Attention机制是深度学习中的一种技术,特别是在自然语言处理(NLP)和计算机视觉领域中得到了广泛的应用。它的核心思想是模仿人类的注意力机制,即人类在处理信息时会集中注意力在某些关键部分上,而忽略其他不那么重要的信息。在机器学习模型中,这可以帮助模型更好地捕捉到输入数据中的关键信息。
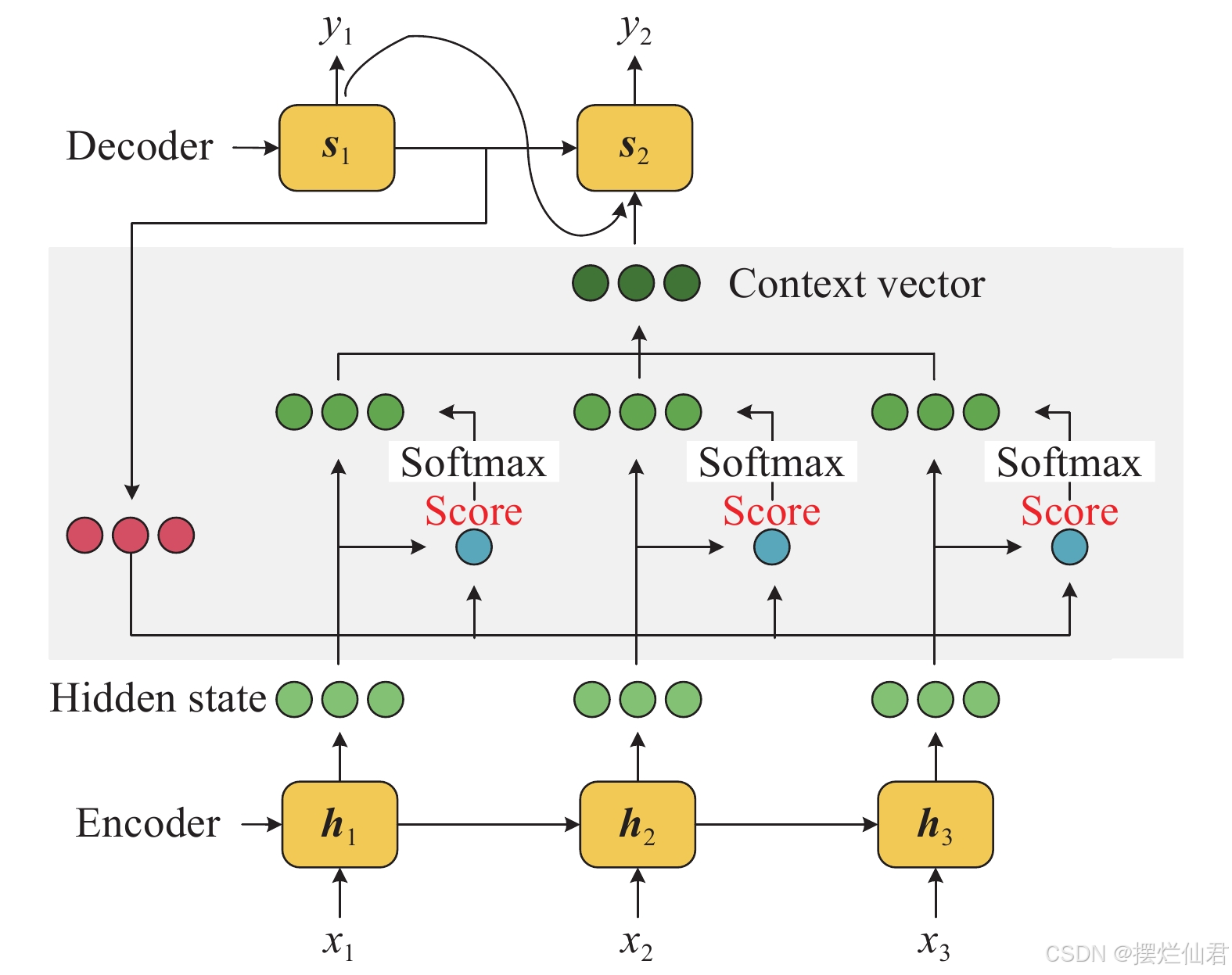
一、 Attention机制的基本原理
1.输入表示
在自然语言处理(NLP)任务中,输入数据通常是文本形式的,我们需要将这些文本转换为模型可以处理的数值形式。这个过程称为嵌入(Embedding)。嵌入层将每个单词映射到一个高维空间中的向量,这些向量被称为词向量。词向量能够捕捉单词的语义信息,并且可以被神经网络处理。
python
# 定义一个简单的嵌入层
class EmbeddingLayer(nn.Module):
def __init__(self, vocab_size, embed_dim):
super(EmbeddingLayer, self).__init__()
self.embedding = nn.Embedding(vocab_size, embed_dim)
def forward(self, x):
return self.embedding(x)
# 随机生成一个输入序列
input_seq = torch.randint(0, vocab_size, (32, 50)) # (batch_size, seq_len)
# 获取输入表示
input_repr = embedding_layer(input_seq)
在代码中,我们定义了一个EmbeddingLayer
类,它包含一个nn.Embedding
层,用于将输入的索引转换为对应的词向量。然后,我们生成一个随机的输入序列input_seq
,它模拟了一个批量大小为32,序列长度为50的文本数据。通过嵌入层,我们将这些索引转换为词向量,得到输入表示input_repr
。
2.计算注意力权重
注意力机制允许模型在处理序列数据时,动态地聚焦于当前步骤最相关的信息。在自注意力(Self-Attention)中,每个元素都会计算与其他所有元素的关联程度,这通过计算查询(Q)、键(K)和值(V)的线性变换来实现。
python
class Attention(nn.Module):
def __init__(self, embed_dim):
super(Attention, self).__init__()
self.query = nn.Linear(embed_dim, embed_dim)
self.key = nn.Linear(embed_dim, embed_dim)
self.value = nn.Linear(embed_dim, embed_dim)
def forward(self, x):
Q = self.query(x)
K = self.key(x)
V = self.value(x)
attention_scores = torch.matmul(Q, K.transpose(-1, -2)) / math.sqrt(embed_dim)
attention_weights = F.softmax(attention_scores, dim=-1)
return attention_weights
在这段代码中,我们定义了一个Attention
类,它包含三个线性层,分别用于计算Q、K和V。然后,我们通过矩阵乘法和softmax函数计算注意力权重,这些权重表示序列中每个元素对当前元素的重要性。
3.加权求和
一旦我们有了注意力权重,我们就可以使用它们来加权求和序列中的元素,从而生成一个综合了所有元素信息的表示。
python
def weighted_sum(attention_weights, input_repr):
return torch.matmul(attention_weights, input_repr)
这个简单的函数weighted_sum
接受注意力权重和输入表示作为输入,然后通过矩阵乘法计算加权求和,得到一个综合了序列中所有元素信息的新表示。
4.输出
最后,我们使用一个输出层将加权求和得到的表示转换为最终的输出,这可以是分类任务的类别概率,也可以是其他任务的预测结果。
python
class OutputLayer(nn.Module):
def __init__(self, embed_dim, output_dim):
super(OutputLayer, self).__init__()
self.fc = nn.Linear(embed_dim, output_dim)
def forward(self, x):
return self.fc(x)
在这个代码段中,我们定义了一个OutputLayer
类,它包含一个线性层,用于将模型的内部表示映射到输出空间。例如,在分类任务中,我们可以将嵌入维度的表示映射到类别数量的输出空间,并通过softmax函数或其他激活函数得到最终的预测概率。
5.实例代码
以下是使用Python和PyTorch实现上述内容的示例代码。这段代码将展示如何使用一个简单的Transformer模型来处理文本数据,包括输入表示、计算注意力权重、加权求和以及输出。
python
import torch
import torch.nn as nn
import torch.nn.functional as F
class TransformerBlock(nn.Module):
def __init__(self, embed_dim, num_heads, dropout=0.1):
super(TransformerBlock, self).__init__()
self.attn = nn.MultiheadAttention(embed_dim, num_heads, dropout=dropout)
self.ffn = nn.Sequential(
nn.Linear(embed_dim, 4 * embed_dim),
nn.GELU(),
nn.Linear(4 * embed_dim, embed_dim),
)
self.norm1 = nn.LayerNorm(embed_dim)
self.norm2 = nn.LayerNorm(embed_dim)
self.dropout = nn.Dropout(dropout)
def forward(self, x):
# 输入表示
# x: (seq_len, batch_size, embed_dim)
attn_output, _ = self.attn(x, x, x) # 自注意力,输入和输出都是x
attn_output = self.dropout(attn_output)
x = self.norm1(x + attn_output) # 加权求和和残差连接
# 前馈网络
ffn_output = self.ffn(x)
ffn_output = self.dropout(ffn_output)
x = self.norm2(x + ffn_output) # 加权求和和残差连接
return x
class TextTransformer(nn.Module):
def __init__(self, vocab_size, embed_dim, num_heads, num_layers, dropout=0.1):
super(TextTransformer, self).__init__()
self.embedding = nn.Embedding(vocab_size, embed_dim)
self.positional_encoding = nn.Parameter(torch.randn(1, 1, embed_dim))
self.encoder = nn.Sequential(*[TransformerBlock(embed_dim, num_heads, dropout) for _ in range(num_layers)])
self.fc_out = nn.Linear(embed_dim, vocab_size) # 假设是分类任务
def forward(self, x):
# 输入表示
embeds = self.embedding(x) # (batch_size, seq_len, embed_dim)
embeds = embeds + self.positional_encoding[:, :embeds.size(1), :] # 添加位置编码
embeds = embeds.transpose(0, 1) # (seq_len, batch_size, embed_dim)
# 计算注意力权重和加权求和
out = self.encoder(embeds)
# 输出
out = out.transpose(0, 1) # (batch_size, seq_len, embed_dim)
out = self.fc_out(out[:, -1, :]) # 假设只取序列的最后一个向量进行分类
return out
# 模型参数
vocab_size = 10000 # 词汇表大小
embed_dim = 256 # 嵌入层维度
num_heads = 8 # 注意力头数
num_layers = 6 # Transformer层数
# 实例化模型
model = TextTransformer(vocab_size, embed_dim, num_heads, num_layers)
# 随机生成一个输入序列
input_seq = torch.randint(0, vocab_size, (32, 100)) # (batch_size, seq_len)
# 前向传播
output = model(input_seq)
print(output.shape) # 应该输出 (batch_size, vocab_size)
这段代码首先定义了一个TransformerBlock
类,它包含了自注意力机制和前馈网络,然后定义了一个TextTransformer
类,它包含了嵌入层、位置编码、编码器和输出层。在TextTransformer
的前向传播中,我们首先将输入序列转换为嵌入表示,然后通过Transformer编码器进行处理,最后通过一个全连接层输出结果。这个例子展示了如何使用Transformer模型处理文本数据,并进行分类任务。
二、Attention机制的类型
1.Soft Attention
这种类型的注意力机制会输出一个概率分布,每个输入元素都有一个对应的权重,这些权重的和为1。Soft attention通常可以微分,因此可以用于梯度下降。Soft Attention输出一个概率分布,可以通过梯度下降进行优化。
python
import torch
import torch.nn as nn
import torch.nn.functional as F
class SoftAttention(nn.Module):
def __init__(self, embed_dim):
super(SoftAttention, self).__init__()
self.weight = nn.Parameter(torch.randn(embed_dim, 1))
def forward(self, x):
# x: (batch_size, seq_len, embed_dim)
scores = torch.matmul(x, self.weight).squeeze(-1) # (batch_size, seq_len)
weights = F.softmax(scores, dim=-1) # Softmax to get probabilities
return weights
# 示例使用
embed_dim = 128
soft_attn = SoftAttention(embed_dim)
input_seq = torch.randn(32, 50, embed_dim) # (batch_size, seq_len, embed_dim)
attention_weights = soft_attn(input_seq)
print("Soft Attention Weights:", attention_weights.sum(dim=1)) # 应该接近于1
2.Hard Attention
与soft attention不同,hard attention会随机或确定性地选择一个输入元素,并只关注这个元素。Hard attention通常不可微分,因此训练时可能需要使用强化学习或变分方法。Hard Attention随机选择一个输入元素,这里我们使用一个简单的采样策略。
python
import torch
class HardAttention(nn.Module):
def __init__(self, embed_dim):
super(HardAttention, self).__init__()
def forward(self, x):
# x: (batch_size, seq_len, embed_dim)
probs = torch.rand(x.size(0), x.size(1), device=x.device)
_, idx = torch.topk(probs, k=1, dim=1)
selected = torch.gather(x, 1, idx.unsqueeze(-1).expand(-1, -1, x.size(-1)))
return selected.squeeze(1)
# 示例使用
hard_attn = HardAttention(embed_dim)
selected_elements = hard_attn(input_seq)
print("Hard Attention Selected Elements:", selected_elements.shape) # (batch_size, embed_dim)
3.Self-Attention
即自注意力机制,这是一种特殊的注意力机制,它允许输入序列中的元素相互之间计算注意力权重,这在Transformer模型中得到了广泛应用。Self-Attention允许输入序列中的元素相互之间计算注意力权重。
python
class SelfAttention(nn.Module):
def __init__(self, embed_dim):
super(SelfAttention, self).__init__()
self.query = nn.Linear(embed_dim, embed_dim)
self.key = nn.Linear(embed_dim, embed_dim)
self.value = nn.Linear(embed_dim, embed_dim)
def forward(self, x):
# x: (batch_size, seq_len, embed_dim)
Q = self.query(x)
K = self.key(x)
V = self.value(x)
attention_scores = torch.matmul(Q, K.transpose(-1, -2)) / math.sqrt(embed_dim)
attention_weights = F.softmax(attention_scores, dim=-1)
output = torch.matmul(attention_weights, V)
return output, attention_weights
# 示例使用
self_attn = SelfAttention(embed_dim)
output, weights = self_attn(input_seq)
print("Self Attention Output:", output.shape) # (batch_size, seq_len, embed_dim)
4.Multi-Head Attention
在Transformer模型中,为了捕捉不同子空间中的信息,会使用多头注意力机制,即并行地运行多个自注意力机制,然后将结果合并。Multi-Head Attention并行地运行多个自注意力机制,然后将结果合并。
python
class MultiHeadAttention(nn.Module):
def __init__(self, embed_dim, num_heads):
super(MultiHeadAttention, self).__init__()
self.num_heads = num_heads
self.head_dim = embed_dim // num_heads
assert self.head_dim * num_heads == embed_dim, "embed_dim must be divisible by num_heads"
self.query = nn.Linear(embed_dim, embed_dim)
self.key = nn.Linear(embed_dim, embed_dim)
self.value = nn.Linear(embed_dim, embed_dim)
self.fc_out = nn.Linear(embed_dim, embed_dim)
def forward(self, x):
# x: (batch_size, seq_len, embed_dim)
batch_size, seq_len, embed_dim = x.size()
Q = self.query(x).view(batch_size, seq_len, self.num_heads, self.head_dim).transpose(1, 2)
K = self.key(x).view(batch_size, seq_len, self.num_heads, self.head_dim).transpose(1, 2)
V = self.value(x).view(batch_size, seq_len, self.num_heads, self.head_dim).transpose(1, 2)
attention_scores = torch.matmul(Q, K.transpose(-1, -2)) / math.sqrt(self.head_dim)
attention_weights = F.softmax(attention_scores, dim=-1)
output = torch.matmul(attention_weights, V).transpose(1, 2).contiguous()
output = output.view(batch_size, seq_len, embed_dim)
output = self.fc_out(output)
return output
# 示例使用
num_heads = 8
multi_head_attn = MultiHeadAttention(embed_dim, num_heads)
multi_head_output = multi_head_attn(input_seq)
print("Multi-Head Attention Output:", multi_head_output.shape) # (batch_size, seq_len, embed_dim)
Soft Attention和Self-Attention可以直接用于梯度下降优化,而Hard Attention由于其不可微分的特性,可能需要特殊的训练技巧。Multi-Head Attention则通过并行处理捕捉更丰富的信息。
三、Attention机制的应用
1.机器翻译
机器翻译是注意力机制最著名的应用之一。在这个任务中,模型需要将一种语言(源语言)的文本转换为另一种语言(目标语言)的文本。注意力机制在这里的作用是在生成目标语言的每个单词时,动态地聚焦于源语言中相关的部分,这有助于捕捉长距离依赖关系,并提高翻译的准确性和流畅性。
python
import torch
import torch.nn as nn
import torch.optim as optim
class Encoder(nn.Module):
def __init__(self, input_dim, emb_dim, hid_dim, n_layers, dropout):
super().__init__()
self.embedding = nn.Embedding(input_dim, emb_dim)
self.rnn = nn.LSTM(emb_dim, hid_dim, n_layers, dropout=dropout)
self.dropout = nn.Dropout(dropout)
def forward(self, src):
embedded = self.dropout(self.embedding(src))
outputs, (hidden, cell) = self.rnn(embedded)
return hidden, cell
class Attention(nn.Module):
def __init__(self, enc_hid_dim, dec_hid_dim):
super().__init__()
self.attn = nn.Linear((enc_hid_dim * 2) + dec_hid_dim, dec_hid_dim)
self.v = nn.Linear(dec_hid_dim, 1, bias=False)
def forward(self, hidden, encoder_outputs):
hidden = hidden.repeat(encoder_outputs.shape[0], 1).transpose(0, 1)
encoder_outputs = encoder_outputs.transpose(0, 1)
attn_energies = self.score(hidden, encoder_outputs)
return F.softmax(attn_energies, dim=-1)
def score(self, hidden, encoder_outputs):
energy = torch.tanh(self.attn(torch.cat([hidden, encoder_outputs], dim=2)))
energy = self.v(energy).squeeze(2)
return energy
class Decoder(nn.Module):
def __init__(self, output_dim, emb_dim, hid_dim, n_layers, dropout):
super().__init__()
self.output_dim = output_dim
self.embedding = nn.Embedding(output_dim, emb_dim)
self.rnn = nn.LSTM(emb_dim, hid_dim, n_layers, dropout=dropout)
self.attention = Attention(hid_dim, hid_dim)
self.fc_out = nn.Linear(hid_dim, output_dim)
self.dropout = nn.Dropout(dropout)
def forward(self, input, hidden, cell, encoder_outputs):
input = input.unsqueeze(0)
embedded = self.dropout(self.embedding(input))
attn_weights = self.attention(hidden, encoder_outputs)
context = attn_weights.bmm(encoder_outputs.transpose(0, 1))
rnn_input = torch.cat((embedded, context), dim=2)
output, (hidden, cell) = self.rnn(rnn_input, (hidden, cell))
output = output.squeeze(0)
out = self.fc_out(output)
return out, hidden, cell
# 假设参数
input_dim = 1000 # 源语言词汇表大小
output_dim = 1000 # 目标语言词汇表大小
emb_dim = 256 # 嵌入层维度
hid_dim = 512 # 隐藏层维度
n_layers = 2 # LSTM层数
dropout = 0.1 # Dropout
# 实例化模型
encoder = Encoder(input_dim, emb_dim, hid_dim, n_layers, dropout)
decoder = Decoder(output_dim, emb_dim, hid_dim, n_layers, dropout)
# 假设输入
src = torch.randint(0, input_dim, (10, 32)) # (seq_len, batch_size)
input = torch.randint(0, output_dim, (1, 32)) # (seq_len, batch_size)
# 前向传播
hidden, cell = encoder(src)
output, hidden, cell = decoder(input, hidden, cell, src)
print("Translation Output:", output.shape) # (batch_size, output_dim)
在示例代码中,我们定义了一个基于注意力的Seq2Seq模型,它由一个编码器和一个解码器组成。编码器读取源语言文本,并输出一个上下文向量和隐藏状态。解码器则使用这个上下文向量来生成目标语言文本,同时更新隐藏状态。注意力机制通过计算源语言文本中每个单词的重要性,并将这些信息合并到解码器的每一步中,从而允许模型在生成每个单词时"回顾"源语言文本的相关部分。
2.文本摘要
在自动文本摘要任务中,模型需要从长文本中提取关键信息,并生成一个简短的摘要。注意力机制可以帮助模型识别哪些句子或短语对于理解全文内容最为重要,从而在生成摘要时保留这些关键信息。
python
import torch
import torch.nn as nn
import torch.optim as optim
class Encoder(nn.Module):
def __init__(self, input_dim, emb_dim, hid_dim, n_layers, dropout):
super().__init__()
self.embedding = nn.Embedding(input_dim, emb_dim)
self.rnn = nn.LSTM(emb_dim, hid_dim, n_layers, dropout=dropout)
self.dropout = nn.Dropout(dropout)
def forward(self, src):
embedded = self.dropout(self.embedding(src))
outputs, (hidden, cell) = self.rnn(embedded)
return hidden, cell
class Attention(nn.Module):
def __init__(self, enc_hid_dim, dec_hid_dim):
super().__init__()
self.attn = nn.Linear((enc_hid_dim * 2) + dec_hid_dim, dec_hid_dim)
self.v = nn.Linear(dec_hid_dim, 1, bias=False)
def forward(self, hidden, encoder_outputs):
hidden = hidden.repeat(encoder_outputs.shape[0], 1).transpose(0, 1)
encoder_outputs = encoder_outputs.transpose(0, 1)
attn_energies = self.score(hidden, encoder_outputs)
return F.softmax(attn_energies, dim=-1)
def score(self, hidden, encoder_outputs):
energy = torch.tanh(self.attn(torch.cat([hidden, encoder_outputs], dim=2)))
energy = self.v(energy).squeeze(2)
return energy
class Decoder(nn.Module):
def __init__(self, output_dim, emb_dim, hid_dim, n_layers, dropout):
super().__init__()
self.output_dim = output_dim
self.embedding = nn.Embedding(output_dim, emb_dim)
self.rnn = nn.LSTM(emb_dim, hid_dim, n_layers, dropout=dropout)
self.attention = Attention(hid_dim, hid_dim)
self.fc_out = nn.Linear(hid_dim, output_dim)
self.dropout = nn.Dropout(dropout)
def forward(self, input, hidden, cell, encoder_outputs):
input = input.unsqueeze(0)
embedded = self.dropout(self.embedding(input))
attn_weights = self.attention(hidden, encoder_outputs)
context = attn_weights.bmm(encoder_outputs.transpose(0, 1))
rnn_input = torch.cat((embedded, context), dim=2)
output, (hidden, cell) = self.rnn(rnn_input, (hidden, cell))
output = output.squeeze(0)
out = self.fc_out(output)
return out, hidden, cell
# 假设参数
input_dim = 1000 # 源语言词汇表大小
output_dim = 1000 # 目标语言词汇表大小
emb_dim = 256 # 嵌入层维度
hid_dim = 512 # 隐藏层维度
n_layers = 2 # LSTM层数
dropout = 0.1 # Dropout
# 实例化模型
encoder = Encoder(input_dim, emb_dim, hid_dim, n_layers, dropout)
decoder = Decoder(output_dim, emb_dim, hid_dim, n_layers, dropout)
# 假设输入
src = torch.randint(0, input_dim, (10, 32)) # (seq_len, batch_size)
input = torch.randint(0, output_dim, (1, 32)) # (seq_len, batch_size)
# 前向传播
hidden, cell = encoder(src)
output, hidden, cell = decoder(input, hidden, cell, src)
print("Translation Output:", output.shape) # (batch_size, output_dim)
虽然示例代码没有详细展示,但可以想象,一个基于注意力的文本摘要模型会有一个编码器来处理输入文本,并生成一系列隐藏状态。然后,一个解码器会使用这些隐藏状态和注意力权重来生成摘要,同时关注输入文本中与当前生成摘要最相关的部分。这样,生成的摘要不仅包含了原文的核心信息,而且更加紧凑和连贯。
3.图像识别
在图像识别任务中,模型的目标是识别图像中的对象。注意力机制可以帮助模型集中注意力在图像中的关键特征上,例如人脸的眼睛或汽车的轮子,这些特征对于识别任务至关重要。
python
import torchvision.models as models
class AttentionCNN(nn.Module):
def __init__(self):
super().__init__()
self.cnn = models.resnet18(pretrained=True)
self.fc = nn.Linear(512, 1000) # 假设有1000个类别
def forward(self, x):
x = self.cnn(x)
# 假设我们添加一个简单的注意力层
attention_weights = torch.sigmoid(self.cnn.fc.weight)
x = torch.sum(x * attention_weights, dim=1)
x = self.fc(x)
return x
# 实例化模型
attention_cnn = AttentionCNN()
# 假设输入
input_image = torch.randn(32, 3, 224, 224) # (batch_size, channels, height, width)
# 前向传播
output = attention_cnn(input_image)
print("Image Recognition Output:", output.shape) # (batch_size, num_classes)
在示例代码中,我们定义了一个带有简单注意力层的CNN模型。这个注意力层通过学习图像中不同区域的重要性,为每个特征分配权重。这样,模型就可以更加关注于对分类任务最重要的特征,而不是平等对待图像中的所有像素。这种方法可以提高模型对图像中关键信息的敏感性,从而提高识别的准确性。
4.语音识别
语音识别是将语音信号转换为文本的任务。在这个任务中,模型需要理解语音中的语义信息,并将其转换为书面语言。注意力机制可以帮助模型在处理语音信号时,关注那些携带重要信息的部分,例如特定的音素或单词。
python
class SpeechRecognitionModel(nn.Module):
def __init__(self, input_dim, emb_dim, hid_dim, output_dim, n_layers, dropout):
super().__init__()
self.rnn = nn.LSTM(input_dim, emb_dim, n_layers, dropout=dropout, batch_first=True)
self.attention = Attention(emb_dim, emb_dim)
self.fc_out = nn.Linear(emb_dim, output_dim)
self.dropout = nn.Dropout(dropout)
def forward(self, x):
# x: (batch_size, seq_len, input_dim)
outputs, (hidden, cell) = self.rnn(x)
attn_weights = self.attention(hidden, outputs)
context = torch.bmm(attn_weights, outputs)
output = self.fc_out(context.squeeze(1))
return output
# 假设参数
input_dim = 128 # 特征维度
output_dim = 1000 # 词汇表大小
# 实例化模型
speech_recognition = SpeechRecognitionModel(input_dim, emb_dim, hid_dim, output_dim, n_layers, dropout)
# 假设输入
speech_signal = torch.randn(32, 100, input_dim) # (batch_size, seq_len, input_dim)
# 前向传播
output = speech_recognition(speech_signal)
print("Speech Recognition Output:", output.shape) # (batch_size, output_dim)
在示例代码中,我们定义了一个基于注意力的RNN模型,用于处理语音信号。模型的RNN部分处理序列化的语音特征,而注意力机制则帮助模型在生成每个单词时,关注语音信号中最相关的部分。这样,模型可以更准确地捕捉到语音中的语义信息,并将其转换为正确的文本输出。