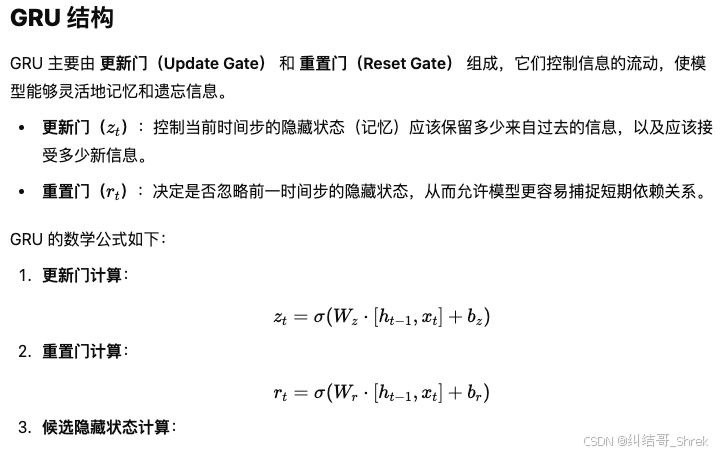
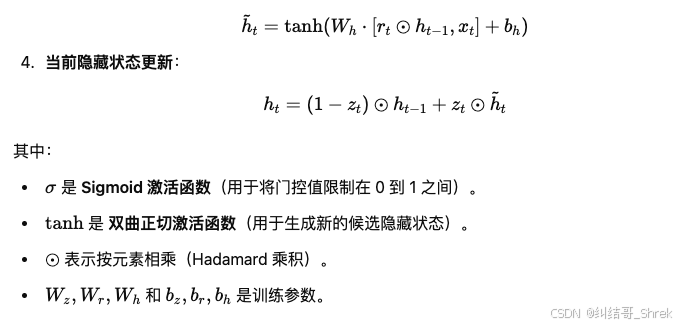
特性 | GRU | LSTM |
---|---|---|
计算效率 | 更快,参数更少 | 相对较慢,参数更多 |
结构复杂度 | 只有两个门(更新门和重置门) | 三个门(输入门、遗忘门、输出门) |
处理长时依赖 | 一般适用于中等长度依赖 | 更适合处理超长时序依赖 |
训练速度 | 训练更快,梯度更稳定 | 训练较慢,占用更多内存 |
例子:
import torch
import torch.nn as nn
import torch.optim as optim
import random
import matplotlib.pyplot as plt
# 🏁 迷宫环境(5×5)
class MazeEnv:
def __init__(self, size=5):
self.size = size
self.state = (0, 0) # 起点
self.goal = (size-1, size-1) # 终点
self.actions = [(0,1), (0,-1), (1,0), (-1,0)] # 右、左、下、上
def reset(self):
self.state = (0, 0) # 重置起点
return self.state
def step(self, action):
dx, dy = self.actions[action]
x, y = self.state
nx, ny = max(0, min(self.size-1, x+dx)), max(0, min(self.size-1, y+dy))
reward = 1 if (nx, ny) == self.goal else -0.1
done = (nx, ny) == self.goal
self.state = (nx, ny)
return (nx, ny), reward, done
# 🤖 GRU 策略网络
class GRUPolicy(nn.Module):
def __init__(self, input_size, hidden_size, output_size):
super(GRUPolicy, self).__init__()
self.gru = nn.GRU(input_size, hidden_size, batch_first=True)
self.fc = nn.Linear(hidden_size, output_size)
def forward(self, x, hidden):
out, hidden = self.gru(x, hidden)
out = self.fc(out[:, -1, :]) # 只取最后时间步
return out, hidden
# 🎯 训练参数
env = MazeEnv(size=5)
policy = GRUPolicy(input_size=2, hidden_size=16, output_size=4)
optimizer = optim.Adam(policy.parameters(), lr=0.01)
loss_fn = nn.CrossEntropyLoss()
# 🎓 训练
num_episodes = 500
epsilon = 1.0 # 初始的ε值,控制探索的概率
epsilon_min = 0.01 # 最小ε值
epsilon_decay = 0.995 # ε衰减率
best_path = [] # 用于存储最佳路径
for episode in range(num_episodes):
state = env.reset()
hidden = torch.zeros(1, 1, 16) # GRU 初始状态
states, actions, rewards = [], [], []
logits_list = []
for _ in range(20): # 最多 20 步
state_tensor = torch.tensor([[state[0], state[1]]], dtype=torch.float32).unsqueeze(0)
logits, hidden = policy(state_tensor, hidden)
logits_list.append(logits)
# ε-greedy 策略
if random.random() < epsilon:
action = random.choice(range(4)) # 随机选择动作
else:
action = torch.argmax(logits, dim=1).item() # 选择最大值对应的动作
next_state, reward, done = env.step(action)
states.append(state)
actions.append(action)
rewards.append(reward)
if done:
print(f"Episode {episode} - Reached Goal!")
# 找到最优路径
best_path = states + [next_state] # 当前 episode 的路径
break
state = next_state
# 计算损失
logits = torch.cat(logits_list, dim=0) # (T, 4)
action_tensor = torch.tensor(actions, dtype=torch.long) # (T,)
loss = loss_fn(logits, action_tensor)
optimizer.zero_grad()
loss.backward()
optimizer.step()
# 衰减 ε
epsilon = max(epsilon_min, epsilon * epsilon_decay)
if episode % 100 == 0:
print(f"Episode {episode}, Loss: {loss.item():.4f}, Epsilon: {epsilon:.4f}")
# 🧐 确保 best_path 已经记录
if len(best_path) == 0:
print("No path found during training.")
else:
print(f"Best path: {best_path}")
# 🚀 测试路径(只绘制最佳路径)
fig, ax = plt.subplots(figsize=(6,6))
# 初始化迷宫图
maze = [[0 for _ in range(5)] for _ in range(5)] # 5×5 迷宫
ax.imshow(maze, cmap="coolwarm", origin="upper")
# 画网格
ax.set_xticks(range(5))
ax.set_yticks(range(5))
ax.grid(True, color="black", linewidth=0.5)
# 画出最佳路径(红色)
for (x, y) in best_path:
ax.add_patch(plt.Rectangle((y, x), 1, 1, color="red", alpha=0.8))
# 画起点和终点
ax.text(0, 0, "S", ha="center", va="center", fontsize=14, color="white", fontweight="bold")
ax.text(4, 4, "G", ha="center", va="center", fontsize=14, color="white", fontweight="bold")
plt.title("GRU RL Agent - Best Path")
plt.show()