HGCN结构
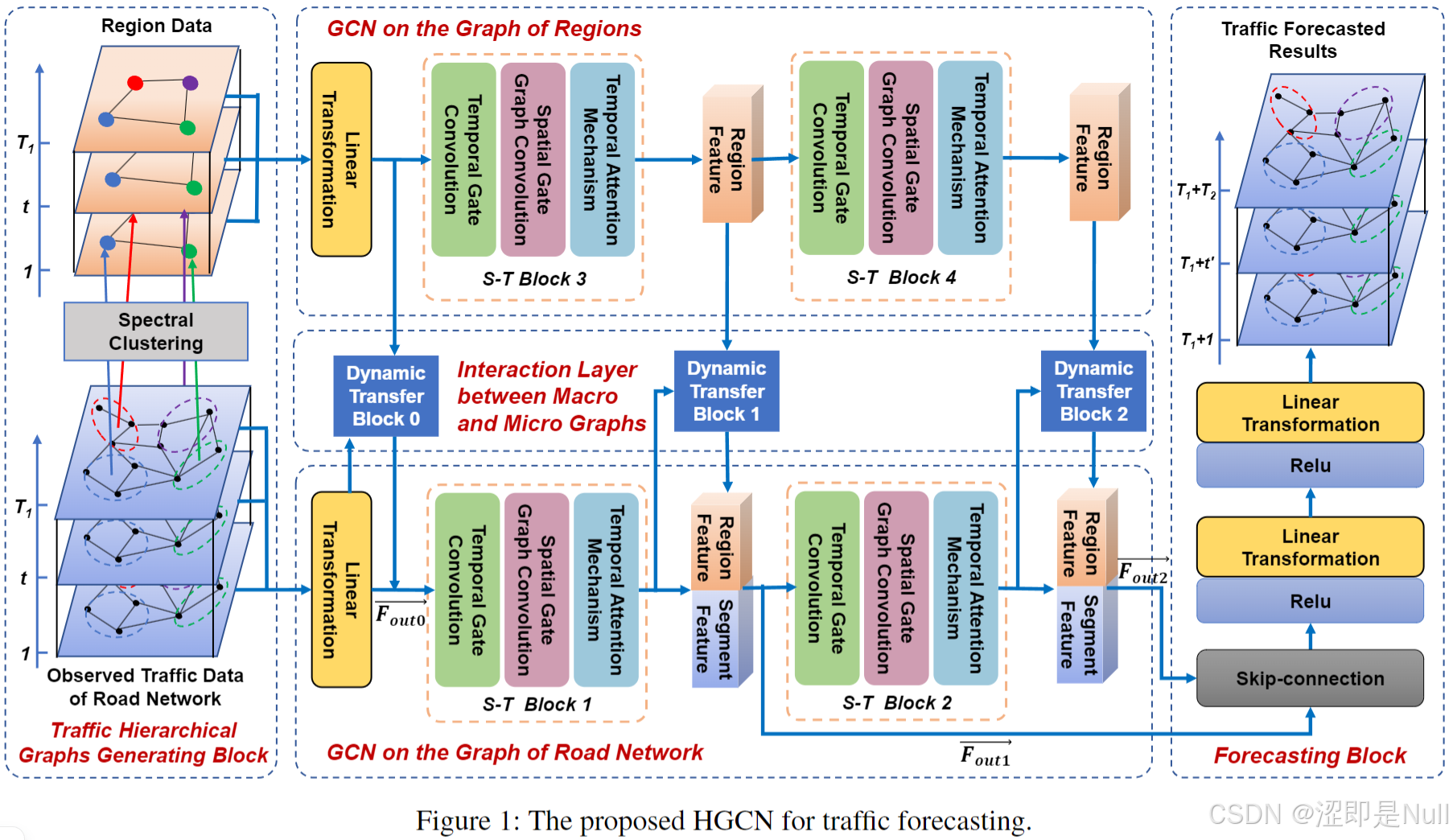
本篇论文解决交通预测问题共分为五个部分,分别是谱聚类(Spectral Clustering)、区域图山的GCN(GCN on the Graph of Regions)、道路网络图山的GCN(GCN on the Graph of Road Network)、宏观图和微观图之间的交互层(Interaction Layer between Macro and Micro Graphs)、交通预测块(Traffic Forecasting Block)。
1 生成交通层次图(Spectral Clustering)
1.1 构建微观道路网络图
所谓 "微观" ,就是道路图上节点的交通数据(如速度、流量或密度)。
1.2 构建宏观区域图
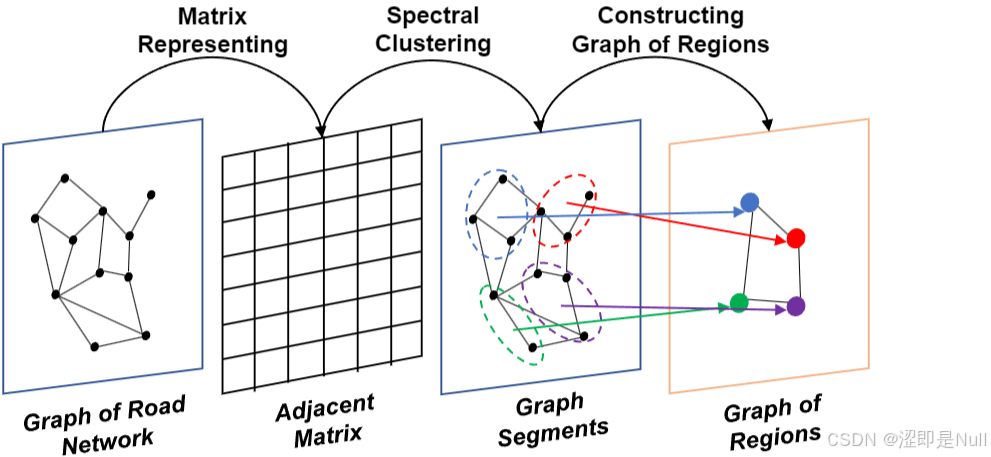
所谓 "宏观区域" ,就是指把之前的微观节点聚类为一个区域。本文采用谱聚类方法,对道路网络图进行处理,从而构建区域的宏观图。如上图所示,一共分为4步:
- 获取邻接矩阵:首先从道路网络图中获得邻接矩阵。
- 谱聚类:对邻接矩阵的拉普拉斯矩阵进行谱聚类,将整个道路网络划分为若干簇。每个簇即视作区域图中的一个宏观节点。
- 区域特征计算:每个宏观节点(4个小点儿)的特征由该簇内所有微观节点特征的均值和最小值组合而成。
- 构造宏观图边:基于微观图构造宏观图。
2 区域图上的GCN(GCN on the Graph of Regions)
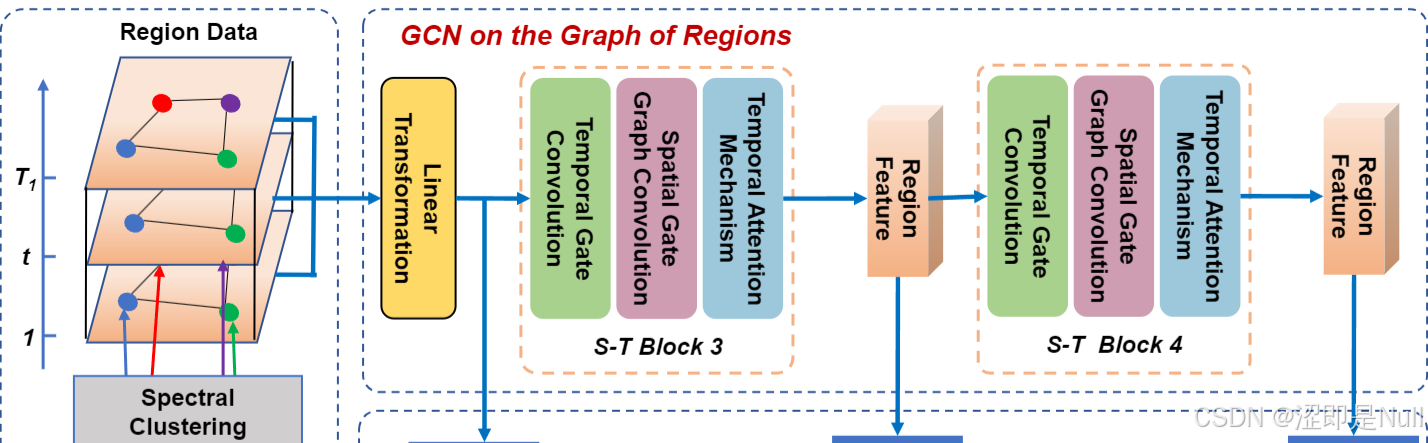
针对第一步中产生的基于区域的宏观图(Region Data),先来一个线性变换(Linear Transformation)对其进行初步转换。 其主要目的是将输入数据投影到一个新的特征空间,以便后续的图卷积操作能够更有效地学习数据表示。
python
# 线性变换:将 in_dim(1) 维度转换为 residual_channels(32) 维度
self.start_conv_cluster = nn.Conv2d(in_channels=in_dim_cluster,
out_channels=residual_channels,
kernel_size=(1, 1))
input_c = input_cluster
x_cluster = self.bn_cluster(input_c)
x_cluster = self.start_conv_cluster(x_cluster) # 关于区域图的特征张量
接下来,是连续的两次时空图卷积(S-T Block)。在第一个时空卷积块(S-T Block)中,采用一个较小的卷积核,感受野增长很慢,模型主要处理一些最近的数据。比如:突发事件、红绿灯导致的交通波动、局部区域的流量变化。
在第二个时空卷积块中,它的输入已经包含了过去几个时间步的汇总信息,因此可以处理处理更长时间范围的数据。比如:上下班高峰、天气影响、节假日模式。
python
# 计算区域图的邻接矩阵 new_supports_cluster
A_cluster = F.relu(torch.mm(self.nodevec1_c, self.nodevec2_c))
d_c = 1 / (torch.sum(A_cluster, -1))
D_c = torch.diag_embed(d_c)
A_cluster = torch.matmul(D_c, A_cluster)
new_supports_cluster = self.supports_cluster + [A_cluster]
# 定义卷积块
self.block_cluster1 = GCNPool(dilation_channels, dilation_channels, cluster_nodes, length - 6, 3, dropout,
cluster_nodes,
self.supports_len)
# 卷积
x_cluster = self.block_cluster1(x_cluster, new_supports_cluster)
3 道路网络图上的 GCN(GCN on the Graph of Road Network)
道路网络图上的 GCN 和区域图上的 GCN 算法基本相同,只不过输入数据从区域图变成了道路节点图,也就是最传统的卷积方式。
4 宏观图和微观图之间的交互层(Interaction Layer between Macro and Micro Graphs)
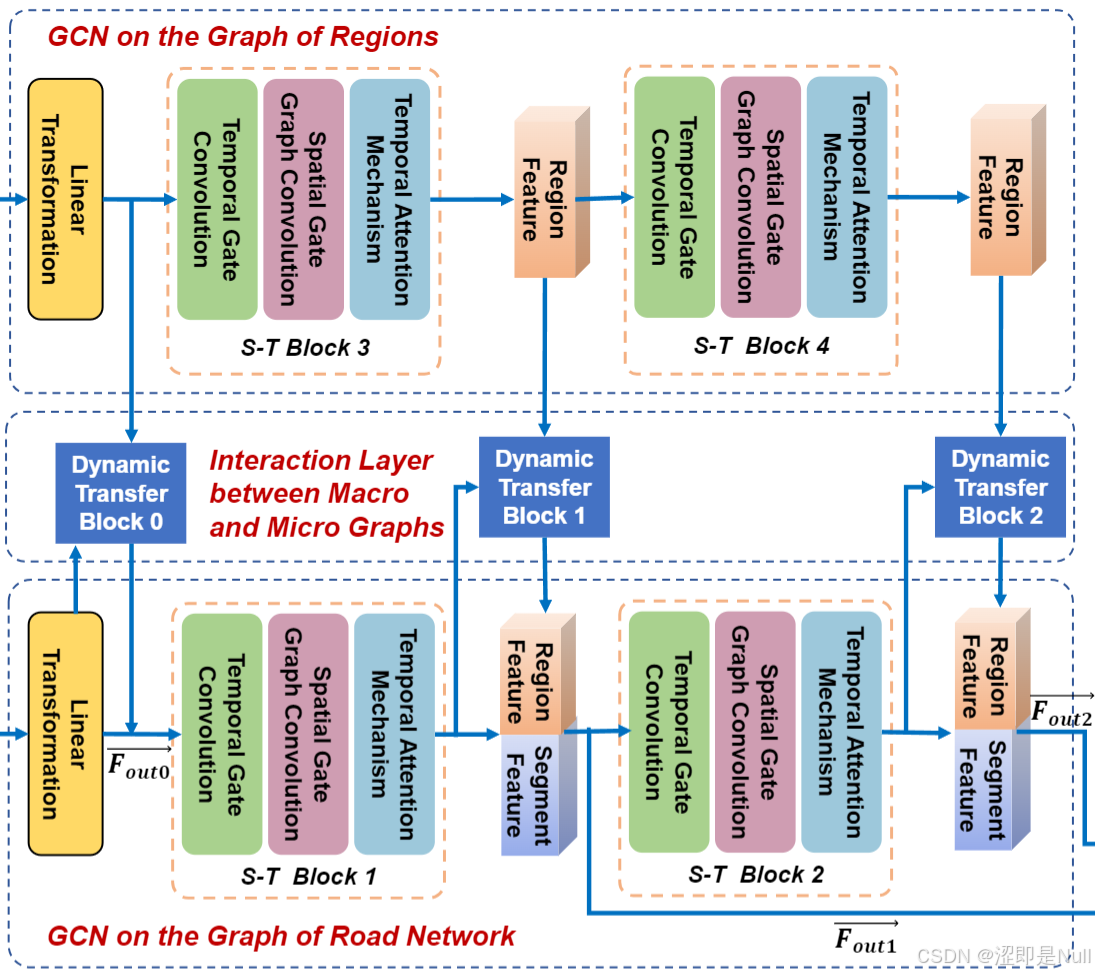
如上图所示, 在区域图和道路网之间存在一个动态传递模块(Dynamic Transfer Block),用于融合区域特征与道路段特征。
首先,构造一个传递函数 Tran 。对于道路节点 i 与区域 j 的对应关系,如果节点 i 属于区域 j,则将区域 j 的特征复制并与道路段 i 的特征级联。

因为交通数据具有动态变化的特性,道路段与区域之间的关系也应随之变化。因此作者采用了注意力机制优化传递函数。其中 为注意力分数,减去均值有助于消除不同特征维度间数值分布的偏差,使后续计算更稳定。
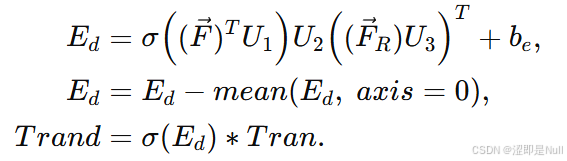
python
# 道路网络特征
c1 = seq
f1 = self.conv1(c1).squeeze(1)#b,n,l
# 区域特征
c2 = seq_cluster.permute(0,3,1,2)#b,c,n,l->b,l,n,c
f2 = self.conv2(c2).squeeze(1)#b,c,n
logits=torch.sigmoid(torch.matmul(torch.matmul(f1,self.w),f2)+self.b)
a = torch.mean(logits, 1, True)
logits = logits - a
logits = torch.sigmoid(logits)
coefs = (logits)*self.transmit
最后,按照上述方法进行特征传递。设道路特征为 ,区域特征为
。

python
x = self.start_conv(x) # 道路网特征张量
x_cluster = self.start_conv_cluster(x_cluster) # 区域图特征张量
transmit1 = self.transmit1(x, x_cluster)
x_1 = (torch.einsum('bmn,bcnl->bcml', transmit1, x_cluster))
x = self.gate1(x, x_1)
5 交通预测块(Traffic Forecasting Block)
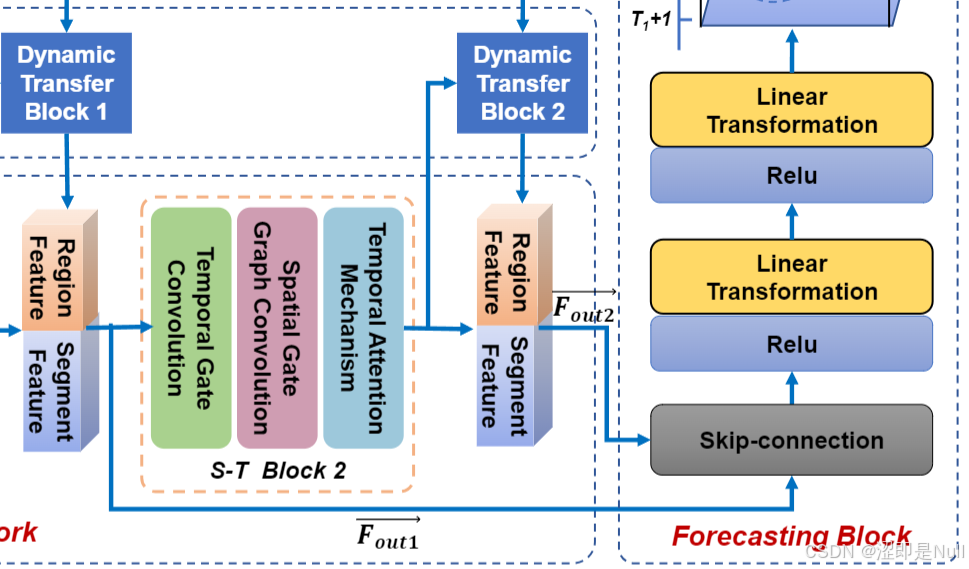
为从不同阶段的特征中提取更多信息,设计了跳跃连接(skip-connection)来汇聚上一步得到的两个不同特征,然后将汇聚结果输入预测模块。对比其他论文使用的残差网络(Res Net),残差连接的过程中加法操作是固定的,总是把原始信息无条件地加回来。而本篇论文使用的跳跃连接 + 动态传递模块则好比是一条"智能通道",不仅传递原始信息,还会根据当前情况自动调节信息的"强度"。如果某部分信息更关键,它就会给它更大的权重;如果不那么重要,就会弱化它。
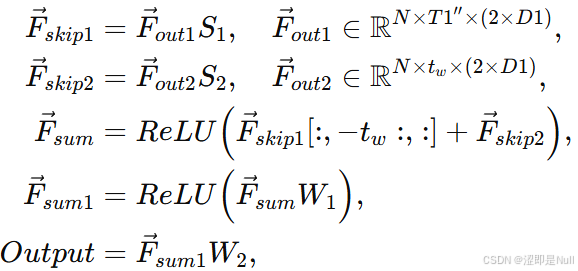
python
self.skip_conv1 = Conv2d(2 * dilation_channels, skip_channels, kernel_size=(1, 1), stride=(1, 1), bias=True)
self.end_conv_1 = nn.Conv2d(in_channels=skip_channels, out_channels=end_channels, kernel_size=(1, 3), bias=True)
self.end_conv_2 = nn.Conv2d(in_channels=end_channels, out_channels=out_dim, kernel_size=(1, 1), bias=True)
s1 = self.skip_conv1(x)
skip = s1 + skip
# output
x = F.relu(skip)
x = F.relu(self.end_conv_1(x))
x = self.end_conv_2(x)