一 图像可行驶区域方案
1.1 标定场景
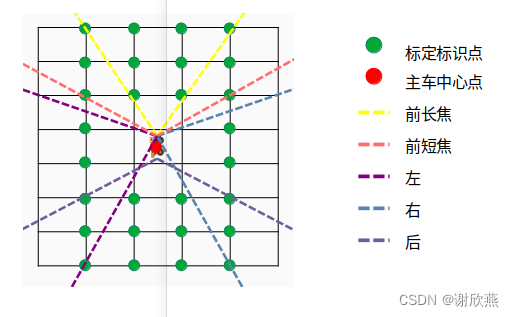
1.2 标定步骤
- 设计一定间距标定场,在标定场固定位置设置摄像头标定标识点。
- 主车开到标定场固定位置
- 录制主车在该位置各个摄像头数据,通过摄像头捕获图像获取图像上关键点坐标pts-2d
- 基于标定场设计,计算图像关键点对应车体坐标系中的3d坐标pts-3d
- 通过cv2.findHomography(obj_points, img_points, cv2.RANSAC, 5.0) 获取相机坐标系到地面的单应性变换矩阵H
1.3 实车使用
实时获取车辆行进过程中的固定纵向距离的轨迹点信息,使用单应性变换矩阵H反向计算轨迹信息在图像中的投影位置,从而获取到图像中检测目标的距离区间。
二 初步验证结果
'''
Author: XIEXINYAN "1532642675@qq.com"
Date: 2024-07-01 04:52:07
LastEditors: XIEXINYAN "1532642675@qq.com"
LastEditTime: 2024-07-03 05:40:06
FilePath: /202407/hom_matrix.py
Description:
Copyright (c) 2024 by 1532642675@qq.com, All Rights Reserved.
'''
import cv2
import numpy as np
import os
import argparse
class Counter:
cnt = 0
def __init__(self):
Counter.cnt +=1
@classmethod
def get_counter(cls):
return cls.cnt
class Calibrate:
def __init__(self, pattern_size, real_square_size, offset_x, offset_y):
self.pattern_size = pattern_size
self.real_square_size = real_square_size
self.offset_x = offset_x
self.offset_y = offset_y
# 1. 检测棋格板角点
def find_chessboard_corners(self, image, color=(0, 255, 0), vis=False, save=False, calib=False):
self.image = image.copy()
image_painted = image.copy()
gray = cv2.cvtColor(image, cv2.COLOR_BGR2GRAY)
ret, corners = cv2.findChessboardCorners(gray, pattern_size, None)
if ret:
criteria = (cv2.TERM_CRITERIA_EPS + cv2.TERM_CRITERIA_MAX_ITER, 30, 0.001)
#按照pattern_size[0]的个数排序,绘制的第一组数据个数=pattern_size[0]的个数
self.corner = cv2.cornerSubPix(gray, corners, (11, 11), (-1, -1), criteria)
if self.corner is not None:
'''
0 3 6 2 5 8 6 3 0 8 5 2
1 4 7 1 4 7 7 4 1 7 4 1
2 5 8 0 3 6 8 5 2 6 3 0
'''
if vis:
#判断corners排序顺序
index_list = []
if (self.corner[0][0][1]<self.corner[1][0][1]
and self.corner[0][0][0]<self.corner[np.prod(self.pattern_size)-1][0][0]):
print("mode A")
elif (self.corner[0][0][1]>self.corner[1][0][1]
and self.corner[0][0][0]<self.corner[np.prod(self.pattern_size)-1][0][0]):
print("mode B")
for j in range(pattern_size[1]):
for i in range(pattern_size[0]):
index_list.append(pattern_size[0]-1-i+j*pattern_size[0])
elif (self.corner[0][0][1]<self.corner[1][0][1]
and self.corner[0][0][0]>self.corner[np.prod(self.pattern_size)-1][0][0]):
print("mode C")
index_list = []
for j in range(pattern_size[1]):
for i in range(pattern_size[0]):
index_list.append(i+(pattern_size[1]-1-j)*pattern_size[0])
print(index_list)
elif (self.corner[0][0][1]>self.corner[1][0][1]
and self.corner[0][0][0]>self.corner[np.prod(self.pattern_size)-1][0][0]):
print("mode D")
index_list = []
for j in range(pattern_size[1]):
for i in range(pattern_size[0]):
index_list.append(i+(pattern_size[1]-1-j)*pattern_size[0])
print(index_list)
else:
print("horizonal mode")
self.corner = self.corner[index_list]
cv2.circle(image_painted, (int(self.corner[0][0][0]), int(self.corner[0][0][1])), 25, (0, 255, 255), -1)
cv2.circle(image_painted, (int(self.corner[1][0][0]), int(self.corner[1][0][1])), 25, (0, 0, 255), -1)
for corner in self.corner:
cv2.circle(image_painted, (int(corner[0][0]), int(corner[0][1])), 5, (0, 0, 255), -1)
cv2.drawChessboardCorners(image_painted, pattern_size, self.corner, True)
cv2.imshow("chess",image_painted)
if save:
instance=Counter()
cv2.imwrite(str(instance.get_counter())+".jpg", image)
if calib:
self.find_hom_matrix(vis=True, save=True)
return True
return False
def world_chess_board_loc(self):
# 初始化obj_points数组,注意使用齐次坐标(即每个点都是[x, y, 1])
obj_points = np.zeros((np.prod(self.pattern_size), 3), dtype=np.float32)
# x_start是每行开始的x坐标 # y_start是每行开始的y坐标
# # 填充obj_points数组
'''
从左上角开始
0 3 6 9
1 4 7 10
2 5 8 11
'''
# obj_points =np.array([
# [ 0., 0., 1.],[ 0., 60., 1.],[ 0., 120., 1.],
# [ 60., 0., 1.],[ 60., 60., 1.],[ 60., 120., 1.],
# [120., 0., 1.],[120., 60., 1.],[120., 120., 1.],
# [180., 0., 1.],[180., 60., 1.],[180., 120., 1.]], dtype=np.float32)
index = 0
for i in range(pattern_size[1]):
for j in range(pattern_size[0]):
# 计算x和y坐标
x = i * self.real_square_size
y = j * self.real_square_size
# 将点添加到obj_points数组中,注意使用齐次坐标形式
obj_points[index, :] = [x, y, 1.0]
index += 1
return obj_points
'''
将世界坐标系下点转化为wraped图像上点
'''
def world_transation(self):
obj_points = self.world_chess_board_loc()
obj_points_t = obj_points.copy()
obj_points_t[:,0] += self.offset_x
obj_points_t[:,1] += self.offset_y
obj_points_t[:,:2] *= 1000
return obj_points_t
def world_to_image(self, img, vis=False, save=False):
# obj_points 是世界坐标系下的点,需要是齐次坐标形式
obj_points_t = self.world_transation()
# 使用np.dot进行矩阵乘法,并计算归一化的图像坐标
img_points_homogeneous = np.dot(self.H, obj_points_t.T).T
img_points = img_points_homogeneous[:, :2] / img_points_homogeneous[:, 2:].reshape(-1, 1)
for pt_2d in img_points:
cv2.circle(img, (int(pt_2d[0]), int(pt_2d[1])), 5, (0, 0, 255), -1)
if vis:
cv2.imshow("eval image", img)
if save:
counter = Counter()
cv2.imwrite("eval_"+str(counter.get_counter())+".jpg",img)
return
def find_hom_matrix(self, vis=False, save=False):
# 世界坐标值 【横,纵,高】
obj_points = self.world_chess_board_loc()
# 偏移到某个坐标系
obj_points[:,0] += self.offset_x
obj_points[:,1] += self.offset_y
obj_points[:,:2] *= 1000
# print(obj_points)
# 3. 计算单应性矩阵
img_points = self.corner.reshape(-1, 1, 2).astype(np.float32)
self.H, _ = cv2.findHomography(obj_points, img_points, cv2.RANSAC, 5.0)
warped_image = cv2.warpPerspective(self.image, self.H, (self.image.shape[1], self.image.shape[0]))
if vis:
cv2.imshow("wrapped image",warped_image)
cv2.waitKey()
if save:
cv2.imwrite("wrapped_"+str(Counter.cnt)+".jpg", warped_image)
return
def find_qrcode_corners(image, vis=False):
gray = cv2.cvtColor(image, cv2.COLOR_BGR2GRAY)
# 二值化
_, binary = cv2.threshold(gray, 127, 255, cv2.THRESH_BINARY)
# # 查找轮廓
# contours, _ = cv2.findContours(binary, cv2.RETR_EXTERNAL, cv2.CHAIN_APPROX_SIMPLE)
# 查找轮廓(OpenCV 4.x)
contours, hierarchy = cv2.findContours(binary, cv2.RETR_EXTERNAL, cv2.CHAIN_APPROX_SIMPLE)
#打印轮廓信息
print("总轮廓数:", len(contours))
# 筛选和排序轮廓(这里我们假设二维码是最大的轮廓)
contours = sorted(contours, key=cv2.contourArea, reverse=True)
qrcode_contour = contours[0]
# 多边形近似
epsilon = 0.02 * cv2.arcLength(qrcode_contour, True)
approx = cv2.approxPolyDP(qrcode_contour, epsilon, True)
image_out = image.copy()
# 提取角点坐标
corners = approx.reshape((-1, 2))
# 在原图上绘制角点
if len(corners) == 4:
for corner in corners:
cv2.circle(image_out, (int(corner[0]), int(corner[1])), 25, (0, 0, 255), -1)
# 显示图像
cv2.imshow('QRCode Corners', image_out)
cv2.drawContours(image_out, qrcode_contour, -1, (0, 255, 0), 3)
cv2.imshow('Corners', image_out)
cv2.waitKey(1)
def argParser():
parser = argparse.ArgumentParser()
parser.add_argument('--rows', type=int, default=3,help='chess board raw num')
parser.add_argument('--cols', type=int, default=4,help='chess board col num')
parser.add_argument('--online',type=bool, default=False, help='online camera calib')
opt = parser.parse_args()
return opt
if __name__ == '__main__':
opt = argParser()
pattern_size = (opt.rows, opt.cols)
calib = Calibrate(pattern_size, 0.06, 0.3, 0.7)
cap = cv2.VideoCapture(0)
if not cap.isOpened():
exit()
print(" now start calib %d\n",opt.online)
if opt.online:
while True:
ret, frame = cap.read()
flag = calib.find_chessboard_corners(frame, vis=True, save=True, calib=True)
cv2.waitKey(1)
if flag:
break
else:
path = "img"
image_list = os.listdir(path)
for img in image_list:
img_path = os.path.join(path,img)
if os.path.isfile(img_path):
frame = cv2.imread(img_path)
else:
continue
print("image path is: ",img_path)
flag = calib.find_chessboard_corners(frame, vis=True, save=False, calib=True)
cv2.waitKey(1)
if flag:
break
print(calib.H)
print(" now start eval \n")
if opt.online:
while True:
ret, frame = cap.read()
# 如果正确读取帧,ret为True
if not ret:
print("无法接收帧,请退出")
break
calib.world_to_image(frame,True, False)
# 显示实时画面
cv2.imshow('raw', frame)
# 按 'q' 键退出循环
if cv2.waitKey(1) & 0xFF == ord('q'):
break
# 释放摄像头资源并关闭所有窗口
cap.release()
else:
path = "img"
image_list = os.listdir(path)
for img in image_list:
img_path = os.path.join(path,img)
if os.path.isfile(img_path):
frame = cv2.imread(img_path)
else:
continue
calib.world_to_image(frame, True, True)
# 显示实时画面
cv2.imshow('raw', frame)
# 按 'q' 键退出循环
if cv2.waitKey(1) & 0xFF == ord('q'):
break
cv2.destroyAllWindows()
三 存在问题
- 本方案在设计标定场过程中需要精确计算每个相机的FOV,与地面的交点,设计地面标志物,使得每个相机可以准确有效的提取地面标志物
- 会受到道路坡度和车辆pitch角影响,需要模拟分析pitch角对距离的影响度