1、目的
探索visual tokenizer编码下的MIM(Masked Image Modeling)
2、方法
iBOT(i mage B ERT pre-training with O nline Tokenizer)
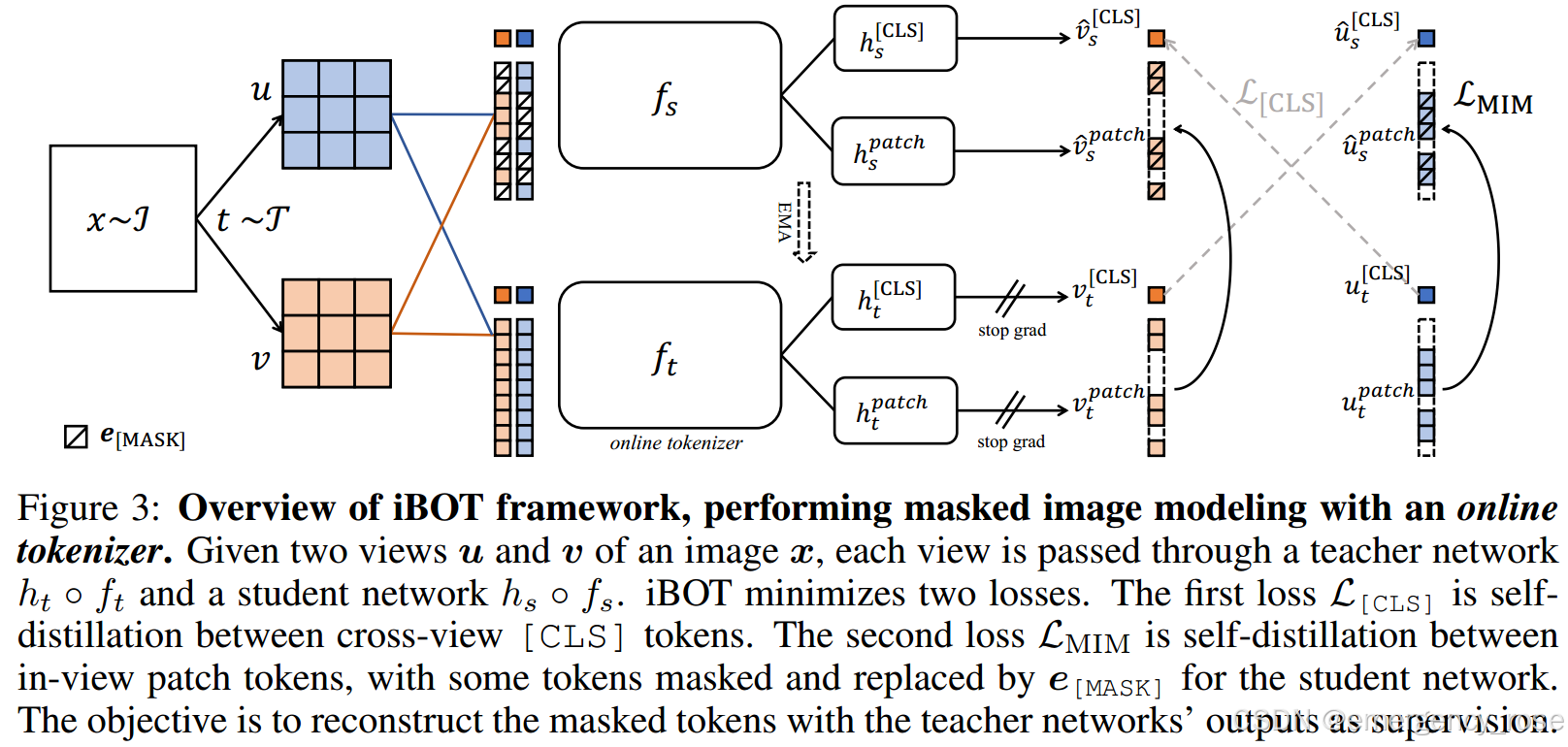
1)knowledge distillation(KD)
distill knowledge from the tokenizer
2)self-distillation
twin teacher as online tokenizer
3)visual tokenizer
-> transform the masked patches to supervisory signals for the target model
-> 通过enforce the similarity of cross-view images on class tokens,来捕获到high-level visual semantics
-> 无须额外的训练,通过momentum update来和MIM一同被优化
-> online,而不是pre-fixed
4)网络结构
-> ,
-> 用softmax之后的token,而非ont-hot
5)multi-crop
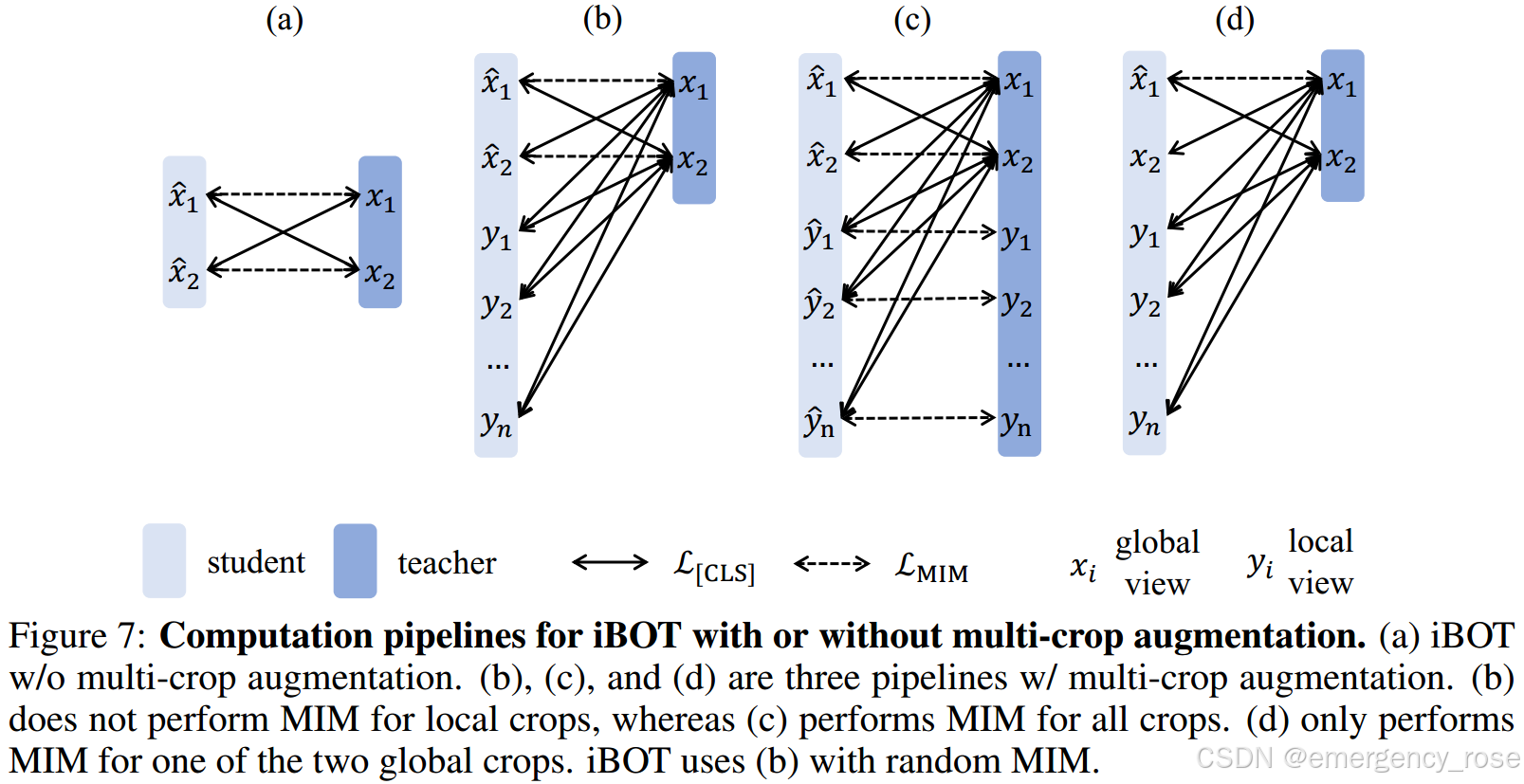
6)MIM
3、结论
1)iBOT is more scalable to larger models
2)iBOT requires more data to train larger model