《------往期经典推荐------》
二、机器学习实战专栏【链接】 ,已更新31期,欢迎关注,持续更新中~~
三、深度学习【Pytorch】专栏【链接】
四、【Stable Diffusion绘画系列】专栏【链接】
五、YOLOv8改进专栏【链接】,持续更新中~~
六、YOLO性能对比专栏【链接】,持续更新中~
《------正文------》
目录
基于U-Net结构的图像分割
图像分割是计算机视觉中的一项重要任务,其目标是将图像中的每个像素分类到特定类别中。UNet是一种流行的深度学习架构,广泛用于生物医学和一般分割任务。
什么是UNet?
UNet是一个完全卷积神经网络(CNN),专为图像分割而设计。它包括:
- 编码器: 使用卷积层捕获空间信息。
- 解码器: 对特征图进行上采样以匹配输入分辨率。
- 跳跃连接: 编 码器和解码器之间的桥梁,以保留细粒度的细节。
在PyTorch中实现UNet进行图像分割
步骤1:安装库
pip install torch torchvision matplotlib
步骤2:定义UNet模型
python
import torch
import torch.nn as nn
import torch.nn.functional as F
class UNet(nn.Module):
def __init__(self, in_channels=1, out_channels=1):
super(UNet, self).__init__()
self.encoder = nn.Sequential(
nn.Conv2d(in_channels, 64, kernel_size=3, padding=1),
nn.ReLU(),
nn.Conv2d(64, 64, kernel_size=3, padding=1),
nn.ReLU()
)
self.decoder = nn.Sequential(
nn.Conv2d(64, out_channels, kernel_size=1)
)
def forward(self, x):
x = self.encoder(x)
x = self.decoder(x)
return torch.sigmoid(x)
# Initialize the model
model = UNet()
步骤3:加载图像并执行推理
python
from PIL import Image
import torchvision.transforms as T
import matplotlib.pyplot as plt
# Load an image and preprocess it
image_path = "image.jpg"
image = Image.open(image_path).convert("L")
transform = T.Compose([T.ToTensor()])
image_tensor = transform(image).unsqueeze(0)
# Run inference
with torch.no_grad():
output = model(image_tensor)
# Display segmentation result
plt.imshow(output.squeeze(), cmap="gray")
plt.show()
结论
UNet是一个强大的图像分割任务架构,适用于医学成像、自动驾驶和许多其他应用。PyTorch提供了一个灵活的框架,可以有效地构建和微调U-net模型。
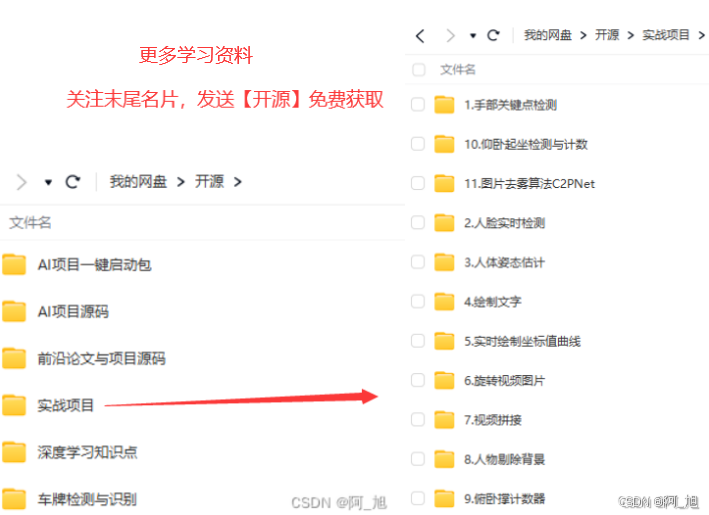
好了,这篇文章就介绍到这里,喜欢的小伙伴感谢给点个赞和关注,更多精彩内容持续更新~~
关于本篇文章大家有任何建议或意见,欢迎在评论区留言交流!