一、背景和发展历程
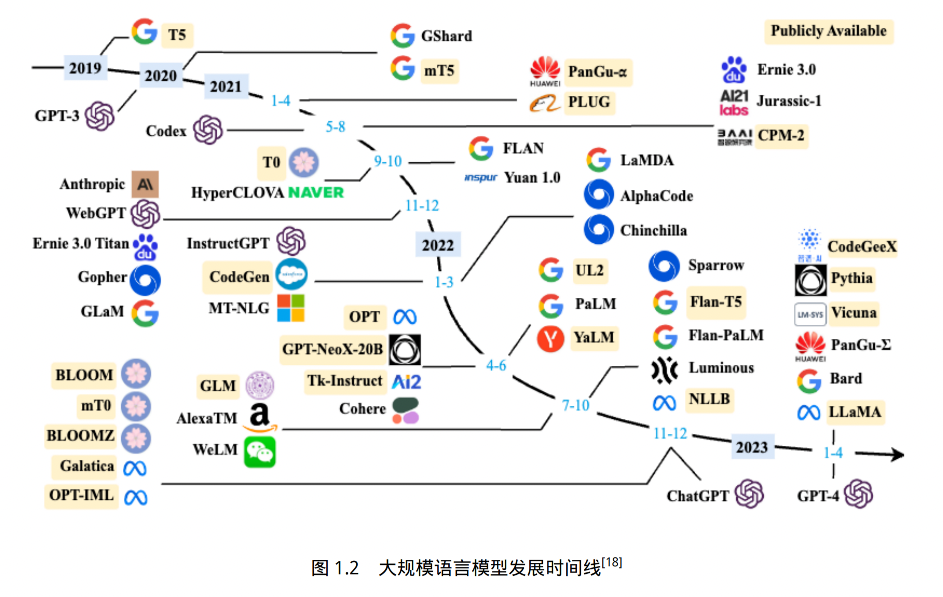
大语言模型四个训练阶段:
-
预训练:
利用海量的训练数据,包括互联网网页、维基百科、书籍、GitHub、 论文、问答网站等,构建包含数千亿甚至数万亿单词的具有多样性的内容。利用由数千块高性能 GPU 和高速网络组成超级计算机,花费数十天完成深度神经网络参数训练,构建基础语言模型
(Base Model)。基础大模型构建了长文本的建模能力,使得模型具有语言生成能力,根据输入的提示词(Prompt),模型可以生成文本补全句子。
-
有监督微调 SFT:也叫指令微调(Instruction Tuning)
利用少量高质量数据几何,包含 prompt 和对应的理想输出。利用这些有监督数据,使用与预训练阶段相同的语言模型训练算法,在基础语言模型基础上再进行训练,从而得到有监督微调模型(SFT 模型)。经过训练的 SFT 模型具备了初步的指令理解能力和上下文理解能力,能够完成开放领域问题、阅读理解、翻译、生成代码等能力,也具备了一定的对未知任务的泛化能力。由于有监督微调阶段的所需的训练语料数量较少,SFT 模型的训练过程并不需要消耗非常大量的计算。根据模型的大小和训练数据量,通常需要数十块 GPU,花费数天时间完成训练。SFT 模型具备了初步的任务完成能力,可以开放给用户使用,很多类 ChatGPT 的模型都属于该类型,包括:Alpaca[38]、Vicuna[39]、MOSS、ChatGLM-6B 等。
-
奖励建模(Reward Modeling,RM):构建一个文本质量对比模型
对于同一个提示词,SFT
模型给出的多个不同输出结果的质量进行排序。奖励模型(RM 模型)可以通过二分类模型,对输入的两个结果之间的优劣进行判断。RM 模型与基础语言模型和 SFT 模型不同,RM 模型本身并不能单独提供给用户使用。奖励模型的训练通常和 SFT 模型一样,使用数十块 GPU,通过几天时间完成训练。由于 RM 模型的准确率对于强化学习阶段的效果有着至关重要的影响,因此对于该模型的训练通常需要大规模的训练数据。
-
强化学习:RL
根据数十万用户给出的提示词,利用在前一阶段训练的 RM 模型,给出 SFT 模型对用户提示词补全结果的质量评估,并与语言模型建模目标综合得到更好的效果。该阶段所使用的提示词数量与有监督微调阶段类似,数量在十万量级,并且不需要人工提前给出该提示词所对应的理想回复。使用强化学习,在 SFT 模型基础上调整参数,使得最终生成的文本可以获得更高的奖励(Reward)。该阶段所需要的计算量相较预训练阶段也少很多,通常也仅需要数十块 GPU,经过数天时间的即可完成训练。
二、大语言模型基础
2.1 Transformer
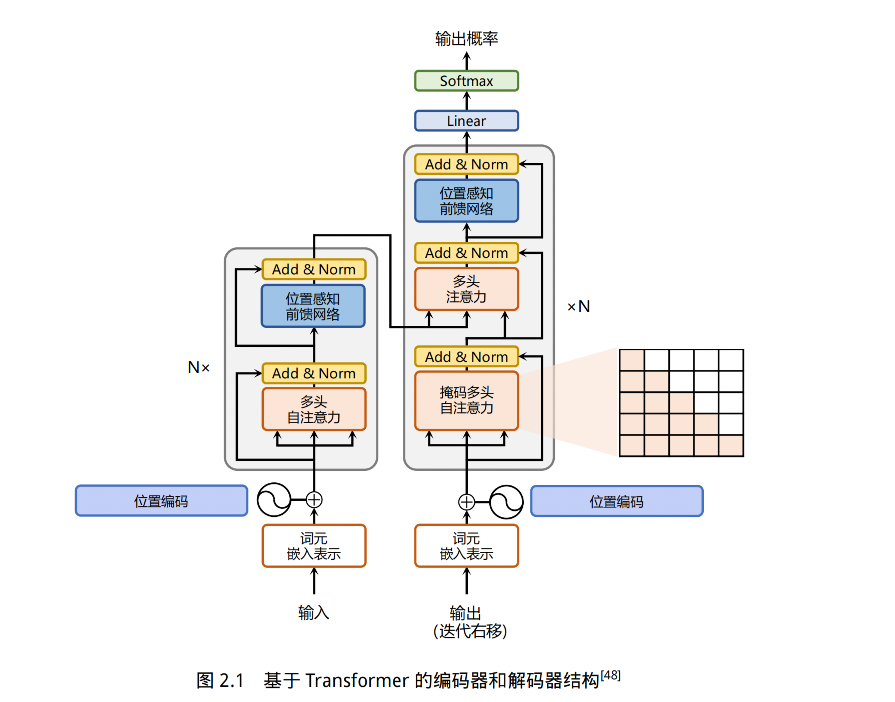
1、嵌入表示层
对于输入的文本,需要经过嵌入表示层,将单词转换为向量,还需要加上位置编码,表明单词的相对位置,在训练的过程中模型会自动的学习到如何利用这部分位置信息
对于不同位置的编码,Transformer 使用不同频率的正余弦函数:

- pos 表示单词所在的位置
- 2i 和 2i+1 表示位置编码向量中的对应维度
- d 是位置编码的总维度
这样计算位置编码的好处:
- 正余弦函数范围为 [-1,1],导出的位置编码与原词嵌入相加不会是的结果偏离过远而破坏原有单词的语义信息
- 根据三角函数的性质,可以得知第 pos+k 个位置的编码是第 pos 个位置编码的线性组合,这就意味着位置编码中蕴含着单词之间的距离信息
python
class PositionalEncoder(nn.Module):
def __init__(self, d_model, max_seq_len = 80):
super().__init__()
self.d_model = d_model
# 根据 pos 和 i 创建一个常量 PE 矩阵
pe = torch.zeros(max_seq_len, d_model)
for pos in range(max_seq_len):
for i in range(0, d_model, 2):
pe[pos, i] = math.sin(pos / (10000 ** ((2 * i)/d_model)))
pe[pos, i + 1] = math.cos(pos / (10000 ** ((2 * (i + 1))/d_model)))
pe = pe.unsqueeze(0)
self.register_buffer('pe', pe)
def forward(self, x):
# 使得单词嵌入表示相对大一些
x = x * math.sqrt(self.d_model)
# 增加位置常量到单词嵌入表示中
seq_len = x.size(1)
x = x + Variable(self.pe[:,:seq_len], requires_grad=False).cuda()
return x
2、注意力层
self-attention 是 Transformer 的基础操作,为了实现对上下文语义依赖的建模,其中涉及到 query、key、value 这三部分
这些权重反应了在编码当前单词的表达时,对于上下文不同部分所需要关注的程度,如图 2.2,通过三个线性变换(右边三个多维向量)将输入序列中每个单词表示转换为三个不同的向量
当要计算第 i 个位置上的单词和其他位置单词的上下文关系时,就计算 如下的分数,为了防止过大的匹配分数在后续的 softmax 计算过程中导致的梯度爆炸以及收敛效率差的我那天,会除以根号 d 来稳定优化过程。

上述过程公式如下:

为了增强自注意力机制聚合上下文信息的能力,进一步还有多头自注意力,让模型关注上下文的不同方面,也就是会有多个 Z 返回,然后使用 WO 来综合不同 head 的输出,得到最终的结果
python
class MultiHeadAttention(nn.Module):
def __init__(self, heads, d_model, dropout = 0.1):
super().__init__()
self.d_model = d_model
self.d_k = d_model // heads
self.h = heads
self.q_linear = nn.Linear(d_model, d_model)
self.v_linear = nn.Linear(d_model, d_model)
self.k_linear = nn.Linear(d_model, d_model)
self.dropout = nn.Dropout(dropout)
self.out = nn.Linear(d_model, d_model)
def attention(q, k, v, d_k, mask=None, dropout=None):
scores = torch.matmul(q, k.transpose(-2, -1)) / math.sqrt(d_k)
# 掩盖掉那些为了填补长度增加的单元,使其通过 softmax 计算后为 0
if mask is not None:
mask = mask.unsqueeze(1)
scores = scores.masked_fill(mask == 0, -1e9)
scores = F.softmax(scores, dim=-1)
if dropout is not None:
scores = dropout(scores)
output = torch.matmul(scores, v)
return output
def forward(self, q, k, v, mask=None):
bs = q.size(0)
# 进行线性操作划分为成 h 个头
k = self.k_linear(k).view(bs, -1, self.h, self.d_k)
q = self.q_linear(q).view(bs, -1, self.h, self.d_k)
v = self.v_linear(v).view(bs, -1, self.h, self.d_k)
# 矩阵转置
k = k.transpose(1,2)
q = q.transpose(1,2)
v = v.transpose(1,2)
# 计算 attention
scores = attention(q, k, v, self.d_k, mask, self.dropout)
# 连接多个头并输入到最后的线性层
concat = scores.transpose(1,2).contiguous().view(bs, -1, self.d_model)
output = self.out(concat)
return output
3、前馈层
前馈层接受自注意力子层的输出作为输入,并通过一个带有 Relu 激活函数的两层全连接网络对输入进行更加复杂的非线性变换。实验证明,这一非线性变换会对模型最终的性能产生十分重要的影响。实验结果表明,增大前馈子层隐状态的维度有利于提升最终翻译结果的质量,因此,前馈子层隐状态的维度一般比自注意力子层要大。

python
class FeedForward(nn.Module):
def __init__(self, d_model, d_ff=2048, dropout = 0.1):
super().__init__()
# d_ff 默认设置为 2048
self.linear_1 = nn.Linear(d_model, d_ff)
self.dropout = nn.Dropout(dropout)
self.linear_2 = nn.Linear(d_ff, d_model)
def forward(self, x):
x = self.dropout(F.relu(self.linear_1(x)))
x = self.linear_2(x)
4、残差连接和层归一化
由 Transformer 结构组成的网络结构通常都是非常庞大。编码器和解码器均由很多层基本的Transformer 块组成,每一层当中都包含复杂的非线性映射,这就导致模型的训练比较困难。因此,研究者们在 Transformer 块中进一步引入了残差连接与层归一化技术以进一步提升训练的稳定性。残差连接能够避免梯度小时,层归一化能够缓解训练过程中潜在的不稳定、收敛速度慢的问题。
残差连接:

层归一化:用于将数据平移缩放为均值为 0 方差为 1 的标准分布

python
class NormLayer(nn.Module):
def __init__(self, d_model, eps = 1e-6):
super().__init__()
self.size = d_model
# 层归一化包含两个可以学习的参数
self.alpha = nn.Parameter(torch.ones(self.size))
self.bias = nn.Parameter(torch.zeros(self.size))
self.eps = eps
def forward(self, x):
norm = self.alpha * (x - x.mean(dim=-1, keepdim=True)) \
/ (x.std(dim=-1, keepdim=True) + self.eps) + self.bias
return norm
5、编码器和解码器结构
解码器比编码器更复杂,解码器的每个 Transformer 的第一个自注意力层额外增加了注意力掩码 mask,对应 2.1 图中掩码多头注意力
主要原因在于,在翻译的过程中,编码器主要用于编码源语言序列的信息,这个序列是完全已知的,所以编码器只需要考虑如何融合上下文语义即可
而解码器负责生成语音序列,这个生成过程是自回归的,对于每个单词生成的过程,只有当前单词之前的单词是可以看到的,所以需要增加额外的掩码来掩盖掉后面的文本信息。
而且解码器还增加了一个多头注意力,使用 cross-attention 的方法同时接收编码器的输出和当前 Transformer 块的前一个掩码注意力层的输出,query 是Transformer 块的前一个掩码注意力层的输出的投影,key 和 value 是编码器输出投影得到的
这个 cross-attention 的作用是在翻译的过程中为了生存合理的目标语言序列需要观测待翻译的源语言序列是什么
基于上述的编码器和解码器结构,待翻译的源语言文本,首先经过编码器端的每个Transformer 块对其上下文语义的层层抽象,最终输出每一个源语言单词上下文相关的表示。解码器端以自回归的方式生成目标语言文本,即在每个时间步 t,根据编码器端输出的源语言文本表示,以及前 t − 1 个时刻生成的目标语言文本,生成当前时刻的目标语言单词。
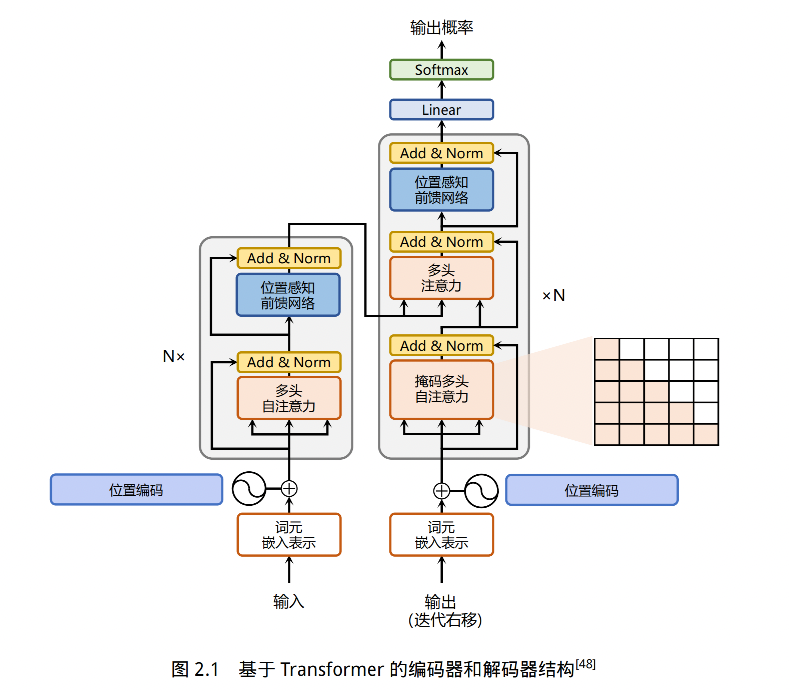
编码器代码:
python
class EncoderLayer(nn.Module):
def __init__(self, d_model, heads, dropout=0.1):
super().__init__()
self.norm_1 = Norm(d_model)
self.norm_2 = Norm(d_model)
self.attn = MultiHeadAttention(heads, d_model, dropout=dropout)
self.ff = FeedForward(d_model, dropout=dropout)
self.dropout_1 = nn.Dropout(dropout)
self.dropout_2 = nn.Dropout(dropout)
def forward(self, x, mask):
x2 = self.norm_1(x)
x = x + self.dropout_1(self.attn(x2,x2,x2,mask))
x2 = self.norm_2(x)
x = x + self.dropout_2(self.ff(x2))
return x
class Encoder(nn.Module):
def __init__(self, vocab_size, d_model, N, heads, dropout):
super().__init__()
self.N = N
self.embed = Embedder(vocab_size, d_model)
self.pe = PositionalEncoder(d_model, dropout=dropout)
self.layers = get_clones(EncoderLayer(d_model, heads, dropout), N)
self.norm = Norm(d_model)
def forward(self, src, mask):
x = self.embed(src)
x = self.pe(x)
for i in range(self.N):
x = self.layers[i](x, mask)
return self.norm(x)
解码器代码:
python
class DecoderLayer(nn.Module):
def __init__(self, d_model, heads, dropout=0.1):
super().__init__()
self.norm_1 = Norm(d_model)
self.norm_2 = Norm(d_model)
self.norm_3 = Norm(d_model)
self.dropout_1 = nn.Dropout(dropout)
self.dropout_2 = nn.Dropout(dropout)
self.dropout_3 = nn.Dropout(dropout)
self.attn_1 = MultiHeadAttention(heads, d_model, dropout=dropout)
self.attn_2 = MultiHeadAttention(heads, d_model, dropout=dropout)
self.ff = FeedForward(d_model, dropout=dropout)
def forward(self, x, e_outputs, src_mask, trg_mask):
x2 = self.norm_1(x)
x = x + self.dropout_1(self.attn_1(x2, x2, x2, trg_mask))
x2 = self.norm_2(x)
x = x + self.dropout_2(self.attn_2(x2, e_outputs, e_outputs, \
src_mask))
x2 = self.norm_3(x)
x = x + self.dropout_3(self.ff(x2))
return x
class Decoder(nn.Module):
def __init__(self, vocab_size, d_model, N, heads, dropout):
super().__init__()
self.N = N
self.embed = Embedder(vocab_size, d_model)
self.pe = PositionalEncoder(d_model, dropout=dropout)
self.layers = get_clones(DecoderLayer(d_model, heads, dropout), N)
self.norm = Norm(d_model)
def forward(self, trg, e_outputs, src_mask, trg_mask):
x = self.embed(trg)
x = self.pe(x)
for i in range(self.N):
x = self.layers[i](x, e_outputs, src_mask, trg_mask)
return self.norm(x)
整体结构:
python
class Transformer(nn.Module):
def __init__(self, src_vocab, trg_vocab, d_model, N, heads, dropout):
super().__init__()
self.encoder = Encoder(src_vocab, d_model, N, heads, dropout)
self.decoder = Decoder(trg_vocab, d_model, N, heads, dropout)
self.out = nn.Linear(d_model, trg_vocab)
def forward(self, src, trg, src_mask, trg_mask):
e_outputs = self.encoder(src, src_mask)
d_output = self.decoder(trg, e_outputs, src_mask, trg_mask)
output = self.out(d_output)
return output
训练和测试:
python
d_model = 512
heads = 8
N = 6
src_vocab = len(EN_TEXT.vocab)
trg_vocab = len(FR_TEXT.vocab)
model = Transformer(src_vocab, trg_vocab, d_model, N, heads)
for p in model.parameters():
if p.dim() > 1:
nn.init.xavier_uniform_(p)
optim = torch.optim.Adam(model.parameters(), lr=0.0001, betas=(0.9, 0.98), eps=1e-9)
# 模型训练
def train_model(epochs, print_every=100):
model.train()
start = time.time()
temp = start
total_loss = 0
for epoch in range(epochs):
for i, batch in enumerate(train_iter):
src = batch.English.transpose(0,1)
trg = batch.French.transpose(0,1)
# the French sentence we input has all words except
# the last, as it is using each word to predict the next
trg_input = trg[:, :-1]
# the words we are trying to predict
targets = trg[:, 1:].contiguous().view(-1)
# create function to make masks using mask code above
src_mask, trg_mask = create_masks(src, trg_input)
preds = model(src, trg_input, src_mask, trg_mask)
optim.zero_grad()
loss = F.cross_entropy(preds.view(-1, preds.size(-1)),
results, ignore_index=target_pad)
loss.backward()
optim.step()
total_loss += loss.data[0]
if (i + 1) % print_every == 0:
loss_avg = total_loss / print_every
print("time = %dm, epoch %d, iter = %d, loss = %.3f,
%ds per %d iters" % ((time.time() - start) // 60,
epoch + 1, i + 1, loss_avg, time.time() - temp,
print_every))
total_loss = 0
temp = time.time()
# 模型测试
def translate(model, src, max_len = 80, custom_string=False):
model.eval()
if custom_sentence == True:
src = tokenize_en(src)
sentence=Variable(torch.LongTensor([[EN_TEXT.vocab.stoi[tok] for tok in sentence]])).cuda()
src_mask = (src != input_pad).unsqueeze(-2)
e_outputs = model.encoder(src, src_mask)
outputs = torch.zeros(max_len).type_as(src.data)
outputs[0] = torch.LongTensor([FR_TEXT.vocab.stoi['<sos>']])
for i in range(1, max_len):
trg_mask = np.triu(np.ones((1, i, i),
k=1).astype('uint8')
trg_mask= Variable(torch.from_numpy(trg_mask) == 0).cuda()
out = model.out(model.decoder(outputs[:i].unsqueeze(0),
e_outputs, src_mask, trg_mask))
out = F.softmax(out, dim=-1)
val, ix = out[:, -1].data.topk(1)
outputs[i] = ix[0][0]
if ix[0][0] == FR_TEXT.vocab.stoi['<eos>']:
break
return ' '.join([FR_TEXT.vocab.itos[ix] for ix in outputs[:i]])
2.2 生成式预训练语言模型 GPT
Generative Pre-Training,生成式语言模型,也叫 GPT,结构如图 2.3,由多层 Transformer 组成的单向语言模型,包括输入层、编码层、输出层
2.2.1 无监督预训练
GPT 采用的是生成式预训练方法,单向就是说模型只能从左到右或从右到左对文本序列建模
给定文本序列 w = w 1 w 2 . . . w n w=w_1w_2...w_n w=w1w2...wn,GPT 首先将其映射为稠密向量:

- v i t v_i^t vit:词 w i w_i wi 的词向量
- v i p v_i^p vip:词 w i w_i wi 的位置向量
- v i v_i vi:第 i 个位置的单词经过模型输入后的输入
经过输入层编码,模型得到表示向量序列 v = v 1 . . . v n v=v_1 ... v_n v=v1...vn,然后将 v 送入模型编码层(L 个 Transformer 模块),GPT 能得到每个单词层次化的组合式表示:

GPT 模型的输出层基于最后一层的表示 h ( L ) h^{(L)} h(L) 来预测每个位置上的条件概率,其计算过程可以表示为:

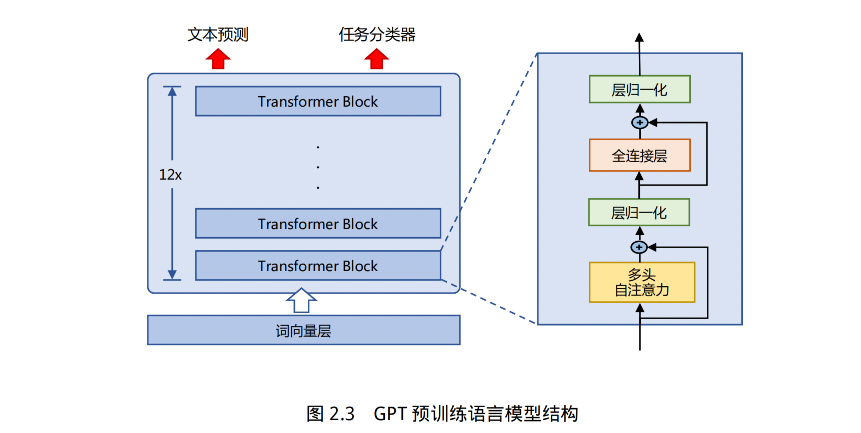
优化的目标函数:
单向语言模型时按照阅读顺序输入文本序列 w,用常规语言模型目标来优化 w 的最大似然估计,使之能根据输入历史序列对当前词能做出准确的预测

2.2.2 有监督下游任务微调
通过预训练阶段,GPT 模型就具有了一定的通用语义表示能力,下游任务微调的目的是在通用语义的基础上,根据下游任务的特性进行适配
下游任务需要利用有标注的数据集来训练,每个数据样例由长度为 n 的文本序列 x = x 1 x 2 . . . x n x=x_1x_2...x_n x=x1x2...xn 和对应的标签 y 构成
首先将文本序列 x 输入 GPT 模型,获得最后一层的最后一个词所对应的隐藏层输入 h n ( L ) h_n^{(L)} hn(L),再次基础上通过全连接和 softmax 得到标签预测结果:

下游任务的目标函数如下:

下游任务在微调过程中,针对目标任务优化时,容易使得模型遗忘预训练阶段学习到的通用语义信息,损失模型的通用性和泛化性,造成灾难性遗忘
因此,通常会采用混合预训练任务损失和下游微调损失的方法来缓解这个问题,一般使用这样的结合方式来微调下游任务:

2.2.3 基于 HuggingFace 的预训练语言模型实战
1、数据集合准备
python
from datasets import concatenate_datasets, load_dataset
bookcorpus = load_dataset("bookcorpus", split="train")
wiki = load_dataset("wikipedia", "20230601.en", split="train")
# 仅保留 'text' 列
wiki = wiki.remove_columns([col for col in wiki.column_names if col != "text"])
dataset = concatenate_datasets([bookcorpus, wiki])
# 将数据集合切分为 90% 用于训练,10% 用于测试
d = dataset.train_test_split(test_size=0.1)
将训练和测试数据保存本地
python
def dataset_to_text(dataset, output_filename="data.txt"):
"""Utility function to save dataset text to disk,
useful for using the texts to train the tokenizer
(as the tokenizer accepts files)"""
with open(output_filename, "w") as f:
for t in dataset["text"]:
print(t, file=f)
# save the training set to train.txt
dataset_to_text(d["train"], "train.txt")
# save the testing set to test.txt
dataset_to_text(d["test"], "test.txt")
2、训练词元分析器(tokenizer)
BERT 采用了 WordPiece 分词,根据训练语料中的词频决定是否将一个完整的词切分为多个词元。因此,需要首先训练词元分析器(Tokenizer)。可以使用 transformers 库中的 BertWordPieceTokenizer 类来完成任务,代码如下所示:
python
special_tokens = [
"[PAD]", "[UNK]", "[CLS]", "[SEP]", "[MASK]", "<S>", "<T>"
]
# if you want to train the tokenizer on both sets
# files = ["train.txt", "test.txt"]
# training the tokenizer on the training set
files = ["train.txt"]
# 30,522 vocab is BERT's default vocab size, feel free to tweak
vocab_size = 30_522
# maximum sequence length, lowering will result to faster training (when increasing batch size)
max_length = 512
# whether to truncate
truncate_longer_samples = False
# initialize the WordPiece tokenizer
tokenizer = BertWordPieceTokenizer()
# train the tokenizer
tokenizer.train(files=files, vocab_size=vocab_size, special_tokens=special_tokens)
# enable truncation up to the maximum 512 tokens
tokenizer.enable_truncation(max_length=max_length)
model_path = "pretrained-bert"
# make the directory if not already there
if not os.path.isdir(model_path):
os.mkdir(model_path)
# save the tokenizer
tokenizer.save_model(model_path)
# dumping some of the tokenizer config to config file,
# including special tokens, whether to lower case and the maximum sequence length
with open(os.path.join(model_path, "config.json"), "w") as f:
tokenizer_cfg = {
"do_lower_case": True,
"unk_token": "[UNK]",
"sep_token": "[SEP]",
"pad_token": "[PAD]",
"cls_token": "[CLS]",
"mask_token": "[MASK]",
"model_max_length": max_length,
"max_len": max_length,
}
json.dump(tokenizer_cfg, f)
# when the tokenizer is trained and configured, load it as BertTokenizerFast
tokenizer = BertTokenizerFast.from_pretrained(model_path)
3、预处理预料集合
在启动整个模型训练之前,还需要将预训练语料根据训练好的 Tokenizer 进行处理。如果文档长度超过 512 个词元(Token),那么就直接进行截断。数据处理代码如下所示:
python
def encode_with_truncation(examples):
"""Mapping function to tokenize the sentences passed with truncation"""
return tokenizer(examples["text"], truncation=True, padding="max_length",
max_length=max_length, return_special_tokens_mask=True)
def encode_without_truncation(examples):
"""Mapping function to tokenize the sentences passed without truncation"""
return tokenizer(examples["text"], return_special_tokens_mask=True)
# the encode function will depend on the truncate_longer_samples variable
encode = encode_with_truncation if truncate_longer_samples else encode_without_truncation
# tokenizing the train dataset
train_dataset = d["train"].map(encode, batched=True)
# tokenizing the testing dataset
test_dataset = d["test"].map(encode, batched=True)
if truncate_longer_samples:
# remove other columns and set input_ids and attention_mask as PyTorch tensors
train_dataset.set_format(type="torch", columns=["input_ids", "attention_mask"])
test_dataset.set_format(type="torch", columns=["input_ids", "attention_mask"])
else:
# remove other columns, and remain them as Python lists
test_dataset.set_format(columns=["input_ids", "attention_mask", "special_tokens_mask"])
train_dataset.set_format(columns=["input_ids", "attention_mask", "special_tokens_mask"])
truncate_longer_samples 布尔变量来控制用于对数据集进行词元处理的 encode() 回调函数。如果设置为 True,则会截断超过最大序列长度(max_length)的句子。否则,不会截断。如果设为truncate_longer_samples 为 False,需要将没有截断的样本连接起来,并组合成固定长度的向量。
python
from itertools import chain
# Main data processing function that will concatenate all texts from our dataset
# and generate chunks of max_seq_length.
def group_texts(examples):
# Concatenate all texts.
concatenated_examples = {k: list(chain(*examples[k])) for k in examples.keys()}
total_length = len(concatenated_examples[list(examples.keys())[0]])
# We drop the small remainder, we could add padding if the model supported it instead of
# this drop, you can customize this part to your needs.
if total_length >= max_length:
total_length = (total_length // max_length) * max_length
# Split by chunks of max_len.
result = {
k: [t[i : i + max_length] for i in range(0, total_length, max_length)]
for k, t in concatenated_examples.items()
}
return result
# Note that with `batched=True`, this map processes 1,000 texts together, so group_texts throws
# away a remainder for each of those groups of 1,000 texts. You can adjust that batch_size here but
# a higher value might be slower to preprocess.
#
# To speed up this part, we use multiprocessing. See the documentation of the map method
#for more information:
# https://huggingface.co/docs/datasets/package_reference/main_classes.html#datasets.Dataset.map
if not truncate_longer_samples:
train_dataset = train_dataset.map(group_texts, batched=True,
desc=f"Grouping texts in chunks of {max_length}")
test_dataset = test_dataset.map(group_texts, batched=True,
desc=f"Grouping texts in chunks of {max_length}")
# convert them from lists to torch tensors
train_dataset.set_format("torch")
test_dataset.set_format("torch")
4、模型训练
python
# initialize the model with the config
model_config = BertConfig(vocab_size=vocab_size, max_position_embeddings=max_length)
model = BertForMaskedLM(config=model_config)
# initialize the data collator, randomly masking 20% (default is 15%) of the tokens
# for the Masked Language Modeling (MLM) task
data_collator = DataCollatorForLanguageModeling(
tokenizer=tokenizer, mlm=True, mlm_probability=0.2
)
training_args = TrainingArguments(
output_dir=model_path, # output directory to where save model checkpoint
evaluation_strategy="steps", # evaluate each `logging_steps` steps
overwrite_output_dir=True,
num_train_epochs=10, # number of training epochs, feel free to tweak
per_device_train_batch_size=10, # the training batch size, put it as high as your GPU memory fits
gradient_accumulation_steps=8, # accumulating the gradients before updating the weights
per_device_eval_batch_size=64, # evaluation batch size
logging_steps=1000, # evaluate, log and save model checkpoints every 1000 step
save_steps=1000,
# load_best_model_at_end=True, # whether to load the best model (in terms of loss)
# at the end of training
# save_total_limit=3, # whether you don't have much space so you
# let only 3 model weights saved in the disk
)
trainer = Trainer(
model=model,
args=training_args,
data_collator=data_collator,
train_dataset=train_dataset,
eval_dataset=test_dataset,
)
# train the model
trainer.train()
5、模型使用
python
# load the model checkpoint
model = BertForMaskedLM.from_pretrained(os.path.join(model_path, "checkpoint-10000"))
# load the tokenizer
tokenizer = BertTokenizerFast.from_pretrained(model_path)
fill_mask = pipeline("fill-mask", model=model, tokenizer=tokenizer)
# perform predictions
examples = [
"Today's most trending hashtags on [MASK] is Donald Trump",
"The [MASK] was cloudy yesterday, but today it's rainy.",
]
for example in examples:
for prediction in fill_mask(example):
print(f"{prediction['sequence']}, confidence: {prediction['score']}")
print("="*50)
2.3 大语言模型结构
当前绝大多数大语言模型的结构都类似于 GPT,使用基于 Transformer 架构构造的仅由解码器组成的网络结构,采用自回归的方式构建语言模型。但是在位置编码、层归一化位置以及激活函数等细节上各有不同。
由于 GPT-3 并没有开放源代码,因此文献 [31] 介绍了根据 GPT-3 的描述复现的过程,并构造开源了系统 OPT(Open
Pre-trained Transformer Language Models)。
Meta AI 也仿照 GPT-3 架构开源了 LLaMA 模型[37],下面将以 LLaMA 为例来讲解结构
LLaMA 的结构和 gpt-2 类似,使用了前置归一化层,归一化函数为 RMSNorm,激活函数为 SwiGLU,使用了旋转位置嵌入 RoP
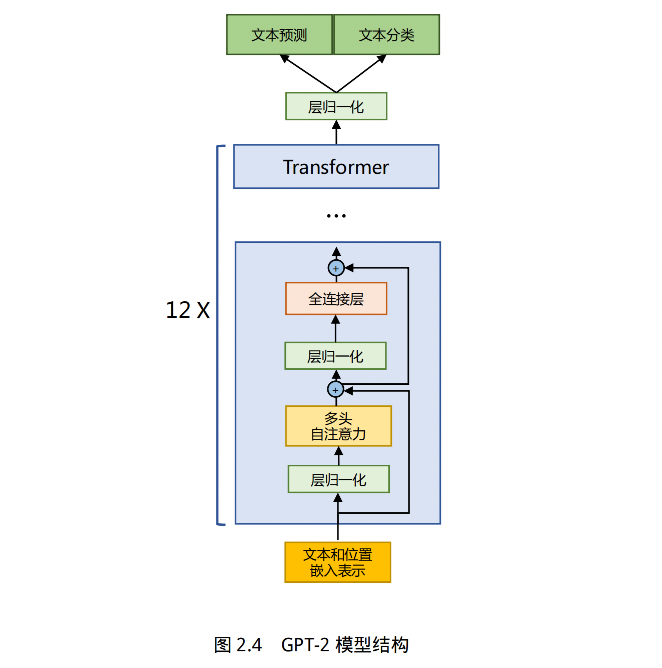
三、语言模型的训练数据
3.1 数据来源
OpenAI 训练 GPT-3 所使用的主要数据来源,包含经过过滤的 CommonCrawl 数据集[19]、WebText2、Books1、Books2 以及英文 wikipedia 等数据集合。
- 其中 CommonCrawl 的原始数据有 45TB,进行过滤后仅保留了 570GB 的数据。通过词元方式对上述语料进行切分,大约一共包含 5000 亿词元。为了保证模型使用更多高质量数据进行训练,
- 在 GPT-3 训练时,根据语料来源的不同,设置不同的采样权重。在完成 3000 亿词元训练时,英文 Wikipedia 的语料平均训练轮数为 3.4 次,而 CommonCrawl 和 Books 2 仅有 0.44 次和 0.43 次。
- 由于 CommonCrawl 数据集合的过滤过程繁琐复杂,Meta 公司的研究人员在训练 OPT[31] 模型时则采用了混合 RoBERTa[67]、Pile[68] 和 PushShift.io Reddit[69] 数据的方法。
- 由于这些数据集合中包含的绝大部分都是英文数据,因此 OPT 也从 CommonCrawl 数据集中抽取了部分非英文数据加入训练语料。
大语言模型训练所需的数据来源大体上可以分为通用数据和专业数据两大类:
- 通用数据(General Data):
- 包括网页、图书、新闻、对话文本等内容[14, 31, 46]
- 通用数据具有规模大、多样性和易获取等特点,因此可以支持大语言模型的构建语言建模和泛化能力
专业数据(Specialized Data): - 包括多语言数据、科学数据、代码以及领域特有资料等数据。
- 通过在预训练阶段引入专业数据可以有效提供大语言模型的任务解决能力。
图3.1给出了一些典型大语言模型所使用数量类型的分布情况。可以看到不同的大语言模型在训练类型分布上的差距很大,
3.2 数据处理
大语言模型的相关研究表明,数据质量对于模型的影响非常大。因此在收集到各类型数据之后,需要对数据进行处理,去除低质量数据、重复数据、有害信息、个人隐私等内容[14, 85]。典型的数据处理过程如图3.2所示,主要包含质量过滤、冗余去除、隐私消除、词元切分等几个步骤
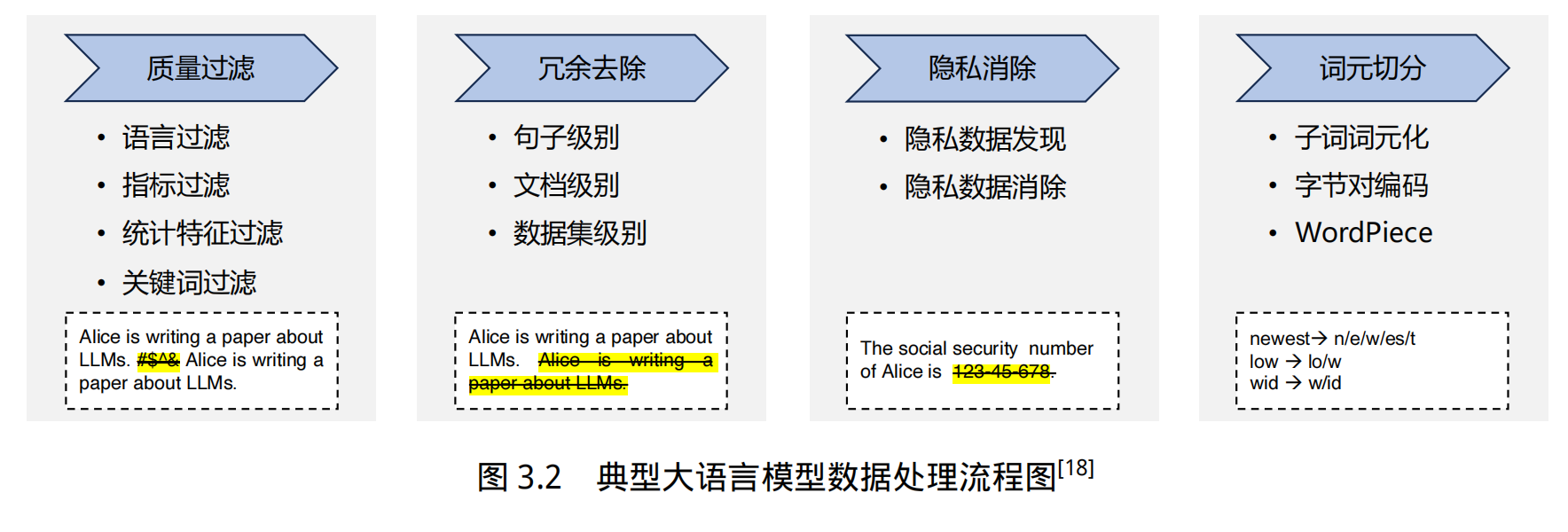
词元切分:
- 传统的自然语言处理通常以单词为基本处理单元,模型都依赖预先确定的词表 V,在编码输入词序列时,这些词表示模型只能处理词表中存在的词。
- 因此,在使用中,如果遇到不在词表中的未登录词,模型无法为其生成对应的表示,只能给予这些未登录词(Out-of-vocabulary,OOV)一个默认的通用表示。
- 在深度学习模型中,词表示模型会预先在词表中加入一个默认的"[UNK]"(unknown)标识,表示未知词,并在训练的过程中将 [UNK] 的向量作为词表示矩阵的一部分一起训练,通过引入某些相应机制来更新 [UNK] 向量的参数。
- 在使用时,对于全部的未登录词,都使用 [UNK] 的向量作为这些词的表示向量。
词表大小的影响:
- 基于固定词表的词表示模型对词表大小的选择比较敏感
- 词表大小过小时,未登录词的比例较高,影响模型性能
- 词表大小过大时,大量低频词出现在词表中,而这些词的词向量很难得到充分学习。
- 理想模式下,词表示模型应能覆盖绝大部分的输入词,并避免词表过大所造成的数据稀疏问题。
数据的质量、数据的丰富性都会影响模型的效果,且模型参数量大小和数据量大小是由一定关系的
四、并行训练
DeepSeed 架构
五、有监督微调
有监督微调(Supervised Finetuning, SFT)又称指令微调(Instruction Tuning),是指在已经训练好的语言模型的基础上,通过使用有标注的特定任务数据进行进一步的微调,从而使得模型具备遵循指令的能力。
经过海量数据预训练后的语言模型虽然具备了大量的"知识",但是由于其训练时的目标仅是进行下一个词的预测,此时的模型还不能够理解并遵循人类自然语言形式的指令。
为了能够使得模型具有理解并响应人类指令的能力,还需要使用指令数据对其进行微调。指令数据如何构造,如何高效低成本地进行指令微调训练,以及如何在语言模型基础上进一步扩大上下文等问题是大语言模型在有监督微调阶段所关注的核心。
5.1 提示学习和语境学习
5.2 高效模型微调
5.2.1 LoRA
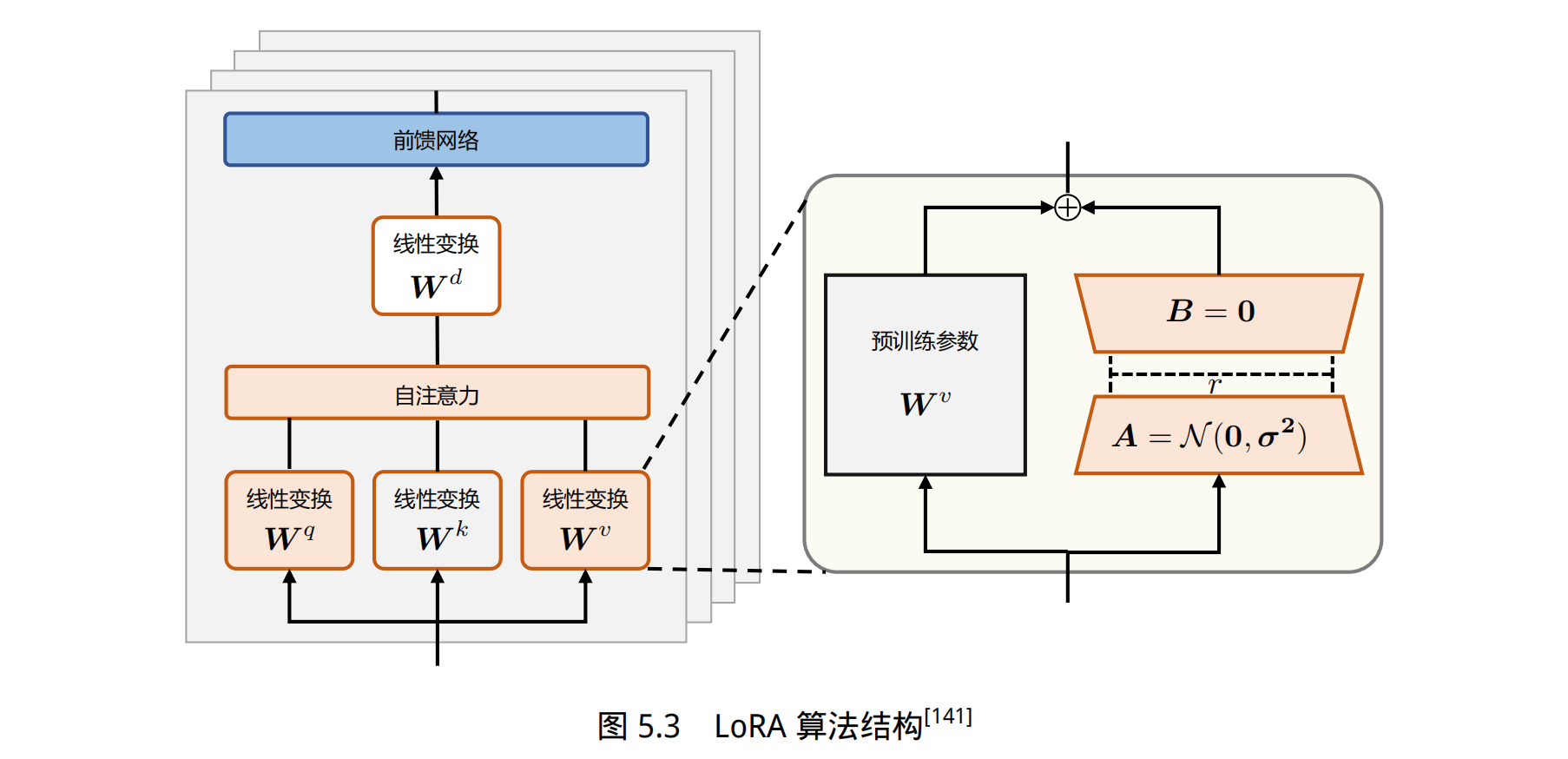
语言模型针对特定任务微调之后,权重矩阵通常具有很低的本征秩(Intrinsic Rank)。研究人员认为参数更新量即便投影到较小的子空间中,也不会影响学习的有效性[140]。因此,提出固定预训练模型参数不变,在原本权重矩阵旁路添加低秩矩阵的乘积作为可训练参数,用以模拟参数的变化量。
peft 库中含有包括 LoRA 在内的多种高效微调方法,且与 transformer 库兼容。使用示例如下所示。其中,lora_alpha(α)表示放缩系数。表示参数更新量的 ∆W 会与 α/r 相乘后再与原本的模型参数相加。
5.2.2 LoRA 的变体
AdaLoRA
QLoRA