💡💡💡本文内容:针对基于YOLOv8的无人机高空红外(HIT-UAV)检测算法进行性能提升,加入各个创新点做验证性试验。
💡💡💡一种新的混合型扩张型残差注意力(HDRAB),去除图像采集或传输过程中产生的真实噪声(即空间变异噪声)
1)混合型扩张型残差注意力(HDRAB):mAP从原始的0.773 提升至 0 .779
1.无人机高空红外数据集介绍
无人机高空红外检测数据集大小,训练集2008,验证集287,测试集571张,类别
0: Person
1: Car
2: Bicycle
3: OtherVehicle
4: DontCare
细节图如下:
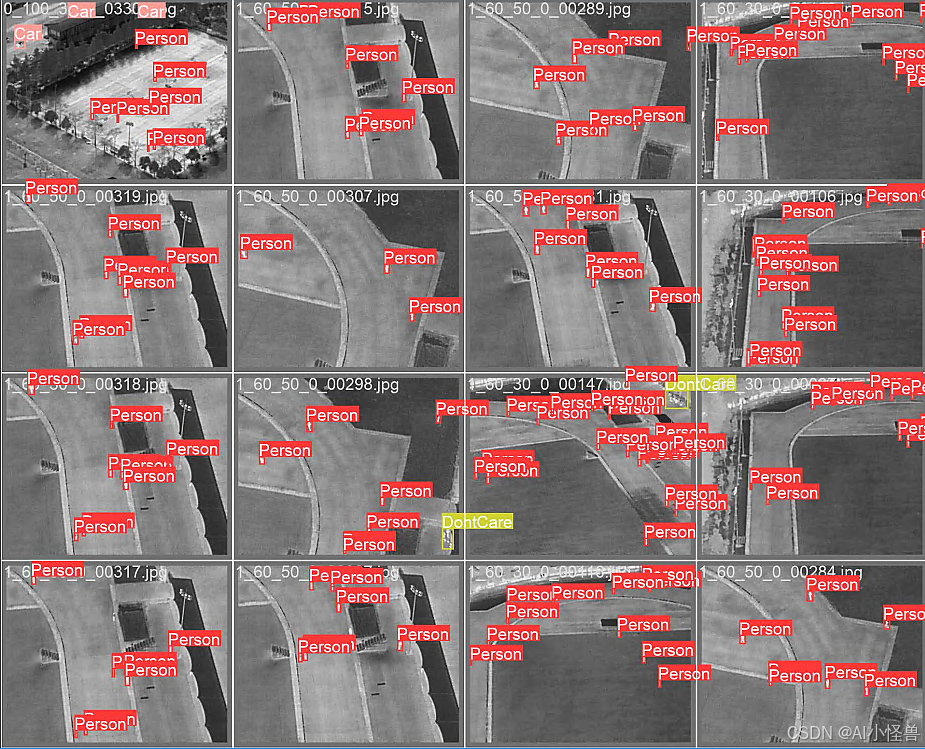
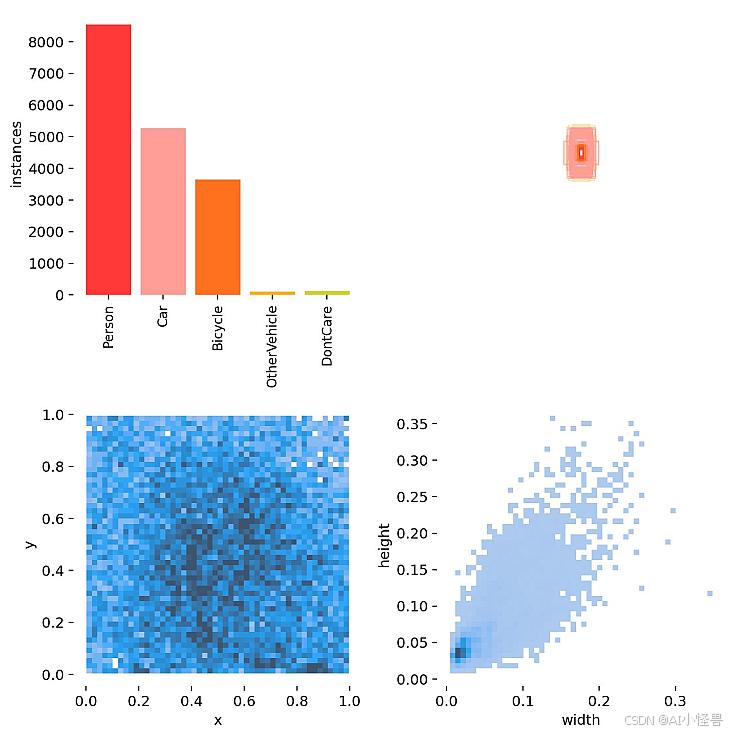
1.1 split_train_val.py
# coding:utf-8
import os
import random
import argparse
parser = argparse.ArgumentParser()
#xml文件的地址,根据自己的数据进行修改 xml一般存放在Annotations下
parser.add_argument('--xml_path', default='Annotations', type=str, help='input xml label path')
#数据集的划分,地址选择自己数据下的ImageSets/Main
parser.add_argument('--txt_path', default='ImageSets/Main', type=str, help='output txt label path')
opt = parser.parse_args()
trainval_percent = 0.9
train_percent = 0.8
xmlfilepath = opt.xml_path
txtsavepath = opt.txt_path
total_xml = os.listdir(xmlfilepath)
if not os.path.exists(txtsavepath):
os.makedirs(txtsavepath)
num = len(total_xml)
list_index = range(num)
tv = int(num * trainval_percent)
tr = int(tv * train_percent)
trainval = random.sample(list_index, tv)
train = random.sample(trainval, tr)
file_trainval = open(txtsavepath + '/trainval.txt', 'w')
file_test = open(txtsavepath + '/test.txt', 'w')
file_train = open(txtsavepath + '/train.txt', 'w')
file_val = open(txtsavepath + '/val.txt', 'w')
for i in list_index:
name = total_xml[i][:-4] + '\n'
if i in trainval:
file_trainval.write(name)
if i in train:
file_train.write(name)
else:
file_val.write(name)
else:
file_test.write(name)
file_trainval.close()
file_train.close()
file_val.close()
file_test.close()
1.2 voc_label.py生成适合YOLOv8训练的txt
# -*- coding: utf-8 -*-
import xml.etree.ElementTree as ET
import os
from os import getcwd
sets = ['train', 'val', 'test']
classes = ["Person","Car","Bicycle","OtherVehicle","DontCare"] # 改成自己的类别
abs_path = os.getcwd()
print(abs_path)
def convert(size, box):
dw = 1. / (size[0])
dh = 1. / (size[1])
x = (box[0] + box[1]) / 2.0 - 1
y = (box[2] + box[3]) / 2.0 - 1
w = box[1] - box[0]
h = box[3] - box[2]
x = x * dw
w = w * dw
y = y * dh
h = h * dh
return x, y, w, h
def convert_annotation(image_id):
in_file = open('Annotations/%s.xml' % (image_id), encoding='UTF-8')
out_file = open('labels/%s.txt' % (image_id), 'w')
tree = ET.parse(in_file)
root = tree.getroot()
size = root.find('size')
w = int(size.find('width').text)
h = int(size.find('height').text)
for obj in root.iter('object'):
difficult = obj.find('difficult').text
#difficult = obj.find('Difficult').text
cls = obj.find('name').text
if cls not in classes or int(difficult) == 1:
continue
cls_id = classes.index(cls)
xmlbox = obj.find('bndbox')
b = (float(xmlbox.find('xmin').text), float(xmlbox.find('xmax').text), float(xmlbox.find('ymin').text),
float(xmlbox.find('ymax').text))
b1, b2, b3, b4 = b
# 标注越界修正
if b2 > w:
b2 = w
if b4 > h:
b4 = h
b = (b1, b2, b3, b4)
bb = convert((w, h), b)
out_file.write(str(cls_id) + " " + " ".join([str(a) for a in bb]) + '\n')
wd = getcwd()
for image_set in sets:
if not os.path.exists('labels/'):
os.makedirs('labels/')
image_ids = open('ImageSets/Main/%s.txt' % (image_set)).read().strip().split()
list_file = open('%s.txt' % (image_set), 'w')
for image_id in image_ids:
list_file.write(abs_path + '/images/%s.png\n' % (image_id))
convert_annotation(image_id)
list_file.close()
2.基于YOLOv8的无人机高空红外识别
2.1 原始结果
原始mAP为0.773
YOLOv8n summary (fused): 168 layers, 3006623 parameters, 0 gradients, 8.1 GFLOPs
Class Images Instances Box(P R mAP50 mAP50-95): 100%|██████████| 9/9 [00:07<00:00, 1.13it/s]
all 287 2460 0.818 0.707 0.773 0.525
Person 287 1168 0.933 0.799 0.908 0.495
Car 287 719 0.942 0.945 0.979 0.743
Bicycle 287 554 0.911 0.756 0.885 0.513
OtherVehicle 287 12 0.865 0.75 0.781 0.722
DontCare 287 7 0.439 0.286 0.314 0.152
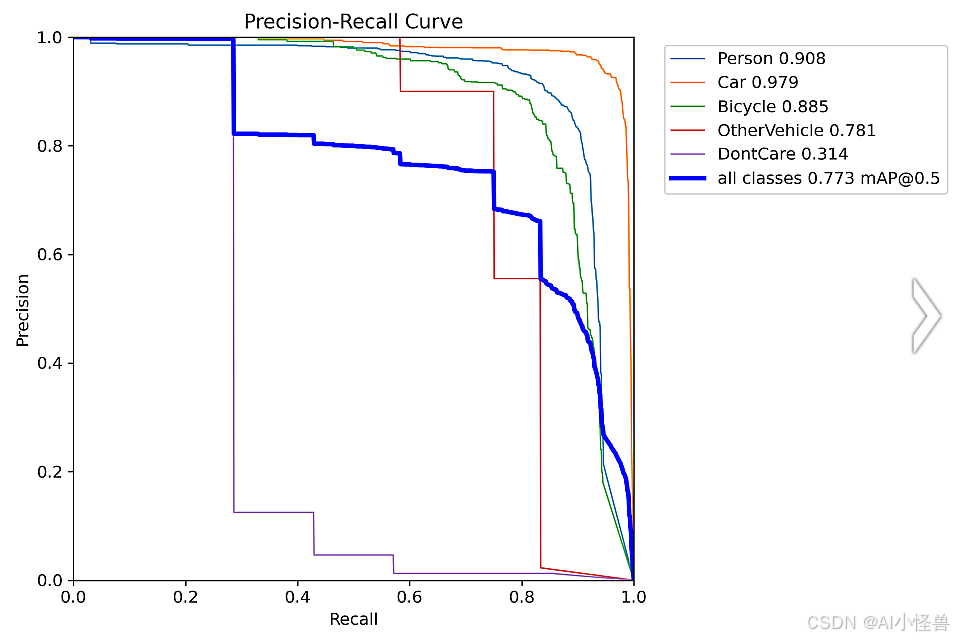
2.2 一种新颖的双分支残差注意,助力低光照、红外小目标检测
原文链接:YOLOv8独家原创改进:图像去噪 |一种新颖的双分支残差注意,助力低光照、红外小目标检测 | 2024年最新发表(全网独家首发)_yolo双分支网络-CSDN博客
💡💡💡解决什么问题:许多网络不能很好地去除图像采集或传输过程中产生的真实噪声(即空间变异噪声),这严重阻碍了它们在实际图像去噪任务中的应用。
💡💡💡创新点: 提出了一种新的双分支残差注意网络用于图像去噪 ,它具有广泛的模型架构和注意引导特征学习的优点。该模型包含两个不同的并行分支,可以捕获互补特征,增强模型的学习能力。我们分别设计了一种新的残差注意力(RAB)和一种新的混合型扩张型残差注意力(HDRAB)。
改进1结构图如下:
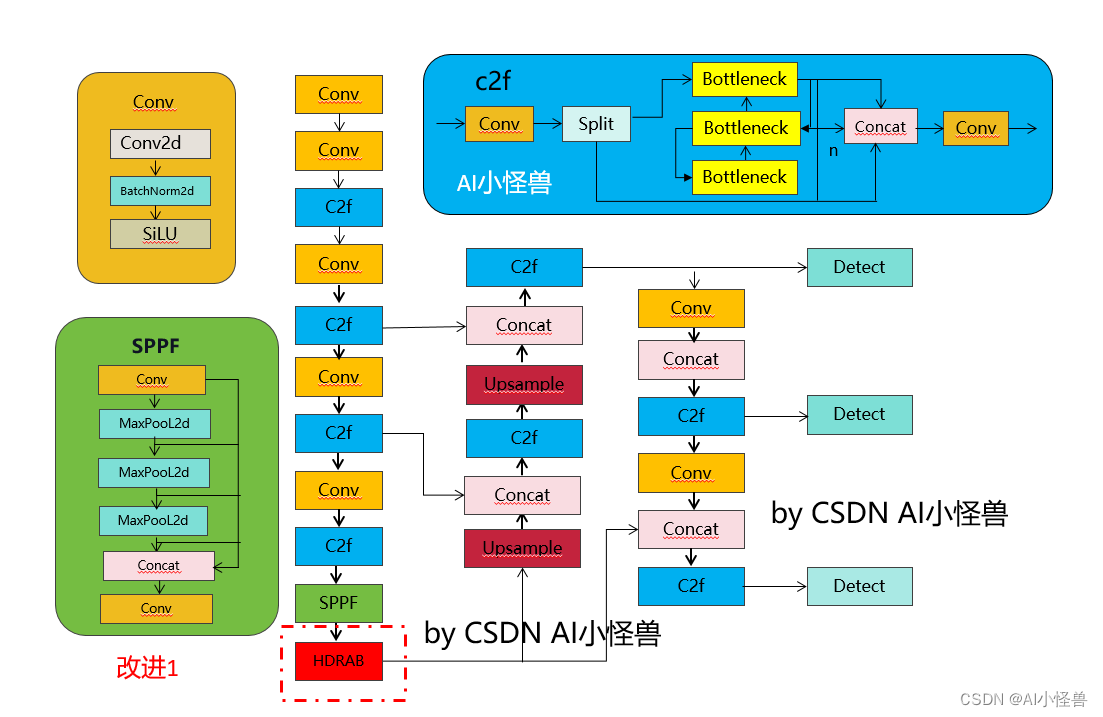
mAP有原始的0.773提升至0.779
YOLOv8-HDRAB summary (fused): 248 layers, 10410279 parameters, 0 gradients, 23.2 GFLOPs
Class Images Instances Box(P R mAP50 mAP50-95): 100%|██████████| 9/9 [00:09<00:00, 1.02s/it]
all 287 2460 0.832 0.754 0.779 0.498
Person 287 1168 0.841 0.887 0.904 0.489
Car 287 719 0.911 0.962 0.978 0.746
Bicycle 287 554 0.8 0.843 0.892 0.52
OtherVehicle 287 12 0.869 0.667 0.729 0.611
DontCare 287 7 0.738 0.408 0.392 0.125
3.系列篇
3) 一种基于YOLOv8的高精度无人机高空红外检测算法(原创自研)
关注下方名片点击关注,源码获取途径。