首先创建一个常量张量x1 = tf.constant([1., 2., 3.], dtype=tf.float64)
print("x1:",x1)
x1这里就创建了一个一维的张量,包含3个元素,分别为1,2,3 dtype则指定了类型为64位浮点数
输出结果:x1: tf.Tensor([1. 2. 3.], shape=(3,), dtype=float64)
x2 = tf.cast(x1, tf.int32)
tf.cast 是 TensorFlow 中用于进行数据类型转换的函数。它接受两个参数,第一个参数是要转换的张量,第二个参数是目标数据类型。
这里将 x1 从 tf.float64 类型转换为 tf.int32 类型,转换过程中会进行截断操作(直接舍弃小数部分)。
输出结果:x2 tf.Tensor([1 2 3], shape=(3,), dtype=int32)
print("minimum of x2:", tf.reduce_min(x2))
print("maxmum of x2:", tf.reduce_max(x2))
分别计算张量x2的最小值和最大值
输出结果:minimum of x2: tf.Tensor(1, shape=(), dtype=int32)
maxmum of x2: tf.Tensor(3, shape=(), dtype=int32)
axis:
axis的值,零竖 一横。
Variable函数
只有当两个张量的维度相同的时候才可以进行四则运算。
举个例子:
import tensorflow as tf
a = tf.ones([1, 3])
b = tf.fill([1, 3], 3.)
print("a:", a)
print("b:", b)
print("a+b:", tf.add(a, b))
print("a-b:", tf.subtract(a, b))
print("a*b:", tf.multiply(a, b))
print("b/a:", tf.divide(b, a))
输出结果为
a: tf.Tensor([[1. 1. 1.]], shape=(1, 3), dtype=float32)
b: tf.Tensor([[3. 3. 3.]], shape=(1, 3), dtype=float32)
a+b: tf.Tensor([[4. 4. 4.]], shape=(1, 3), dtype=float32)
a-b: tf.Tensor([[-2. -2. -2.]], shape=(1, 3), dtype=float32)
a*b: tf.Tensor([[3. 3. 3.]], shape=(1, 3), dtype=float32)
b/a: tf.Tensor([[3. 3. 3.]], shape=(1, 3), dtype=float32)
tf.ones意思是创建一个全为1的张量,[1,3]表示的是这里创建的是形状为(1,3)的二维张量,也就是1行3列矩阵,每个元素的值为1。
b则是创建一个同样的1行3列矩阵,但是每个元素的值为3。
a = tf.fill([1, 2], 3.)
print("a:", a)
print("a的平方:", tf.pow(a, 3))
print("a的平方:", tf.square(a))
print("a的开方:", tf.sqrt(a))
第一个操作,进行幂运算,指数幂为3,也就是3次方。
第二个是平方操作
第三个是开放操作
输出结果为
a: tf.Tensor([[3. 3.]], shape=(1, 2), dtype=float32)
a的平方: tf.Tensor([[27. 27.]], shape=(1, 2), dtype=float32)
a的平方: tf.Tensor([[9. 9.]], shape=(1, 2), dtype=float32)
a的开方: tf.Tensor([[1.7320508 1.7320508]], shape=(1, 2), dtype=float32)
a = tf.ones([3, 2])
b = tf.fill([2, 3], 3.)
print("a:", a)
print("b:", b)
print("a*b:", tf.matmul(a, b))
进行矩阵相乘操作,这里是3X2矩阵和一个2X3矩阵相乘,得到一个3X3的矩阵。
为:
故输出结果为:
a: tf.Tensor(
[[1. 1.]
[1. 1.]
[1. 1.]], shape=(3, 2), dtype=float32)
b: tf.Tensor(
[[3. 3. 3.]
[3. 3. 3.]], shape=(2, 3), dtype=float32)
a*b: tf.Tensor(
[[6. 6. 6.]
[6. 6. 6.]
[6. 6. 6.]], shape=(3, 3), dtype=float32)
features = tf.constant([12, 23, 10, 17])
labels = tf.constant([0, 1, 1, 0])
dataset = tf.data.Dataset.from_tensor_slices((features, labels))
for element in dataset:
print(element)
features 张量存储了特征数据,这里有 4 个特征值 [12, 23, 10, 17]。
labels 张量存储了对应的标签数据,同样有 4 个标签值 [0, 1, 1, 0]。
dataset = tf.data.Dataset.from_tensor_slices((features, labels)),这个函数用于创建数据集对象,把标签和特征配成对。
12与0配对
23与1配对
10与1配对
17与0配对
输出结果为:
(<tf.Tensor: shape=(), dtype=int32, numpy=12>, <tf.Tensor: shape=(), dtype=int32, numpy=0>)
(<tf.Tensor: shape=(), dtype=int32, numpy=23>, <tf.Tensor: shape=(), dtype=int32, numpy=1>)
(<tf.Tensor: shape=(), dtype=int32, numpy=10>, <tf.Tensor: shape=(), dtype=int32, numpy=1>)
(<tf.Tensor: shape=(), dtype=int32, numpy=17>, <tf.Tensor: shape=(), dtype=int32, numpy=0>)
求导函数:
with tf.GradientTape() as tape:
x = tf.Variable(tf.constant(3.0))
y = tf.pow(x, 2)
grad = tape.gradient(y, x)
print(grad)
这里创建初始值x,值为3,损失函数为x^2,损失函数对其求导数,为2x, 带入x=3,得到结果为6。
输出结果:tf.Tensor(6.0, shape=(), dtype=float32)
如何把待转换的数据转换成one_hot类型?如下操作:
import tensorflow as tf
classes = 3
labels = tf.constant([1, 0, 2]) # 输入的元素值最小为0,最大为2
output = tf.one_hot(labels, depth=classes)
print("result of labels1:", output)
print("\n")
使用方法:
tf.one_hot(待转换数据,depth=几分类)
classes=3,这里意思是分三类
这组标签是1,0,2
输出结果:
result of labels1: tf.Tensor(
[[0. 1. 0.]
[1. 0. 0.]
[0. 0. 1.]], shape=(3, 3), dtype=float32)
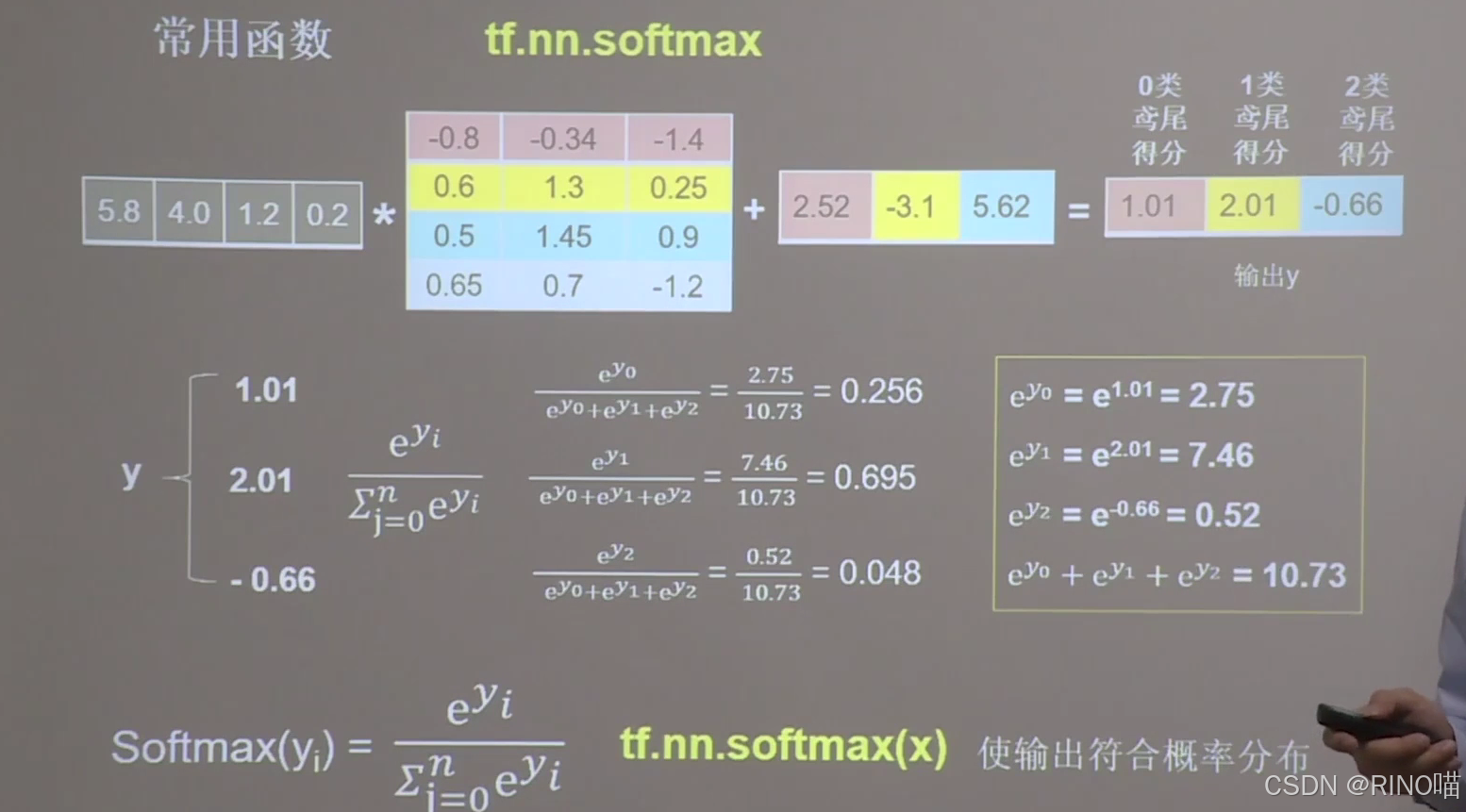
数据经过处理后得到了每种数字的可能性大小,但是他们目前不符合概率分布,因为我们要得到一个Ω的情况下三个可能性相加的数字应该为1。经过函数处理后,可以得到中间的部分,其意思是0类鸾尾的几率为0.256,1类鸾尾的几率为0.695,2类鸾尾的几率为0.048,这三个合就为1了。
softmax()函数用于实现的计算。
softmax函数可以使n分类的n个函数输出Y0,y1,到yn-1的概率,这些概率和为1.
例子为:
import tensorflow as tf
x1 = tf.constant([[5.8, 4.0, 1.2, 0.2]]) # 5.8,4.0,1.2,0.2(0)
w1 = tf.constant([[-0.8, -0.34, -1.4],
[0.6, 1.3, 0.25],
[0.5, 1.45, 0.9],
[0.65, 0.7, -1.2]])
b1 = tf.constant([2.52, -3.1, 5.62])
y = tf.matmul(x1, w1) + b1
print("x1.shape:", x1.shape)
print("w1.shape:", w1.shape)
print("b1.shape:", b1.shape)
print("y.shape:", y.shape)
print("y:", y)
#####以下代码可将输出结果y转化为概率值#####
y_dim = tf.squeeze(y) # 去掉y中纬度1(观察y_dim与 y 效果对比)
y_pro = tf.nn.softmax(y_dim) # 使y_dim符合概率分布,输出为概率值了
print("y_dim:", y_dim)
print("y_pro:", y_pro)
#请观察打印出的shape
输出结果是:
x1.shape: (1, 4)
w1.shape: (4, 3)
b1.shape: (3,)
y.shape: (1, 3)
y: tf.Tensor([[ 1.0099998 2.008 -0.65999985]], shape=(1, 3), dtype=float32)
y_dim: tf.Tensor([ 1.0099998 2.008 -0.65999985], shape=(3,), dtype=float32)
y_pro: tf.Tensor([0.2563381 0.69540703 0.04825491], shape=(3,), dtype=float32)
最后一个就是各自的概率值了。
各张量值的含义
y: tf.Tensor([[ 1.0099998 2.008 -0.65999985]], shape=(1, 3), dtype=float32)
y 的具体值是一个二维数组 [[ 1.0099998, 2.008, -0.65999985]],表示该样本在 3 个不同类别上的得分。得分越高,说明该样本属于对应类别的可能性相对越大,但这些得分还不是概率值。
y_dim: tf.Tensor([ 1.0099998 2.008 -0.65999985], shape=(3,), dtype=float32)
y_dim 是 y 经过 tf.squeeze 函数处理后的结果,它移除了 y 中维度为 1 的维度,将二维张量转换为一维张量。其值与 y 中的一行相同,仍然表示该样本在 3 个不同类别上的得分。
y_pro: tf.Tensor([0.2563381 0.69540703 0.04825491], shape=(3,), dtype=float32)
y_pro 是 y_dim 经过 tf.nn.softmax 函数处理后的结果,它将 y_dim 转换为概率分布。每个元素的值都在 0 到 1 之间,并且它们的和为 1。具体来说,这 3 个概率值分别表示该样本属于 3 个不同类别的概率。例如,该样本属于第二个类别的概率最高,为 0.69540703,属于第三个类别的概率最低,为 0.04825491。在分类任务中,通常会选择概率最大的类别作为该样本的预测类别。
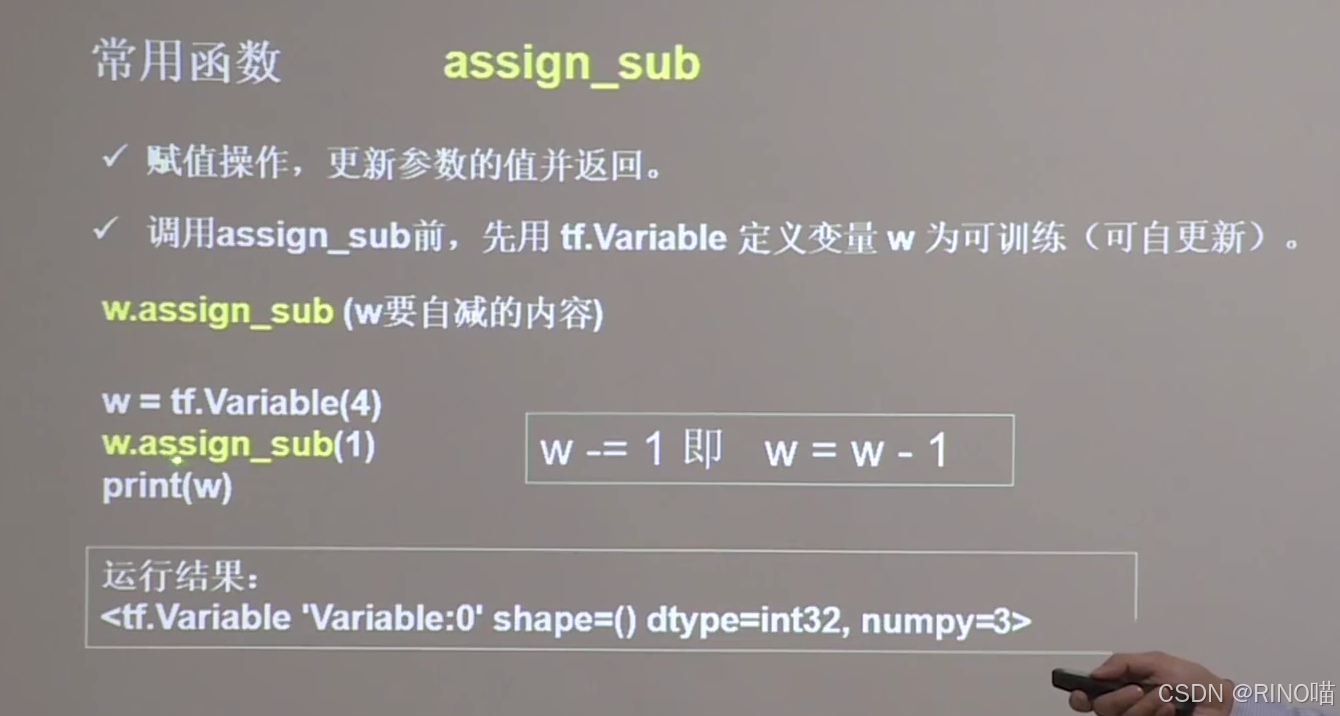
运行内容是w初始值为4,做自减1的操作,结果是3
argmax函数:
import numpy as np
import tensorflow as tf
test = np.array([[1, 2, 3], [2, 3, 4], [5, 4, 3], [8, 7, 2]])
print("test:\n", test)
print("每一列的最大值的索引:", tf.argmax(test, axis=0)) # 返回每一列最大值的索引
print("每一行的最大值的索引", tf.argmax(test, axis=1)) # 返回每一行最大值的索引
输出结果:
test:
[[1 2 3]
[2 3 4]
[5 4 3]
[8 7 2]]
每一列的最大值的索引: tf.Tensor([3 3 1], shape=(3,), dtype=int64)
每一行的最大值的索引 tf.Tensor([2 2 0 0], shape=(4,), dtype=int64)
索引都是从0开始的。