广义傅里叶族变换是一种时频变换方法,傅里叶变换、短时傅里叶变换、S变换和许多小波变换都是其特殊情况,完整代码及子函数如下,很容易读懂:
Matlab
% Run a demo by creating a signal, transforming it, and plotting the results
% Create a fake signal
N = 256;
x = linspace(0,1,N);
sig = zeros(1,length(x));
% signal example 1 (a single delta)
sig(N/2) = 1.0;
% signal example 2 (a mixture of sinusoids and a delta)
% sig(1:N/2) += (sin((N/16)*2*pi*x)*1.0)(1:N/2);
% sig(N/2+1:N) += (cos((N/8)*2*pi*x)*1.0)(N/2+1:N);
% sig(2*N/16+1:3*N/16) += (sin((N/4)*2*pi*x)*1.0)(2*N/16+1:3*N/16);
% sig(N/2+N/4+1) = 2.0;
% Do the transform
partitions = octavePartitions(N);
windows = boxcarWindows(partitions);
SIG = GFT(sig,partitions,windows);
% Interpolate to get a spectrogram
% The third and fourth parameters set the time and frequency axes respectively,
% and can be changed to raise or lower the resolution, or zoom in on
% a feature of interest
spectrogram = interpolateGFT(SIG,partitions,1024,1024);
% Display
figure();
subplot(3,1,1);
plot(x,sig,'DisplayName','signal');
legend('Location','northeast')
ax = subplot(3,1,2);
hold on;
for p = partitions
line([x(p),x(p)],[0,max(abs(SIG))],'Color',[1 0 0],'linestyle','--');
end
p = plot(x,abs(SIG),'DisplayName','SIGNAL');
legend(p,'Location','northeast');
subplot(3,1,3);
imagesc(abs(spectrogram));
%%
function partitions = octavePartitions(N)
widths = 2.^(0:round(log(N)/log(2)-2));
widths = [1,widths,flip(widths)];
partitions = [0,cumsum(widths)]+1;
end
%%
function widths = partitionWidths(partitions)
widths = circshift(partitions,-1) - partitions;
widths(length(partitions)) = widths(length(partitions)) + max(partitions);
end
%%
function windows = boxcarWindows(partitions)
windows = ones(1,max(partitions));
end
%%
function SIG = GFT(sig,partitions,windows)
SIG = fft(complex(sig));
SIG = SIG.*windows;
for p = 1:(length(partitions)-1)
SIG(partitions(p):partitions(p+1)-1) = ifft(SIG(partitions(p):partitions(p+1)-1));
end
end
%%
function spectrogram = interpolateGFT(SIG,partitions,tAxis,fAxis,method)
% Interpolate a 1D GFT onto a grid. If axes is specified it should be a
% list or tuple consisting of two arrays, the sampling points on the time and frequency
% axes, respectively. Alternatively, M can be specified, which gives the number
% of points along each axis.
% introduced in R2019 is the arguments block
% https://www.mathworks.com/help/matlab/ref/arguments.html
% arguments
% SIG;
% partitions;
% tAxis;
% fAxis;
% method (1,:) char = 'linear';
% end
% if you don't have have the arguments block, then you can still do input defaults like this:
if nargin<5
method = 'linear';
end
% Caller specified M rather than the actual sampling points
if length(tAxis) == 1
tAxis = 1:length(SIG) / tAxis:length(SIG);
% Centre the samples
tAxis = tAxis + (length(SIG) - tAxis(length(tAxis))) / 2;
end
if length(fAxis) == 1
fAxis = 1:length(SIG) / fAxis:length(SIG);
% Centre the samples
fAxis = fAxis + (length(SIG) - fAxis(length(fAxis))) / 2;
end
N = length(SIG);
widths = partitionWidths(partitions);
spectrogram = complex(length(partitions),zeros(length(tAxis)));
% interpolate each frequency band in time
for p = 1:length(partitions)
% indices of sample points, plus 3 extra on each side in case of cubic interpolation
indices = (-3:widths(p)+2);
% time coordinates of samples
t = indices .* (N/widths(p));
% values at sample points
if (p < length(partitions))
temp = SIG(partitions(p):partitions(p+1)-1);
f = temp(mod(indices,widths(p))+1);
else
temp = SIG(partitions(p):N);
f = temp(mod(indices,widths(p))+1);
end
if (length(f) > 1)
spectrogram(p,:) = interp1(t,f,tAxis,method);
else
spectrogram(p,:) = f;
end
end
% Interpolate in frequency
indices = mod(-3:length(partitions)+2,length(partitions));
f = partitions(indices+1) + widths(indices+1)/2;
f(1:3) = f(1:3) - N;
f(length(f)-2:length(f)) = f(length(f)-2:length(f)) + N;
t = spectrogram(indices+1,:);
spectrogram = interp1(f,t,fAxis,method);
end
function [sig,partitions,windows,SIG] = demo()
% Run a demo by creating a signal, transforming it, and plotting the results
% Create a fake signal
N = 256;
x = linspace(0,1,N);
sig = zeros(1,length(x));
% signal example 1 (a single delta)
sig(N/2) = 1.0;
% signal example 2 (a mixture of sinusoids and a delta)
% sig(1:N/2) += (sin((N/16)*2*pi*x)*1.0)(1:N/2);
% sig(N/2+1:N) += (cos((N/8)*2*pi*x)*1.0)(N/2+1:N);
% sig(2*N/16+1:3*N/16) += (sin((N/4)*2*pi*x)*1.0)(2*N/16+1:3*N/16);
% sig(N/2+N/4+1) = 2.0;
% Do the transform
partitions = octavePartitions(N);
windows = boxcarWindows(partitions);
SIG = GFT(sig,partitions,windows);
% Interpolate to get a spectrogram
% The third and fourth parameters set the time and frequency axes respectively,
% and can be changed to raise or lower the resolution, or zoom in on
% a feature of interest
spectrogram = interpolateGFT(SIG,partitions,1024,1024);
% Display
figure();
subplot(3,1,1);
plot(x,sig,'DisplayName','signal');
legend('Location','northeast')
ax = subplot(3,1,2);
hold on;
for p = partitions
line([x(p),x(p)],[0,max(abs(SIG))],'Color',[1 0 0],'linestyle','--');
end
p = plot(x,abs(SIG),'DisplayName','SIGNAL');
legend(p,'Location','northeast');
subplot(3,1,3);
imagesc(abs(spectrogram));
end
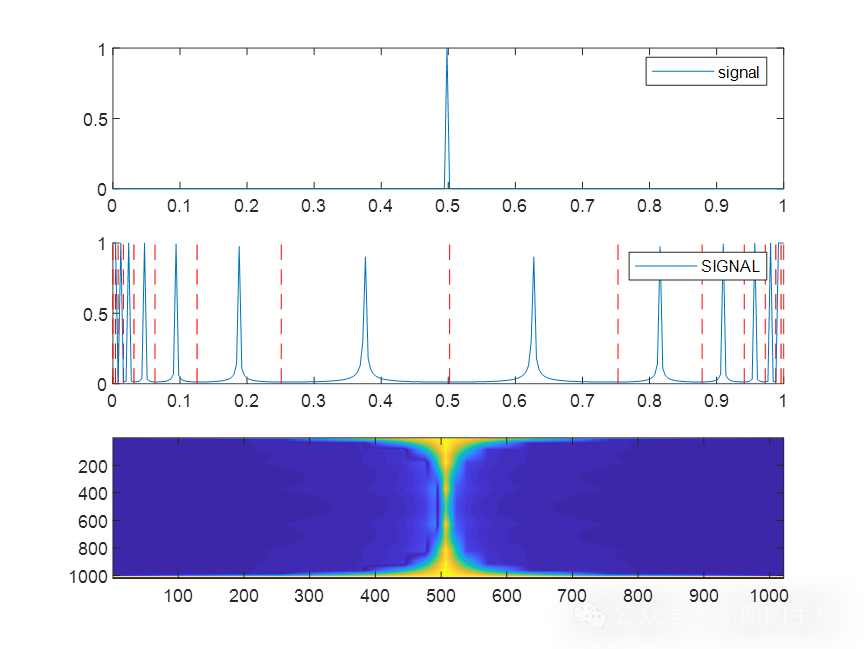
工学博士,担任《Mechanical System and Signal Processing》《中国电机工程学报》《控制与决策》等期刊审稿专家,擅长领域:现代信号处理,机器学习,深度学习,数字孪生,时间序列分析,设备缺陷检测、设备异常检测、设备智能故障诊断与健康管理PHM等。