Prediction of the Dst Index with Bagging Ensemble-learning Algorithm
The Astrophysical Journal Supplement Series, 248:14 (11pp), 2020 May
Prediction of the Dst Index with Bagging Ensemble-learning Algorithm (iop.org)
Abstract
The Dst index is a commonly geomagnetic index used to measure the strength of geomagnetic activity. The accurate prediction of the Dst index is one of the main subjects of space weather studies. In this study, we use the Bagging ensemble-learning algorithm, which combines three algorithms---the artificial neural network, support vector regression, and long short-term memory network---to predict the Dst index 1--6 hr in advance. Taking solar wind parameters (including the interplanetary total magnetic field, magnetic field Bz component, total electric field, solar wind speed, plasma temperature, and proton pressure) as inputs, we establish the Dst index models and complete not only the point prediction but also the interval prediction in forecasting the Dst index. The results show that the root mean square error (rmse) of the point prediction is always lower than 8.0936 nT, the correlation coefficient (R) is always higher than 0.8572 and the accuracy of interval prediction is always higher than 90%, implying that our model can improve the accuracy of point prediction and significantly promote the accuracy of interval prediction. In addition, an new proposed metric shows that the Bagging algorithm brings better stability to the model. Our model was also used to predicate a magnetic storm event from 2016 October 12--17. The most accurate prediction of this storm event is the 1 hr ahead prediction, which holds a result with the rmse of 3.7327 nT, the correlation coefficient of 0.9928, and the interval prediction accuracy of 96.69%. Moreover, we also discuss the balance in the Bagging ensemble model in this paper.
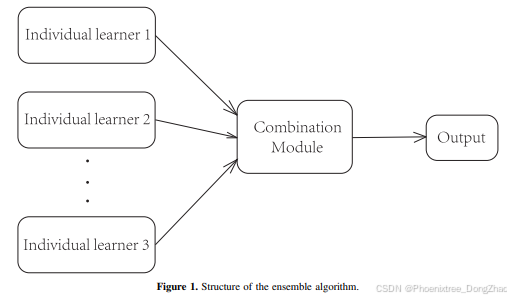
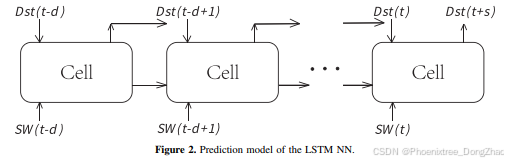
Selection of the Main Control Parameters for the Dst Index Prediction Model Based on a Layer-wise Relevance Propagation Method
The Astrophysical Journal Supplement Series, 260:6 (8pp), 2022 May
Abstract
The prediction of the Dst index is an important subject in space weather. It has significant progress with the prevalent applications of neural networks. The selection of input parameters is critical for the prediction model of the Dst index or other space-weather models. In this study, we perform a layer-wise relevance propagation (LRP) method to select the main parameters for the prediction of the Dst index and understand the physical interpretability of neural networks for the first time. Taking an hourly Dst index and 10 types of solar wind parameters as the inputs, we utilize a long short-term memory network to predict the Dst index and present the LRP method to analyze the dependence of the Dst index on these parameters. LRP defines the relevance score for each input, and a higher relevance score indicates that the corresponding input parameter contributes more to the output. The results show that Dst, Ey, Bz, and V are the main control parameters for Dst index prediction. In order to verify the LRP method, we design two more supplementary experiments for further confirmation. These results confirm that the LRP method can reduce the initial dimension of neural network input at the cost of minimum information loss and contribute to the understanding of physical processes in space weather.
Multi-Hour-Ahead Dst Index Prediction Using Multi-Fidelity Boosted Neural Networks
Space Weather, 30 March 2023 Volume21, Issue4
Abstract
The Disturbance storm time (Dst) index has been widely used as a proxy for the ring current intensity, and therefore as a measure of geomagnetic activity. It is derived by measurements from four ground magnetometers in the geomagnetic equatorial region. We present a new model for predicting Dst with a lead time between 1 and 6 hr. The model is first developed using a Gated Recurrent Unit (GRU) network that is trained using solar wind parameters. The uncertainty of the Dst model is then estimated by using the Accurate and Reliable Uncertainty Estimate method (Camporeale & Carè, 2021, ACCRUE: ACCURATE AND RELIABLE UNCERTAINTY ESTIMATE IN DETERMINISTIC MODELS - International Journal for Uncertainty Quantification, Volume 11, 2021, Issue 4 - Begell House Digital Library). Finally, a multi-fidelity boosting method is developed in order to enhance the accuracy of the model and reduce its associated uncertainty. It is shown that the developed model can predict Dst 6 hr ahead with a root-mean-square-error of 13.54 nT. This is significantly better than a persistence model or a single GRU model.
MagNet---A Data-Science Competition to Predict Disturbance Storm-Time Index (Dst) From Solar Wind Data
Space Weather, 05 October 2023 Volume21, Issue10
Abstract
Enhanced interaction between solar-wind and Earth's magnetosphere can cause space weather and geomagnetic storms that have the potential to damage critical technologies, such as magnetic navigation, radio communications, and power grids. The severity of a geomagnetic storm is measured using the disturbance-storm-time (Dst ) index. The Dst index is calculated by averaging the horizontal component of the magnetic field observed at four near-equatorial observatories and is used to drive geomagnetic disturbance models. As a key specification of the magnetospheric dynamics, the Dst index is used to drive geomagnetic disturbance models such as the High Definition Geomagnetic Model---Real Time. Since 1975, forecasting models have been proposed to forecast Dst solely from solar wind observations at the Lagrangian-1 position. However, while the recent Machine-Learning (ML) models generally perform better than other approaches, many are unsuitable for operational use. Recent exponential growth in data-science research and the democratization of ML tools have opened up the possibility of crowd-sourcing specific problem-solving tasks with clear constraints and evaluation metrics. To this end, National Oceanic and Atmospheric Administration (NOAA)'s National Centers for Environmental Information and the University of Colorado's Cooperative Institute for Research in Environmental Sciences conducted an open data-science challenge called "MagNet: Model the Geomagnetic Field." The challenge attracted 622 participants, resulting in 1,197 model submissions that used various ML approaches. The top models that met the evaluation criteria are operationally viable and retrainable and suitable for NOAA's operational needs. The paper summarizes the competition results and lessons learned.
Forecasting the Dst Index with Temporal Convolutional Network and Integrated Gradients
Sol Phys 299, 98 (2024)
Abstract
The Disturbance Storm Time (Dst) Index stands as a crucial geomagnetic metric, serving to quantify the intensity of geomagnetic disturbances. The accurate prediction of the Dst index plays a pivotal role in mitigating the detrimental effects caused by severe space-weather events. Therefore, Dst prediction has been a long-standing focal point within the realms of space physics and space-weather forecasting. In this study, a Temporal Convolutional Network (TCN) is deployed in tandem with the Integrated Gradient (IG) algorithm to predict the Dst index and scrutinize its associated physical processes. With these two components, our model can give the contribution of each input parameter to the outcome along with the forecast. The TCN component of our model utilizes interplanetary observational data, encompassing the vector magnetic field, solar-wind velocity, proton temperature, proton density, interplanetary electric field, and other relevant parameters for forecasting Dst indices. Despite the disparity in test sets, our model's forecast accuracy approximates the error levels of the prior models. Remarkably, the prediction error of these machine-learning models has become comparable to the inherent error between the Dst index itself and the actual ring-current strength.
To understand the physical process behind the forecasting model, the IG algorithm was applied in our prediction model, in an attempt to analyze the underlying physical process of the machine-learning black box. In the temporal dimension, it is evident that the more recent the time, the more substantial the influence on the final prediction. Regarding the physical parameters, besides the historical Dst index itself, the flow pressure, the z-component of the magnetic field, and the proton density all significantly contribute to the final prediction. Additionally, IG attributions were analyzed for subsets of data, including different Dst-index ranges, different observation times, and different interplanetary structures. Most of the subsets exhibit an IG matrix with deviations from the mean distribution, which indicates a complex nonlinear system and sensitivity of the prediction to input values. These analyses align with physical reasoning and are in good agreement with previous research. The results affirm that the TCN+IG technique not only enhances space-weather forecast accuracy but also advances our comprehension of the underlying physical processes in space weather.
Figure 2
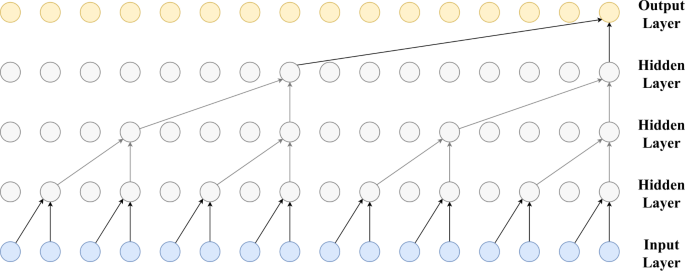
An example TCN structure with dilation factor list
Figure 3
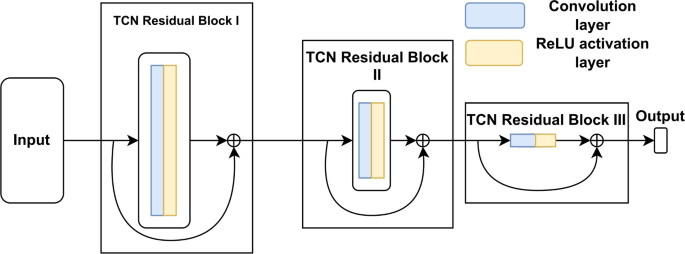
The TCN network structure used for Dst-index prediction in this paper.
Prediction of the Dst Geomagnetic Index Using Adaptive Methods
Russ. Meteorol. Hydrol. 46, 157--162 (2021)
Abstract
The potential is investigated of predicting the time series of the Dst geomagnetic index using various adaptive methods: artificial neural networks (classical multilayer perceptrons), decision trees (random forest), gradient boosting. The prediction is based on the parameters of the solar wind and interplanetary magnetic field measured at the Lagrange point L1 in the ACE spacecraft experiment. It is shown that the best prediction skill of the three adaptive methods is demonstrated by gradient boosting.
One-hour ahead prediction of the Dst index based on the optimum state space reconstruction and pattern recognition
Eur. Phys. J. Plus 137, 479 (2022)
Abstract
The Disturbance storm time (Dst) index is the global estimation of the intensity of the terrestrial geomagnetic activities as well as the primary indicator of the geomagnetic storm. As the adverse effect of a powerful geomagnetic event has become a serious threat to modern human society, extracting meaningful information concealed in the complex structures of this time series can form the ground of a successful prediction algorithm. In this paper, we introduce a new probabilistic model based on the concept of adaptive delta modulation and optimum state space to analyze, identify and characterize the patterns enfolded in the layers of the 1-h Dst index and then predict Dst data using these patterns. The study reveals some significant insights. The exact dimension M of the optimum state space for the Dst index is found to be at M = 10. Also, the series is a combination of multiple distinguished repeating and non-repeating patterns, denoting a high degree of predictability of the M th data of the series from its previous (M-1) binary data. Eventually, the simulated output of the probabilistic model exhibits a high value of correlation coefficient with the real-time Dst index. Interestingly, the technique only requires the Dst index data as an input of the probabilistic model and is found to be very effective for the entire 24th solar cycle.
Domain Adaptation of Spacecraft Data in Neural Network Prediction of Geomagnetic Dst Index
NEUROINFORMATICS 2023. Studies in Computational Intelligence, vol 1120. Springer, Cham.
Abstract
This study focuses on improving the neural network prediction of the geomagnetic indexes, in particular Dst-index, in a scenario, where input data is collected by two spacecraft (SC) with different data availability. One of the SC is approaching the end of its operational lifespan, while the other one lacks sufficient data history for constructing a high-quality neural network prediction. To effectively perform the transition between the two SC data, domain adaptation methods are needed. The study evaluates and compares various data translation techniques and optimizes the parameters for each translated feature to minimize domain discrepancies. The findings highlight the enhancement in the forecast, when employing domain adaptation methods and selecting relevant features, surpassing the results obtained using untranslated data.