掩膜
矩阵上的掩码操作其实很简单,其思路是我们根据掩膜矩阵(即内核)重新计算图像中每个像素的值,此掩码保存的值将调整相邻像素(和当前像素)对新像素值的影响程度。从数学的角度来看,我们用我们指定的值做一个加权平均。
掩码相当于是在图片上增加了一个过滤膜,其实就是用选定的图像、图形或物体,对待处理的图像(局部或全部)进行遮挡来控制图像处理的区域或处理过程。
测试案例
让我们考虑图像对比度增强方法的问题。基本上,我们是对图像的每个像素应用以下公式:
第一种表示法是使用公式,而第二种表示法是第一种表示法的压缩版本,使用掩码。通过将掩码矩阵的中心(由0 - 0索引标注的大写字母)放在要计算的像素上,并将像素值与重叠的矩阵值相乘,从而使用掩码。这是一样的,但是在大矩阵的情况下后一种符号更容易看。
代码
cpp
#include <opencv2/imgcodecs.hpp>
#include <opencv2/highgui.hpp>
#include <opencv2/imgproc.hpp>
#include <iostream>
using namespace std;
using namespace cv;
static void help(char* progName)
{
cout << endl
<< "This program shows how to filter images with mask: the write it yourself and the"
<< "filter2d way. " << endl
<< "Usage:" << endl
<< progName << " [image_path -- default lena.jpg] [G -- grayscale] " << endl << endl;
}
void Sharpen(const Mat& myImage,Mat& Result);
int main( int argc, char* argv[])
{
help(argv[0]);
const char* filename = argc >=2 ? argv[1] : "lena.jpg";
Mat src, dst0, dst1;
if (argc >= 3 && !strcmp("G", argv[2]))
src = imread( samples::findFile( filename ), IMREAD_GRAYSCALE);
else
src = imread( samples::findFile( filename ), IMREAD_COLOR);
if (src.empty())
{
cerr << "Can't open image [" << filename << "]" << endl;
return EXIT_FAILURE;
}
namedWindow("Input", WINDOW_AUTOSIZE);
namedWindow("Output", WINDOW_AUTOSIZE);
imshow( "Input", src );
double t = (double)getTickCount();
Sharpen( src, dst0 );
t = ((double)getTickCount() - t)/getTickFrequency();
cout << "Hand written function time passed in seconds: " << t << endl;
imshow( "Output", dst0 );
waitKey();
Mat kernel = (Mat_<char>(3,3) << 0, -1, 0,
-1, 5, -1,
0, -1, 0);
t = (double)getTickCount();
filter2D( src, dst1, src.depth(), kernel );
t = ((double)getTickCount() - t)/getTickFrequency();
cout << "Built-in filter2D time passed in seconds: " << t << endl;
imshow( "Output", dst1 );
waitKey();
return EXIT_SUCCESS;
}
void Sharpen(const Mat& myImage,Mat& Result)
{
CV_Assert(myImage.depth() == CV_8U); // accept only uchar images
const int nChannels = myImage.channels();
Result.create(myImage.size(),myImage.type());
for(int j = 1 ; j < myImage.rows-1; ++j)
{
const uchar* previous = myImage.ptr<uchar>(j - 1);
const uchar* current = myImage.ptr<uchar>(j );
const uchar* next = myImage.ptr<uchar>(j + 1);
uchar* output = Result.ptr<uchar>(j);
for(int i= nChannels;i < nChannels*(myImage.cols-1); ++i)
{
output[i] = saturate_cast<uchar>(5*current[i]
-current[i-nChannels] - current[i+nChannels] - previous[i] - next[i]);
}
}
Result.row(0).setTo(Scalar(0));
Result.row(Result.rows-1).setTo(Scalar(0));
Result.col(0).setTo(Scalar(0));
Result.col(Result.cols-1).setTo(Scalar(0));
}
基本方法
使用**filter2D()**函数或者使用基础的像素获取方法来实现掩膜的使用。代码如下:
cpp
void Sharpen(const Mat& myImage,Mat& Result)
{
//判断所获取的图像格式是否是uchar
CV_Assert(myImage.depth() == CV_8U);
const int nChannels = myImage.channels();
Result.create(myImage.size(),myImage.type());
for(int j = 1 ; j < myImage.rows-1; ++j)
{
const uchar* previous = myImage.ptr<uchar>(j - 1);
const uchar* current = myImage.ptr<uchar>(j );
const uchar* next = myImage.ptr<uchar>(j + 1);
uchar* output = Result.ptr<uchar>(j);
for(int i= nChannels;i < nChannels*(myImage.cols-1); ++i)
{
//计算锐化后的像素值
output[i] = saturate_cast<uchar>(5*current[i]-current[i-nChannels]
- current[i+nChannels] - previous[i] - next[i]);
}
}
// 将结果图像的边界设置为0,确保图像边界不会因为锐化收到影响,避免异常值
Result.row(0).setTo(Scalar(0));
Result.row(Result.rows-1).setTo(Scalar(0));
Result.col(0).setTo(Scalar(0));
Result.col(Result.cols-1).setTo(Scalar(0));
// 图像处理过程中,边界像素容易由于缺少领域信息而变得不可靠,因此需要将边界设为0,保持图像一致性。
}
filter2D函数
在opencv的图像处理中。使用这样的过滤器是如此的频繁以至于存在一个应用掩膜的函数。首先,我们需要定义一个掩膜的对象。
cpp
Mat kernel = (Mat_<char>(3,3)
<< 0, -1, 0,
-1, 5, -1,
0, -1, 0);
然后再调用**filter2D()**函数,使用该卷积。
cpp
filter2D( src, dst1, src.depth(), kernel );
该函数甚至还有第五个可选参数来指定内核的中心,第六个参数用于在将过滤后的像素存储在K中之前向其添加可选值,第七个参数用于确定在未定义操作的区域(边界)中执行什么操作。
这个函数更短、更简洁,而且由于进行了一些优化,它通常比手工编码的方法更快。例如,在我的测试中,第二个只花了13毫秒,而第一个花了31毫秒。差别很大。
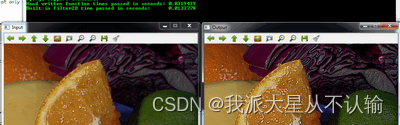
此外,笔者在学习过程中发现了一个更好的例子,链接如下: