Improving LoRA: Implementing Weight-Decomposed Low-Rank Adaptation (DoRA) from Scratch
LoRA
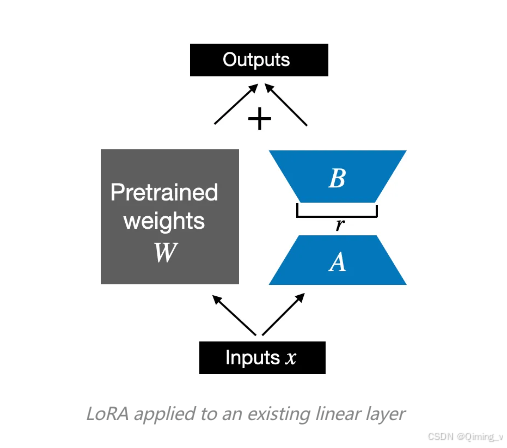
LoRA初始化时,A使用正态分布,B使用0.
python
class LoRALayer(nn.Module):
def __init__(self, in_dim, out_dim, rank, alpha):
super().__init__()
std_dev = 1 / torch.sqrt(torch.tensor(rank).float())
self.A = nn.Parameter(torch.randn(in_dim, rank) * std_dev)
self.B = nn.Parameter(torch.zeros(rank, out_dim))
self.alpha = alpha
def forward(self, x):
x = self.alpha * (x @ self.A @ self.B)
return x
class LinearWithLoRA(nn.Module):
def __init__(self, linear, rank, alpha):
super().__init__()
self.linear = linear
self.lora = LoRALayer(
linear.in_features, linear.out_features, rank, alpha
)
def forward(self, x):
return self.linear(x) + self.lora(x)
在训练的时候,使用LinearWithLoRA 替换Linear层,并且freeze Linear的参数,只训练lora的参数。
python
model_lora = copy.deepcopy(model_pretrained)
model_lora.layers[0] = LinearWithLoRA(model_lora.layers[0], rank=4, alpha=8)
freeze_linear_layers(model_lora)
#然后就可以正常训练了
freeze Linear的参数
python
def freeze_linear_layers(model):
for child in model.children():
if isinstance(child, nn.Linear):
for param in child.parameters():
param.requires_grad = False
else:
# Recursively freeze linear layers in children modules
freeze_linear_layers(child)
DoRA (Weight-Decomposed Low-Rank Adaptation)
权重weight矩阵W,可以分为 模向量m(magnitude vector)和方向矩阵V(directional matrix)。
把LoRA加入到方向矩阵V中,然后再和m计算出新的权重W。
使用方法和LoRA相同。
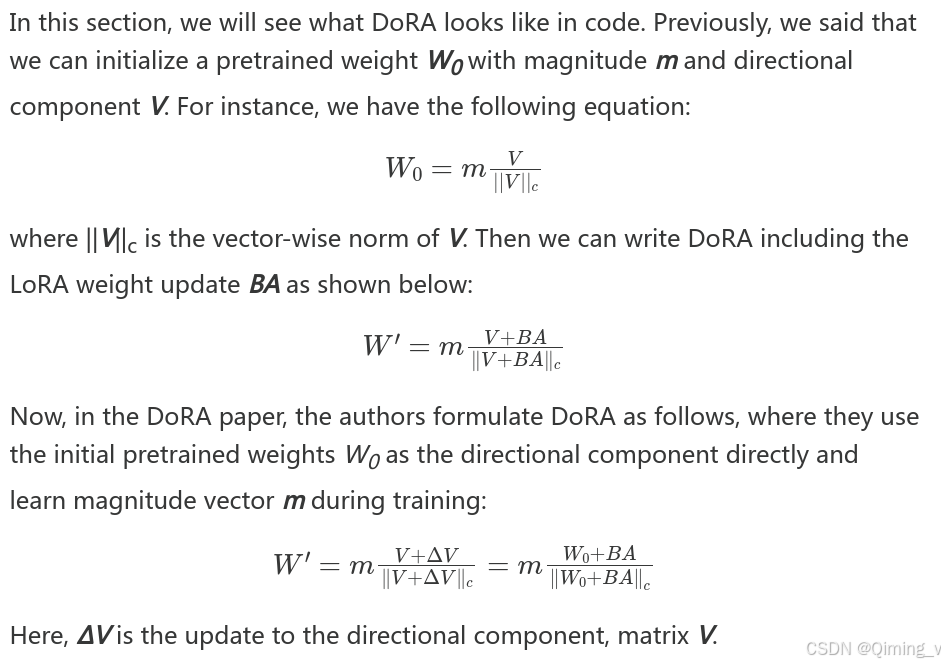
python
#训练时
class LinearWithDoRA(nn.Module):
def __init__(self, linear, rank, alpha):
super().__init__()
self.linear = linear
self.lora = LoRALayer(linear.in_features, linear.out_features, rank, alpha)
self.m = nn.Parameter(torch.ones(1, linear.out_features))
def forward(self, x):
linear_output = self.linear(x)
lora_output = self.lora(x)
lora_output_norm = lora_output / (lora_output.norm(p=2, dim=1, keepdim=True) + 1e-9)
dora_modification = self.m * lora_output_norm
return linear_output + dora_modification
#合并时
# Code inspired by https://github.com/catid/dora/blob/main/dora.py
class LinearWithDoRAMerged(nn.Module):
def __init__(self, linear, rank, alpha):
super().__init__()
self.linear = linear
self.lora = LoRALayer(
linear.in_features, linear.out_features, rank, alpha
)
self.m = nn.Parameter(
self.linear.weight.norm(p=2, dim=0, keepdim=True))
def forward(self, x):
lora = self.lora.A @ self.lora.B
numerator = self.linear.weight + self.lora.alpha*lora.T
denominator = numerator.norm(p=2, dim=0, keepdim=True)
directional_component = numerator / denominator
new_weight = self.m * directional_component
return F.linear(x, new_weight, self.linear.bias)