最近在做一个知识库问答项目,就是现在大模型浪潮下比较火的 RAG 应用。LangChain 可以说是 RAG 最受欢迎的工具,因此我首选 LangChain 来快速构建我的应用。坦白来讲 LangChain 本身一套对于组件的定义已经让我感觉很复杂,为什么采用 f-string
或 string.format
就能完成的事情必须要抽出一个这么复杂的对象。
当然上面种种原因可能是我不理解 LangChain 设计之禅,但是下面这个坑确实实实在在让我对 LangChain 感到失望的地方。
起因
事情起因很简单,我很快构建好了一个最简单的 RAG 应用,无非以下三步:
- 用户输入
query
。 - 将用户的
query
进行embedding
之后进行相似度检索,并按照阈值过滤相似度低的文本。 - 整合检索的文本并按照一定格式送入大模型。
但是在第二步出现了问题。我在测试的时候发现我总是会召回很多无关的文本,并且我把相似度阈值调高之后,仍然没有把这些不相干的文本过滤掉,这让我十分困惑,但是翻看 LangChain 调用代码之后我瞬间一个恍然大明白,这里 xxx 有坑!
回顾
LangChain 中对于文本检索有个类叫做 BaseRetriever
,刚刚开始我只使用向量数据库进行最简单的检索,但是考虑后续会加入多种检索方式,为了组合方便我采用了 VectorStoreRetriever
进行检索。基本代码是这样的:
# 省略加载db的过程
retriever = db.as_retriever()
docs = retriever.get_relevant_documents(query, score_threshold=threshold)
就是这样,我把 threshold
调高也不会过滤那些显然无关的文本。于是我就想看看 LangChain 是怎么调用的。
排查
首先看一下 get_relevant_documents()
这个函数调用流程,它在 BaseRetriever
是这么定义的,源码贴脸警告!!!
def get_relevant_documents(
self,
query: str,
*,
callbacks: Callbacks = None,
tags: Optional[List[str]] = None,
metadata: Optional[Dict[str, Any]] = None,
run_name: Optional[str] = None,
**kwargs: Any,
) -> List[Document]:
"""Retrieve documents relevant to a query.
Users should favor using `.invoke` or `.batch` rather than
`get_relevant_documents directly`.
Args:
query: string to find relevant documents for
callbacks: Callback manager or list of callbacks
tags: Optional list of tags associated with the retriever. Defaults to None
These tags will be associated with each call to this retriever,
and passed as arguments to the handlers defined in `callbacks`.
metadata: Optional metadata associated with the retriever. Defaults to None
This metadata will be associated with each call to this retriever,
and passed as arguments to the handlers defined in `callbacks`.
run_name: Optional name for the run.
Returns:
List of relevant documents
"""
from langchain_core.callbacks.manager import CallbackManager
callback_manager = CallbackManager.configure(
callbacks,
None,
verbose=kwargs.get("verbose", False),
inheritable_tags=tags,
local_tags=self.tags,
inheritable_metadata=metadata,
local_metadata=self.metadata,
)
run_manager = callback_manager.on_retriever_start(
dumpd(self),
query,
name=run_name,
run_id=kwargs.pop("run_id", None),
)
try:
_kwargs = kwargs if self._expects_other_args else {}
if self._new_arg_supported:
result = self._get_relevant_documents(
query, run_manager=run_manager, **_kwargs
)
else:
result = self._get_relevant_documents(query, **_kwargs)
except Exception as e:
run_manager.on_retriever_error(e)
raise e
else:
run_manager.on_retriever_end(
result,
)
return result
这个函数文档说建议使用 .invoke()
而不是直接调用这个函数,但是 .invoke()
也是间接调用这个函数。这个函数的流程还是挺清晰的,它会处理一些 callback
然后继续调用 _get_relevant_documents()
这个函数,这个函数由每个子类自己实现,我们看看 VectorStoreRetriever
对于这个函数的实现:
def _get_relevant_documents(
self, query: str, *, run_manager: CallbackManagerForRetrieverRun
) -> List[Document]:
if self.search_type == "similarity":
docs = self.vectorstore.similarity_search(query, **self.search_kwargs)
elif self.search_type == "similarity_score_threshold":
docs_and_similarities = (
self.vectorstore.similarity_search_with_relevance_scores(
query, **self.search_kwargs
)
)
docs = [doc for doc, _ in docs_and_similarities]
elif self.search_type == "mmr":
docs = self.vectorstore.max_marginal_relevance_search(
query, **self.search_kwargs
)
else:
raise ValueError(f"search_type of {self.search_type} not allowed.")
return docs
这个函数本身逻辑也不难,就是按照 search_type
的不同,调用 vectorstore
的不同方法。所以这个 VectorStoreRetriever
其实就是对 vectorstore
的再一次封装,核心还是调用 vectorstore
的方法。
回到函数本身来,这里出现了一个新的变量叫 search_type
,这个其实在 VectorStoreRetriever
中给出了:
class VectorStoreRetriever(BaseRetriever):
"""Base Retriever class for VectorStore."""
vectorstore: VectorStore
"""VectorStore to use for retrieval."""
search_type: str = "similarity"
"""Type of search to perform. Defaults to "similarity"."""
search_kwargs: dict = Field(default_factory=dict)
"""Keyword arguments to pass to the search function."""
allowed_search_types: ClassVar[Collection[str]] = (
"similarity",
"similarity_score_threshold",
"mmr",
)
其实当我们调用 vectorstore.as_retriever()
时候也可以指定该参数,我们看看 as_retriever()
这个函数的实现。
def as_retriever(self, **kwargs: Any) -> VectorStoreRetriever:
"""Return VectorStoreRetriever initialized from this VectorStore.
Args:
search_type (Optional[str]): Defines the type of search that
the Retriever should perform.
Can be "similarity" (default), "mmr", or
"similarity_score_threshold".
search_kwargs (Optional[Dict]): Keyword arguments to pass to the
search function. Can include things like:
k: Amount of documents to return (Default: 4)
score_threshold: Minimum relevance threshold
for similarity_score_threshold
fetch_k: Amount of documents to pass to MMR algorithm (Default: 20)
lambda_mult: Diversity of results returned by MMR;
1 for minimum diversity and 0 for maximum. (Default: 0.5)
filter: Filter by document metadata
Returns:
VectorStoreRetriever: Retriever class for VectorStore.
Examples:
.. code-block:: python
# Retrieve more documents with higher diversity
# Useful if your dataset has many similar documents
docsearch.as_retriever(
search_type="mmr",
search_kwargs={'k': 6, 'lambda_mult': 0.25}
)
# Fetch more documents for the MMR algorithm to consider
# But only return the top 5
docsearch.as_retriever(
search_type="mmr",
search_kwargs={'k': 5, 'fetch_k': 50}
)
# Only retrieve documents that have a relevance score
# Above a certain threshold
docsearch.as_retriever(
search_type="similarity_score_threshold",
search_kwargs={'score_threshold': 0.8}
)
# Only get the single most similar document from the dataset
docsearch.as_retriever(search_kwargs={'k': 1})
# Use a filter to only retrieve documents from a specific paper
docsearch.as_retriever(
search_kwargs={'filter': {'paper_title':'GPT-4 Technical Report'}}
)
"""
tags = kwargs.pop("tags", None) or []
tags.extend(self._get_retriever_tags())
return VectorStoreRetriever(vectorstore=self, **kwargs, tags=tags)
可以看到这里的 search_type
支持 similarity
、 mmr
和 similarity_score_threshold
三种,默认的是 similarity
。看到这里,第一个引起我疑惑的地方来了,这个 similarity
和 similarity_score_threshold
有什么区别呢?
下面我们分两条线进行分析,按照不同调用链看看他们到底是什么意思。
分支一:similarity
在分支一,会调用 vetorstore.similarity_search()
方法,这是 VectorStore
的一个抽象方法,需要子类自己实现,我们看看 FAISS
是怎么实现的。
def similarity_search(
self,
query: str,
k: int = 4,
filter: Optional[Union[Callable, Dict[str, Any]]] = None,
fetch_k: int = 20,
**kwargs: Any,
) -> List[Document]:
"""Return docs most similar to query.
Args:
query: Text to look up documents similar to.
k: Number of Documents to return. Defaults to 4.
filter: (Optional[Dict[str, str]]): Filter by metadata. Defaults to None.
fetch_k: (Optional[int]) Number of Documents to fetch before filtering.
Defaults to 20.
Returns:
List of Documents most similar to the query.
"""
docs_and_scores = self.similarity_search_with_score(
query, k, filter=filter, fetch_k=fetch_k, **kwargs
)
return [doc for doc, _ in docs_and_scores]
这里可以看到他是调用了 similarity_search_with_score()
方法,然后把结果中的 score
给略去了,这里不得不吐槽这个调用是不是脱裤子放屁,明明可以写在一个方法里面,传入一个 flag
标识是否要返回分数就可以解决,非要封装成两个方法。吐槽结束继续查看 similarity_search_with_score()
方法。
def similarity_search_with_score(
self,
query: str,
k: int = 4,
filter: Optional[Union[Callable, Dict[str, Any]]] = None,
fetch_k: int = 20,
**kwargs: Any,
) -> List[Tuple[Document, float]]:
"""Return docs most similar to query.
Args:
query: Text to look up documents similar to.
k: Number of Documents to return. Defaults to 4.
filter (Optional[Dict[str, str]]): Filter by metadata.
Defaults to None. If a callable, it must take as input the
metadata dict of Document and return a bool.
fetch_k: (Optional[int]) Number of Documents to fetch before filtering.
Defaults to 20.
Returns:
List of documents most similar to the query text with
L2 distance in float. Lower score represents more similarity.
"""
embedding = self._embed_query(query)
docs = self.similarity_search_with_score_by_vector(
embedding,
k,
filter=filter,
fetch_k=fetch_k,
**kwargs,
)
return docs
这个方法就是将 query
进行 embedding
之后,根据向量进行查询,调用了 similarity_search_with_score_by_vector()
方法,我们继续跟踪。
def similarity_search_with_score_by_vector(
self,
embedding: List[float],
k: int = 4,
filter: Optional[Union[Callable, Dict[str, Any]]] = None,
fetch_k: int = 20,
**kwargs: Any,
) -> List[Tuple[Document, float]]:
"""Return docs most similar to query.
Args:
embedding: Embedding vector to look up documents similar to.
k: Number of Documents to return. Defaults to 4.
filter (Optional[Union[Callable, Dict[str, Any]]]): Filter by metadata.
Defaults to None. If a callable, it must take as input the
metadata dict of Document and return a bool.
fetch_k: (Optional[int]) Number of Documents to fetch before filtering.
Defaults to 20.
**kwargs: kwargs to be passed to similarity search. Can include:
score_threshold: Optional, a floating point value between 0 to 1 to
filter the resulting set of retrieved docs
Returns:
List of documents most similar to the query text and L2 distance
in float for each. Lower score represents more similarity.
"""
faiss = dependable_faiss_import()
vector = np.array([embedding], dtype=np.float32)
if self._normalize_L2:
faiss.normalize_L2(vector)
scores, indices = self.index.search(vector, k if filter is None else fetch_k)
docs = []
if filter is not None:
filter_func = self._create_filter_func(filter)
for j, i in enumerate(indices[0]):
if i == -1:
# This happens when not enough docs are returned.
continue
_id = self.index_to_docstore_id[i]
doc = self.docstore.search(_id)
if not isinstance(doc, Document):
raise ValueError(f"Could not find document for id {_id}, got {doc}")
if filter is not None:
if filter_func(doc.metadata):
docs.append((doc, scores[0][j]))
else:
docs.append((doc, scores[0][j]))
score_threshold = kwargs.get("score_threshold")
if score_threshold is not None:
cmp = (
operator.ge
if self.distance_strategy
in (DistanceStrategy.MAX_INNER_PRODUCT, DistanceStrategy.JACCARD)
else operator.le
)
docs = [
(doc, similarity)
for doc, similarity in docs
if cmp(similarity, score_threshold)
]
return docs[:k]
这里就是调用了 FAISS
创建数据库时的索引进行相似度的检索,检索之后,会取关键词参数中是否有 score_threshold
,如果之前的调用中传入了阈值分数,则会进行相似度的过滤。因为我遇到的问题就是无法过滤无关内容,因此这里过滤引起了我的注意。
分析一下这个过滤的代码:
- 定义比较算子,如果距离策略采用最大内积 或者杰卡德系数就采用大于,否则就是小于。
- 按照算子将相似度和阈值计算来进行过滤。
这里我恍然大悟,我赶紧查看了一下我自己采用了什么距离策略,翻看源码得知 FAISS
默认采用的距离策略是 DistanceStrategy.EUCLIDEAN_DISTANCE
。也就是欧式距离,所以算子应该采用小于,也就是说保留相似度低于阈值的。
这里我恍然大明白,这很好理解,如果你采用欧式距离作为相似度计算,确实应该值越小表示越相似,所以我之前调高相似度阈值反而没有过滤是正常的,因为调的越大,反而过滤力度越小!
这就很反直觉,假如我采用内积作为距离策略,则我之前的行为就是正确的。LangChain 并没有对这个情况进行合理的处理,甚至没有看到 LangChain 对此有一个提示。
分支一就此结束,虽然已经解决了我最开始的问题,但是我们还是继续看看分支二。
分支二:similarity_score_threshold
在分支二,VectorStoreRetriever
会调用 vectorstore.similarity_search_with_relevance_scores()
方法。这里多了一个概念叫 relevance_scores
我们姑且暂时叫做相关性分数,这个和之前相似度有啥关系呢,我们先不揭晓答案,先看看这个函数做了啥。
def similarity_search_with_relevance_scores(
self,
query: str,
k: int = 4,
**kwargs: Any,
) -> List[Tuple[Document, float]]:
"""Return docs and relevance scores in the range [0, 1].
0 is dissimilar, 1 is most similar.
Args:
query: input text
k: Number of Documents to return. Defaults to 4.
**kwargs: kwargs to be passed to similarity search. Should include:
score_threshold: Optional, a floating point value between 0 to 1 to
filter the resulting set of retrieved docs
Returns:
List of Tuples of (doc, similarity_score)
"""
score_threshold = kwargs.pop("score_threshold", None)
docs_and_similarities = self._similarity_search_with_relevance_scores(
query, k=k, **kwargs
)
if any(
similarity < 0.0 or similarity > 1.0
for _, similarity in docs_and_similarities
):
warnings.warn(
"Relevance scores must be between"
f" 0 and 1, got {docs_and_similarities}"
)
if score_threshold is not None:
docs_and_similarities = [
(doc, similarity)
for doc, similarity in docs_and_similarities
if similarity >= score_threshold
]
if len(docs_and_similarities) == 0:
warnings.warn(
"No relevant docs were retrieved using the relevance score"
f" threshold {score_threshold}"
)
return docs_and_similarities
这个函数文档中写到返回文档和对应的相关性分数,相关性分数在0到1之间,0表示不相似,1表示最相似。这个流程也不复杂,但是这里需要理一下流程:
- 把关键词参数中
score_threshold
给弹了出来,这意味着后面传入的关键词参数中不会有score_threshold
这个参数。(这里又是一个让人吐槽的地方,后面再说。) - 调用
_similarity_search_with_relevance_scores()
函数,(这里吐槽一下函数名里面是relevance_scores
但是接受变量确实docs_and_similarities
为什么要搞这么多复杂的名称呢?) - 如果第一步中获得的
score_threshold
不为空则进行过滤,保留相似度大于阈值的文档,注意这里并没有分支一最后的算子判断。
到这里我有点懵了,因为引入了一个 relevance_scores
但是似乎和相似度概念差不多,包括在函数文档以及函数内部都是混用的,所以我很好奇为啥要引入一个新概念。但是有一点确认的是,相关性分数越高,文本相似度越高,无论你采用了什么样的距离策略都是这样的。
让我们继续观察调用链,看看第二步中的函数:
def _similarity_search_with_relevance_scores(
self,
query: str,
k: int = 4,
**kwargs: Any,
) -> List[Tuple[Document, float]]:
"""
Default similarity search with relevance scores. Modify if necessary
in subclass.
Return docs and relevance scores in the range [0, 1].
0 is dissimilar, 1 is most similar.
Args:
query: input text
k: Number of Documents to return. Defaults to 4.
**kwargs: kwargs to be passed to similarity search. Should include:
score_threshold: Optional, a floating point value between 0 to 1 to
filter the resulting set of retrieved docs
Returns:
List of Tuples of (doc, similarity_score)
"""
relevance_score_fn = self._select_relevance_score_fn()
docs_and_scores = self.similarity_search_with_score(query, k, **kwargs)
return [(doc, relevance_score_fn(score)) for doc, score in docs_and_scores]
函数文档再次说明返回文档和对应的相关性分数,相关性分数在0到1之间,0表示不相似,1表示最相似。函数也很简单,首先调用了一个相关性分数函数 ,然后调用 similarity_search_with_score()
得到文档和相似度,最后将相似度按照相关性分数函数做一个转换,至此两个分支走到了一起,最终都是调用 similarity_search_with_score()
。
这里就可以回答为什么之前要 pop
关键词参数中的阈值,因为如果关键词参数中有 score_threshold
,那么在 similarity_search_with_score()
这步就会进行过滤,但是这个函数过滤是按照距离策略不同选不同算子,分支二过滤直接按照大于进行过滤。
到了这里在混乱的概念中有个初步的印象,可以得到如下三个观点:
- 相似度和相关性是不同的,至少在 LangChain 中是这样定义的,虽然在函数中两个变量混用,但是按照行为上确实是不同的两个定义。
- 相关性分数越大,则文本越相关;相似度则是根据距离策略决定,对于欧式距离,相似度越小,文本越相关。
- 相关性分数通过相似度计算出来的,计算函数就是
_select_relevance_score_fn()
。
我感觉到了胜利的曙光,只要查明这个 _select_relevance_score_fn()
具体做了啥,就知道这两个定义如何关联的了。
def _select_relevance_score_fn(self) -> Callable[[float], float]:
"""
The 'correct' relevance function
may differ depending on a few things, including:
- the distance / similarity metric used by the VectorStore
- the scale of your embeddings (OpenAI's are unit normed. Many others are not!)
- embedding dimensionality
- etc.
Vectorstores should define their own selection based method of relevance.
"""
raise NotImplementedError
这里可以看到不同的 vectorstore
实现是不同的,这里我当然讨论的是 FAISS
,我们看 LangChain 在 FAISS
中如何定义的。
def _select_relevance_score_fn(self) -> Callable[[float], float]:
"""
The 'correct' relevance function
may differ depending on a few things, including:
- the distance / similarity metric used by the VectorStore
- the scale of your embeddings (OpenAI's are unit normed. Many others are not!)
- embedding dimensionality
- etc.
"""
if self.override_relevance_score_fn is not None:
return self.override_relevance_score_fn
# Default strategy is to rely on distance strategy provided in
# vectorstore constructor
if self.distance_strategy == DistanceStrategy.MAX_INNER_PRODUCT:
return self._max_inner_product_relevance_score_fn
elif self.distance_strategy == DistanceStrategy.EUCLIDEAN_DISTANCE:
# Default behavior is to use euclidean distance relevancy
return self._euclidean_relevance_score_fn
elif self.distance_strategy == DistanceStrategy.COSINE:
return self._cosine_relevance_score_fn
else:
raise ValueError(
"Unknown distance strategy, must be cosine, max_inner_product,"
" or euclidean"
)
这里面可以看到 LangChain 对 FAISS
支持三种距离策略,每个策略有不同的计算公式,这里我直接贴出三个计算公式:
@staticmethod
def _max_inner_product_relevance_score_fn(distance: float) -> float:
"""Normalize the distance to a score on a scale [0, 1]."""
if distance > 0:
return 1.0 - distance
return -1.0 * distance
@staticmethod
def _euclidean_relevance_score_fn(distance: float) -> float:
"""Return a similarity score on a scale [0, 1]."""
# The 'correct' relevance function
# may differ depending on a few things, including:
# - the distance / similarity metric used by the VectorStore
# - the scale of your embeddings (OpenAI's are unit normed. Many
# others are not!)
# - embedding dimensionality
# - etc.
# This function converts the euclidean norm of normalized embeddings
# (0 is most similar, sqrt(2) most dissimilar)
# to a similarity function (0 to 1)
return 1.0 - distance / math.sqrt(2)
@staticmethod
def _cosine_relevance_score_fn(distance: float) -> float:
"""Normalize the distance to a score on a scale [0, 1]."""
return 1.0 - distance
这里我们都考虑 embedding
向量经过 L2
正则化,则内积和余弦相似度计算应该相同,实际上在内积上有存在问题。
首先内积为负值,直接取其相反数没有问题,因为负相关也是相关,但是当为正值时就有问题了,举个例子,假如采用内积计算,得到一个相似度为 0.7 的值,理应这两个比较相关,但是通过这个相关性函数得到只有 0.3 反而变成不相关了。这三个公式只有欧式距离是正确的。
实验
上面说明 LangChain 对于不同距离策略,没能给出正确的过滤方式,且对于相关性的计算,搞反了语义相似性和相关性的关系。
对于 VectorStore
而言,如果采用欧氏距离 ,采用 similarity_search_with_relevance_scores()
才能正确按照相似度过滤文档,相应的 VectorStoreRetriever
中的 search_type
应该采用 similarity_score_threshold
。
如果采用最大内积 ,采用 similarity_search_with_score()
才能正确检索文档,相应的 VectorStoreRetriever
中的 search_type
应该采用 similarity
。
除此之外的组合都不能按照预期的检索出文档。
为了证明我的猜想,下面进行实验环节。
版本信息
我采用的 LangChain 版本如下:
pip show langchain
Name: langchain
Version: 0.1.16
Summary: Building applications with LLMs through composability
Home-page: https://github.com/langchain-ai/langchain
Author:
Author-email:
License: MIT
Location: D:\miniconda3\envs\new\Lib\site-packages
Requires: aiohttp, dataclasses-json, jsonpatch, langchain-community, langchain-core, langchain-text-splitters, langsmith, numpy, pydantic, PyYAML, requests, SQLAlchemy, tenacity
Required-by:
实验过程
导包环节
import numpy as np
from langchain_community.vectorstores.faiss import FAISS, DistanceStrategy
from langchain_openai import OpenAIEmbeddings
我将下面三句毫不相关的话为文档,建立三个不同距离策略的向量库。
text_list = ["今天天气真好", "我喜欢吃苹果", "猴子排序很不可靠"]
embeddings = OpenAIEmbeddings(
openai_api_base="xxx",
openai_api_key="xxx"
)
embedding_list = [embeddings.embed_query(text) for text in text_list]
OpenAIEmbeddings
会将向量进行 L2
正则化。
for embedding in embedding_list:
print(np.linalg.norm(embedding))
0.9999999999999989
1.0000000000000002
1.0000000000000002
建立下面三个向量库:
vs1 = FAISS.from_embeddings(zip(text_list, embedding_list), embeddings, normalize_L2=True, distance_strategy=DistanceStrategy.EUCLIDEAN_DISTANCE)
vs2 = FAISS.from_embeddings(zip(text_list, embedding_list), embeddings, normalize_L2=True, distance_strategy=DistanceStrategy.MAX_INNER_PRODUCT)
vs3 = FAISS.from_embeddings(zip(text_list, embedding_list), embeddings, normalize_L2=True, distance_strategy=DistanceStrategy.COSINE)
我们先都检索一下,确保三个向量库中内容都存在。
print(vs1.similarity_search_with_score("今天天气真好"))
print(vs2.similarity_search_with_score("今天天气真好"))
print(vs3.similarity_search_with_score("今天天气真好"))
[(Document(page_content='今天天气真好'), 0.0), (Document(page_content='我喜欢吃苹果'), 0.40074897), (Document(page_content='猴子排序很不可靠'), 0.5013859)]
[(Document(page_content='今天天气真好'), 0.9999843), (Document(page_content='我喜欢吃苹果'), 0.7995081), (Document(page_content='猴子排序很不可靠'), 0.74908566)]
[(Document(page_content='今天天气真好'), 0.0), (Document(page_content='我喜欢吃苹果'), 0.40074897), (Document(page_content='猴子排序很不可靠'), 0.5013859)]
这里可以看到采用余弦相似度 作为距离策略的向量库,检索分数和欧氏距离 相同,这里我认为是 FAISS
支持的是欧氏距离 和内积 ,虽然正则化后内积 和余弦相似度 等价,但是建立索引时候 FAISS
并不支持余弦相似度 ,于是按照欧氏距离建立的索引。一个猜测,没有证实。
按照上面的猜想,在 VectorStore
中,如果采用 similarity_search_with_score()
给出分数阈值,只有采用内积的能正确过滤文档。
print(vs1.similarity_search_with_score("今天天气真好", score_threshold=0.8))
print(vs2.similarity_search_with_score("今天天气真好", score_threshold=0.8))
print(vs3.similarity_search_with_score("今天天气真好", score_threshold=0.8))
[(Document(page_content='今天天气真好'), 0.0), (Document(page_content='我喜欢吃苹果'), 0.40074897), (Document(page_content='猴子排序很不可靠'), 0.5011895)]
[(Document(page_content='今天天气真好'), 0.9999846)]
[(Document(page_content='今天天气真好'), 0.0), (Document(page_content='我喜欢吃苹果'), 0.40074897), (Document(page_content='猴子排序很不可靠'), 0.5011895)]
事实果真如此,如果采用 similarity_search_with_relevance_scores()
给出阈值分数,只有采用欧氏距离能正确过滤文档。
print(vs1.similarity_search_with_relevance_scores("今天天气真好", score_threshold=0.8))
print(vs2.similarity_search_with_relevance_scores("今天天气真好", score_threshold=0.8))
print(vs3.similarity_search_with_relevance_scores("今天天气真好", score_threshold=0.8))
[(Document(page_content='今天天气真好'), 0.999978158576509)]
d:\miniconda3\envs\new\Lib\site-packages\langchain_core\vectorstores.py:342](): UserWarning: No relevant docs were retrieved using the relevance score threshold 0.8 warnings.warn(
[]
[(Document(page_content='今天天气真好'), 1.0)]
结果也是如此,你可能会疑问余弦相似度 也能正确输出,这是因为首先在距离计算时,它采用了欧氏距离 ,然后相关性分数时采用余弦相似度 也是错的,两次错误导致语义和相关性的关系是对的。但是好的程序不能靠 BUG 过活!
在 VectorStore
层面,证明了我的结论的正确性,那按照调用链来说 VectorStoreRetriever
也满足我的结论,但是还是继续实验。
当 search_type
为 similarity
时,只有内积是正确召回。
search_type = "similarity"
search_kwargs = {
"score_threshold": 0.8
}
re1 = vs1.as_retriever(search_type=search_type, search_kwargs=search_kwargs)
re2 = vs2.as_retriever(search_type=search_type, search_kwargs=search_kwargs)
re3 = vs3.as_retriever(search_type=search_type, search_kwargs=search_kwargs)
print(re1.get_relevant_documents("今天天气真好"))
print(re2.get_relevant_documents("今天天气真好"))
print(re3.get_relevant_documents("今天天气真好"))
[Document(page_content='今天天气真好'), Document(page_content='我喜欢吃苹果'), Document(page_content='猴子排序很不可靠')]
[Document(page_content='今天天气真好')]
[Document(page_content='今天天气真好'), Document(page_content='我喜欢吃苹果'), Document(page_content='猴子排序很不可靠')]
当 search_type
为 similarity_score_threshold
时,只有欧氏距离是正确召回。
search_type = "similarity_score_threshold"
search_kwargs = {
"score_threshold": 0.8
}
re1 = vs1.as_retriever(search_type=search_type, search_kwargs=search_kwargs)
re2 = vs2.as_retriever(search_type=search_type, search_kwargs=search_kwargs)
re3 = vs3.as_retriever(search_type=search_type, search_kwargs=search_kwargs)
print(re1.get_relevant_documents("今天天气真好"))
print(re2.get_relevant_documents("今天天气真好"))
print(re3.get_relevant_documents("今天天气真好"))
[Document(page_content='今天天气真好')]
d:\miniconda3\envs\zhiguo\lib\site-packages\langchain_core\vectorstores.py:323](): UserWarning: No relevant docs were retrieved using the relevance score threshold 0.8 warnings.warn(
[]
[Document(page_content='今天天气真好')]
这里余弦相似度正确召回原因同上,靠 BUG 过活罢了。
实验最后再重申一下我的结论:
对于 VectorStore
而言,如果采用欧氏距离 ,采用 similarity_search_with_relevance_scores()
才能正确按照相似度过滤文档,相应的 VectorStoreRetriever
中的 search_type
应该采用 similarity_score_threshold
。
如果采用最大内积 ,采用 similarity_search_with_score()
才能正确检索文档,相应的 VectorStoreRetriever
中的 search_type
应该采用 similarity
。
注:当前实验只对 LangChain 封装的 FAISS
负责,别的向量库不负责。
后记
如何学习AI大模型?
我在一线互联网企业工作十余年里,指导过不少同行后辈。帮助很多人得到了学习和成长。
我意识到有很多经验和知识值得分享给大家,也可以通过我们的能力和经验解答大家在人工智能学习中的很多困惑,所以在工作繁忙的情况下还是坚持各种整理和分享。但苦于知识传播途径有限,很多互联网行业朋友无法获得正确的资料得到学习提升,故此将并将重要的AI大模型资料包括AI大模型入门学习思维导图、精品AI大模型学习书籍手册、视频教程、实战学习等录播视频免费分享出来。
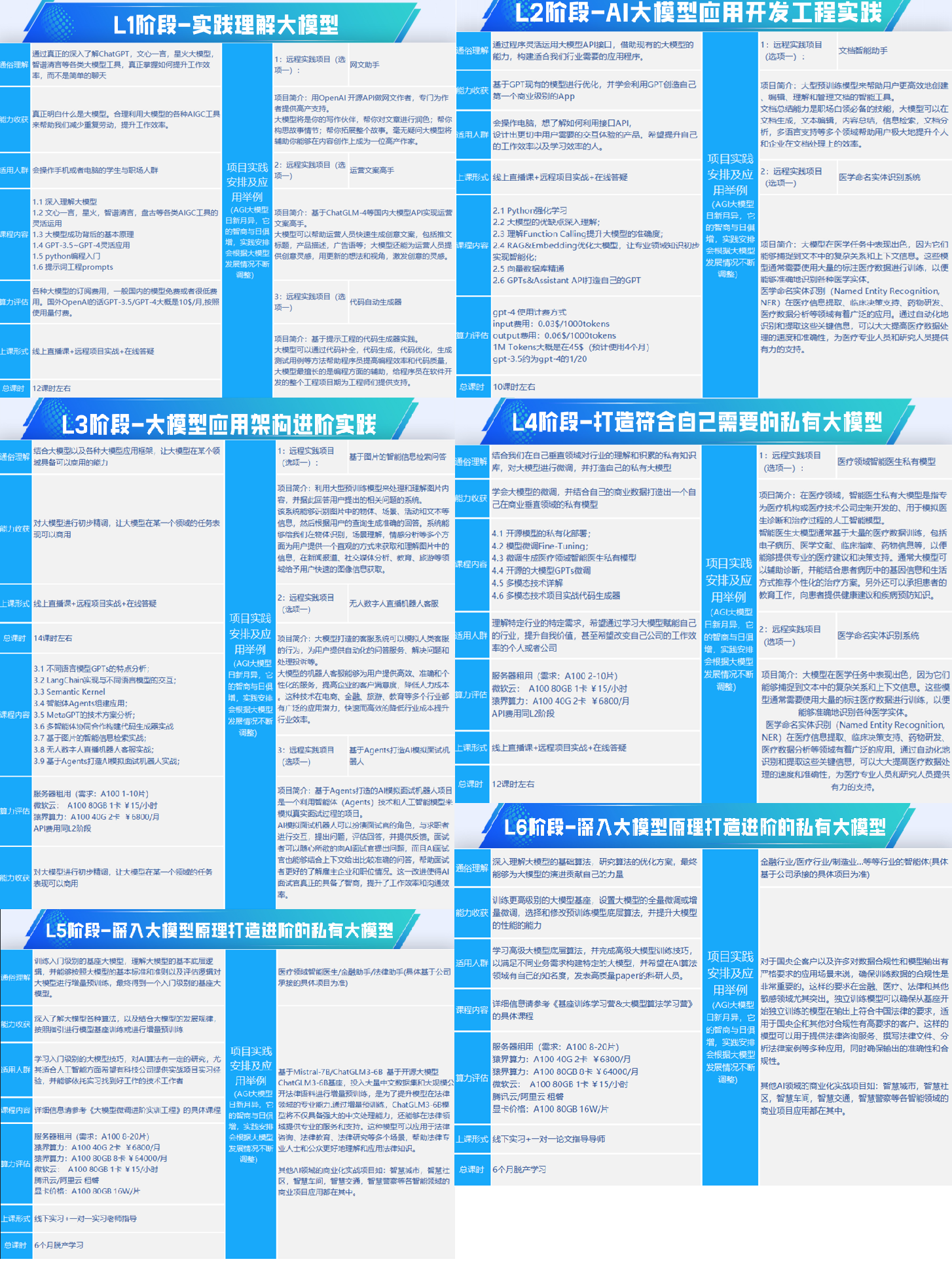
第一阶段: 从大模型系统设计入手,讲解大模型的主要方法;
第二阶段: 在通过大模型提示词工程从Prompts角度入手更好发挥模型的作用;
第三阶段: 大模型平台应用开发借助阿里云PAI平台构建电商领域虚拟试衣系统;
第四阶段: 大模型知识库应用开发以LangChain框架为例,构建物流行业咨询智能问答系统;
第五阶段: 大模型微调开发借助以大健康、新零售、新媒体领域构建适合当前领域大模型;
第六阶段: 以SD多模态大模型为主,搭建了文生图小程序案例;
第七阶段: 以大模型平台应用与开发为主,通过星火大模型,文心大模型等成熟大模型构建大模型行业应用。
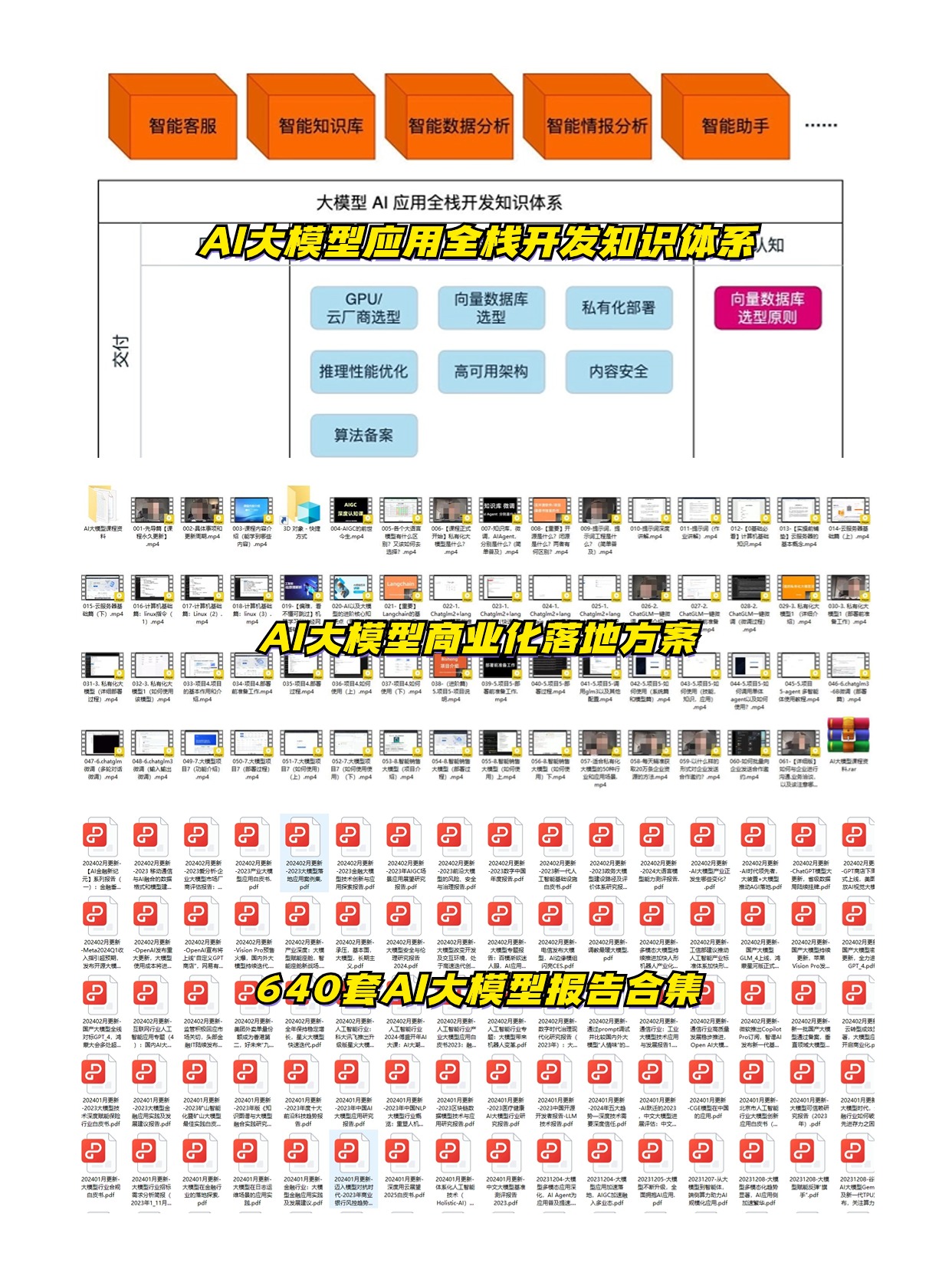
👉学会后的收获:👈
• 基于大模型全栈工程实现(前端、后端、产品经理、设计、数据分析等),通过这门课可获得不同能力;
• 能够利用大模型解决相关实际项目需求: 大数据时代,越来越多的企业和机构需要处理海量数据,利用大模型技术可以更好地处理这些数据,提高数据分析和决策的准确性。因此,掌握大模型应用开发技能,可以让程序员更好地应对实际项目需求;
• 基于大模型和企业数据AI应用开发,实现大模型理论、掌握GPU算力、硬件、LangChain开发框架和项目实战技能, 学会Fine-tuning垂直训练大模型(数据准备、数据蒸馏、大模型部署)一站式掌握;
• 能够完成时下热门大模型垂直领域模型训练能力,提高程序员的编码能力: 大模型应用开发需要掌握机器学习算法、深度学习框架等技术,这些技术的掌握可以提高程序员的编码能力和分析能力,让程序员更加熟练地编写高质量的代码。
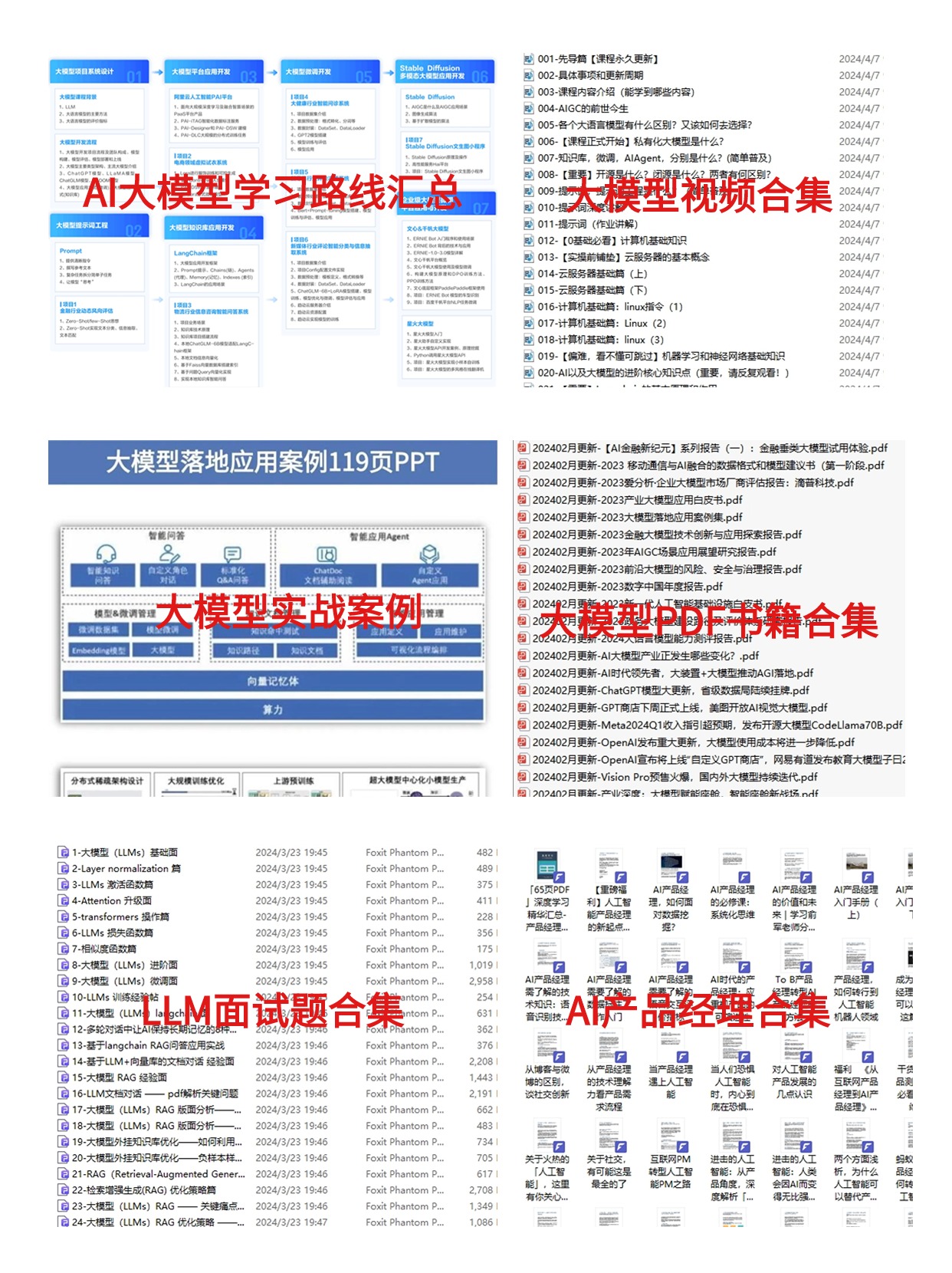
1.AI大模型学习路线图
2.100套AI大模型商业化落地方案
3.100集大模型视频教程
4.200本大模型PDF书籍
5.LLM面试题合集
6.AI产品经理资源合集
👉获取方式:
😝有需要的小伙伴,可以保存图片到wx扫描二v码免费领取【保证100%免费】🆓
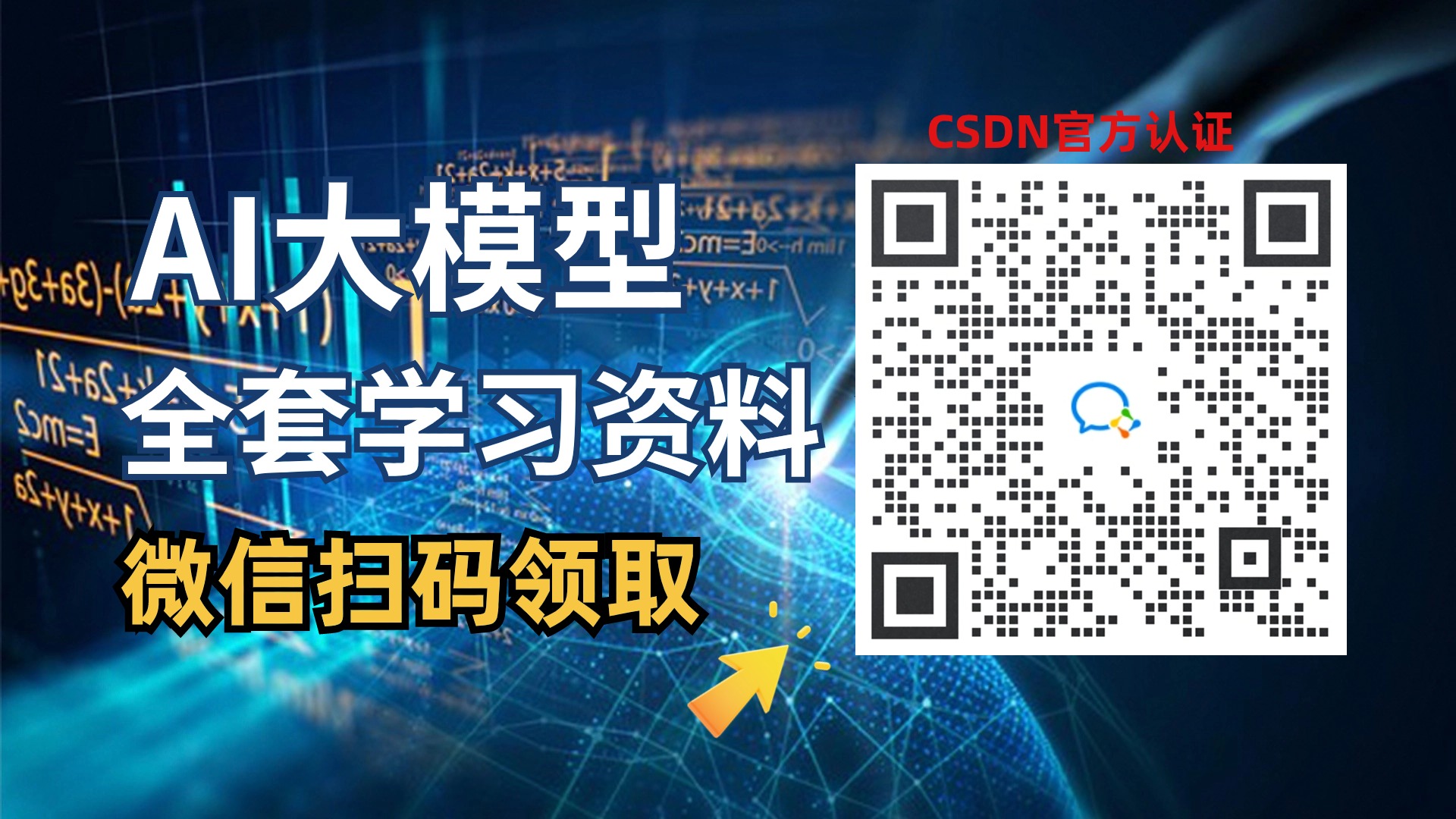