简单来说,LLM是一个大型语言模型,而LLM Chain是由多个LLM或其他组件组成的链式结构,用于在LangChain中构建复杂的自然语言处理流程。
Direct LLM Interface: 直接大型语言模型(LLM)接口:
python
llm = OpenAI(temperature=0.9)
if prompt:
response = llm(prompt)
st.write(response)
In this approach, you're directly using an instance of the OpenAI class. This class likely has an implementation of the model that can directly process a given prompt and return a response. The process is straightforward: you provide a prompt, the model generates a response.
在这种方法中,您直接使用了OpenAI类的一个实例。这个类可能包含了模型的实现,能够直接处理给定的提示并返回响应。整个过程很直接:您提供一个提示,模型生成一个响应。
LLMChain Interface: LLMChain接口
python
from langchain.prompts import PromptTemplate.
from langchain.chains import LLMChain
template = "Write me something about {topic}".
topic_template = PromptTemplate(input_variables=['topic'],
template=template)
topic_chain = LLMChain(llm=llm, prompt=topic_template)
if prompt:
response = topic_chain.run(question).
st.write(response)
This approach involves a higher level of abstraction using the LLMChain and PromptTemplate classes. Here's a breakdown of what each part does:
这种方法涉及更高层次的抽象,使用了LLMChain和PromptTemplate类。以下是每个部分的功能分解:
PromptTemplate: Defines a structured prompt where you can specify variables that get filled in, ensuring that prompts adhere to a specific format.
PromptTemplate:定义了一个结构化的提示,其中可以指定要填充的变量,以确保提示遵循特定的格式。
LLMChain: Seems to be a chain or sequence of processes that likely utilize the underlying LLM. It takes a structured prompt and the underlying model to generate a response.
LLMChain:似乎是一个流程链或流程序列,可能利用了底层的大型语言模型(LLM)。它采用结构化提示和底层模型来生成响应。
When to use which? 它们的使用场景是什么?
Direct LLM Interface: This is suitable for more flexible or ad-hoc tasks where the prompt structure can vary widely and doesn't need to adhere to a predefined format.
直接大型语言模型(LLM)接口:这适用于更灵活或临时性的任务,其中提示结构可以有很大的变化,并且不需要遵循预定义的格式。
LLMChain Interface: This is apt for more structured tasks where consistency in the prompt format is essential. By using a chain, you can also potentially extend functionalities, like adding pre-processing or post-processing steps before and after querying the model.
LLMChain接口:这更适用于结构化的任务,其中提示格式的一致性至关重要。通过使用链式接口,您还可以潜在地扩展功能,例如在查询模型之前和之后添加预处理或后处理步骤。
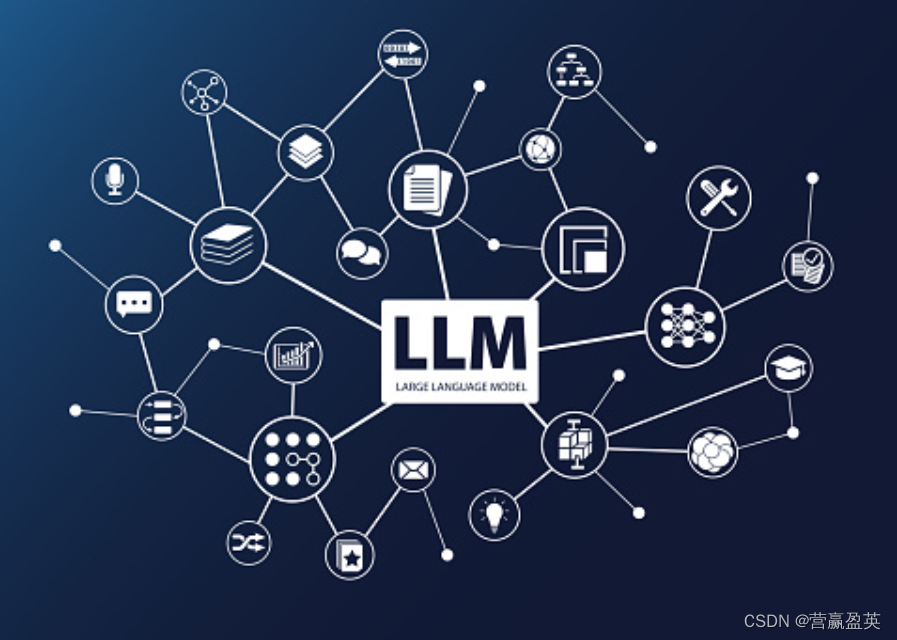