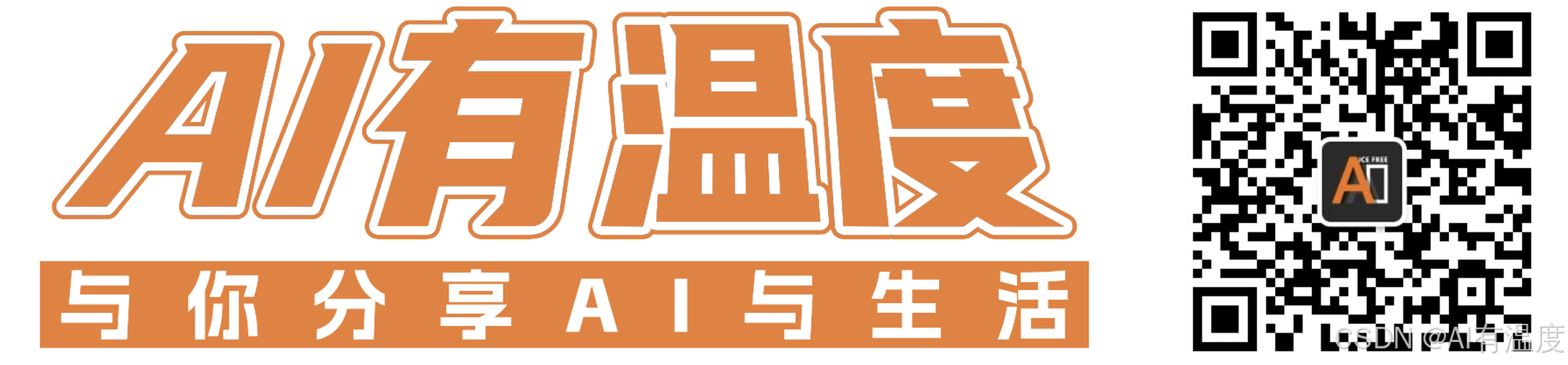
在介绍完PyTorch
中的广播运算后,继续为大家介绍PyTorch
的内置数学运算:
- 首先对内置函数有一个功能印象,知道它的存在,使用时再查具体怎么用
- 其次,我还会介绍
PyTorch
科学运算的注意事项与一些实用小技巧
1 基本数学运算
函数 | 功能 |
---|---|
torch.add(t1,t2 ) | 等效于t1+t2 |
torch.sub(t1,t2) | 等效于t1-t2 |
torch.mul(t1,t2) | 等效于t1*t2 |
torch.div(t1,t2) | 等效于t1/t2 |
2 进行数值调整
Python
t = torch.randn(5)
t
# tensor([ 0.3806, 0.9064, -1.9179, 2.0816, -0.4153])
返回绝对值
Python
torch.abs(t)
# tensor([0.3806, 0.9064, 1.9179, 2.0816, 0.4153])
返回相反数
Python
torch.neg(t)
# tensor([-0.3806, -0.9064, 1.9179, -2.0816, 0.4153])
四舍五入
Python
torch.round(t)
# tensor([ 0., 1., -2., 2., -0.])
向上取整
Python
torch.ceil(t)
# tensor([ 1., 1., -1., 3., -0.])
向下取整
Python
torch.floor(t)
# tensor([ 0., 0., -2., 2., -1.])
注:虽然此类型函数并不会对原对象进行调整,而是输出新的结果。
Python
# t本身并未发生变化
t
# tensor([ 0.3806, 0.9064, -1.9179, 2.0816, -0.4153])
若要对原对象本身进行修改,可使用方法_()
。
Python
# 使用方法_()
t.round_()
# tensor([ 0., 1., -2., 2., -0.])
# 原对象也进行了改变
t
# tensor([ 0., 1., -2., 2., -0.])
3 常用科学计算
需要注意的有以下两点:
- 因为张量能指定在
CPU
或者GPU
上运行,因此tensor
的大多数科学计算只能作用于tensor
对象,而不能和Python
对象混用
Python
# 计算3的3次方
torch.pow(3, 3)
# TypeError
torch.pow(torch.tensor(3), 3)
# tensor(27)
- 由于会涉及
GPU
计算,所以对运算结果一般是小数的函数,要求函数只能输入浮点型张量,而不能是整型
Python
t = torch.arange(1, 4)
t.dtype
# torch.int64
torch.exp(t)
# RuntimeError
torch.exp(t.float())
# tensor([1.0000, 2.7183, 0.1353, 7.3891, 1.0000])
其他常用科学计算有:
数学运算函数 | 数学公式 | 功能 |
---|---|---|
幂运算 | ||
torch.exp(t) | y_{i} = e\^{x_{i}} | 返回以e为底、t中元素为幂的张量 |
torch.pow(t,n) | \\text{out}_i = t \^ \\text{n} | 返回t的n次幂 |
torch.sqrt(t) | \\text{out} = \\sqrt{\\text{input}} | 返回t的平方根 |
torch.square(t) | \\text{out}_i = x_i \^ \\text{2} | 返回输入的元素平方 |
对数运算 | ||
torch.log10(t) | y_{i} = \\log_{10} (x_{i}) | 返回以10为底的t的对数 |
torch.log(t) | y_{i} = \\log_{e} (x_{i}) | 返回以e为底的t的对数 |
torch.log2(t) | y_{i} = \\log_{2} (x_{i}) | 返回以2为底的t的对数 |
torch.log1p(t) | y_i = \\log_{e} (x_i + 1) | 返回一个加自然对数的输入数组。 |
三角运算 | ||
torch.sin(t) | 正弦 | |
torch.cos(t) | 余弦 | |
torch.tan(t) | 正切 |
4 统计分析
此类计算是对某张量进行某种总结,最后得出一个具体总结值的函数。
函数 | 功能 |
---|---|
torch.mean(t) | 返回张量均值 |
torch.var(t) | 返回张量方差 |
torch.std(t) | 返回张量标准差 |
torch.var_mean(t) | 返回张量方差和均值 |
torch.std_mean(t) | 返回张量标准差和均值 |
torch.max(t) | 返回张量最大值 |
torch.argmax(t) | 返回张量最大值索引 |
torch.min(t) | 返回张量最小值 |
torch.argmin(t) | 返回张量最小值索引 |
torch.median(t) | 返回张量中位数 |
torch.sum(t) | 返回张量求和结果 |
torch.logsumexp(t) | 返回张量各元素求和结果,适用于数据量较小的情况 |
torch.prod(t) | 返回张量累乘结果 |
torch.dist(t1, t2) | 计算两个张量的闵式距离,可使用不同范式 |
torch.topk(t) | 返回t中最大的k个值对应的指标 |
- 这里我们常用的距离公式
dist()
,为闵可夫斯基距离,通过输入不同的p值,可方便计算曼哈顿距离、欧拉距离:
Python
# 输入float型
t1 = torch.tensor([1, 2, 3]).float()
t2 = torch.tensor([4, 5, 6]).float()
# 计算曼哈顿距离
torch.dist(t1, t2, 1)
# tensor(9.)
# 计算欧拉距离
torch.dist(t1, t2, 2)
# tensor(5.1962)
- 统计分析是一个张量序列返回一个结果,因此若是针对高维张量,则可指定某维度进行计算:
Python
# 创建一个3*3的二维张量
t2 = torch.arange(6).float().reshape(2, 3)
t2
# 按照第一个维度求和(按列求和)
torch.sum(t2, dim = 0)
# tensor([3., 5., 7.])
理解为:按照第一个维度是行,所以将每行对应元素相加,就是按列求和。
这里一定要将dim参数和shape返回结果一一对应。不理解的同学可看。
Python
# 创建一个2*3*4的三维张量
t3 = torch.arange(24).float().reshape(2, 3, 4)
t3
# tensor([[[ 0., 1., 2., 3.],
# [ 4., 5., 6., 7.],
# [ 8., 9., 10., 11.]],
# [[12., 13., 14., 15.],
# [16., 17., 18., 19.],
# [20., 21., 22., 23.]]])
# 第一维度是代表几个二维矩阵,就是二维矩阵的对应位置相加
torch.sum(t3, dim = 0)
# tensor([[12., 14., 16., 18.],
# [20., 22., 24., 26.],
# [28., 30., 32., 34.]])
# 第二个维度代表行,就是向量,每个二维矩阵中的向量对应位置相加
torch.sum(t3, dim = 1)
# tensor([[12., 15., 18., 21.],
# [48., 51., 54., 57.]])
# 第三个维度是列,就是零维张量,就是每个向量进行相加
torch.sum(t3, dim = 2)
# tensor([[ 6., 22., 38.],
# [54., 70., 86.]])
这样理解维度是不是清晰明了?
5 比较运算
常用于不同张量之间的逻辑运算,最终返回布尔值。需要注意的是eq()
与equal()
的区别。
Python
t1 = torch.tensor([1.0, 2, 4])
t2 = torch.tensor([1.0, 2, 5])
比较各元素是否相等
Python
torch.eq(t1, t2)
# tensor([ True, True, False])
# 等效t1 == t2
t1 == t2
# tensor([ True, True, False])
判断是否是相同的张量
Python
torch.equal(t1, t2)
# False
其它比较计算还有:
函数 | 功能 |
---|---|
torch.gt(t1, t2) | 比较t1各元素是否大于t2各元素,等效> |
torch.lt(t1, t2) | 比较t1各元素是否小于t2各元素,等效< |
torch.ge(t1, t2) | 比较t1各元素是否大于或等于t2各元素,等效>= |
torch.le(t1, t2) | 比较t1各元素是否小于等于t2各元素,等效<= |
torch.ne(t1, t2) | 比较t1、t2各元素是否不相同,等效!= |
Pytorch张量操作大全:
Pytorch使用教学1-Tensor的创建
Pytorch使用教学2-Tensor的维度
Pytorch使用教学3-特殊张量的创建与类型转化
Pytorch使用教学4-张量的索引
Pytorch使用教学5-视图view与reshape的区别
Pytorch使用教学6-张量的分割与合并
Pytorch使用教学7-张量的广播
Pytorch使用教学8-张量的科学运算
Pytorch使用教学9-张量的线性代数运算
Pytorch使用教学10-张量操作方法大总结
有关Pytorch建模相关的AI干货请扫码关注公众号「AI有温度」阅读获取