按:本人开始学习AI了,怎么利用现有的AI开始进行自启发式的学习也是使用AI的一种方式吧。跟着AI学习AI的系列基本上是与ChatGPT或者其他AI产品的对话实录,为了方便特地记录一下。
AI:GPT4o,下面是回答原文拷贝
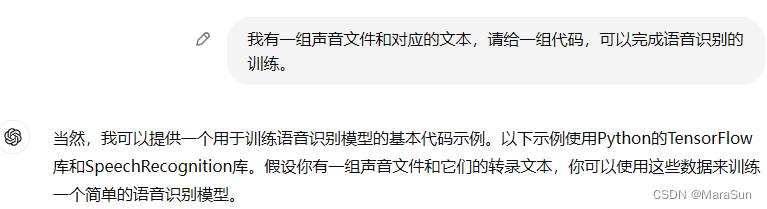
环境准备
首先,确保你已经安装了以下库:
bash
pip install tensorflow
pip install SpeechRecognition
pip install librosa
数据准备
假设你的音频文件位于audio/
目录中,对应的转录文本在transcripts.txt
中,每行的格式为filename transcription
。
代码示例
python
import os
import librosa
import numpy as np
import tensorflow as tf
from tensorflow.keras.layers import Dense, LSTM, Input
from tensorflow.keras.models import Model
# 加载数据
def load_data(audio_dir, transcript_file):
audio_paths = []
transcripts = []
with open(transcript_file, 'r', encoding='utf-8') as f:
for line in f:
parts = line.strip().split(' ', 1)
if len(parts) == 2:
audio_paths.append(os.path.join(audio_dir, parts[0]))
transcripts.append(parts[1])
return audio_paths, transcripts
audio_dir = 'audio'
transcript_file = 'transcripts.txt'
audio_paths, transcripts = load_data(audio_dir, transcript_file)
# 提取特征
def extract_features(audio_paths):
features = []
for path in audio_paths:
y, sr = librosa.load(path, sr=None)
mfcc = librosa.feature.mfcc(y=y, sr=sr, n_mfcc=13)
features.append(np.mean(mfcc.T, axis=0))
return np.array(features)
features = extract_features(audio_paths)
# 对文本进行编码
tokenizer = tf.keras.preprocessing.text.Tokenizer(char_level=True)
tokenizer.fit_on_texts(transcripts)
sequences = tokenizer.texts_to_sequences(transcripts)
transcripts_encoded = tf.keras.preprocessing.sequence.pad_sequences(sequences, padding='post')
# 构建模型
input_dim = features.shape[1]
output_dim = len(tokenizer.word_index) + 1 # 加1是因为Tokenizer从1开始编码
inputs = Input(shape=(input_dim,))
x = Dense(128, activation='relu')(inputs)
x = LSTM(128, return_sequences=True)(x)
x = LSTM(128)(x)
outputs = Dense(output_dim, activation='softmax')(x)
model = Model(inputs, outputs)
model.compile(optimizer='adam', loss='sparse_categorical_crossentropy', metrics=['accuracy'])
# 准备标签
labels = np.array([seq[0] for seq in transcripts_encoded]) # 取每个序列的第一个字符作为标签
# 训练模型
model.fit(features, labels, epochs=10, batch_size=32)
# 保存模型
model.save('speech_recognition_model.h5')
说明
- 数据加载 :
load_data
函数加载音频文件路径和对应的转录文本。 - 特征提取 :
extract_features
函数使用librosa库提取每个音频文件的MFCC特征。 - 文本编码 :使用Keras的
Tokenizer
将文本转为序列,并进行填充。 - 构建模型:构建一个简单的LSTM模型用于语音识别。
- 训练模型:使用提取的特征和编码后的标签进行模型训练。
这只是一个基本的示例,实际应用中你可能需要更复杂的模型架构、更丰富的数据预处理和增强技术来提高模型的准确性。如果你有更多的需求或问题,请告诉我!

2. 特征提取
在语音识别中,特征提取是一个关键步骤。常用的特征之一是MFCC(Mel Frequency Cepstral Coefficients)。
python
def extract_features(audio_paths):
features = []
for path in audio_paths:
y, sr = librosa.load(path, sr=None)
mfcc = librosa.feature.mfcc(y=y, sr=sr, n_mfcc=13)
features.append(np.mean(mfcc.T, axis=0))
return np.array(features)
解释:
librosa.load(path, sr=None)
: 加载音频文件。y
是音频时间序列,sr
是采样率。librosa.feature.mfcc(y=y, sr=sr, n_mfcc=13)
: 计算MFCC特征。n_mfcc=13
表示提取13维的MFCC特征。np.mean(mfcc.T, axis=0)
: 计算MFCC特征的均值。这里简单地取了平均值,实际应用中可能需要更复杂的特征处理。features.append(...)
: 将每个音频文件的特征添加到特征列表中。return np.array(features)
: 返回所有音频文件的特征。
3. 文本编码
为了使模型能够处理文本,我们需要将文本转为数值序列。
python
tokenizer = tf.keras.preprocessing.text.Tokenizer(char_level=True)
tokenizer.fit_on_texts(transcripts)
sequences = tokenizer.texts_to_sequences(transcripts)
transcripts_encoded = tf.keras.preprocessing.sequence.pad_sequences(sequences, padding='post')
解释:
Tokenizer(char_level=True)
: 创建一个字符级别的Tokenizer。这意味着每个字符(而不是单词)将被作为一个标记处理。tokenizer.fit_on_texts(transcripts)
: 根据提供的文本(转录文本)构建字典。tokenizer.texts_to_sequences(transcripts)
: 将文本转为数值序列。例如,"hello" 可能会被编码为 [1, 2, 3, 3, 4]。pad_sequences(sequences, padding='post')
: 对序列进行填充,使它们具有相同的长度(在末尾填充)。
4. 构建模型
我们使用一个简单的LSTM模型来处理音频特征和对应的文本标签。
python
input_dim = features.shape[1]
output_dim = len(tokenizer.word_index) + 1 # 加1是因为Tokenizer从1开始编码
inputs = Input(shape=(input_dim,))
x = Dense(128, activation='relu')(inputs)
x = LSTM(128, return_sequences=True)(x)
x = LSTM(128)(x)
outputs = Dense(output_dim, activation='softmax')(x)
model = Model(inputs, outputs)
model.compile(optimizer='adam', loss='sparse_categorical_crossentropy', metrics=['accuracy'])
解释:
input_dim = features.shape[1]
: 输入特征的维度(MFCC特征的维度)。output_dim = len(tokenizer.word_index) + 1
: 输出维度,即字典中的字符数+1。Input(shape=(input_dim,))
: 输入层,形状为输入特征的维度。Dense(128, activation='relu')
: 全连接层,具有128个神经元和ReLU激活函数。LSTM(128, return_sequences=True)
: 第一层LSTM,有128个神经元,并返回所有时间步的输出。LSTM(128)
: 第二层LSTM,有128个神经元,返回最后一个时间步的输出。Dense(output_dim, activation='softmax')
: 输出层,具有输出维度大小的神经元,并使用softmax激活函数。Model(inputs, outputs)
: 定义模型的输入和输出。model.compile(optimizer='adam', loss='sparse_categorical_crossentropy', metrics=['accuracy'])
: 编译模型,使用Adam优化器和稀疏分类交叉熵损失函数。
5. 训练模型
我们将提取的特征和编码后的标签用于模型的训练。
python
labels = np.array([seq[0] for seq in transcripts_encoded]) # 取每个序列的第一个字符作为标签
model.fit(features, labels, epochs=10, batch_size=32)
# 保存模型
model.save('speech_recognition_model.h5')
解释:
labels = np.array([seq[0] for seq in transcripts_encoded])
: 简单地取每个序列的第一个字符作为标签。实际应用中,标签的处理可能更复杂。model.fit(features, labels, epochs=10, batch_size=32)
: 训练模型,迭代10次,每批次处理32个样本。model.save('speech_recognition_model.h5')
: 保存训练好的模型。
这个例子提供了一个简单的语音识别模型的基本框架。实际应用中,可能需要对数据进行更多的预处理,并且使用更复杂的模型架构来提高性能。
是记:maraSun YBJFWDQ
另:本人将做一个基于AI通告处理系统。