1. Tensorflow vs Pytorch
在这个AI时代,各大厂商都在主推自家的AI框架,因此知名和不知名的大大小小可能十来种。但是我们选型的时候,一般首先考虑是Google家的Tensorflow呢还是Meta家的Pytorch。
在选择 PyTorch 或 TensorFlow 进行工业级开发时,以下是一些考虑因素:
1.1. PyTorch
-
易用性和灵活性:
- PyTorch 的动态计算图使其更易于调试和开发。代码风格类似于 Python,因此对开发者友好。
- 适合研究和快速原型开发。
-
社区和支持:
- PyTorch 拥有活跃的社区和大量的开源项目支持。Facebook 主要推动其发展。
- 在学术界和研究领域非常受欢迎。
-
性能:
- PyTorch 具有优秀的性能,特别是在 GPU 上进行训练和推理。
- 支持分布式训练。
1.2. TensorFlow
-
生产环境和部署:
- TensorFlow 在工业级生产环境中的部署工具更为完善。其 TensorFlow Serving、TensorFlow Lite 和 TensorFlow.js 提供了多种部署选项,涵盖服务器、移动设备和网页。
- TensorFlow Extended (TFX) 提供了一套完整的生产级机器学习流水线工具。
-
社区和支持:
- 由 Google 维护,拥有强大的技术支持和丰富的资源。
- 拥有广泛的企业使用案例和成功部署经验。
-
兼容性和扩展性:
- TensorFlow 2.0 之后引入了更易用的 API,改善了用户体验,同时保留了强大的功能和灵活性。
- 支持多种编程语言,包括 Python、C++ 和 JavaScript。
1.3. 简单的说
- PyTorch:适合需要灵活性、易用性以及快速开发原型的项目,特别是在研究和开发阶段。
- TensorFlow:适合需要稳定性、成熟的生产环境支持和多种部署选项的工业级项目。
如果你的项目需要快速迭代和实验,可以选择 PyTorch。如果你更关注部署和生产环境的稳定性,TensorFlow 可能是更好的选择。
1.4. 但是...
但是,孩子才做选择,成年人都是
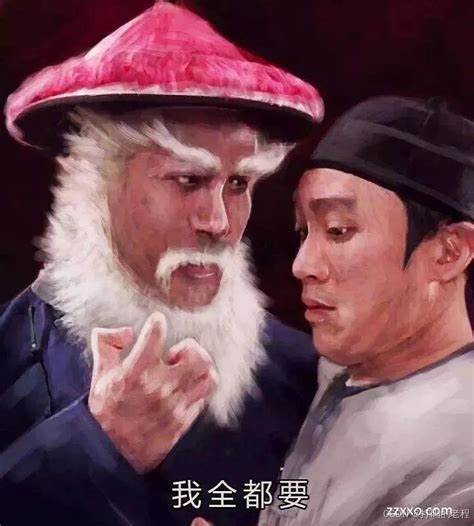
但是,我们不禁又遇到一个问题,怎么即享受Pytorch带来的便利的同时,还能拥有Tensorflow的广泛性。所以这篇文章就是告诉你该怎么做的一个指南。
2. 将Pytorch模型转换成Tensorflow的模型
步骤 1:使用 PyTorch 训练和保存模型
假设我们使用 PyTorch 训练了一个简单的模型,并将其保存为 .pt
文件。
python
import torch
import torch.nn as nn
# 定义一个简单的模型
class SimpleModel(nn.Module):
def __init__(self):
super(SimpleModel, self).__init__()
self.fc1 = nn.Linear(28 * 28, 128)
self.fc2 = nn.Linear(128, 10)
def forward(self, x):
x = torch.relu(self.fc1(x))
x = self.fc2(x)
return x
# 实例化模型并训练(假设训练代码已完成)
model = SimpleModel()
# 保存 PyTorch 模型
torch.save(model.state_dict(), 'simple_model.pth')
步骤 2:将 PyTorch 模型转换为 ONNX 格式
ONNX(Open Neural Network Exchange)是一种开放的中间格式,支持从 PyTorch 导出并在 TensorFlow 中导入。
python
import torch.onnx
# 定义输入张量的大小(例如,MNIST 数据集的输入是 1x28x28)
dummy_input = torch.randn(1, 28 * 28)
# 加载模型并导出为 ONNX 格式
model.load_state_dict(torch.load('simple_model.pth'))
model.eval()
torch.onnx.export(model, dummy_input, 'simple_model.onnx', input_names=['input'], output_names=['output'])
步骤 3:将 ONNX 模型转换为 TensorFlow SavedModel 格式
可以使用 onnx-tf
工具将 ONNX 模型转换为 TensorFlow SavedModel 格式。
-
安装
onnx-tf
:bashpip install onnx-tf
-
使用
onnx-tf
进行转换:bashonnx-tf convert -i simple_model.onnx -o simple_model_saved_model
步骤 4:将 TensorFlow SavedModel 转换为 TensorFlow Lite 格式
有了 TensorFlow SavedModel 后,我们可以使用 TensorFlow Lite 转换器将其转换为 .tflite
文件。
python
import tensorflow as tf
# 加载 SavedModel
converter = tf.lite.TFLiteConverter.from_saved_model('simple_model_saved_model')
# 可选:进行量化来优化模型大小和速度(例如动态范围量化)
converter.optimizations = [tf.lite.Optimize.DEFAULT]
# 转换模型为 TFLite 格式
tflite_model = converter.convert()
# 保存 TFLite 模型
with open('simple_model.tflite', 'wb') as f:
f.write(tflite_model)
步骤 5:将 TensorFlow Lite 模型部署到目标设备上并运行推理
将 .tflite
文件复制到目标设备(例如树莓派)上,并使用 TensorFlow Lite 的解释器运行推理。
在树莓派或其他设备上,可以使用 Python 代码加载和运行 TensorFlow Lite 模型:
python
import numpy as np
import tensorflow as tf
# 加载 TFLite 模型
interpreter = tf.lite.Interpreter(model_path='simple_model.tflite')
interpreter.allocate_tensors()
# 获取输入和输出张量的信息
input_details = interpreter.get_input_details()
output_details = interpreter.get_output_details()
# 创建一个模拟的输入数据(例如手写数字图像)
input_data = np.random.rand(1, 28 * 28).astype(np.float32)
# 填充输入数据
interpreter.set_tensor(input_details[0]['index'], input_data)
# 执行推理
interpreter.invoke()
# 获取输出结果
output_data = interpreter.get_tensor(output_details[0]['index'])
print("Predicted output:", output_data)
3. 如何在树莓派上运行 Tensorflow Lite 模型
在树莓派上运行 TensorFlow Lite (TFLite) 文件,你需要安装 TensorFlow Lite 运行时。这是一个轻量级的版本,专门为边缘设备优化,适合树莓派等资源受限的环境。以下是如何在树莓派上安装 TensorFlow Lite 运行时的步骤:
3.1. 安装 TensorFlow Lite 运行时
TensorFlow Lite 提供了专门为树莓派编译的二进制文件,可以直接通过 pip
安装。你需要确保树莓派上已经安装了 Python 3(建议使用 Python 3.7 或更高版本)。
-
更新系统包:
bashsudo apt-get update sudo apt-get upgrade
-
安装 TensorFlow Lite 运行时:
bashpip3 install tflite-runtime
这将安装 TensorFlow Lite 运行时的轻量级版本,它只包含运行 TFLite 模型所需的必要组件。
3.2. 测试安装
安装完成后,你可以测试一下是否成功安装了 TensorFlow Lite 运行时。
python
import tflite_runtime.interpreter as tflite
print("TensorFlow Lite Runtime version:", tflite.__version__)
如果没有报错并打印出版本信息,就说明安装成功。
3.3. 运行 TFLite 模型
下面是一个在树莓派上运行 TFLite 模型的示例代码:
python
import numpy as np
import tflite_runtime.interpreter as tflite
# 加载 TFLite 模型
interpreter = tflite.Interpreter(model_path="your_model.tflite")
interpreter.allocate_tensors()
# 获取输入和输出张量的信息
input_details = interpreter.get_input_details()
output_details = interpreter.get_output_details()
# 创建一个模拟输入数据(例如 1x28x28 的 MNIST 图像)
input_shape = input_details[0]['shape']
input_data = np.random.rand(*input_shape).astype(np.float32)
# 填充输入数据
interpreter.set_tensor(input_details[0]['index'], input_data)
# 执行推理
interpreter.invoke()
# 获取输出结果
output_data = interpreter.get_tensor(output_details[0]['index'])
print("Predicted output:", output_data)