1、基础配置
底层模型:Qwen开源模型
swarm:0.1.0
2、agent设置
python
agent_a = Agent(name="Agent A", instructions="你是Agent A,是一个有用的助手", functions=[transfer_to_agent_b], model=model_name,
tool_choice='auto')
agent_b = Agent(name="Agent B", instructions="你是Agent B,仅仅使用繁体字说话", functions=[transfer_to_agent_a],model=model_name, tool_choice='auto')
agent之间的跳转函数
python
def transfer_to_agent_b():
return agent_b
def transfer_to_agent_a():
return agent_a
3、单智能体示例
输入示例:
python
msg = [{"role": "user", "content": "你好,小明的年龄是12岁。"},
{"role": "user", "content": "我的年龄是小明的2倍"},
{"role": "user", "content": "请问我的年龄是多少"}]
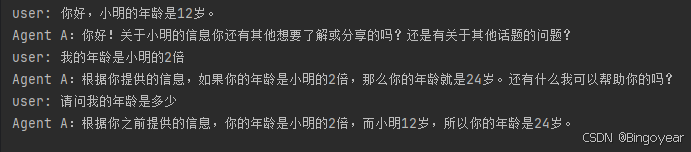
4、多智能体示例
输入示例
python
msg = [{"role": "user", "content": "你好,你是谁?"},
{"role": "user", "content": "我想和Agent B说话"},
{"role": "user", "content": "你好,你是谁?由哪家公司训练出来的?"},
{"role": "user", "content": "写一副春节对联"},
{"role": "user", "content": "帮我转到agent a"},
{"role": "user", "content": "你好,你是哪家公司训练出来的?"},
{"role": "user", "content": "写一首七言绝句,有关爱情的。"}]
初始的agent设置为Agent A,上面问答的基本流程是: Agent A >>>Agent B >>>Agent A,具体输出如下
可以看到,开源模型+Swarm框架,可以完成Agent之间的跳转,回复效果还不错。
5、测试脚本
python
agent = agent_a
history = []
history_dict = {'history_agent_a':[], 'history_agent_b':[]}
for m in msg:
print('{}: {}'.format(m['role'], m['content']))
history = history + [m]
str_name_forward = agent.name.lower().replace(' ','_')
history_dict['history_{}'.format(str_name_forward)] += [m]
response = client.run(agent=agent, messages=history_dict['history_{}'.format(str_name_forward)], history_messages=history_dict)
agent = response.agent
res = response.messages
str_name_backward = agent.name.lower().replace(' ', '_')
history_dict['history_{}'.format(str_name_backward)] += res
history = history + res
print("{}:{}".format(response.agent.name, response.messages[-1]["content"]))
history保存全部会话记录,history_agent_a和history_agent_b分别保存子Agent的会话记录
swarm git链接:swarm