在数据隐私至关重要的时代,建立自己的本地语言模型 (LLM) 为公司和个人都提供了至关重要的解决方案。本教程旨在指导您完成使用 Ollama、Python 3 和 ChromaDB 创建自定义聊天机器人的过程,所有这些机器人都托管在您的系统本地。以下是您需要本教程的主要原因:
完全定制:在本地托管您自己的 Retrieval-Augmented Generation (RAG) 应用程序意味着您可以完全控制设置和定制。您可以微调模型以满足您的特定需求,而无需依赖外部服务。
增强的隐私性:通过在本地设置 LLM 模型,您可以避免与通过 Internet 发送敏感数据相关的风险。这对于处理机密信息的公司尤其重要。在本地使用私有数据训练模型可确保您的数据始终处于您的控制范围内。
数据安全:使用第三方 LLM 模型可能会使您的数据面临潜在的泄露和滥用风险。本地部署通过将训练数据(如 PDF 文档)保存在安全环境中来降低这些风险。
控制数据处理: 当您托管自己的 LLM 时,您能够完全按照自己的方式管理和处理数据。这包括将您的私有数据嵌入到 ChromaDB 矢量存储中,确保您的数据处理符合您的标准和要求。
独立于互联网连接: 在本地运行您的聊天机器人意味着您不依赖于互联网连接。这保证了不间断的服务和对聊天机器人的访问,即使在离线场景中也是如此。
本教程将使您能够构建一个强大且安全的本地聊天机器人,根据您的需求量身定制,而不会影响隐私或控制。
微调模型
检索增强生成 (RAG)
检索增强生成 (RAG) 是一种高级技术,它结合了信息检索和文本生成的优势,以创建更准确且与上下文相关的响应。以下是 RAG 的工作原理及其益处的细分:
什么是 RAG?
RAG 是一种混合模型,它通过整合外部知识库或文档存储来增强语言模型的功能。该过程涉及两个主要部分:
检索:在此阶段,模型根据输入查询从外部源(如数据库或矢量存储)检索相关文档或信息。
生成:然后,生成语言模型使用检索到的信息来生成连贯且上下文适当的响应。
RAG 是如何工作的?
Query Input:用户输入查询或问题。
文档检索:系统使用查询搜索外部知识库,检索最相关的文档或信息片段。
响应生成:生成模型处理检索到的信息,将其与自己的知识集成,以生成详细而准确的响应。
输出:向用户显示最终响应,其中包含知识库中的具体相关详细信息。
RAG 的好处
提高准确性:通过利用外部数据,RAG 模型可以提供更精确和详细的答案,尤其是对于特定于领域的查询。
上下文相关性:检索组件可确保生成的响应基于相关和最新的信息,从而提高响应的整体质量。
可扩展性:RAG 系统可以轻松扩展以整合大量数据,使它们能够处理各种查询和主题。
灵活性:这些模型可以通过简单地更新或扩展外部知识库来适应各种领域,使其具有高度的通用性。
为什么在本地使用 RAG?
隐私和安全:在本地运行 RAG 模型可确保敏感数据保持安全和私密,因为它不需要发送到外部服务器。
自定义:您可以定制检索和生成流程以满足您的特定需求,包括集成专有数据源。
独立性:本地设置可确保您的系统即使在没有互联网连接的情况下也能保持运行,从而提供一致且可靠的服务。
通过使用 Ollama、Python 和 ChromaDB 等工具设置本地 RAG 应用程序,您可以享受高级语言模型的好处,同时保持对数据和自定义选项的控制。
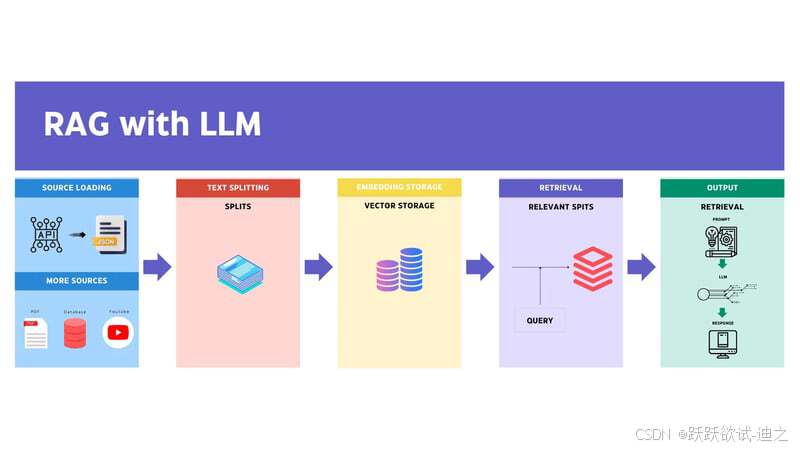
图形处理器
运行大型语言模型 (LLM),如 Retrieval-Augmented Generation (RAG) 中使用的模型,需要强大的计算能力。图形处理单元 (GPU) 是实现在这些模型中高效处理和嵌入数据的关键组件之一。以下是 GPU 对于此任务至关重要的原因,以及它们如何影响本地 LLM 设置的性能:
什么是 GPU?
GPU 是一种专用处理器,旨在加速图像和视频的渲染。与针对顺序处理任务优化的中央处理单元 (CPU) 不同,GPU 在并行处理方面表现出色。这使得它们特别适合机器学习和深度学习模型所需的复杂数学计算。
为什么 GPU 对 LLM 很重要
并行处理能力:GPU 可以同时处理数千次操作,显著加快 LLM 中的训练和推理等任务。这种并行性对于与处理大型数据集和实时生成响应相关的繁重计算负载至关重要。
处理大型模型的效率:RAG 中使用的 LLM 需要大量的内存和计算资源。GPU 配备了高带宽内存 (HBM) 和多核,使其能够管理这些模型所需的大规模矩阵乘法和张量运算。
更快的数据嵌入和检索:在本地 RAG 设置中,将数据嵌入到 ChromaDB 等向量存储中并快速检索相关文档对于性能至关重要。高性能 GPU 可以加速这些过程,确保您的聊天机器人及时准确地做出响应。
改进的训练时间:训练 LLM 涉及调整数百万(甚至数十亿)个参数。与 CPU 相比,GPU 可以大大减少此训练阶段所需的时间,从而更频繁地更新和优化模型。
选择合适的 GPU
在设置本地 LLM 时,GPU 的选择会显著影响性能。以下是一些需要考虑的因素:
内存容量:较大的模型需要更多的 GPU 内存。寻找具有更高 VRAM(视频 RAM)的 GPU,以容纳广泛的数据集和模型参数。
计算能力:GPU 拥有的 CUDA 内核越多,它处理并行处理任务的能力就越好。具有更高计算能力的 GPU 对于深度学习任务的效率更高。
带宽:更高的内存带宽允许在 GPU 与其内存之间更快地传输数据,从而提高整体处理速度。
用于 LLM 的高性能 GPU 示例
NVIDIA RTX 3090:以其高 VRAM (24 GB) 和强大的 CUDA 内核而闻名,它是深度学习任务的热门选择。
NVIDIA A100:专为 AI 和机器学习而设计,它提供卓越的性能、大内存容量和高计算能力。
AMD Radeon Pro VII:另一个强大的竞争者,具有高内存带宽和高效的处理能力。
投资高性能 GPU 对于在本地运行 LLM 模型至关重要。它可确保更快的数据处理、高效的模型训练和快速响应生成,使您的本地 RAG 应用程序更加健壮可靠。通过利用 GPU 的强大功能,您可以充分实现托管自己的自定义聊天机器人的好处,这些聊天机器人是根据您的特定需求和数据隐私要求量身定制的。
先决条件
在深入研究设置之前,请确保您满足以下先决条件:
Python 3:Python 是一种通用编程语言,您将使用它来为您的 RAG 应用程序编写代码。
ChromaDB:一个向量数据库,将存储和管理我们数据的嵌入。
Ollama:在我们的本地计算机中下载和提供自定义 LLM。
第 1 步:安装 Python 3 并设置您的环境
要安装和设置我们的 Python 3 环境,请执行以下步骤:
在您的计算机上下载并设置 Python 3。
然后确保您的 Python 3 安装并成功运行:
$ python3 --version
Python 3.11.7
为项目创建一个文件夹,例如:local-rag
$ mkdir local-rag
$ cd local-rag
创建名为 的虚拟环境 :venv
$ python3 -m venv venv
激活虚拟环境:
$ source venv/bin/activate
Windows
venv\Scripts\activate
第 2 步:安装 ChromaDB 和其他依赖项
使用 pip 安装 ChromaDB:
$ pip install --q chromadb
Install Langchain tools to work seamlessly with your model:
$ pip install --q unstructured langchain langchain-text-splitters
$ pip install --q "unstructured[all-docs]"
安装 Flask 以将应用程序作为 HTTP 服务提供:
$ pip install --q flask
第 3 步:安装 Ollama
要安装 Ollama,请按照下列步骤操作:
前往 Ollama 下载页面,然后下载适用于您的操作系统的安装程序。
通过运行以下命令来验证您的 Ollama 安装:
$ ollama --version
ollama version is 0.1.47
拉取您需要的 LLM 模型。例如,要使用 Mistral 模型:
$ ollama pull mistral
拉取文本嵌入模型。例如,要使用 Nomic 嵌入文本模型:
$ ollama pull nomic-embed-text
然后运行您的 Ollama 模型:
$ ollama serve
构建 RAG 应用程序
现在,您已经使用 Python、Ollama、ChromaDB 和其他依赖项设置了环境,是时候构建自定义本地 RAG 应用程序了。在本节中,我们将演练动手实践的 Python 代码,并概述如何构建您的应用程序。
This is the main Flask application file. It defines routes for embedding files to the vector database, and retrieving the response from the model.
import os
from dotenv import load_dotenv
load_dotenv()
from flask import Flask, request, jsonify
from embed import embed
from query import query
from get_vector_db import get_vector_db
TEMP_FOLDER = os.getenv('TEMP_FOLDER', './_temp')
os.makedirs(TEMP_FOLDER, exist_ok=True)
app = Flask(name)
@app.route('/embed', methods=['POST'])
def route_embed():
if 'file' not in request.files:
return jsonify({"error": "No file part"}), 400
file = request.files['file']
if file.filename == '':
return jsonify({"error": "No selected file"}), 400
embedded = embed(file)
if embedded:
return jsonify({"message": "File embedded successfully"}), 200
return jsonify({"error": "File embedded unsuccessfully"}), 400
@app.route('/query', methods=['POST'])
def route_query():
data = request.get_json()
response = query(data.get('query'))
if response:
return jsonify({"message": response}), 200
return jsonify({"error": "Something went wrong"}), 400
if name == 'main ':
app.run(host="0.0.0.0", port=8080, debug=True)
This module handles the embedding process, including saving uploaded files, loading and splitting data, and adding documents to the vector database.
import os
from datetime import datetime
from werkzeug.utils import secure_filename
from langchain_community.document_loaders import UnstructuredPDFLoader
from langchain_text_splitters import RecursiveCharacterTextSplitter
from get_vector_db import get_vector_db
TEMP_FOLDER = os.getenv('TEMP_FOLDER', './_temp')
Function to check if the uploaded file is allowed (only PDF files)
def allowed_file(filename):
return '.' in filename and filename.rsplit('.', 1)[1].lower() in {'pdf'}
Function to save the uploaded file to the temporary folder
def save_file(file):
Save the uploaded file with a secure filename and return the file path
ct = datetime.now()
ts = ct.timestamp()
filename = str(ts) + "_" + secure_filename(file.filename)
file_path = os.path.join(TEMP_FOLDER, filename)
file.save(file_path)
return file_path
Function to load and split the data from the PDF file
def load_and_split_data(file_path):
Load the PDF file and split the data into chunks
loader = UnstructuredPDFLoader(file_path=file_path)
data = loader.load()
text_splitter = RecursiveCharacterTextSplitter(chunk_size=7500, chunk_overlap=100)
chunks = text_splitter.split_documents(data)
return chunks
Main function to handle the embedding process
def embed(file):
Check if the file is valid, save it, load and split the data, add to the database, and remove the temporary file
if file.filename != '' and file and allowed_file(file.filename):
file_path = save_file(file)
chunks = load_and_split_data(file_path)
db = get_vector_db()
db.add_documents(chunks)
db.persist()
os.remove(file_path)
return True
return False
This module processes user queries by generating multiple versions of the query, retrieving relevant documents, and providing answers based on the context.
import os
from langchain_community.chat_models import ChatOllama
from langchain.prompts import ChatPromptTemplate, PromptTemplate
from langchain_core.output_parsers import StrOutputParser
from langchain_core.runnables import RunnablePassthrough
from langchain.retrievers.multi_query import MultiQueryRetriever
from get_vector_db import get_vector_db
LLM_MODEL = os.getenv('LLM_MODEL', 'mistral')
Function to get the prompt templates for generating alternative questions and answering based on context
def get_prompt():
QUERY_PROMPT = PromptTemplate(
input_variables=["question"],
template="""You are an AI language model assistant. Your task is to generate five
different versions of the given user question to retrieve relevant documents from
a vector database. By generating multiple perspectives on the user question, your
goal is to help the user overcome some of the limitations of the distance-based
similarity search. Provide these alternative questions separated by newlines.
Original question: {question}""",
)
template = """Answer the question based ONLY on the following context:
{context}
Question: {question}
"""
prompt = ChatPromptTemplate.from_template(template)
return QUERY_PROMPT, prompt
Main function to handle the query process
def query(input):
if input:
Initialize the language model with the specified model name
llm = ChatOllama(model=LLM_MODEL)
Get the vector database instance
db = get_vector_db()
Get the prompt templates
QUERY_PROMPT, prompt = get_prompt()
# Set up the retriever to generate multiple queries using the language model and the query prompt
retriever = MultiQueryRetriever.from_llm(
db.as_retriever(),
llm,
prompt=QUERY_PROMPT
)
# Define the processing chain to retrieve context, generate the answer, and parse the output
chain = (
{"context": retriever, "question": RunnablePassthrough()}
| prompt
| llm
| StrOutputParser()
)
response = chain.invoke(input)
return response
return None
get_vector_db.py
This module initializes and returns the vector database instance used for storing and retrieving document embeddings.
import os
from langchain_community.embeddings import OllamaEmbeddings
from langchain_community.vectorstores.chroma import Chroma
CHROMA_PATH = os.getenv('CHROMA_PATH', 'chroma')
COLLECTION_NAME = os.getenv('COLLECTION_NAME', 'local-rag')
TEXT_EMBEDDING_MODEL = os.getenv('TEXT_EMBEDDING_MODEL', 'nomic-embed-text')
def get_vector_db():
embedding = OllamaEmbeddings(model=TEXT_EMBEDDING_MODEL,show_progress=True)
db = Chroma(
collection_name=COLLECTION_NAME,
persist_directory=CHROMA_PATH,
embedding_function=embedding
)
return db
运行您的应用程序!
创建文件来存储您的环境变量:.env
TEMP_FOLDER = './_temp'
CHROMA_PATH = 'chroma'
COLLECTION_NAME = 'local-rag'
LLM_MODEL = 'mistral'
TEXT_EMBEDDING_MODEL = 'nomic-embed-text'
运行该文件以启动您的应用程序服务器:app.py
$ python3 app.py
服务器运行后,您可以开始向以下终端节点发出请求:
嵌入 PDF 文件的示例命令(例如,resume.pdf): '''猛击
$ curl --request POST
--url http://localhost:8080/embed
--header 'Content-Type: multipart/form-data'
--form file=@/Users/nassermaronie/Documents/Nasser-resume.pdf
响应
{
"message": "文件嵌入成功"
}
- Example command to ask a question to your model:
bash
$ curl --request POST \
--url http://localhost:8080/query \
--header 'Content-Type: application/json' \
--data '{ "query": "Who is Nasser?" }'
# Response
{
"message": "Nasser Maronie is a Full Stack Developer with experience in web and mobile app development. He has worked as a Lead Full Stack Engineer at Ulventech, a Senior Full Stack Engineer at Speedoc, a Senior Frontend Engineer at Irvins, and a Software Engineer at Tokopedia. His tech stacks include Typescript, ReactJS, VueJS, React Native, NodeJS, PHP, Golang, Python, MySQL, PostgresQL, MongoDB, Redis, AWS, Firebase, and Supabase. He has a Bachelor's degree in Information System from Universitas Amikom Yogyakarta."
}
总结
按照这些说明,您可以使用 Python、Ollama 和 ChromaDB 根据您的需求有效地运行自定义本地 RAG 应用程序并与之交互。根据需要调整和扩展功能,以增强应用程序的功能。
通过利用本地部署的功能,您不仅可以保护敏感信息,还可以优化性能和响应能力。无论您是增强客户互动还是简化内部流程,本地部署的 RAG 应用程序都能提供灵活性和稳健性,以适应您的需求并随之增长。
检查此存储库中的源代码: