首个糖尿病诊疗多模态大模型DeepDR-LLM已成功发表在Nature子刊。
这是全球首个面向糖尿病诊疗的视觉-大语言模型集成系统,结合了语言模型和基于眼底图像的深度学习技术。该系统旨在为基层医生提供个性化的糖尿病管理建议和辅助诊断结果,特别对中低收入国家具有重要意义。通过引入这一革命性的数字解决方案,希望显著改善基层医生处理糖尿患者和筛查视网膜病变水平。
此项工作由51家机构共同完成,包括上海交通大学医学院、清华大学医学院等单位。
Abstract
Primary diabetes care and diabetic retinopathy (DR) screening persist as major public health challenges due to a shortage of trained primary care physicians (PCPs), particularly in low-resource settings. Here, to bridge the gaps, we developed an integrated image--language system (DeepDR-LLM), combining a large language model (LLM module) and image-based deep learning (DeepDR-Transformer), to provide individualized diabetes management recommendations to PCPs. In a retrospective evaluation, the LLM module demonstrated comparable performance to PCPs and endocrinology residents when tested in English and outperformed PCPs and had comparable performance to endocrinology residents in Chinese. For identifying referable DR, the average PCP's accuracy was 81.0% unassisted and 92.3% assisted by DeepDR-Transformer. Furthermore, we performed a single-center real-world prospective study, deploying DeepDR-LLM. We compared diabetes management adherence of patients under the unassisted PCP arm (n = 397) with those under the PCP+DeepDR-LLM arm (n = 372). Patients with newly diagnosed diabetes in the PCP+DeepDR-LLM arm showed better self-management behaviors throughout follow-up (P < 0.05). For patients with referral DR, those in the PCP+DeepDR-LLM arm were more likely to adhere to DR referrals (P < 0.01). Additionally, DeepDR-LLM deployment improved the quality and empathy level of management recommendations. Given its multifaceted performance, DeepDR-LLM holds promise as a digital solution for enhancing primary diabetes care and DR screening.
摘要:
目前,在资源匮乏地区缺乏训练有素的初级保健医生(PCP)仍然是一个主要公共卫生挑战,特别是在初级护理和视网膜筛查领域。为填补这一差距,本文开发了一个名为DeepDR-LLM的集成图像语言系统。该系统结合了大型语言模型(LLM模块)和基于图像的深度学习(DeepDR-Transformer),旨在为PCP提供个性化的糖尿管理建议。回顾性评估显示,在英文测试中,LLM模块与PCP和内分泌科住院医师表现相当;而在中文测试中优于PCP并与内分泌科住院医师相当。对于可转诊至专科进行进一步检查治理需求的DR患者,在无辅助时PCP平均准确率达到81.0%,而使用DeepDR-Transformer辅助后准确率达到92.3%。此外,我们还进行了一项单中心真实世界前景性调查,部署DeepDR-LLM,并比较无辅助组(n=397)与PCP+DeepDR-LLM组(n=372)患者关于遵循治理咨询情况发现:PCP+DeepDR-LLM组新被诊断出患有DN(DiabeticNephropathy)耐药时整个随访过程更好地展示出自我管控行为(P<0.05)。对需要转介至专业人员进一步检测治理需求的DR患者,使用DeepDR-Transformer辅助后更容易接受转介(P<0.01)。此外,部署DeepDR-LLM还提高了咨询质量和同理心水平。鉴于其多方面出色表现,DeepDR-LLM很可能成为增强初级护理和DR筛查数字解决方案。
Main
It has been estimated that more than 500 million people had diabetes worldwide in 2021, with 80% living in low- and middle-income countries (LMICs)1,2. The escalating prevalence imposes a substantial public health challenge, particularly in these low-resource settings1,3,4,5. In LMICs, insufficient healthcare resource and a lack of trained primary care physicians (PCPs) remain principal barriers, resulting in widespread underdiagnosis, poor primary diabetes management and inadequate and/or inappropriate referrals to diabetes specialist care4,6,7. This not only impacts on individual health outcomes but also has broader socioeconomic consequences4,8,9,10.
据估计,2021年全球有超过5亿人罹患糖尿病,其中80%居住在收入较低和中等的国家(LMICs)[1,2]。这种不断上升的发病率对公共卫生构成了巨大挑战,特别是在资源匮乏地区[1,3,4,5]。在LMICs中,医疗资源紧缺和缺乏受过培训的初级保健医师(PCPs)仍然是主要障碍,导致未诊断情况普遍存在、初级糖尿病管理不善以及对专科护理的转诊不足或不合适[4,6,7]。这不仅对个体健康结果产生影响,还会造成更广泛的社会经济后果[4,8,9,10]。
Diabetic retinopathy (DR) is the most common specific complication of diabetes, affecting 30--40% of individuals with diabetes11,12,13, and remains the leading cause of blindness in economically active, working-aged adults11,14,15. The presence of DR also signifies a heightened risk of other complications elsewhere (for example, kidney, heart and brain)16. Thus, regular DR screening has been universally recommended as a key part of primary diabetes care17. However, DR screening is often neglected in low-resource settings in LMICs owing to a scarcity of infrastructure, manpower and sustainable cost-effective DR screening programs.
糖尿病视网膜病变(DR)是最常见的糖尿病特定并发症,影响30-40%的患有糖尿病的人群11,12,13,并且仍然是经济活跃、工作年龄成人失明的主要原因11,14,15。DR存在还意味着其他部位(例如肾脏、心脏和大脑)并发症风险增加16。因此,定期进行DR筛查已被普遍推荐为初级糖尿病护理的关键部分17。然而,在低资源环境下,由于基础设施、人力资源和可持续性具有成本效益的DR筛查计划匮乏,往往忽视了DR筛查在低收入国家中不足之处。
Several digital technologies have emerged to address gaps in diabetes care and DR screening, including telemedicine18,19,20, artificial intelligence (AI)-assisted glucose monitoring and prediction21, retinal image-based deep learning (DL) models22,23,24 and the development of low-cost and portable retinal cameras25,26. However, these solutions often focus either on enhancing diabetes management or on providing DR screening but rarely integrate both important aspects for diabetes care. These current solutions also require sufficiently trained PCPs capable of utilizing these digital tools, understanding diabetes care, and referral guidelines for severe DR cases that require specialists interventions, but there are few trained PCPs in low-resource settings27.
近年来,出现了几种数字技术用于解决糖尿病护理和视网膜筛查的问题。这些技术包括远程医疗、人工智能辅助血糖监测和预测、基于视网膜图像的深度学习模型以及低成本便携式视网膜相机的开发。然而,目前存在一个问题,即这些解决方案要么专注于改善糖尿病管理,要么专注于提供视网膜筛查,并很少将两个重要方面结合起来进行综合处理。此外,在资源有限的环境中受过培训的初级保健医生(PCPs)数量较少,因此他们需要接受足够培训才能使用这些数字工具,并且还需要了解关于糖尿病护理和对重度DR(需专家干预)情况下转诊指南等相关知识。
Recently, large language models (LLMs)28,29,30,31, achieving natural language understanding and generation, have been developing rapidly and show promise in enhancing healthcare service delivery. LLMs have the potential to optimize patient monitoring, personalization of treatment plans, and patient education, potentially resulting in improved outcomes for patients with diabetes32,33,34 and retinal diseases35,36. However, while they perform well in answering some general medical queries31,37, current LLMs fall short in providing reliable and detailed management recommendations for major specific diseases31,38,39, such as diabetes.
近期,大型语言模型(LLMs)在自然语言理解和生成方面取得了迅猛发展,并显示出增强医疗服务交付的潜力。LLMs有望优化患者监测、个性化治疗计划和患者教育,从而可能改善糖尿病和视网膜疾病等患者的预后结果。然而,尽管它们在回答一些常见医学问题上表现良好,但目前的LLMs在提供针对特定主要疾病(如糖尿病)可靠且详细的管理建议方面还存在不足之处。
To address these interrelated gaps in diabetes care, we developed an innovative image--language system---DeepDR-LLM---which integrates an LLM module with an image-based DL module to offer a comprehensive approach for primary diabetes care and DR screening. Our system is tailored for PCPs, particularly those working in high-volume and low-resource settings. The DeepDR-LLM system comprises two core components: an LLM module and an image-based DL module, referred to as DeepDR-Transformer (Fig. 1). Our evaluation of DeepDR-LLM's performance relied on four experiments outlined in Fig. 2a--d. First, we developed the LLM module by fine-tuning LLaMA38, an open-source LLM that used 371,763 real-world management recommendations from 267,730 participants. We then performed a head-to-head comparative analysis, where we examined the system's LLM module's proficiency in providing evidence-based diabetes management recommendations against that of LLaMA, PCPs and in-training specialists (endocrinology residents), with assessments conducted in both English and Chinese languages (Fig. 2a). Second, we trained and tested the performance of DeepDR-Transformer for referable DR detection, using multiethnic, multicountry datasets comprising 1,085,295 standard (table-top) and 161,840 portable (mobile) retinal images (Fig. 2b). Third, we evaluated the impact of DeepDR-Transformer in assisting PCPs and professional graders to identify referable DR (Fig. 2c). Finally, we conducted a two-arm, real-world prospective study to determine the impact of DeepDR-LLM system when integrated into clinical workflow in the primary care setting. Over a 4-week period, we monitored and compared the adherence to diabetes management recommendations between patients under the care of unassisted PCPs and those under the care of PCPs assisted by DeepDR-LLM (Fig. 2d). Collectively, our work offers a digital solution for primary diabetes care combining DR screening and referral, particularly useful in high-volume, low-resource settings in LMICs.
为了解决糖尿病护理中存在的差距,我们开发了一种创新的系统DeepDR-LLM,它将一个LLM模块和一个基于图像的DL模块结合在一起,提供全面的初级糖尿病护理和DR筛查方法。我们的系统专门针对PCPs(主要保健医生),特别是那些在负荷高、资源有限环境下工作的医生。DeepDR-LLM系统包括两个核心组件:一个LLM模块和一个基于图像的DL模块,称为DeepDR-Transformer。我们通过使用真实世界管理建议数据集来开发LLM模块,并进行了性能评估。然后,在英文和中文语言下进行了系统性能比较分析,并评估其与其他方法之间的差异。接着,我们使用多民族、多国家数据集进行了深度学习转换器(DeepDR-Transformer)用于可引用视网膜底层检测(DR detection) 的训练和测试,并使用大量标准台式机(retinal images) 和便携式移动设备(retinal images) 进行测试。第三,在协助PCPs和专业分级人员识别可引用视网膜底层时评估 Deep DR 转换器影响。最后,在初级保健设置中将 Deep DR LLM 系统整合到临床工作流程中进行了现实前景研究,以确定 Deep DR LLM 系统对未经协助 PCP 和经过协助 PCP 治疗患者遵循 diabetes management recommendations 的影响。总体而言,我们提出了一种数字化解决方案结合 DR 筛查和转介(primary diabetes care),特别适用于负荷高、资源有限情况下的应用场景
Fig. 1: Architecture of the DeepDR-LLM system.
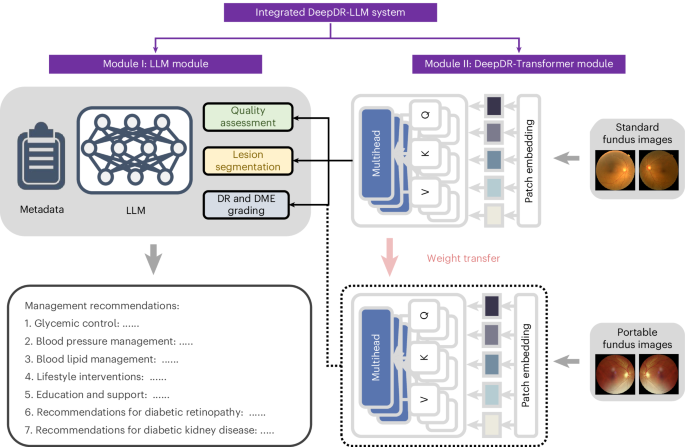
The DeepDR-LLM system consists of two modules: (1) module I (LLM module), which provides individualized management recommendations for patients with diabetes; (2) module II (DeepDR-Transformer module), which performs image quality assessment, DR lesion segmentation and DR/DME grading from standard or portable fundus images. There are two modes of integrating module I and module II in the DeepDR-LLM system. In the physician-involved integration mode, the outputs of module II (that is, fundus image gradability; the lesion segmentation of microaneurysm, cotton-wool spot, hard exudate and hemorrhage; DR grade; and DME grade) could assist physicians in generating DR/DME diagnosis results (that is, fundus image gradability, DR grade, DME grade and the presence of lesions). In the automated integration mode, the DR/DME diagnosis results include fundus image gradability, DR grade, DME grade classified by module II, and the presence of lesions segmented out by module II. These DR/DME diagnosis results and other clinical metadata will be fed into module I to generate individualized management recommendations for people with diabetes.
Fig. 2: Study design overview for the DeepDR-LLM system evaluation.
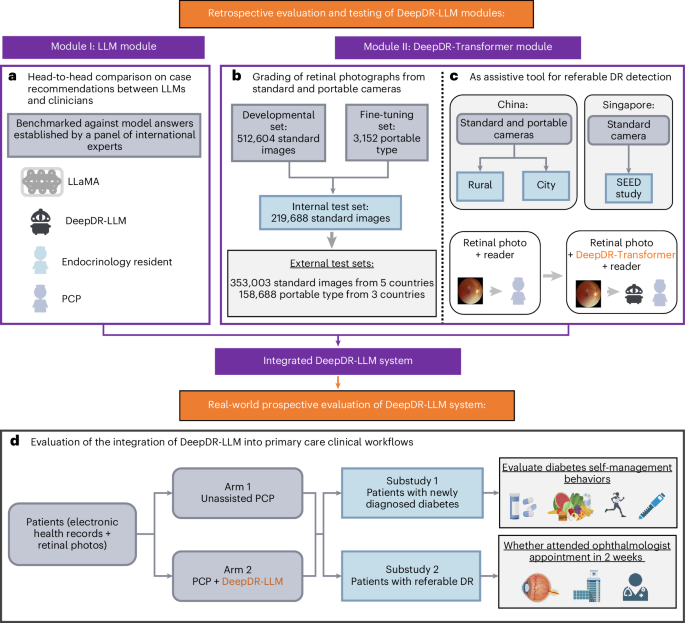
a , Head-to-head comparative assessment of diabetes management recommendations generated by DeepDR-LLM, nontuned LLaMA, PCPs and endocrinology residents, using 100 cases randomly selected from CNDCS. b , Efficacy analysis of the DeepDR-Transformer module on multiethnic datasets of standard and portable fundus images. c , Utility evaluation of the DeepDR-Transformer module as an assistive tool for PCPs and professional graders in the detection of referable DR. d, Study design of a two-arm, real-world, prospective study to evaluate the impact of DeepDR-LLM on patients' self-management behavior. In the outcome analysis, for substudy I, 253 participants in the unassisted PCP arm and 234 participants in the PCP+DeepDR-LLM arm were included; for substudy II, 154 participants in the unassisted PCP arm and 144 participants in the PCP+DeepDR-LLM arm were included.
Fig. 6: Envisioning the future of primary diabetes care with the clinical integration of the DeepDR-LLM system.
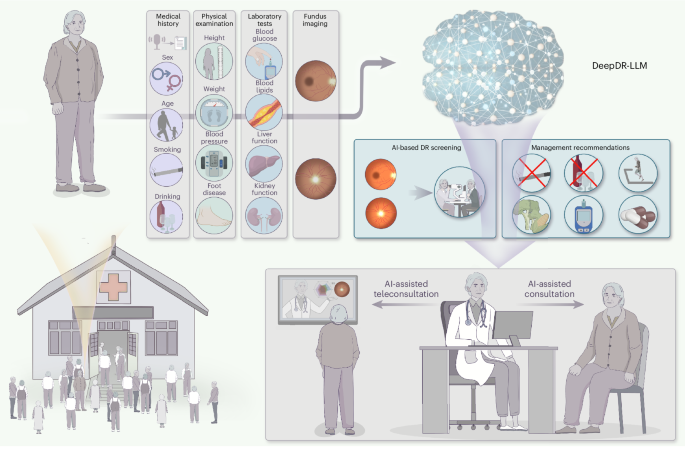
First, patients with diabetes undergo comprehensive evaluations that include medical history taking that can be augmented by automated voice-to-text technology, physical examinations, laboratory assessments and fundus imaging. Following this, the DeepDR-LLM system processes the accumulated clinical data to concurrently deliver DR screening results and tailored management recommendations for PCPs. Subsequently, augmented with these AI-derived insights, PCPs then offer treatment guidance and health education to patients, either in person or through teleconsultation services.