)
db = Chroma(client=client, embedding_function=embed)
db.search(query="今天心情好吗?", search_type="similarity_score_threshold")
from models import get_chat
model = get_chat()
model.invoke(input="你好")
retriever = db.as_retriever(search_type="similarity_score_threshold",
search_kwargs={"score_threshold": 0.5, "k": 2})
retriever.invoke(input="外面天气怎么样?")
db.similarity_search_with_relevance_scores(query="你好")
数据入库
- load
- 读取原始数据(只要文本,不要其他数据)
- split
- 把文本切分成一个一个语义独立的段落
- embed
- 向量化,入库
python
file_name = "knowledge/产品介绍.txt"
with open(file=file_name, mode="r", encoding="utf8") as f:
data = f.read()
print(data)
python
# 方式一:按照自己的语义逻辑来切分
documents = [chunk.strip() for chunk in data.split("###") if chunk]
bash
pip install langchain_text_splitters -U
python
from langchain_text_splitters import RecursiveCharacterTextSplitter
# 定义一个分割器
# 设置的256为切分指导数,实际要小,因为会结合语义切分
splitter = RecursiveCharacterTextSplitter(chunk_size=256)
# splitter.split_text(text=data)
for chunk in splitter.split_text(text=data):
print(len(chunk))
print(chunk)
print('--------------------------')
chunk_size 无论怎么设置都不具备普世性,那么长度到底设置为多少合理呢?
最好的办法是手动区分,即文档手动的去划分语义。
所以第一期项目,设置差不多大小切完即可;到第二期项目时,可以手动去调整一些文档段落的内容,编辑调整一些格式。