目录

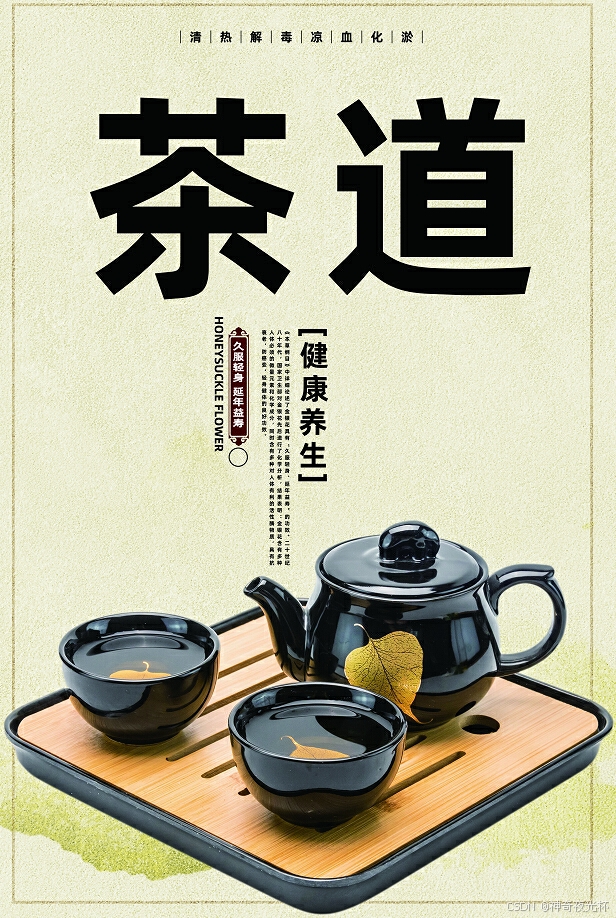

一、用法精讲
426、pandas.DataFrame.at属性
426-1、语法
python
# 426、pandas.DataFrame.at属性
pandas.DataFrame.at
Access a single value for a row/column label pair.
Similar to loc, in that both provide label-based lookups. Use at if you only need to get or set a single value in a DataFrame or Series.
Raises:
KeyError
If getting a value and 'label' does not exist in a DataFrame or Series.
ValueError
If row/column label pair is not a tuple or if any label from the pair is not a scalar for DataFrame. If label is list-like (excluding NamedTuple) for Series.
426-2、参数
无
426-3、功能
用于基于行标签和列标签快速访问或设置单个元素的值,它具备高效性,因此非常适合在需要处理单个元素时使用。
426-4、返回值
返回指定行和列位置的单个元素的值或直接修改DataFrame中指定位置的值,并不返回任何内容。
426-5、说明
无
426-6、用法
426-6-1、数据准备
python
无
426-6-2、代码示例
python
# 426、pandas.DataFrame.at属性
import pandas as pd
data = {
'A': [1, 2, 3],
'B': [4, 5, 6],
'C': [7, 8, 9]
}
df = pd.DataFrame(data)
# 访问单个值
value = df.at[1, 'B']
print(value)
# 设置单个值
df.at[1, 'B'] = 10
print(df)
426-6-3、结果输出
python
# 426、pandas.DataFrame.at属性
# 5
# A B C
# 0 1 4 7
# 1 2 10 8
# 2 3 6 9
427、pandas.DataFrame.iat属性
427-1、语法
python
# 427、pandas.DataFrame.iat属性
pandas.DataFrame.iat
Access a single value for a row/column pair by integer position.
Similar to iloc, in that both provide integer-based lookups. Use iat if you only need to get or set a single value in a DataFrame or Series.
Raises:
IndexError
When integer position is out of bounds.
427-2、参数
无
427-3、功能
用于基于整数位置(即行索引和列索引)快速访问或设置单个元素的值,与基于标签的.at
不同,.iat
采用的是位置索引,因此更加类似于NumPy的访问方式。
427-4、返回值
返回指定行索引和列索引位置的单个元素的值或直接修改DataFrame中指定位置的值,并不返回任何内容。
427-5、说明
无
427-6、用法
427-6-1、数据准备
python
无
427-6-2、代码示例
python
# 427、pandas.DataFrame.iat属性
import pandas as pd
data = {
'A': [1, 2, 3],
'B': [4, 5, 6],
'C': [7, 8, 9]
}
df = pd.DataFrame(data)
# 访问单个值
value = df.iat[1, 1]
print(value)
# 设置单个值
df.iat[1, 1] = 10
print(df)
427-6-3、结果输出
python
# 427、pandas.DataFrame.iat属性
# 5
# A B C
# 0 1 4 7
# 1 2 10 8
# 2 3 6 9
428、pandas.DataFrame.loc属性
428-1、语法
python
# 428、pandas.DataFrame.loc属性
pandas.DataFrame.loc
Access a group of rows and columns by label(s) or a boolean array.
.loc[] is primarily label based, but may also be used with a boolean array.
Allowed inputs are:
A single label, e.g. 5 or 'a', (note that 5 is interpreted as a label of the index, and never as an integer position along the index).
A list or array of labels, e.g. ['a', 'b', 'c'].
A slice object with labels, e.g. 'a':'f'.
Warning
Note that contrary to usual python slices, both the start and the stop are included
A boolean array of the same length as the axis being sliced, e.g. [True, False, True].
An alignable boolean Series. The index of the key will be aligned before masking.
An alignable Index. The Index of the returned selection will be the input.
A callable function with one argument (the calling Series or DataFrame) and that returns valid output for indexing (one of the above)
See more at Selection by Label.
Raises:
KeyError
If any items are not found.
IndexingError
If an indexed key is passed and its index is unalignable to the frame index.
428-2、参数
无
428-3、功能
428-3-1、单个元素访问和修改: 通过指定行标签和列标签来访问或修改DataFrame
中的单个元素。
**428-3-2、行访问和修改:**通过行标签来访问或修改整行数据。
**428-3-3、列访问和修改:**通过列标签来访问或修改整列数据。
**428-3-4、多行或多列访问和修改:**通过传递行标签或列标签的列表来访问或修改多个行或列的数据。
**428-3-5、条件筛选:**通过布尔条件筛选出满足条件的行或列。
428-3-6、子集访问和修改: 访问和修改DataFrame
的特定子集(基于行标签和列标签)。
428-4、返回值
**428-4-1、单个元素访问和修改:**返回指定的元素值(可以是任何类型,如整数、字符串、浮点数等)。
428-4-2、行访问和修改: 返回一个包含该行所有列数据的Series
对象,索引为列标签。
428-4-3、列访问和修改: 返回一个包含该列所有行数据的Series
对象,索引为行标签。
428-4-4、多行或多列访问和修改: 返回一个包含所选行或列数据的DataFrame
对象。
428-4-5、条件筛选: 返回一个DataFrame
对象,包含所有满足条件的行或列。
428-4-6、子集访问和修改: 返回一个包含子集数据的DataFrame
对象。
428-5、说明
无
428-6、用法
428-6-1、数据准备
python
无
428-6-2、代码示例
python
# 428、pandas.DataFrame.loc属性
# 428-1、数据筛选
import pandas as pd
# 创建一个示例DataFrame
data = {
'date': ['2024-01-01', '2024-01-02', '2024-01-03', '2024-01-04'],
'value': [10, 20, 30, 40]
}
df = pd.DataFrame(data)
df['date'] = pd.to_datetime(df['date'])
# 筛选出'value'大于20的行
filtered_df = df.loc[df['value'] > 20]
print(filtered_df, end='\n\n')
# 428-2、数据更新
import pandas as pd
# 创建一个示例DataFrame
data = {
'date': ['2024-01-01', '2024-01-02', '2024-01-03', '2024-01-04'],
'value': [10, 20, 30, 40]
}
df = pd.DataFrame(data)
df['date'] = pd.to_datetime(df['date'])
# 将'value'大于20的行的'value'列更新为100
df.loc[df['value'] > 20, 'value'] = 100
print(df, end='\n\n')
# 428-3、多列操作
import pandas as pd
# 创建一个示例DataFrame
data = {
'A': [1, 2, 3, 4],
'B': [5, 6, 7, 8],
'C': [9, 10, 11, 12]
}
df = pd.DataFrame(data)
# 选择'A'和'B'两列并计算它们的和
df['A_B_sum'] = df.loc[:, ['A', 'B']].sum(axis=1)
print(df, end='\n\n')
# 428-4、行操作
import pandas as pd
# 创建一个示例DataFrame
data = {
'A': [1, 2, 3, 4],
'B': [5, 6, 7, 8],
'C': [9, 10, 11, 12]
}
df = pd.DataFrame(data)
# 选择第1行和第3行并计算它们的总和
row_sum = df.loc[[0, 2], :].sum()
print(row_sum, end='\n\n')
# 428-5、多条件筛选
import pandas as pd
# 创建一个示例DataFrame
data = {
'A': [1, 2, 3, 4],
'B': [5, 6, 7, 8],
'C': [9, 10, 11, 12]
}
df = pd.DataFrame(data)
# 筛选'A'列大于2且'B'列小于8的行
filtered_df = df.loc[(df['A'] > 2) & (df['B'] < 8)]
print(filtered_df, end='\n\n')
# 428-6、数据子集提取
import pandas as pd
# 创建一个示例DataFrame
data = {
'A': [1, 2, 3, 4],
'B': [5, 6, 7, 8],
'C': [9, 10, 11, 12]
}
df = pd.DataFrame(data)
# 提取'A'列和'C'列,以及前两行构成的子集
subset_df = df.loc[:1, ['A', 'C']]
print(subset_df, end='\n\n')
# 428-7、数据分组与聚合
import pandas as pd
# 创建一个示例DataFrame
data = {
'category': ['A', 'A', 'B', 'B'],
'value': [10, 20, 30, 40]
}
df = pd.DataFrame(data)
# 按'category'分组并计算每组的总和
grouped_df = df.groupby('category').sum()
# 筛选出总和值大于30的组
filtered_grouped_df = grouped_df.loc[grouped_df['value'] > 30]
print(filtered_grouped_df, end='\n\n')
# 428-8、合并与连接
import pandas as pd
# 创建两个示例DataFrame
data1 = {
'key': [1, 2, 3],
'value1': [10, 20, 30]
}
data2 = {
'key': [2, 3, 4],
'value2': [40, 50, 60]
}
df1 = pd.DataFrame(data1)
df2 = pd.DataFrame(data2)
# 合并两个DataFrame
merged_df = pd.merge(df1, df2, on='key', how='outer')
# 筛选出'value1'和'value2'都不为NaN的行
filtered_merged_df = merged_df.loc[~merged_df['value1'].isna() & ~merged_df['value2'].isna()]
print(filtered_merged_df)
428-6-3、结果输出
python
# 428、pandas.DataFrame.loc属性
# 428-1、数据筛选
# date value
# 2 2024-01-03 30
# 3 2024-01-04 40
# 428-2、数据更新
# date value
# 0 2024-01-01 10
# 1 2024-01-02 20
# 2 2024-01-03 100
# 3 2024-01-04 100
# 428-3、多列操作
# A B C A_B_sum
# 0 1 5 9 6
# 1 2 6 10 8
# 2 3 7 11 10
# 3 4 8 12 12
# 428-4、行操作
# A 4
# B 12
# C 20
# dtype: int64
# 428-5、多条件筛选
# A B C
# 2 3 7 11
# 428-6、数据子集提取
# A C
# 0 1 9
# 1 2 10
# 428-7、数据分组与聚合
# value
# category
# B 70
# 428-8、合并与连接
# key value1 value2
# 1 2 20.0 40.0
# 2 3 30.0 50.0
429、pandas.DataFrame.iloc属性
429-1、语法
python
# 429、pandas.DataFrame.iloc属性
pandas.DataFrame.iloc
Purely integer-location based indexing for selection by position.
Deprecated since version 2.2.0: Returning a tuple from a callable is deprecated.
.iloc[] is primarily integer position based (from 0 to length-1 of the axis), but may also be used with a boolean array.
Allowed inputs are:
An integer, e.g. 5.
A list or array of integers, e.g. [4, 3, 0].
A slice object with ints, e.g. 1:7.
A boolean array.
A callable function with one argument (the calling Series or DataFrame) and that returns valid output for indexing (one of the above). This is useful in method chains, when you don't have a reference to the calling object, but would like to base your selection on some value.
A tuple of row and column indexes. The tuple elements consist of one of the above inputs, e.g. (0, 1).
.iloc will raise IndexError if a requested indexer is out-of-bounds, except slice indexers which allow out-of-bounds indexing (this conforms with python/numpy slice semantics).
See more at Selection by Position.
429-2、参数
无
429-3、功能
429-3-1、单行选择: 可以通过整数索引来选择DataFrame中的一行。
429-3-2、单列选择: 可以通过整数索引来选择DataFrame中的一列。
429-3-3、特定单元格选择: 可以通过行列的整数位置索引来选择DataFrame中的特定单元格。
429-3-4、区域选择(子集选择): 可以通过切片操作选择DataFrame的一个子集。
429-3-5、数据更新: 可以通过iloc
来更新DataFrame中某个位置的值。
429-4、返回值
429-4-1、单行选择: 返回一个Series
对象,索引为列标签。
429-4-2、单列选择: 返回一个Series
对象,索引为行标签。
**429-4-3、特定单元格选择:**返回具体单元格的值,数据类型视该单元格内容而定。
429-4-4、区域选择(子集选择): 返回一个新的DataFrame
对象。
429-5、说明
无
429-6、用法
429-6-1、数据准备
python
无
429-6-2、代码示例
python
# 429、pandas.DataFrame.iloc属性
# 429-1、数据清洗和预处理
import pandas as pd
# 创建一个示例DataFrame
data = {'A': [1, 2, None, 4],
'B': [5, 6, 7, 8],
'C': [9, None, 11, 12]}
df = pd.DataFrame(data)
# 选择前两行的数据
subset = df.iloc[:2, :]
print("选择前两行的数据:\n", subset)
# 填补某个单元格的缺失值
df.iloc[2, 0] = 3
print("\n填补缺失值后的DataFrame:\n", df)
# 429-2、数据分析和探索
import pandas as pd
# 创建一个示例DataFrame
data = {'A': [1, 2, None, 4],
'B': [5, 6, 7, 8],
'C': [9, None, 11, 12]}
df = pd.DataFrame(data)
# 分析第三和第四列的数据
subset = df.iloc[:, 2:]
print("第三和第四列的数据:\n", subset)
# 选择某一行进行特定分析
row = df.iloc[1]
print("\n第二行的数据:\n", row)
# 429-3、数据分区和批量处理
import pandas as pd
# 创建一个较大的DataFrame
data = {'A': range(10), 'B': range(10, 20)}
large_df = pd.DataFrame(data)
# 将数据分成两部分
batch_1 = large_df.iloc[:5, :]
batch_2 = large_df.iloc[5:, :]
# 处理每个批次的数据
print("第一批数据:\n", batch_1)
print("\n第二批数据:\n", batch_2)
# 429-4、模型训练和测试
from sklearn.model_selection import train_test_split
# 假设 large_df 是我们的完整数据集
X_train, X_test = train_test_split(large_df, test_size=0.2)
# 使用iloc进一步选择特定的行进行验证
X_validate = X_train.iloc[:2, :]
print("训练数据的前两行用于验证:\n", X_validate)
# 429-5、特定条件下的数据访问和更新
import pandas as pd
# 假设我们有一个时间序列数据
date_rng = pd.date_range(start='2024-01-01', end='2024-01-10', freq='D')
ts_data = pd.DataFrame(date_rng, columns=['date'])
ts_data['data'] = range(10)
ts_data.set_index('date', inplace=True)
# 使用iloc更新特定时间段的数据
ts_data.iloc[0:3, 0] = [20, 30, 40]
print("更新特定时间段后的时间序列数据:\n", ts_data)
429-6-3、结果输出
python
# 429、pandas.DataFrame.iloc属性
# 429-1、数据清洗和预处理
# 选择前两行的数据:
# A B C
# 0 1.0 5 9.0
# 1 2.0 6 NaN
#
# 填补缺失值后的DataFrame:
# A B C
# 0 1.0 5 9.0
# 1 2.0 6 NaN
# 2 3.0 7 11.0
# 3 4.0 8 12.0
# 429-2、数据分析和探索
# 第三和第四列的数据:
# C
# 0 9.0
# 1 NaN
# 2 11.0
# 3 12.0
#
# 第二行的数据:
# A 2.0
# B 6.0
# C NaN
# Name: 1, dtype: float64
# 429-3、数据分区和批量处理
# 第一批数据:
# A B
# 0 0 10
# 1 1 11
# 2 2 12
# 3 3 13
# 4 4 14
#
# 第二批数据:
# A B
# 5 5 15
# 6 6 16
# 7 7 17
# 8 8 18
# 9 9 19
# 429-4、模型训练和测试
# 训练数据的前两行用于验证:
# A B
# 7 7 17
# 9 9 19
# 429-5、特定条件下的数据访问和更新
# 更新特定时间段后的时间序列数据:
# data
# date
# 2024-01-01 20
# 2024-01-02 30
# 2024-01-03 40
# 2024-01-04 3
# 2024-01-05 4
# 2024-01-06 5
# 2024-01-07 6
# 2024-01-08 7
# 2024-01-09 8
# 2024-01-10 9
430、pandas.DataFrame.insert方法
430-1、语法
python
# 430、pandas.DataFrame.insert方法
pandas.DataFrame.insert(loc, column, value, allow_duplicates=_NoDefault.no_default)
Insert column into DataFrame at specified location.
Raises a ValueError if column is already contained in the DataFrame, unless allow_duplicates is set to True.
Parameters:
loc
int
Insertion index. Must verify 0 <= loc <= len(columns).
column
str, number, or hashable object
Label of the inserted column.
value
Scalar, Series, or array-like
Content of the inserted column.
allow_duplicates
bool, optional, default lib.no_default
Allow duplicate column labels to be created.
430-2、参数
430-2-1、loc**(必须)****:**整数,指定新列插入的位置,loc
是一个整数,表示新列将插入到的列索引位置。比如loc=0
表示将新列插入到最左边,loc=2
表示将新列插入到现有DataFrame的第三列位置(索引从0开始)。
430-2-2、column**(必须)****:**新列的名字,可以是字符串、整型等任何可哈希的值,这个名字将用作新列的列标签。
430-2-3、value**(必须)****:**标量、序列或数组,插入的数据,长度应与DataFrame的长度匹配。如果是标量值,则该值将广播到整列;如果是序列或数组,则其长度必须与DataFrame的行数一致。
430-2-4、allow_duplicates**(可选)****:**布尔值,是否允许插入重复的列标签,如果为False
而插入的列标签已经存在,则会引发一个ValueError
,默认设置是不允许重复的列标签。
430-3、功能
在现有DataFrame的指定位置插入一列新的数据,这在添加新特征(变量)或组合数据集时尤其有用。比如,你可以轻松地在DataFrame的特定位置添加一个新的计算列,或者在从文件或数据库加载数据后插入额外的信息。
430-4、返回值
该方法没有返回值,调用此方法会直接修改原有的DataFrame,因此要注意这一点。如果你希望保留原始DataFrame,可以考虑在插入新列之前先创建DataFrame的副本。
430-5、说明
无
430-6、用法
430-6-1、数据准备
python
无
430-6-2、代码示例
python
# 430、pandas.DataFrame.insert方法
import pandas as pd
# 创建一个示例DataFrame
data = {
'A': [1, 2, 3],
'B': [4, 5, 6]
}
df = pd.DataFrame(data)
print("原始DataFrame:")
print(df)
# 在位置1插入新列'C'
df.insert(1, 'C', [7, 8, 9])
print("\n插入新列后的DataFrame:")
print(df)
# 尝试插入重复的列标签
try:
df.insert(1, 'C', [10, 11, 12])
except ValueError as e:
print("\n尝试插入重复列标签时捕获的异常:")
print(e)
# 当设置allow_duplicates=True时,允许插入重复的列标签
df.insert(1, 'C', [10, 11, 12], allow_duplicates=True)
print("\n允许重复列标签时插入新列后的DataFrame:")
print(df)
430-6-3、结果输出
python
# 430、pandas.DataFrame.insert方法
# 原始DataFrame:
# A B
# 0 1 4
# 1 2 5
# 2 3 6
#
# 插入新列后的DataFrame:
# A C B
# 0 1 7 4
# 1 2 8 5
# 2 3 9 6
#
# 尝试插入重复列标签时捕获的异常:
# cannot insert C, already exists
#
# 允许重复列标签时插入新列后的DataFrame:
# A C C B
# 0 1 10 7 4
# 1 2 11 8 5
# 2 3 12 9 6