HuBERT预训练过程中会用到K-means算法,本文简单介绍一下K-means算法的基本流程。
简单地讲,K-means就是给特征向量集进行聚类。给定一个特征向量集{X}
和目标聚类数N
,K-means会不断迭代,直到X被分成N类,且每一类的中心点不再明显变化。
先看一个简单例子:
python
from sklearn.cluster import KMeans
import numpy as np
import matplotlib.pyplot as plt
# Example data: 2D points
data = np.array([
[1.0, 2.0],
[1.5, 1.8],
[5.0, 8.0],
[8.0, 8.0],
[1.0, 0.6],
[9.0, 11.0],
[8.0, 2.0],
[10.0, 2.0],
[9.0, 3.0]
])
# Create a KMeans instance with 3 clusters
kmeans = KMeans(n_clusters=3, random_state=42)
# Fit the KMeans model to the data
kmeans.fit(data)
# Get the cluster centroids
centroids = kmeans.cluster_centers_
# Get the labels for each point
labels = kmeans.labels_
# Plot the data points and the cluster centroids
plt.scatter(data[:, 0], data[:, 1], c=labels, cmap='viridis', marker='o', label='Data Points')
plt.scatter(centroids[:, 0], centroids[:, 1], s=300, c='red', marker='x', label='Centroids')
plt.title('K-means Clustering')
plt.xlabel('Feature 1')
plt.ylabel('Feature 2')
plt.legend()
plt.show()
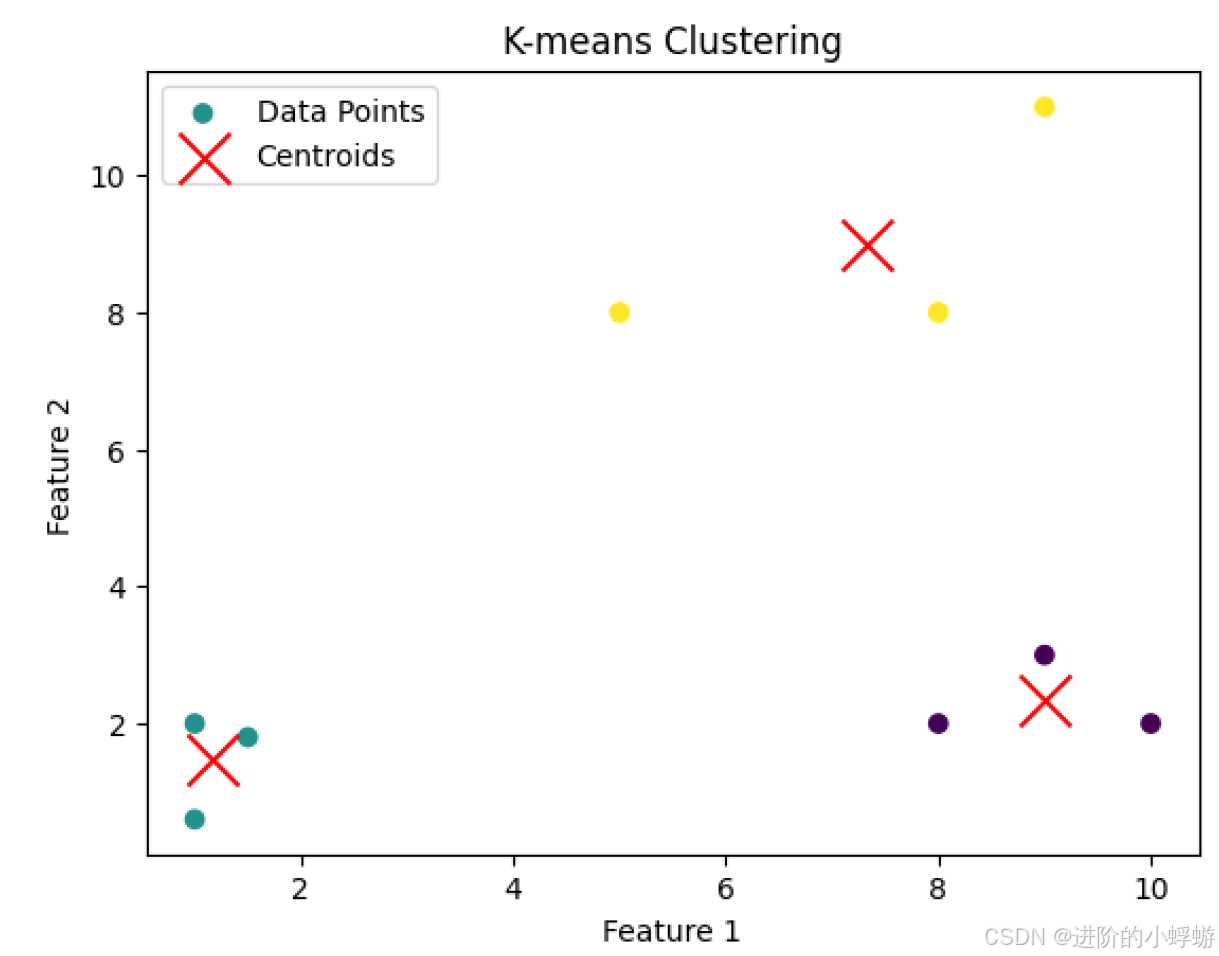
这个例子中,特征向量集是二维点,目标聚类数是分成3类,调用fit()
后K-means模型就会不断迭代直到收敛,即每一类的中心点不再明显变化。可以看到,最后的二维点集被分成了三类。那调用fit()
后K-means模型具体是怎么迭代的呢?
- Initialization :首先进行初始化,模型会先选择中心点的初始位置。初始位置的选择有多种方法,比较常用的是
k-means++
和random
,可以在创建KMeans
对象时通过init=
参数设置。如果选择k-means++
来初始化中心点,还可以通过n_init=
参数来设置random start的次数,比如n_init=10
表示模型会用不同的初始中心点跑10次,选择最优的那次作为最后的结果。 - Assignment Step :每一个特征向量都会被指定到离它最近的中心点,衡量与中心点的距离通常使用
Euclidean distance
。 - Update Step :当所有的特征向量都被指定好后,中心点的位置会重新计算。中心点新的位置是所有指定给它的特征向量的平均值。比如
(x1,y1)
,(x2,y2)
,(x3,y3)
这三个特征向量是一类,那么该类新的中心点位置就是((x1+x2+x3)/3, (y1+y2+y3)/3)
- Iteration :步骤2和步骤3会重复执行直到收敛。收敛的标准是中心点的位置的变化量低于设置的阈值。另外,当迭代次数达到设置的最大迭代数时,迭代也会停止。收敛阈值可以通过
tol=
参数设置,最大迭代数可以通过max_iter=
参数设置。
K-means模型训练好后,可以dump成文件,以便重复使用。dump可以通过`joblib`或`pickle`来实现。那dump好的文件里存的都是什么呢?
dump文件里存的是模型序列化之后的数据:
- Cluster Centers(cluster_centers_):中心点的位置
- Labels(labels_):每个特征向量被指定的聚类的标签
- Inertia(inertia_):每个聚类的紧凑程度。它的值是所有特征向量到离它最近的中心点的平方距离的和
- Number of Iterations(n_iter_):模型收敛前迭代的次数
- Model Parameters :聚类的个数,中心点初始化方法,收敛的指标,以及其他在创建
KMeans
对象时的参数。
当重新load模型时,这些数据会被恢复使得可以重新使用训练好的模型。
下面是更详细的例子:
python
from sklearn.cluster import KMeans
import numpy as np
import joblib
import matplotlib.pyplot as plt
# Example data: 2D points
data = np.array([
[1.0, 2.0],
[1.5, 1.8],
[5.0, 8.0],
[8.0, 8.0],
[1.0, 0.6],
[9.0, 11.0],
[8.0, 2.0],
[10.0, 2.0],
[9.0, 3.0]
])
# Create a KMeans instance with 3 clusters
kmeans = KMeans(n_clusters=3, init='k-means++', n_init=10, max_iter=300, tol=1e-4, random_state=42)
# Fit the KMeans model to the data
kmeans.fit(data)
# Accessing internal components
print("Cluster Centers:\n", kmeans.cluster_centers_)
print("Labels:", kmeans.labels_)
print("Inertia:", kmeans.inertia_)
print("Number of Iterations:", kmeans.n_iter_)
# Calculate cluster sizes
unique_labels, counts = np.unique(kmeans.labels_, return_counts=True)
cluster_sizes = dict(zip(unique_labels, counts))
print("Cluster Sizes:", cluster_sizes)
# Save the model to a .bin file
joblib.dump(kmeans, 'kmeans_model.bin')
# Later, load the model from the .bin file
loaded_model = joblib.load('kmeans_model.bin')
# Use the loaded model
print("Loaded Model Centroids:\n", loaded_model.cluster_centers_)
# Get the cluster centroids
centroids = loaded_model.cluster_centers_
# Get the labels for each point
labels = loaded_model.labels_
# Plot the data points and the cluster centroids
plt.scatter(data[:, 0], data[:, 1], c=labels, cmap='viridis', marker='o', label='Data Points')
plt.scatter(centroids[:, 0], centroids[:, 1], s=300, c='red', marker='x', label='Centroids')
plt.title('K-means Clustering')
plt.xlabel('Feature 1')
plt.ylabel('Feature 2')
plt.legend()
plt.show()