摘要
在日常生活中,发票的处理是一项常见的任务。然而,由于拍摄角度、光线等因素的影响,直接从照片中提取发票信息往往存在困难。本文将介绍如何使用OpenCV库进行图像处理和透视变换,从而有效地从发票图片中提取有用信息。我们将通过一个具体的例子,展示如何从一张发票图片中提取出清晰的发票内容。
1. 引言
发票处理是许多企业和个人经常面临的问题。传统的手动处理方式不仅耗时费力,而且容易出错。随着计算机视觉技术的发展,利用图像处理技术自动提取发票信息成为可能。本文将详细介绍如何使用OpenCV库进行图像处理和透视变换,以实现从发票图片中提取清晰的发票内容。
2. 环境准备
在开始之前,确保已经安装了以下库:
- OpenCV
- NumPy
可以使用以下命令安装这些库:
python
pip install opencv-python
pip install numpy
3. 代码实现
3.1 导入库
首先,导入所需的库:
python
import numpy as np
import cv2
3.2 辅助函数
定义一些辅助函数,用于显示图像、排序坐标点、进行透视变换和图像缩放。
3.2.1 显示图像
python
def cv_show(name, img):
cv2.imshow(name, img)
cv2.waitKey(0)
3.2.2 排序坐标点
python
def order_points(pts):
rect = np.zeros((4, 2), dtype="float32")
s = pts.sum(axis=1)
rect[0] = pts[np.argmin(s)]
rect[2] = pts[np.argmax(s)]
diff = np.diff(pts, axis=1)
rect[1] = pts[np.argmin(diff)]
rect[3] = pts[np.argmax(diff)]
return rect
3.2.3 透视变换
python
def four_point_transform(image, pts):
rect = order_points(pts)
(tl, tr, br, bl) = rect
widthA = np.sqrt(((br[0] - bl[0]) ** 2) + ((br[1] - bl[1]) ** 2))
widthB = np.sqrt(((tr[0] - tl[0]) ** 2) + ((tr[1] - tl[1]) ** 2))
maxWidth = max(int(widthA), int(widthB))
heightA = np.sqrt(((tr[0] - br[0]) ** 2) + ((tr[1] - br[1]) ** 2))
heightB = np.sqrt(((tl[0] - bl[0]) ** 2) + ((tl[1] - bl[1]) ** 2))
maxHeight = max(int(heightA), int(heightB))
dst = np.array([
[0, 0],
[maxWidth - 1, 0],
[maxWidth - 1, maxHeight - 1],
[0, maxHeight - 1]], dtype="float32")
M = cv2.getPerspectiveTransform(rect, dst)
warped = cv2.warpPerspective(image, M, (maxWidth, maxHeight))
return warped
3.2.4 图像缩放
python
def resize(image, width=None, height=None, inter=cv2.INTER_AREA):
dim = None
(h, w) = image.shape[:2]
if width is None and height is None:
return image
if width is None:
r = height / float(h)
dim = (int(w * r), height)
else:
r = width / float(w)
dim = (width, int(h * r))
resized = cv2.resize(image, dim, interpolation=inter)
return resized
3.3 主程序
3.3.1 读取和显示图像
python
image = cv2.imread('picture_video/fapiao.jpg')
cv_show('image', image)
3.3.2 图像缩放
python
ratio = image.shape[0] / 500.0
orig = image.copy()
image = resize(orig, height=500)
cv_show('1', image)
3.3.3 轮廓检测
python
print("STEP 1: 轮廓检测")
gray = cv2.cvtColor(image, cv2.COLOR_BGR2GRAY)
edged = cv2.threshold(gray, 0, 255, cv2.THRESH_BINARY | cv2.THRESH_OTSU)[1]
cnts = cv2.findContours(edged.copy(), cv2.RETR_LIST, cv2.CHAIN_APPROX_SIMPLE)[1]
image_contours = cv2.drawContours(image.copy(), cnts, -1, (0, 0, 255), 1)
cv_show('image_contours', image_contours)
3.3.4 获取最大轮廓
python
print("STEP 2: 获取最大轮廓")
screenCnt = sorted(cnts, key=cv2.contourArea, reverse=True)[0]
peri = cv2.arcLength(screenCnt, True)
screenCnt = cv2.approxPolyDP(screenCnt, 0.02 * peri, True)
image_contour = cv2.drawContours(image.copy(), [screenCnt], -1, (0, 255, 0), 2)
cv_show('image_contour', image_contour)
3.3.5 透视变换
python
warped = four_point_transform(orig, screenCnt.reshape(4, 2) * ratio)
cv2.imwrite('picture_video/invoice_new.jpg', warped)
cv2.namedWindow('xx', cv2.WINDOW_NORMAL)
cv2.imshow('xx', warped)
cv2.waitKey(0)
3.3.6 图像处理
python
warped = cv2.cvtColor(warped, cv2.COLOR_BGR2GRAY)
ref = cv2.threshold(warped, 0, 255, cv2.THRESH_BINARY | cv2.THRESH_OTSU)[1]
kernel = np.ones((2, 2), np.uint8)
ref_new = cv2.morphologyEx(ref, cv2.MORPH_CLOSE, kernel)
ref_new = resize(ref_new.copy(), width=500)
cv_show('yy', ref_new)
rotated_image = cv2.rotate(ref_new, cv2.ROTATE_90_COUNTERCLOCKWISE)
cv2.imshow("result", rotated_image)
cv2.waitKey(0)
4. 结果展示
通过上述步骤,我们成功地从发票图片中提取出了清晰的发票内容。以下是处理前后的对比图:
- 处理前:
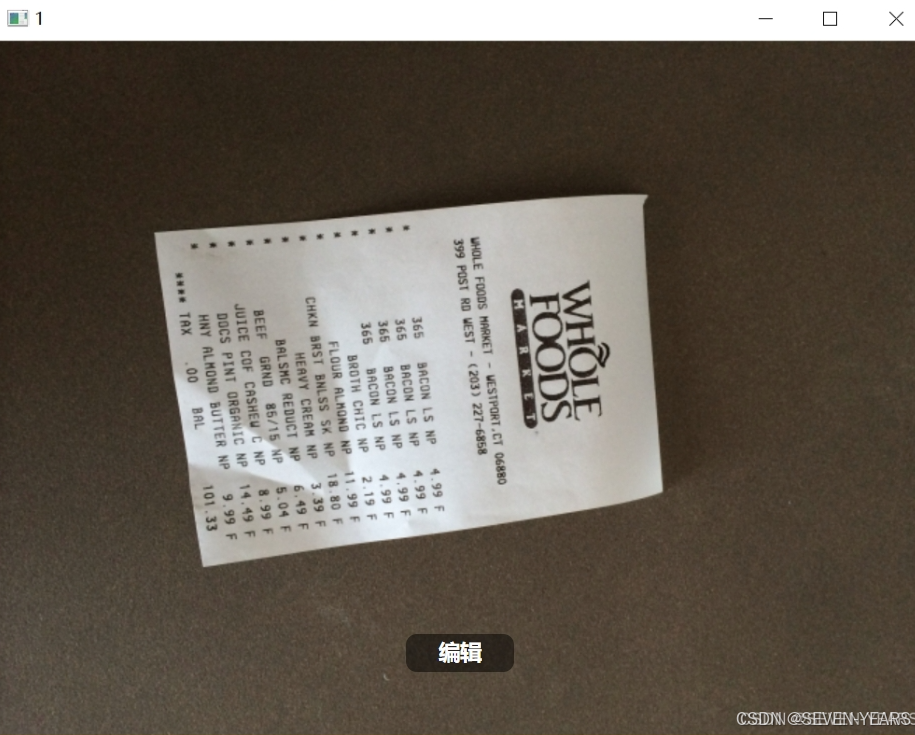
-
处理后:
-
5. 结论
本文详细介绍了如何使用OpenCV库进行图像处理和透视变换,以从发票图片中提取清晰的信息。通过轮廓检测、透视变换和图像处理等步骤,我们可以有效地解决因拍摄角度、光线等因素导致的图像质量问题。希望本文能为读者在图像处理领域的研究和开发提供有益的参考。