SparkML
一、介绍
Apache Spark ML 是机器学习库在 Apache Spark 上运行的模块。
功能模块介绍
名称 | 功能 |
---|---|
ML Pipeline APIs | 数据模型管道API |
pyspark.ml.param module | 模型参数模块 |
pyspark.ml.feature module | 模型变量相关模块 |
pyspark.ml.classfication module | 分类算法模块 |
pyspark.ml.culstering module | 聚类算法模块 |
pyspark.ml.recommendation module | 推荐系统模块 |
pyspark.ml.regression module | 回归算法模块 |
pyspark.ml.tuning module | 参数调整模块 |
pyspark.ml.evaluation module | 模型验证模块 |
二、模型开发流程
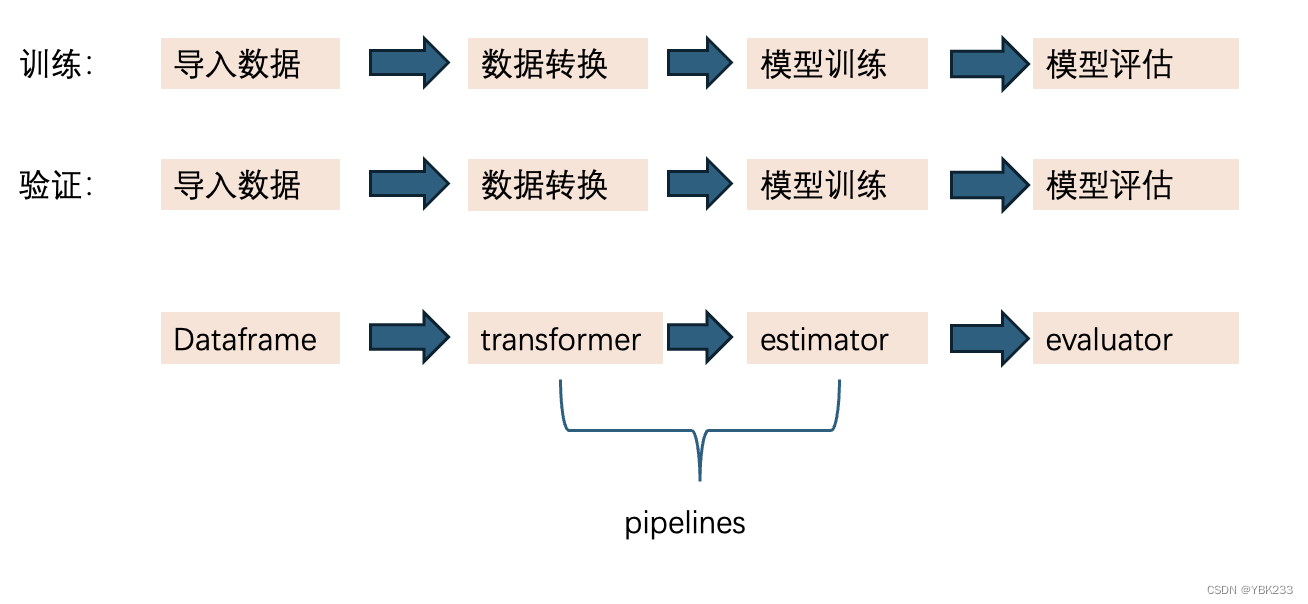
1、dataframe数据模型
ML可以语言于各种数据模型,比如向量、文本、图形等,API采用spark SQL的dataframe来支持各类数据模型
2、transformer转换器
将一个dataframe转换为另一个dataframe,转换过程中,会修改原始变量,或创建新变量
3、estimators模型学习器
- 模型学习器是拟合和训练数据的机器学习算法或其他算法的抽象
- 实现fit()方法,这个方法输入一个dataframe并产生一个model即一个transformer转换器
- 例如:一个机器学习算法是一个estimators模型学习器,比如这个算法是logisticregressionmodel,因此也是一个transformer转换器
4、pipeline管道
- 将多个transformer和estimators绑在一起,形成一个工作流
- 在机器学习中,通常会执行一系列算法来处理和学习模型,比如,一个简单的分类模型开发流程kennel包括以下步骤:
- 将字符变量转换为数值变量
- 进行缺失值、异常值等数据处理
- 使用特征向量和标签学习一个预测模型
三、示例:基于随机森林的新闻分类任务
1、引入相关包
from pyspark.sql import SparkSession
import warnings
from pyspark.sql.functions import *
from pyspark import StorageLevel
warnings.filterwarnings('ignore')
2、初始化spark
spark = (SparkSession
.builder
.appName('文本分类器')
.getOrCreate())
3、读取数据
spark_sinanews = spark.read.json('./data/spark_data/sinaNews_201501.json')
spark_sinanews.show(5)
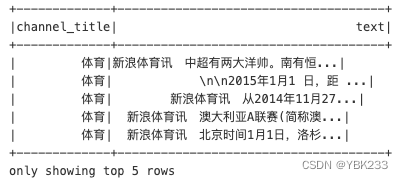
4、查看数据情况
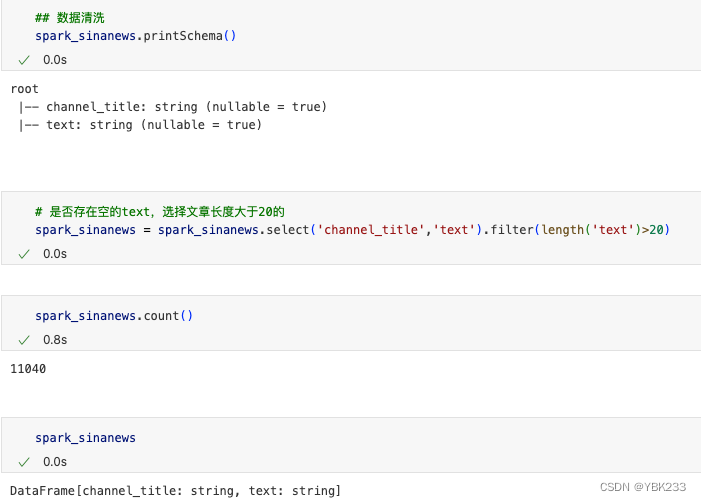
5、数据处理
1、分词
使用jieba分词,安装方法:pip install jieba -i https://pypi.tuna.tsinghua.edu.cn/simple
引入并实例化
import jieba
jieba.initialize()
# 定义udf函数
from pyspark.sql.types import StringType
def cut_words(input_str):
if not jieba.dt.initialized: #主要是应用于分布式的情况
jieba.initialize()
ret = " ".join([w for w in jieba.lcut(input_str)])
return ret
# 向spark注册自定义函数
preprocess_udf = udf(cut_words,StringType())
查看分词效果
# 添加新列
spark_sinanews = spark_sinanews.withColumn('text_words',preprocess_udf('text'))
spark_sinanews.show(2)
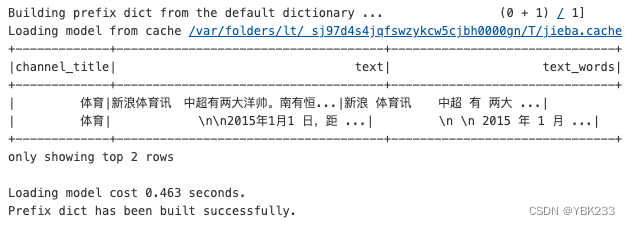
2、类别编码
# 对类别进行编码
spark_sinanews.groupBy('channel_title').count().orderBy(col('count').desc()).show()
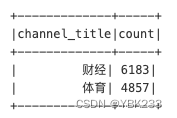
from pyspark.ml.feature import StringIndexer,IndexToString
# 字符------》编码
label_stringIdx = StringIndexer(inputCol='channel_title',outputCol='label').fit(spark_sinanews)
# 编码------》字符 用来看预测结果的
labelConverter = IndexToString(inputCol='prediction',outputCol='predictedLabel',labels=label_stringIdx.labels)
3、去除停用词
# 分词与去除停用词
from pyspark.ml.feature import Tokenizer,StopWordsRemover
# 分词
tokenizer = Tokenizer(inputCol='text_words',outputCol='words')
with open('./data/spark_data/my_stop_words.txt',encoding='utf8')as f:
stop_words = list(f.read().split('\n'))
# 停用词
stop_words_Remover = StopWordsRemover(inputCol='words',outputCol='filtered').setStopWords(stop_words)
4、bow特征
from pyspark.ml import Pipeline
from pyspark.ml.feature import CountVectorizer
#(个数 ,[编码],[频次])
# 计算总的字数
vocab_tmp = spark_sinanews.select('text_words').rdd.flatMap(lambda line :line['text_words'].split(" "))
vocab = vocab_tmp.map(lambda word :(word,1)).reduceByKey(lambda a,b:a+b)
vocab.count()
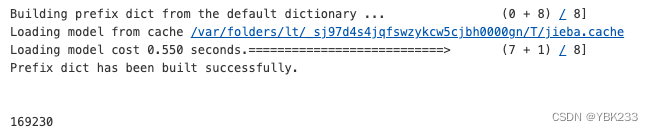
# bag of words count
# CountVectorizer将根据语料库中的词频排序选出前vocabSize个词,由于内存限制,取小些
countVectors = CountVectorizer(inputCol='filtered',outputCol='features',vocabSize=10000)
# 1、分词 2、去除停用词 3、bow特征 4、y标签转换
pipeline = Pipeline(stages=[tokenizer,stop_words_Remover,countVectors,label_stringIdx])
# fit the pipeline to training documents
pipelineFit = pipeline.fit(spark_sinanews)
dataSet = pipelineFit.transform(spark_sinanews)
dataSet.show(1)

dataSet.select('features').show(2)
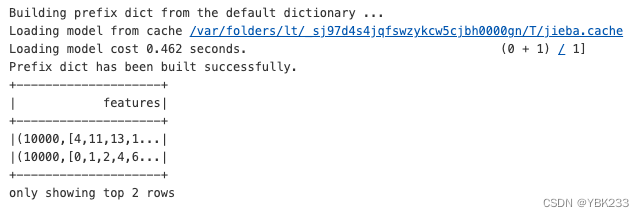
5、数据集切分
dataSet.persist(storageLevel=StorageLevel(True,False,False,False))
# set seed for reproducibility
trainData,testData = dataSet.randomSplit([0.7,0.3],seed=100)
print('train data count:'+str(trainData.count()))
print('test data count:'+str(testData.count()))
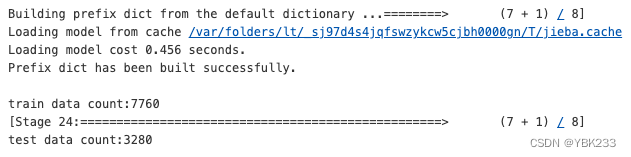
6、建立随机森林模型
# 随机森林模型
from pyspark.ml.classification import RandomForestClassifier
# 随便拍一个参数
rf = RandomForestClassifier(labelCol='label',\
featuresCol='features',\
numTrees=100,\
maxDepth=4,\
maxBins=32)
pipeline = Pipeline(stages=[tokenizer,stop_words_Remover,countVectors,label_stringIdx,rf,labelConverter])
trainData,testData = spark_sinanews.randomSplit([0.7,0.3])
trainData.persist()
testData.persist()

7、模型训练
# train model ,this also runs the indexers
model = pipeline.fit(trainData)
8、模型预测
predictions = model.transform(testData)
predictions.select('filtered','channel_title','features','prediction','label','predictedLabel').show(5)
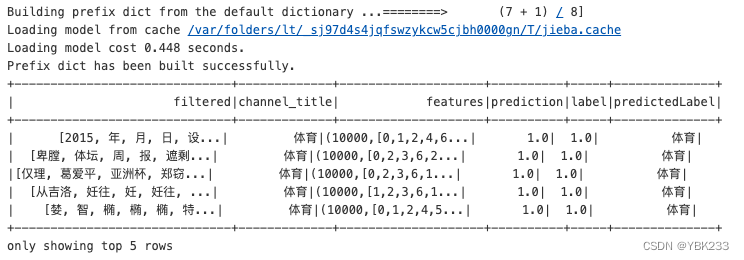
9、关闭spark资源
spark.stop()