Title
题目
MedLSAM: Localize and segment anything model for 3D CT images
MedLSAM: 用于3D CT图像的局部化和分割模型
01
文献速递介绍
最近,计算机视觉领域对开发大规模的基础模型的兴趣不断增加,这些模型能够同时处理多个视觉任务,例如图像分类、目标检测和图像分割。例如,CLIP(Radford等,2021)通过对来自网络的大量图像和文本对进行对齐,可以利用文本提示识别新的视觉类别。同样,GLIP(Li等,2022;Zhang等,2022)通过将目标检测与短语嵌入预训练集成,利用来自网络的图像--文本对,具有强大的零-shot和few-shot转移能力,特别是在目标检测任务中。在分割方面,Segment Anything Model (SAM)(Kirillov等,2023)最近在广泛的分割任务中展现了显著的能力,通过适当的提示,例如点、边界框(bbox)和文本。
随着基础模型在一般计算机视觉任务中的能力逐渐展现,医学影像领域,尤其是图像数据有限且标注成本高昂的特点,引起了对这些模型潜力的关注。医学影像中的这些挑战凸显了基础模型的迫切需求,吸引了更多研究者的关注(Zhang和Metaxas,2023)。一些研究致力于设计与数据集特征相匹配的自监督学习任务,通过大量未标注数据进行预训练,然后在特定的下游任务上进行微调,取得了可喜的成果(Wang等,2023;Zhou等,2023;Vorontsov等,2023)。相比之下,其他研究则专注于使用大规模标注数据集进行预训练,或者为了在新任务上进行微调(Huang等,2023b),或者直接实现分割而无需进一步训练或微调(Butoi等,2023)。总体而言,这些模型提供了许多优势:简化了开发过程,减少了对大量标注数据集的依赖,并增强了患者数据隐私。作为医学图像分析的关键组成部分,医学图像定位包括关键点检测(Chen等,2021;Wan等,2023)、器官定位(Xu等,2019;Navarro等,2020;Hussain等,2021;Navarro等,2022)和疾病检测(Yan等,2018;Zhang等,2018)。然而,据我们所知,专门为医学图像定位任务设计的基础模型仍然非常有限。在2D医学图像定位领域,MIU-VL(Qin等,2022)作为一种开创性努力脱颖而出。这种方法巧妙地将预训练的视觉语言模型与医学文本提示结合起来,以促进医学图像中的目标检测。然而,将这些预训练模型应用于专业的医学影像领域,尤其是像CT扫描这样的3D模式,仍然具有挑战性。这一限制归因于显著的领域差距,因为这些模型最初是在2D自然图像上进行训练的。同时,在医学图像分割领域,像SAM及其医学适配(Wu等,2023;Cheng等,2023;He等,2023b;Huang等,2023a;He等,2023a;Zhang和Liu,2023;Mazurowski等,2023;Zhang和Jiao,2023)等模型已展示出显著的潜力,甚至在某些任务中达到了与完全监督分割模型相当的性能(Ma等,2024)。尽管有这些进展,这些模型仍然需要手动提示,例如标注的点或边界框。然而,一次3D扫描通常包含几十甚至上百张切片。当每张切片包含多个目标结构时,对整个3D扫描的标注需求呈指数增长(Wang等,2018;Lei等,2019)。为了消除这种庞大的标注需求并促进SAM及其医学变种在3D数据上的应用,一个有效的方法是自动生成适合的提示来标注数据。虽然可以通过训练一个专门的检测器来实现这一点,但这会增加标注数据以训练检测器的额外负担,并且可检测的类别仍然是固定的(Baumgartner等,2021)。更优的解决方案是开发一个3D医学图像定位基础模型,仅需最少的用户输入来指定感兴趣的类别,从而能够在任何数据集中直接定位所需的目标,而无需进一步训练。这一挑战突显了3D医学图像定位基础模型的迫切需求。为了解决3D医学图像定位中缺乏基础模型的问题,我们提出了MedLAM(Localize Anything Model for 3D Medical Images)。MedLAM是一个专门的3D定位基础模型,旨在使用最少的提示准确定位任何解剖结构。此外,我们将MedLAM与SAM模型结合,创建了MedLSAM,一个框架,显著减少了SAM在3D医学图像中的人工交互需求,同时展示了实现竞争性能的潜力。MedLSAM采用两阶段方法。第一阶段使用MedLAM自动识别体积医学图像中目标结构的位置。在随后的阶段,SAM模型使用第一阶段得到的边界框(bboxes)实现精确的图像分割。最终结果是一个完全自动化的管道,最小化了人工干预的需求。
MedLAM定位基础模型是我们会议版(Lei等,2021b)的扩展,基于这样的观察:不同个体之间的器官空间分布保持较强的相似性。我们假设存在一个标准的解剖坐标系统,在该系统中,不同人群中相同的解剖部分具有相似的坐标。因此,我们可以通过在未标注的扫描中找到相似的坐标来定位目标解剖结构。在我们之前的版本中,模型分别针对不同的解剖结构进行训练,每个结构仅使用少量几十张扫描图像。相比之下,本研究显著扩展了数据集,包含来自16个不同数据集的14,012个CT扫描。这使我们能够训练一个统一的综合模型,能够定位全身的任何结构。训练过程涉及一个投影网络,用于预测同一图像中任何两个补丁之间的3D物理偏移,从而将扫描的每个部分映射到共享的3D潜在坐标系统中。在分割阶段,我们采用原始的SAM和成熟的MedSAM(Ma等,2024)作为分割过程的基础。MedSAM之前已在全面的医学图像数据集上进行了微调,在2D和3D医学图像分割任务中展现了显著的性能。使用这样的强大模型增强了我们提议管道的可靠性和有效性。
我们的贡献总结如下:
- 我们提出了MedLAM,这是第一个3D医学图像定位基础模型。该模型是我们之前会议版(Lei等,2021b)的扩展,结合了像素级自监督任务,并在包含14,012个CT扫描的全面数据集上进行了训练,覆盖了全身。因此,它能够直接定位人体内的任何解剖结构,并且在大多数情况下的性能可与完全监督的定位模型媲美; 我们提出了MedLSAM,这是第一个完全自动化的医学适配SAM模型。它不仅最小化了人工干预的需求,而且推动了医学图像分析中完全自动化基础分割模型的发展。据我们所知,这是第一个通过集成SAM模型实现医学图像分割的完全自动化的工作;我们在两个涵盖38个器官的3D数据集上验证了MedLAM和MedLSAM的有效性。如表2和表3所示,我们的定位基础模型MedLAM展现了不仅显著优于现有医学定位基础模型的定位准确性,而且与完全监督模型的性能相当。同时,如表4所示,MedLSAM与SAM及其医学适配模型的性能相符,大大减少了对人工标注的依赖。为了进一步推进医学影像领域基础模型的研究,我们已公开了所有模型和代码。
Aastract
摘要
Recent advancements in foundation models have shown significant potential in medical image analysis.However, there is still a gap in models specifically designed for medical image localization. To address this,we introduce MedLAM, a 3D medical foundation localization model that accurately identifies any anatomicalpart within the body using only a few template scans. MedLAM employs two self-supervision tasks: unifiedanatomical mapping (UAM) and multi-scale similarity (MSS) across a comprehensive dataset of 14,012 CTscans. Furthermore, we developed MedLSAM by integrating MedLAM with the Segment Anything Model(SAM). This innovative framework requires extreme point annotations across three directions on severaltemplates to enable MedLAM to locate the target anatomical structure in the image, with SAM performingthe segmentation. It significantly reduces the amount of manual annotation required by SAM in 3D medicalimaging scenarios. We conducted extensive experiments on two 3D datasets covering 38 distinct organs. Ourfindings are twofold: (1) MedLAM can directly localize anatomical structures using just a few template scans,achieving performance comparable to fully supervised models; (2) MedLSAM closely matches the performanceof SAM and its specialized medical adaptations with manual prompts, while minimizing the need for extensivepoint annotations across the entire dataset. Moreover, MedLAM has the potential to be seamlessly integratedwith future 3D SAM models, paving the way for enhanced segmentation performance.
基础模型的最新进展在医学图像分析中显示了巨大的潜力。然而,专门为医学图像定位设计的模型仍然存在一定的空白。为了解决这一问题,我们提出了MedLAM,一个3D医学基础定位模型,能够仅使用少量模板扫描准确识别体内任何解剖部位。MedLAM采用了两种自监督任务:统一解剖映射(UAM)和多尺度相似性(MSS),并在14,012个CT扫描的全面数据集上进行了训练。此外,我们通过将MedLAM与Segment Anything Model(SAM)结合,开发了MedLSAM。这个创新框架要求在多个模板上进行三个方向的极端点标注,以使MedLAM能够定位图像中的目标解剖结构,随后由SAM进行分割。它显著减少了在3D医学影像场景中SAM所需的手动标注量。我们在两个包含38个不同器官的3D数据集上进行了广泛实验。我们的研究结果有两个方面:(1) MedLAM仅使用少量模板扫描即可直接定位解剖结构,其性能可与完全监督模型相媲美;(2) MedLSAM的性能与SAM及其针对医学领域的专门适配在手动提示下的表现相当,同时大幅度减少了整个数据集上对点标注的需求。此外,MedLAM有潜力与未来的3D SAM模型无缝集成,推动分割性能的提升。
Method
方法
As illustrated in Fig. 5, MedLSAM comprises two core components:the automated localization algorithm MedLAM and the automatedsegmentation algorithm SAM. In the subsequent sections, we first delveinto the architecture and training of MedLAM. Following that, we detailhow MedLAM synergizes with SAM to realize the complete autonomoussegmentation framework.
如图5所示,MedLSAM由两个核心组件组成:自动化定位算法MedLAM和自动化分割算法SAM。在接下来的部分中,我们首先深入探讨MedLAM的架构和训练过程。随后,我们将详细介绍MedLAM如何与SAM协同工作,以实现完整的自动化分割框架。
Conclusion
结论
In this study, we introduced MedLAM, a foundational localization model for medical images capable of identifying any anatomicalstructure within 3D medical images. By integrating it with the SAMmodel, we developed MedLSAM, an automated segmentation framework designed to reduce the annotation workload in medical imagesegmentation.We validated MedLAM and MedLSAM across two 3D datasets, covering 38 different organs. The results demonstrate that MedLAM, usingonly a few support samples, can achieve performance comparable toor better than existing fully supervised models. MedLAM's ability tolocalize anatomical structures efficiently and accurately underscores itspotential for broader applications in medical imaging, including rapidand automatic localization of user-specified landmarks or regions of interest. This makes it particularly suited for scenarios requiring flexibleand scalable localization without the need for extensive fine-tuning.While MedLSAM shows promise in reducing annotation workloads,it still does not match the performance of fully supervised modelssuch as nnU-Net, especially in cases involving organs with significant anatomical abnormalities. This highlights the need for furtherrefinement of prompt-based segmentation methods.Future work should focus on enhancing MedLAM's versatility andintegrating it with emerging generalist medical AI (GMAI) (Moor et al., applications. Doing so could further improve its generalizabilityand close the performance gap with fully supervised models, while alsoreducing the resources and time required for medical imaging analysis. MedLAM's strong performance in anatomical structure localizationsuggests potential for real-time, interactive medical applications, particularly in segmenting and analyzing specific anatomical regions thatmay involve novel combinations of categories not yet covered by publicdatasets.
在本研究中,我们介绍了MedLAM,一种基础的医学图像定位模型,能够识别3D医学图像中的任何解剖结构。通过将其与SAM模型集成,我们开发了MedLSAM,这是一种自动化分割框架,旨在减少医学图像分割中的标注工作量。我们在两个3D数据集上验证了MedLAM和MedLSAM,涵盖了38个不同的器官。结果表明,MedLAM只使用少量支持样本,就能够达到与现有完全监督模型相当或更好的性能。MedLAM高效且准确地定位解剖结构,突显了其在医学影像中的广泛应用潜力,包括快速和自动化的用户指定标记点或感兴趣区域的定位。这使得它特别适用于需要灵活且可扩展定位的场景,而不需要大量的精调。尽管MedLSAM在减少标注工作量方面显示出潜力,但在性能上仍不及完全监督模型(如nnU-Net),尤其是在涉及具有显著解剖异常的器官时。这突出了基于提示的分割方法进一步完善的必要性。
未来的工作应重点提升MedLAM的多功能性,并将其与新兴的通用医学AI(GMAI)应用(Moor等,2023)集成。这样可以进一步提高其通用性,并缩小与完全监督模型的性能差距,同时减少医学影像分析所需的资源和时间。MedLAM在解剖结构定位中的强大性能表明其在实时互动医学应用中的潜力,特别是在分割和分析可能涉及尚未在公共数据集中涵盖的类别新组合的特定解剖区域时。
Figure
图
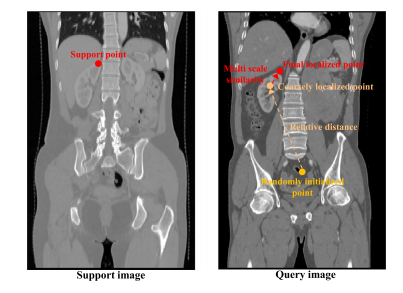
Fig. 1. Structure of the inference stage in the Medical Localization Anything Model(MedLAM). The process involves moving an agent from a randomly initialized positiontoward the target landmark, guided by 3D relative distance and multi-scale featurevectors
图1. 医学定位任何模型(MedLAM)推理阶段的结构。该过程涉及将代理从随机初始化的位置移动到目标标志点,过程中通过3D相对距离和多尺度特征向量进行引导。
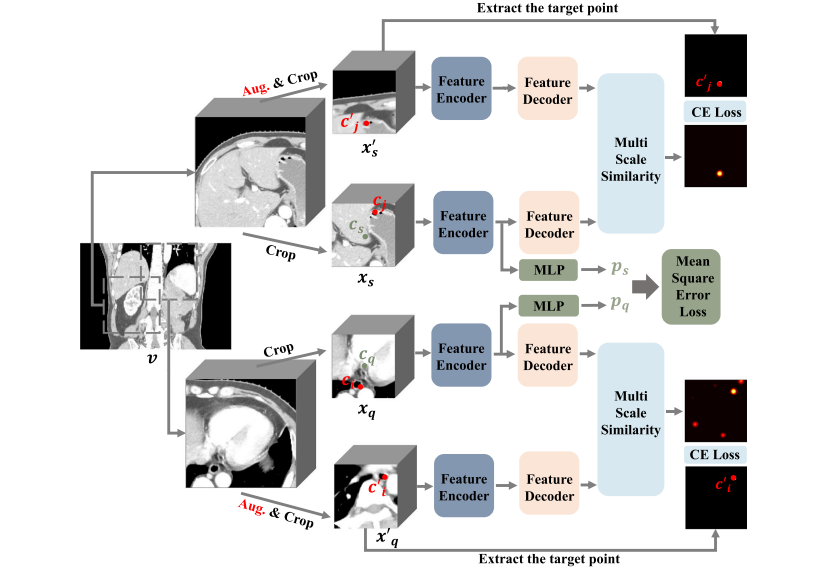
Fig. 2. The learning process of MedLAM. We first randomly extract two large image patches from the scan. Then we randomly crop a small image patch from each of the twolarge image patches and their augmented versions to produce two pairs of patches, namely, the original patch pair 𝒙𝑞 , 𝒙𝑠 and the augmented patch pair 𝒙 ′ 𝒒 , 𝒙 ′ 𝒔 . All these patches arepassed through the MedLAM and the training objectives contain twofold: (1) Unified Anatomical Mapping (UAM): By predicting the relative distance between the original imagepatches 𝒙𝑞 and 𝒙𝑠 , MedLAM project images from different individuals onto a shared anatomical coordinate space. (2) Multi-Scale Similarity (MSS): Ensure that the similaritybetween features corresponding to the same region is maximized in both the original image patches 𝒙𝑞 , 𝒙𝑠 and their augmented counterparts 𝒙 ′ 𝒒 , 𝒙 ′ 𝒔 .
图2. MedLAM的学习过程。我们首先从扫描中随机提取两个较大的图像补丁。然后,从这两个大的图像补丁及其增强版本中随机裁剪出一个小的图像补丁,形成两个补丁对,即原始补丁对 𝒙𝑞 和 𝒙𝑠 以及增强后的补丁对 𝒙 ′ 𝒒 和 𝒙 ′ 𝒔。所有这些补丁都通过MedLAM,训练目标包括两个方面:(1)统一解剖学映射(UAM):通过预测原始图像补丁 𝒙𝑞 和 𝒙𝑠 之间的相对距离,MedLAM将来自不同个体的图像映射到一个共享的解剖学坐标空间。(2)多尺度相似性(MSS):确保同一区域对应的特征在原始图像补丁 𝒙𝑞 和 𝒙𝑠 以及它们的增强版本 𝒙 ′ 𝒒 和 𝒙 ′ 𝒔 中的相似性最大化。
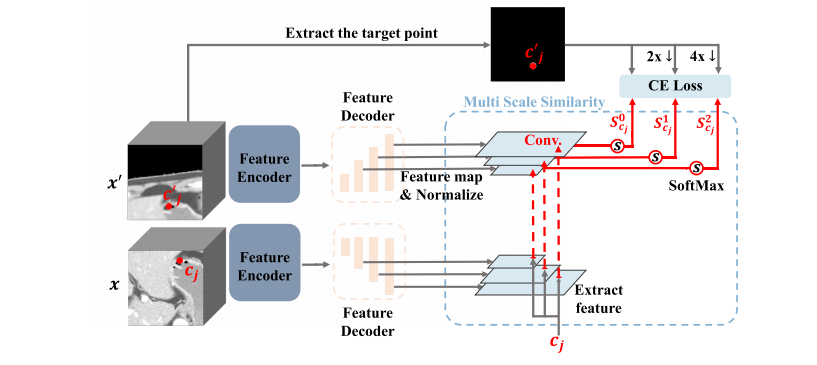
Fig. 3. Details of the Multi Scale Similarity (MSS). The origin small patch 𝒙 and augmented patch 𝒙 ′ are all passed through the feature encoder and feature decoder to obtain thenormalized multi-scale feature maps. Then we extract the multi-scale feature vectors of point 𝒄𝒋 to compute the similarity with 𝒙 ′ corresponding feature maps, applying the softmaxoperation across all values in each similarity map to achieve the final probability maps set {𝑺 𝟎 𝒄𝒋,𝑺 𝟏 𝒄𝒋,𝑺 𝟐 𝒄𝒋}. Finally, the probability maps are restricted with the Cross-Entropy loss
图3. 多尺度相似性(MSS)的详细信息。原始小补丁 𝒙 和增强补丁 𝒙 ′ 都通过特征编码器和特征解码器,得到归一化的多尺度特征图。然后,我们提取点 𝒄𝒋 的多尺度特征向量,计算其与 𝒙 ′ 对应特征图的相似性,应用softmax操作对每个相似性图中的所有值进行归一化,得到最终的概率图集 {𝑺 𝟎 𝒄𝒋, 𝑺 𝟏 𝒄𝒋, 𝑺 𝟐 𝒄𝒋}。最后,使用交叉熵损失函数对概率图进行约束。
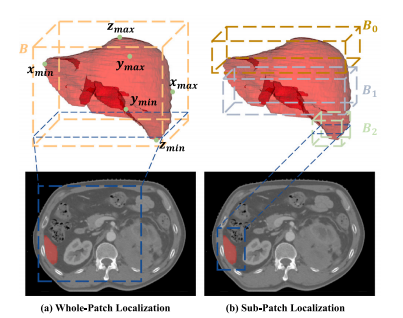
Fig. 4. Comparison between Whole-Patch Localization (WPL) and Sub-Patch Localization (SPL) strategies. (a) WPL generates a single minimal 3D bounding box (𝑩) byidentifying the six extreme points of the target anatomical structure. (b) SPL dividesthe target structure into multiple segments, each with its own localized 3D boundingbox (𝑩𝑖 ), resulting in a more accurate representation at each slice.
图4. 整块补丁定位(WPL)与子块补丁定位(SPL)策略的比较。(a) WPL通过识别目标解剖结构的六个极端点生成一个最小的3D边界框(𝑩)。(b) SPL将目标结构划分为多个部分,每个部分都有自己局部化的3D边界框(𝑩𝑖),从而在每一切片上实现更精确的表示。
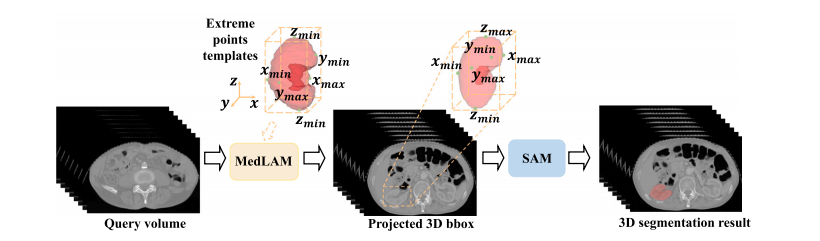
Fig. 5. The overall segmentation pipeline of MedLSAM operates as follows. Given a dataset of any size, MedLSAM first applies MedLAM to identify the six extreme points (inthe z, x, and 𝑦 directions) of any anatomical structure of interest. This process results in the generation of a 3D bbox encompassing the targeted organ or structure. Subsequently,for each slice within this 3D bbox, a 2D bbox is derived, representing the projection of the 3D bbox onto that specific slice. These 2D bboxes are then utilized by the SegmentAnything Model (SAM) to carry out precise segmentation of the target anatomy, thereby automating the entire segmentation process.
图5. MedLSAM的整体分割流程如下所示。给定任何大小的数据集,MedLSAM首先应用MedLAM来识别目标解剖结构的六个极端点(在z、x和𝑦方向上)。这个过程生成一个包含目标器官或结构的3D边界框。随后,对于这个3D边界框内的每个切片,推导出一个2D边界框,表示3D边界框在该切片上的投影。然后,这些2D边界框被Segment Anything Model(SAM)用于精确分割目标解剖结构,从而实现整个分割过程的自动化。
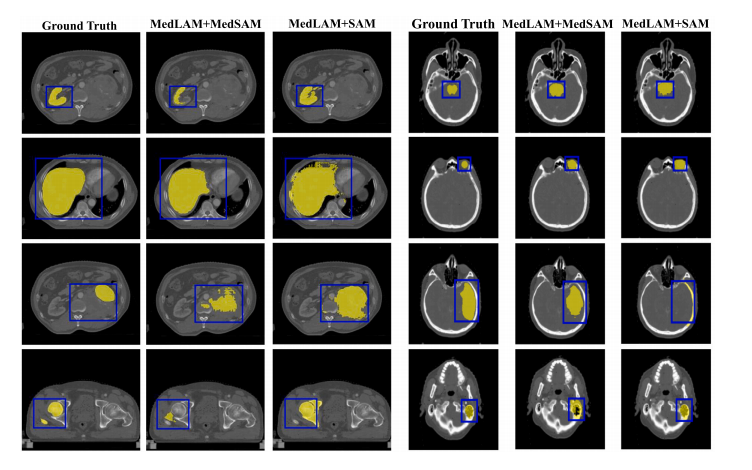
Fig. 6. Visualization examples of segmentation results on WORD and StructSeg Head-and-Neck datasets using pre-trained MedSAM and SAM, post landmark localization withMedLAM
图6 在WORD和StructSeg头颈部数据集上,使用预训练的MedSAM和SAM进行分割结果的可视化示例,经过MedLAM进行地标定位后。
Table
表
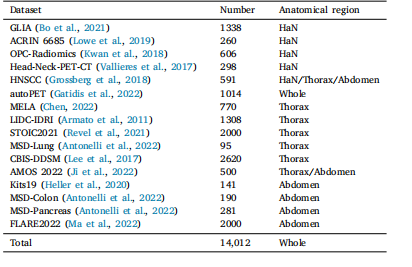
Table 1Detailed information of the 16 CT datasets for MedLAM training.
表1 MedLAM训练所用的16个CT数据集的详细信息。
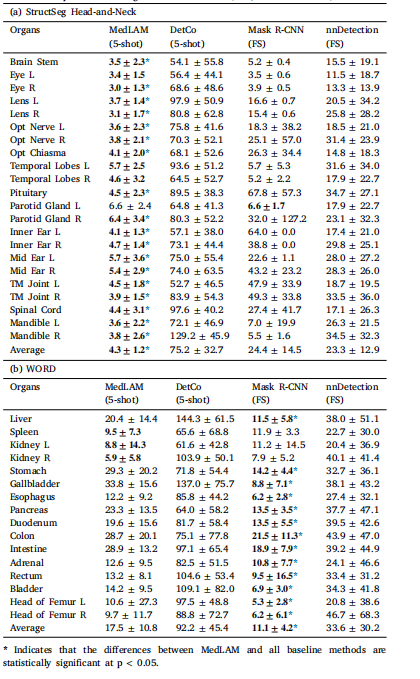
Table 2Comparison of MedLAM with few-shot and fully supervised (FS) localization models onthe landmark localization task using the StructSeg Head-and-Neck and WORD datasets.Results are reported in Average Localization Error (ALE, mean ± std mm).
表2MedLAM与少量样本(few-shot)和全监督(FS)定位模型在使用StructSeg头颈部和WORD数据集进行标志点定位任务中的比较。结果以平均定位误差(ALE,均值 ± 标准差,单位:毫米)报告。
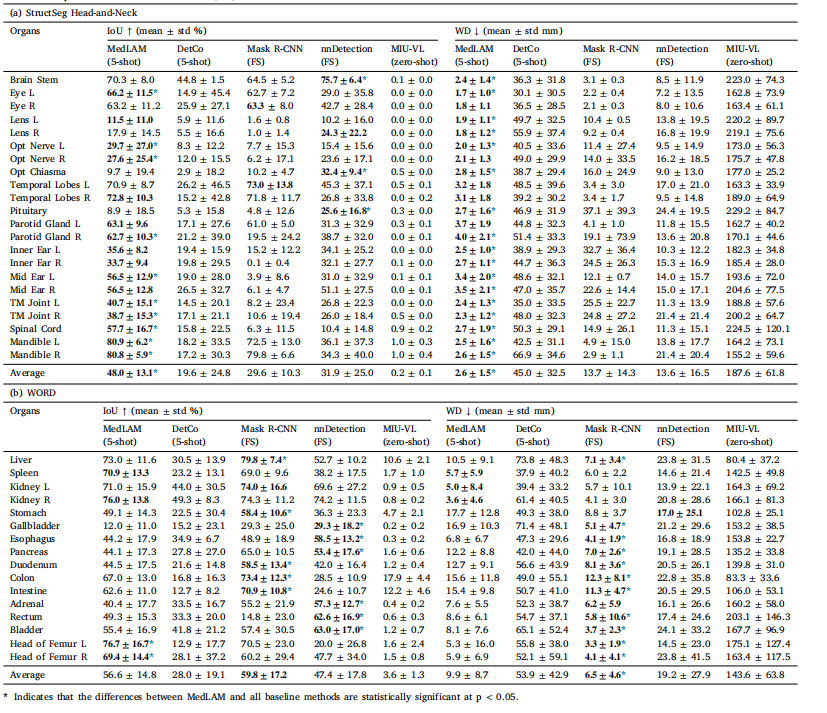
Table 3Comparison of MedLAM with few-shot, fully supervised (FS), and zero-shot localization models on the organ detection task using the StructSeg Head-and-Neck and WORD datasets.Results are reported in IoU and Wall Distance (WD).
表3MedLAM与少量样本(few-shot)、全监督(FS)和零样本(zero-shot)定位模型在使用StructSeg头颈部和WORD数据集进行器官检测任务中的比较。结果以交并比(IoU)和墙距离(WD)报告。
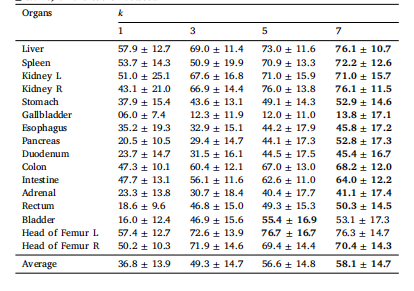
Table 4Impact of the support volume size 𝑘 of MedLAM on organ detection: IoU score ↑ (mean± std %) on the WORD dataset
表4 MedLAM支持体积大小 𝑘 对器官检测的影响:在WORD数据集上,IoU得分 ↑(均值 ± 标准差,单位:%)。
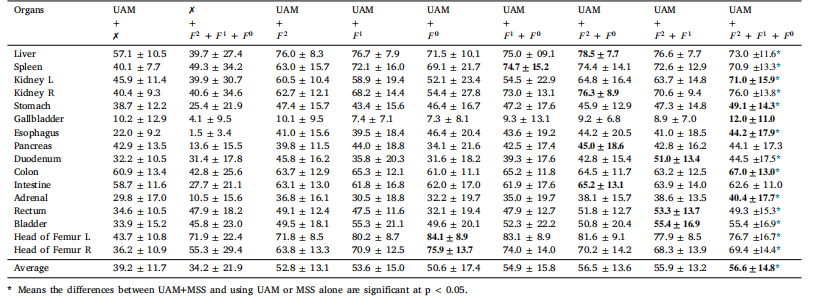
Table 5Ablation study of Unified Anatomical Mapping (UAM) and Multi Scale Similarity (MSS) in MedLAM for organ detection: IoU score ↑ (mean ± std %) on the WORD dataset.
表5 MedLAM中统一解剖映射(UAM)和多尺度相似性(MSS)对器官检测的消融研究:在WORD数据集上,IoU得分 ↑(均值 ± 标准差,单位:%)。
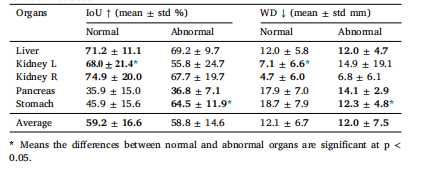
Table 6Performance of MedLAM in localizing normal and abnormal organs in the FLARE2023 validation dataset.
表6MedLAM在FLARE2023验证数据集中定位正常和异常器官的表现。
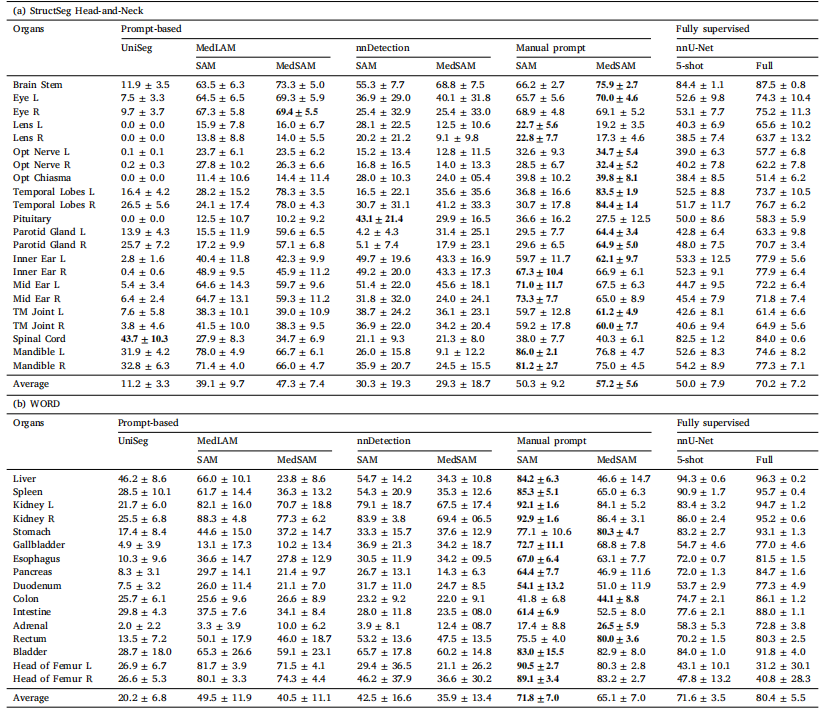
Table 7Comparison of segmentation performance between prompt-based and fully-supervised segmentation methods on the StructSeg Head-and-Neck and the WORD datasets using DSCscores ↑ (mean ± std %). In the table, '5-shot' indicates that nnU-Net was trained using the same five support sets as used for MedLAM and UniverSeg, while 'full' refers toconducting five-fold cross-validation using the entire dataset
表7 基于提示和全监督分割方法在使用DSC得分 ↑(均值 ± 标准差,单位:%)的StructSeg头颈部和WORD数据集上的分割性能比较。表中,"5-shot"表示使用与MedLAM和UniverSeg相同的五个支持集训练nnU-Net,而"full"则指使用整个数据集进行五折交叉验证。
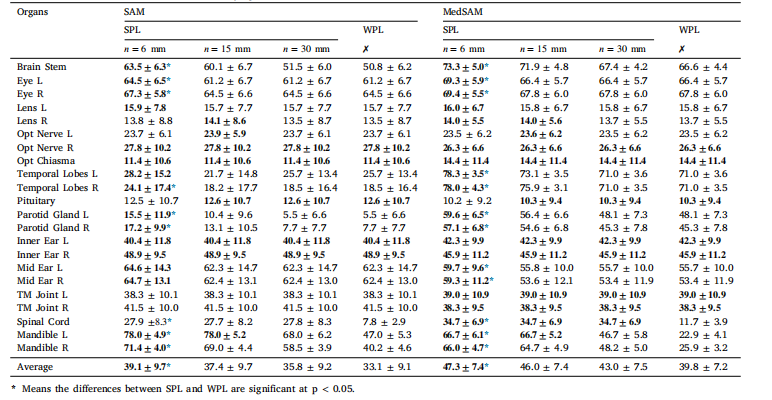
Table 8Comparison of DSC scores ↑ (mean ± std%) for MedLSAM using different localization strategies on the StructSeg Head-and-Neck dataset: Whole-Patch Localization(WPL) and Sub-Patch Localization (SPL) with varying slice intervals ?
表8 MedLSAM在使用不同定位策略下,在StructSeg头颈部数据集上的DSC得分 ↑(均值 ± 标准差,单位:%)比较:全 Patch 定位(WPL)与子 Patch 定位(SPL)在不同切片间隔 ? 下的表现。
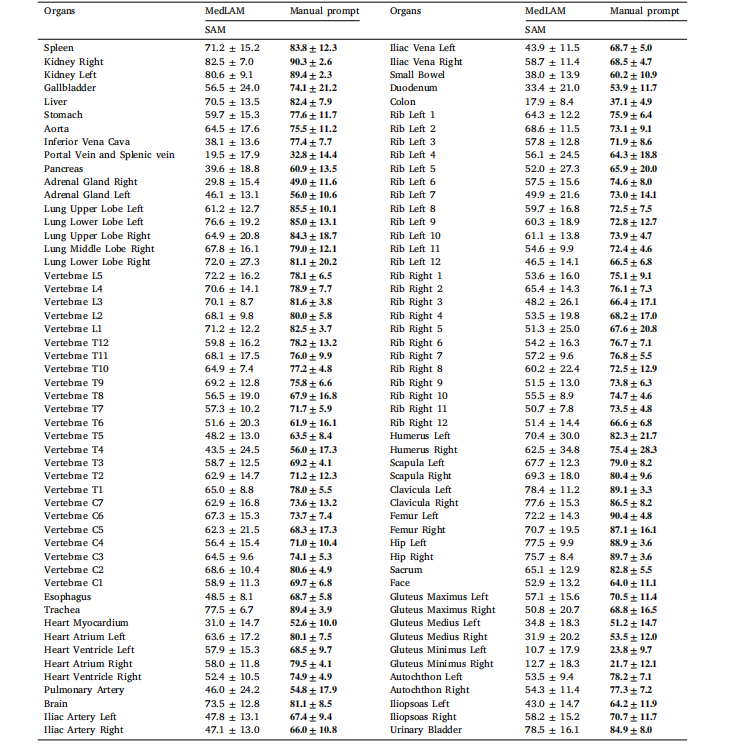
Table 9Comparison of DSC scores ↑ (mean ± std%) between MedLAM-generated bounding box prompts and manually annotated prompts on theTotalsegmentator dataset.
表9 MedLAM生成的边界框提示与手动标注提示在Totalsegmentator数据集上的DSC得分 ↑(均值 ± 标准差,单位:%)比较。