代码较为简单,很容易读懂。
# Importing necessary libraries for TensorFlow, pandas, numpy, and matplotlib
import tensorflow as tf
import pandas as pd
import numpy as np
import matplotlib.pyplot as plt
import copy
# Importing the PyTorch library
import torch
# Importing additional libraries for data manipulation, visualization, and machine learning
import copy
import seaborn as sns
from pylab import rcParams
from matplotlib import rc
from sklearn.model_selection import train_test_split
# Importing PyTorch modules for neural network implementation
from torch import nn, optim
import torch.nn.functional as F
import torch.nn as nn
# Ignoring warnings to enhance code cleanliness
import warnings
warnings.filterwarnings('ignore')
df = pd.read_csv('http://storage.googleapis.com/download.tensorflow.org/data/ecg.csv',header=None)
df.head().T
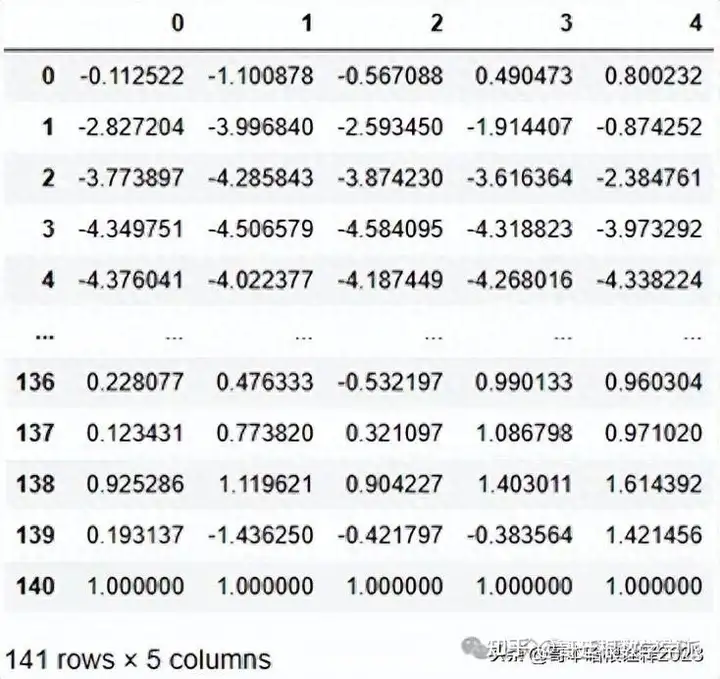
df.describe()
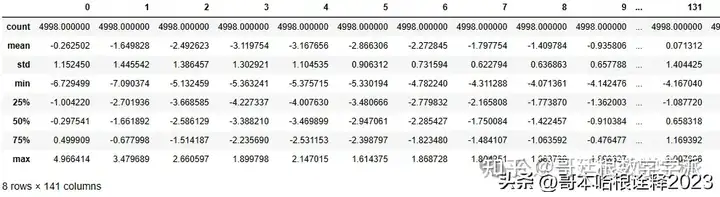
df.isna().sum()
0 0
1 0
2 0
3 0
4 0
..
136 0
137 0
138 0
139 0
140 0
Length: 141, dtype: int64
df.dtypes
0 float64
1 float64
2 float64
3 float64
4 float64
...
136 float64
137 float64
138 float64
139 float64
140 float64
Length: 141, dtype: object
new_columns = list(df.columns)
new_columns[-1] = 'target'
df.columns = new_columns
df.target.value_counts()
1.0 2919
0.0 2079
Name: target, dtype: int64
value_counts = df['target'].value_counts()
# Plotting
plt.figure(figsize=(8, 6))
value_counts.plot(kind='bar', color='skyblue')
plt.title('Value Counts of Target Column')
plt.xlabel('Target Values')
plt.ylabel('Count')
# Display the count values on top of the bars
for i, count in enumerate(value_counts):
plt.text(i, count + 0.1, str(count), ha='center', va='bottom')
plt.show()
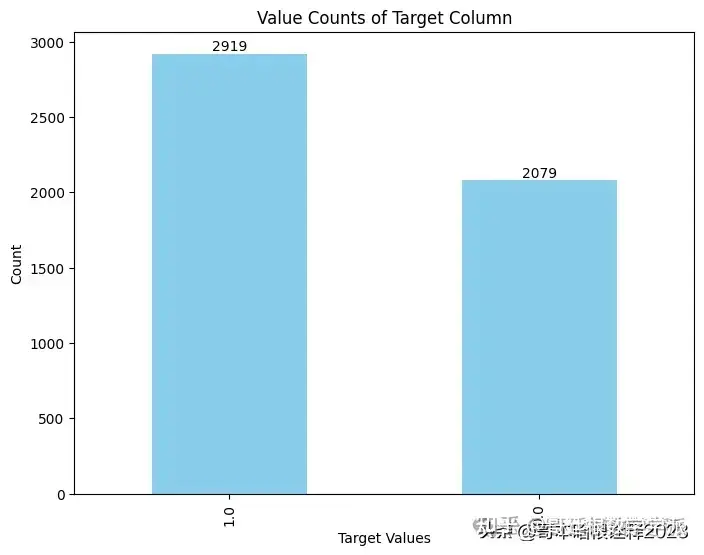
classes = df.target.unique()
def plot_ecg(data, class_name, ax, n_steps=10):
# Convert data to a DataFrame
time_series_df = pd.DataFrame(data)
# Apply a moving average for smoothing
smooth_data = time_series_df.rolling(window=n_steps, min_periods=1).mean()
# Calculate upper and lower bounds for confidence interval
deviation = time_series_df.rolling(window=n_steps, min_periods=1).std()
upper_bound = smooth_data + deviation
lower_bound = smooth_data - deviation
# Plot the smoothed data
ax.plot(smooth_data, color='black', linewidth=2)
# Plot the confidence interval
ax.fill_between(time_series_df.index, lower_bound[0], upper_bound[0], color='black', alpha=0.2)
# Set the title
ax.set_title(class_name)
# Plotting setup
fig, axs = plt.subplots(
nrows=len(classes) // 3 + 1,
ncols=3,
sharey=True,
figsize=(14, 8)
)
# Plot for each class
for i, cls in enumerate(classes):
ax = axs.flat[i]
data = df[df.target == cls].drop(labels='target', axis=1).mean(axis=0).to_numpy()
plot_ecg(data, cls, ax) # Using 'cls' directly as class name
# Adjust layout and remove extra axes
fig.delaxes(axs.flat[-1])
fig.tight_layout()
plt.show()
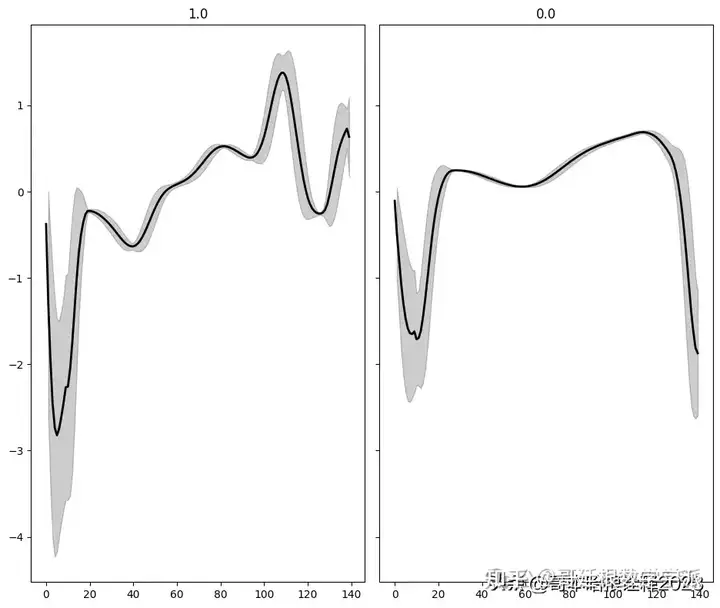
normal_df = df[df.target == 1].drop(labels='target', axis=1)
normal_df.shape
(2919, 140)
anomaly_df = df[df.target != 1].drop(labels='target', axis=1)
anomaly_df.shape
(2079, 140)
# Splitting the Dataset
# Initial Train-Validation Split:
# The dataset 'normal_df' is divided into training and validation sets.
# 15% of the data is allocated to the validation set.
# The use of 'random_state=42' ensures reproducibility.
train_df, val_df = train_test_split(
normal_df,
test_size=0.15,
random_state=42
)
# Further Splitting for Validation and Test:
# The validation set obtained in the previous step is further split into validation and test sets.
# 33% of the validation set is allocated to the test set.
# The same 'random_state=42' is used for consistency in randomization.
val_df, test_df = train_test_split(
val_df,
test_size=0.30,
random_state=42
)
# Function to Create a Dataset
def create_dataset(df):
# Convert DataFrame to a list of sequences, each represented as a list of floats
sequences = df.astype(np.float32).to_numpy().tolist()
# Convert sequences to PyTorch tensors, each with shape (sequence_length, 1, num_features)
dataset = [torch.tensor(s).unsqueeze(1).float() for s in sequences]
# Extract dimensions of the dataset
n_seq, seq_len, n_features = torch.stack(dataset).shape
# Return the dataset, sequence length, and number of features
return dataset, seq_len, n_features
# Create the training dataset from train_df
train_dataset, seq_len, n_features = create_dataset(train_df)
# Create the validation dataset from val_df
val_dataset, _, _ = create_dataset(val_df)
# Create the test dataset for normal cases from test_df
test_normal_dataset, _, _ = create_dataset(test_df)
# Create the test dataset for anomalous cases from anomaly_df
test_anomaly_dataset, _, _ = create_dataset(anomaly_df)
Implementation of LSTM-Based Autoencoder for ECG Anomaly Detection
class Encoder(nn.Module):
def __init__(self, seq_len, n_features, embedding_dim=64):
super(Encoder, self).__init__()
self.seq_len, self.n_features = seq_len, n_features
self.embedding_dim, self.hidden_dim = embedding_dim, 2 * embedding_dim
self.rnn1 = nn.LSTM(
input_size=n_features,
hidden_size=self.hidden_dim,
num_layers=1,
batch_first=True
)
self.rnn2 = nn.LSTM(
input_size=self.hidden_dim,
hidden_size=embedding_dim,
num_layers=1,
batch_first=True
)
def forward(self, x):
x = x.reshape((1, self.seq_len, self.n_features))
x, (_, _) = self.rnn1(x)
x, (hidden_n, _) = self.rnn2(x)
return hidden_n.reshape((self.n_features, self.embedding_dim))
class Decoder(nn.Module):
def __init__(self, seq_len, input_dim=64, n_features=1):
super(Decoder, self).__init__()
self.seq_len, self.input_dim = seq_len, input_dim
self.hidden_dim, self.n_features = 2 * input_dim, n_features
self.rnn1 = nn.LSTM(
input_size=input_dim,
hidden_size=input_dim,
num_layers=1,
batch_first=True
)
self.rnn2 = nn.LSTM(
input_size=input_dim,
hidden_size=self.hidden_dim,
num_layers=1,
batch_first=True
)
self.output_layer = nn.Linear(self.hidden_dim, n_features)
def forward(self, x):
x = x.repeat(self.seq_len, self.n_features)
x = x.reshape((self.n_features, self.seq_len, self.input_dim))
x, (hidden_n, cell_n) = self.rnn1(x)
x, (hidden_n, cell_n) = self.rnn2(x)
x = x.reshape((self.seq_len, self.hidden_dim))
return self.output_layer(x)
class Autoencoder(nn.Module):
def __init__(self, seq_len, n_features, embedding_dim=64):
super(Autoencoder, self).__init__()
self.encoder = Encoder(seq_len, n_features, embedding_dim).to(device)
self.decoder = Decoder(seq_len, embedding_dim, n_features).to(device)
def forward(self, x):
x = self.encoder(x)
x = self.decoder(x)
return x
device = torch.device("cuda" if torch.cuda.is_available() else "cpu")
model = Autoencoder(seq_len, n_features, 128)
model = model.to(device)
Training and Visualization of ECG Autoencoder Model
def plot_input_reconstruction(model, dataset, epoch):
model = model.eval()
plt.figure(figsize=(10, 5))
# Take the first sequence from the dataset
seq_true = dataset[0].to(device)
seq_pred = model(seq_true)
with torch.no_grad():
# Squeeze the sequences to ensure they are 1-dimensional
input_sequence = seq_true.squeeze().cpu().numpy()
reconstruction_sequence = seq_pred.squeeze().cpu().numpy()
# Check the shape after squeezing
if input_sequence.ndim != 1 or reconstruction_sequence.ndim != 1:
raise ValueError("Input and reconstruction sequences must be 1-dimensional after squeezing.")
# Plotting the sequences
plt.plot(input_sequence, label='Input Sequence', color='black')
plt.plot(reconstruction_sequence, label='Reconstruction Sequence', color='red')
plt.fill_between(range(len(input_sequence)), input_sequence, reconstruction_sequence, color='gray', alpha=0.5)
plt.title(f'Input vs Reconstruction - Epoch {epoch}')
plt.legend()
plt.show()
import torch
import numpy as np
import copy
def train_model(model, train_dataset, val_dataset, n_epochs, save_path):
optimizer = torch.optim.Adam(model.parameters(), lr=1e-3, weight_decay=1e-4)
criterion = torch.nn.L1Loss(reduction='sum').to(device)
history = {'train': [], 'val': []}
best_model_wts = copy.deepcopy(model.state_dict())
best_loss = float('inf')
for epoch in range(1, n_epochs + 1):
model.train()
train_losses = []
for seq_true in train_dataset:
optimizer.zero_grad()
seq_true = seq_true.to(device)
seq_pred = model(seq_true)
loss = criterion(seq_pred, seq_true)
loss.backward()
optimizer.step()
train_losses.append(loss.item())
val_losses = []
model.eval()
with torch.no_grad():
for seq_true in val_dataset:
seq_true = seq_true.to(device)
seq_pred = model(seq_true)
loss = criterion(seq_pred, seq_true)
val_losses.append(loss.item())
train_loss = np.mean(train_losses)
val_loss = np.mean(val_losses)
history['train'].append(train_loss)
history['val'].append(val_loss)
if val_loss < best_loss:
best_loss = val_loss
best_model_wts = copy.deepcopy(model.state_dict())
# Save the best model weights
print("Saving best model")
torch.save(model.state_dict(), save_path)
print(f'Epoch {epoch}: train loss {train_loss} val loss {val_loss}')
if epoch == 1 or epoch % 5 == 0:
plot_input_reconstruction(model, val_dataset, epoch)
# Load the best model weights before returning
model.load_state_dict(best_model_wts)
return model.eval(), history
save_path = 'best_model.pth' # Replace with your actual path
model, history = train_model(model, train_dataset, val_dataset, 100, save_path)
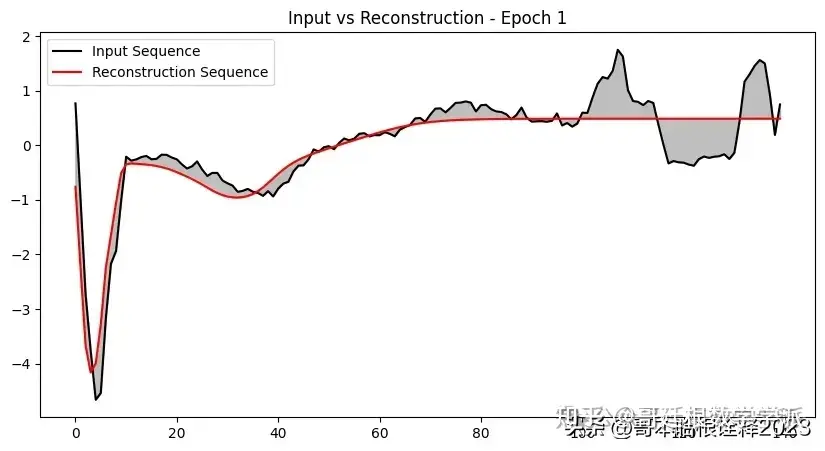
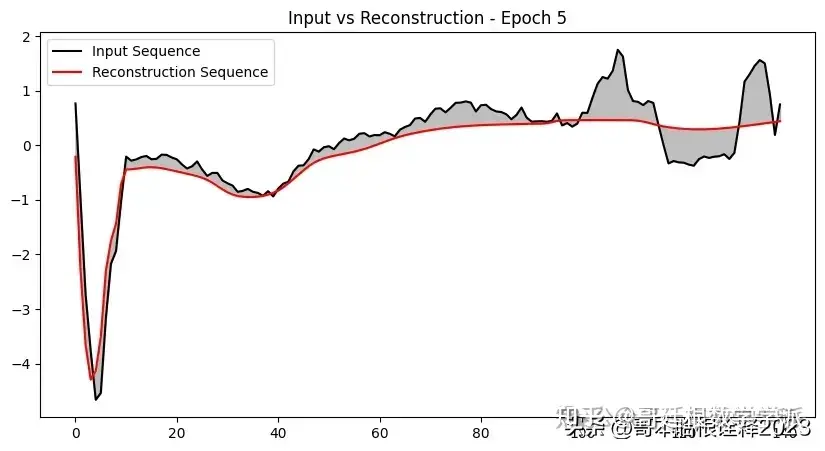
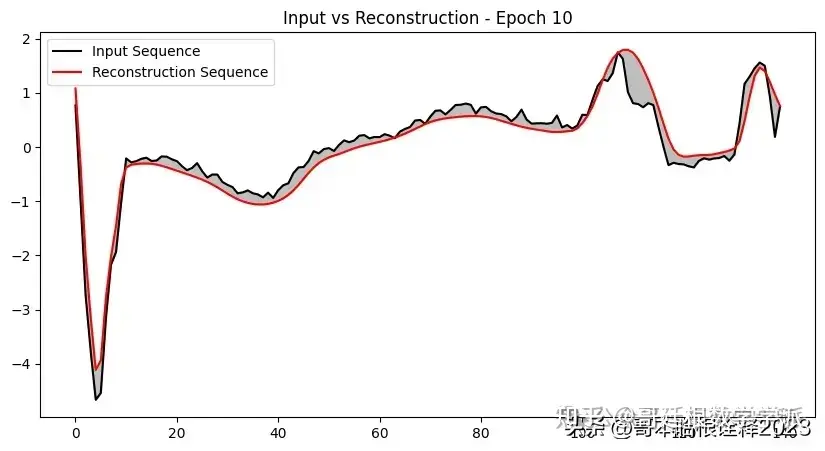
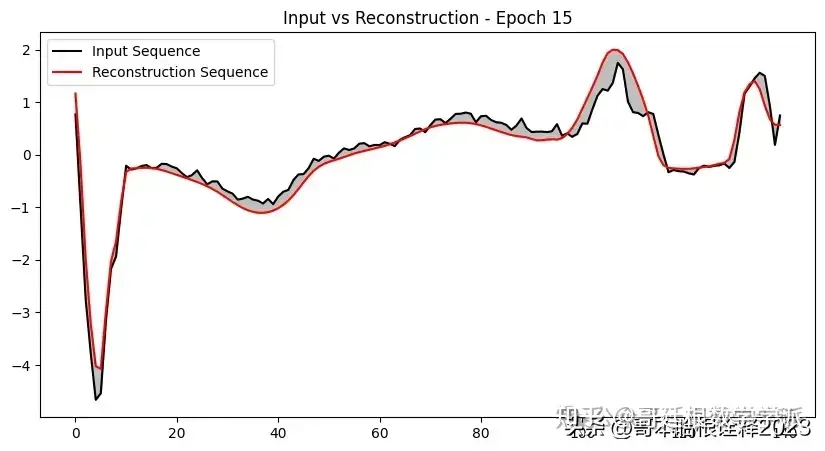
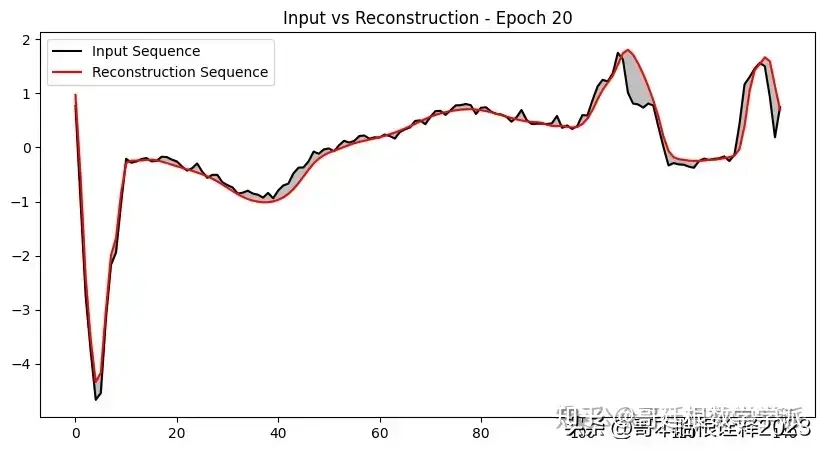
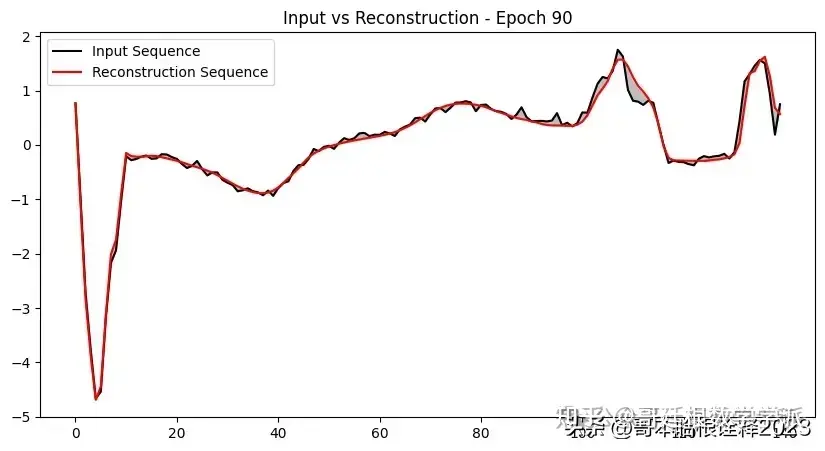
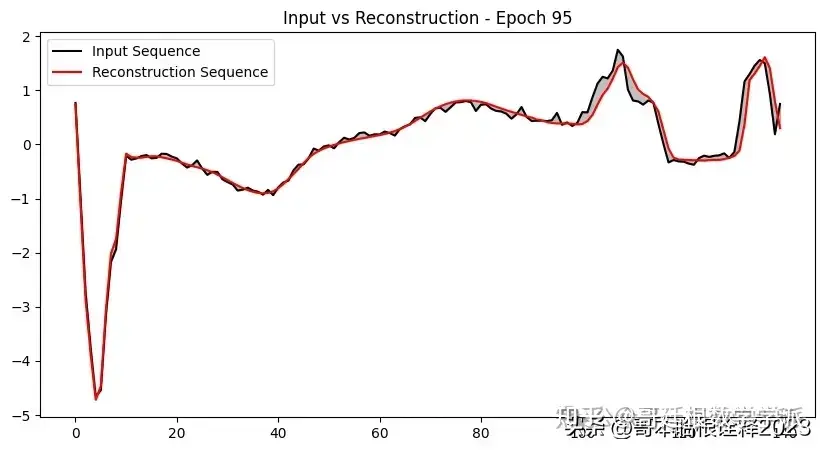
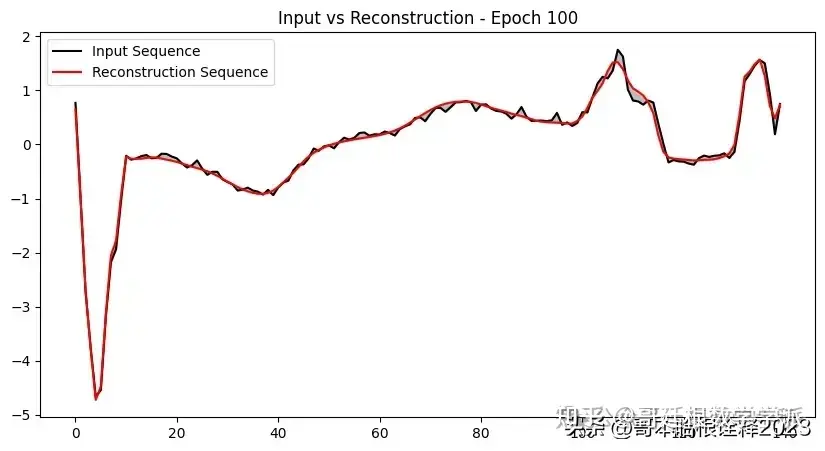
ax = plt.figure().gca()
ax.plot(history['train'],label='Train Loss', color='black')
ax.plot(history['val'],label='Val Loss', color='red')
plt.ylabel('Loss')
plt.xlabel('Epoch')
plt.legend(['train', 'test'])
plt.title('Loss over training epochs')
plt.show();
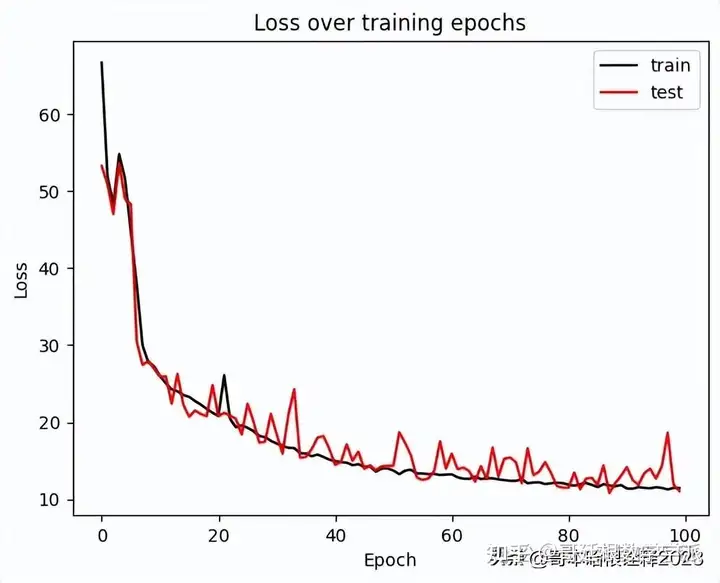
ECG Anomaly Detection Model Evaluation and Visualization
model = Autoencoder(seq_len, n_features, 128)
model.load_state_dict(torch.load('best_model.pth'))
model = model.to(device)
model.eval()
Autoencoder(
(encoder): Encoder(
(rnn1): LSTM(1, 256, batch_first=True)
(rnn2): LSTM(256, 128, batch_first=True)
)
(decoder): Decoder(
(rnn1): LSTM(128, 128, batch_first=True)
(rnn2): LSTM(128, 256, batch_first=True)
(output_layer): Linear(in_features=256, out_features=1, bias=True)
)
)
def predict(model, dataset):
predictions, losses = [], []
criterion = nn.L1Loss(reduction='sum').to(device)
with torch.no_grad():
model = model.eval()
for seq_true in dataset:
seq_true = seq_true.to(device)
seq_pred = model(seq_true)
loss = criterion(seq_pred, seq_true)
predictions.append(seq_pred.cpu().numpy().flatten())
losses.append(loss.item())
return predictions, losses
_, losses = predict(model, train_dataset)
sns.distplot(losses, bins=50, kde=True, label='Train',color='black');
#Visualising train loss
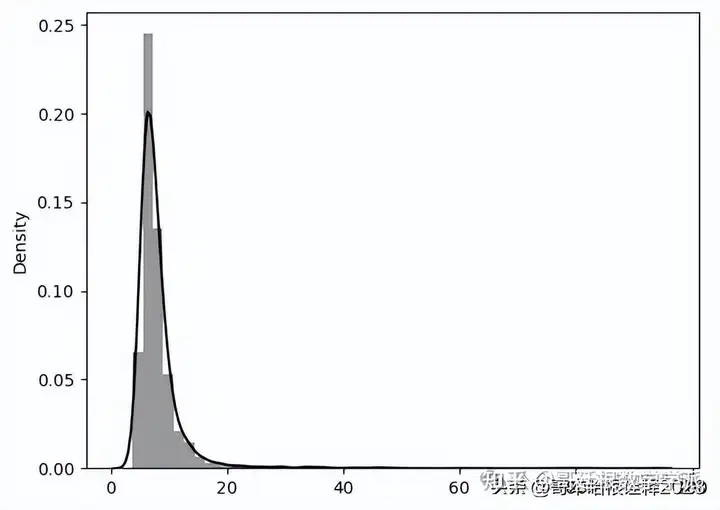
Threshold = 25
predictions, pred_losses = predict(model, test_normal_dataset)
sns.distplot(pred_losses, bins=50, kde=True,color='black')
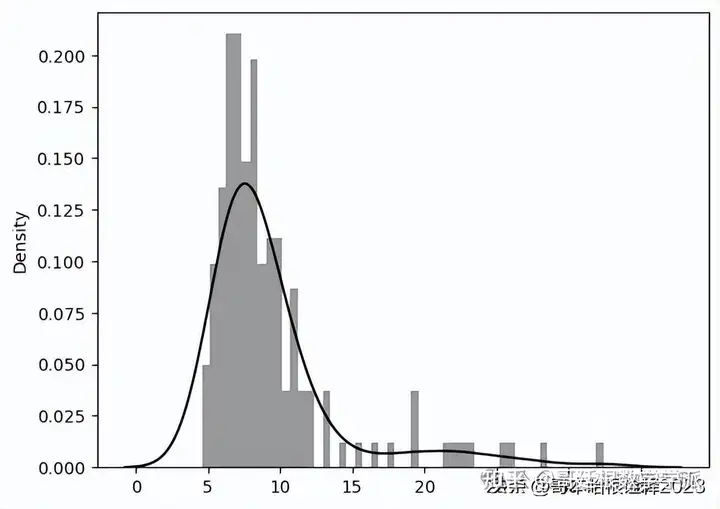
correct = sum(l <= 25 for l in pred_losses)
print(f'Correct normal predictions: {correct}/{len(test_normal_dataset)}')
Correct normal predictions: 141/145
anomaly_dataset = test_anomaly_dataset[:len(test_normal_dataset)]
predictions, pred_losses = predict(model, anomaly_dataset)
sns.distplot(pred_losses, bins=50, kde=True,color='red');
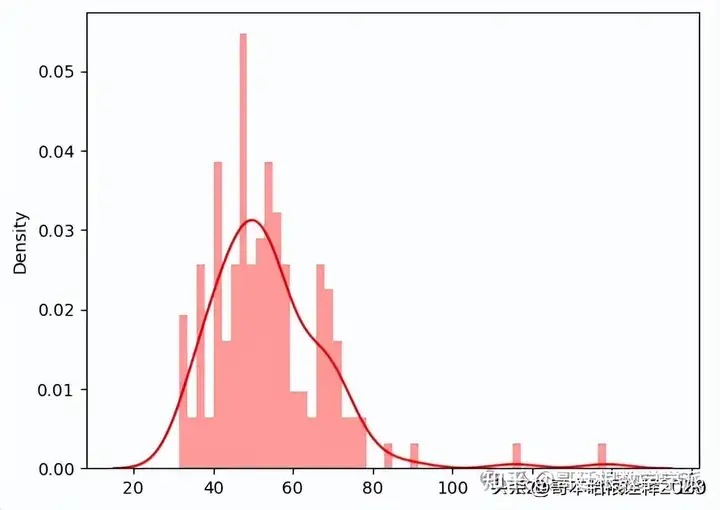
correct = sum(l > 25 for l in pred_losses)
print(f'Correct anomaly predictions: {correct}/{len(anomaly_dataset)}')
Correct anomaly predictions: 145/145
def plot_prediction(data, model, title, ax):
predictions, pred_losses = predict(model, [data])
ax.plot(data, label='true',color='black')
ax.plot(predictions[0], label='reconstructed',color='red')
ax.set_title(f'{title} (loss: {np.around(pred_losses[0], 2)})')
ax.legend()
fig, axs = plt.subplots(
nrows=2,
ncols=4,
sharey=True,
sharex=True,
figsize=(22, 8)
)
for i, data in enumerate(test_normal_dataset[:4]):
plot_prediction(data, model, title='Normal', ax=axs[0, i])
for i, data in enumerate(test_anomaly_dataset[:4]):
plot_prediction(data, model, title='Anomaly', ax=axs[1, i])
fig.tight_layout();
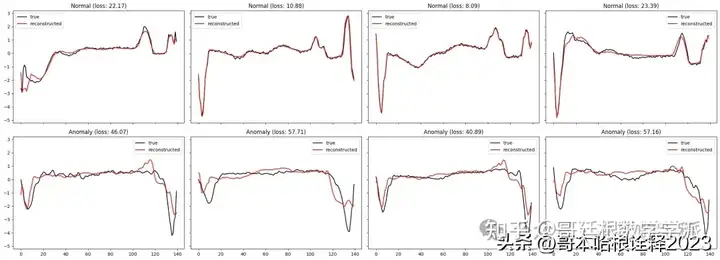
工学博士,担任《Mechanical System and Signal Processing》《中国电机工程学报》《控制与决策》等期刊审稿专家,擅长领域:现代信号处理,机器学习,深度学习,数字孪生,时间序列分析,设备缺陷检测、设备异常检测、设备智能故障诊断与健康管理PHM等。