一、背景
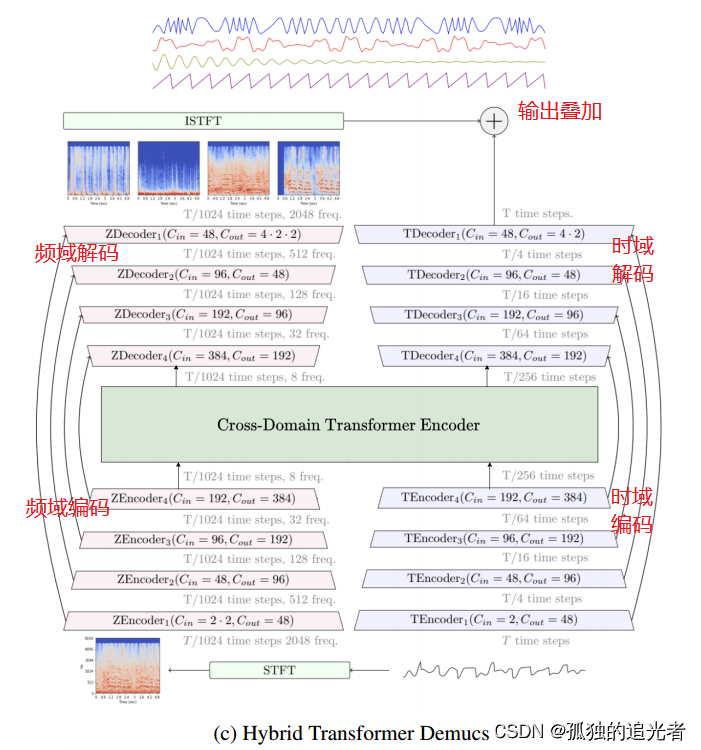
1、代码复现|Demucs Music Source Separation_demucs架构原理-CSDN博客
2、Hybrid Transformer 各个模块对应的代码具体在工程的哪个地方
3、Hybrid Transformer 各个模块的底层到底是个啥(初步感受)?
4、Hybrid Transformer 各个模块处理后,数据的维度大小是咋变换的?
从模块上划分,Hybrid Transformer Demucs 共包含 (STFT模块、时域编码模块、频域编码模块、Cross-Domain Transformer Encoder模块、时域解码模块、频域解码模块、ISTFT模块)7个模块。已完成解读:STFT模块、频域编码模块(时域编码和频域编码类似,后续不再解读时域编码模块),待解读:Cross-Domain Transformer Encoder模块。
本篇目标:拆解****频域解码模块、ISTFT模块的底层。时域解码和频域解码原理类似(后续不再拆解时域解码模块)。
二、频域解码模块
python
class HDecLayer(nn.Module):
def __init__(self, chin, chout, last=False, kernel_size=8, stride=4, norm_groups=1, empty=False,
freq=True, dconv=True, norm=True, context=1, dconv_kw={}, pad=True,
context_freq=True, rewrite=True):
"""
Same as HEncLayer but for decoder. See `HEncLayer` for documentation.
"""
super().__init__()
norm_fn = lambda d: nn.Identity() # noqa
if norm:
norm_fn = lambda d: nn.GroupNorm(norm_groups, d) # noqa
if pad:
pad = kernel_size // 4
else:
pad = 0
self.pad = pad
self.last = last
self.freq = freq
self.chin = chin
self.empty = empty
self.stride = stride
self.kernel_size = kernel_size
self.norm = norm
self.context_freq = context_freq
klass = nn.Conv1d
klass_tr = nn.ConvTranspose1d
if freq:
kernel_size = [kernel_size, 1]
stride = [stride, 1]
klass = nn.Conv2d
klass_tr = nn.ConvTranspose2d
self.conv_tr = klass_tr(chin, chout, kernel_size, stride)
self.norm2 = norm_fn(chout)
if self.empty:
return
self.rewrite = None
if rewrite:
if context_freq:
self.rewrite = klass(chin, 2 * chin, 1 + 2 * context, 1, context)
else:
self.rewrite = klass(chin, 2 * chin, [1, 1 + 2 * context], 1,[0, context])
self.norm1 = norm_fn(2 * chin)
self.dconv = None
if dconv:
self.dconv = DConv(chin, **dconv_kw)
def forward(self, x, skip, length):
if self.freq and x.dim() == 3:
B, C, T = x.shape
x = x.view(B, self.chin, -1, T)
if not self.empty:
x = x + skip
if self.rewrite:
y = F.glu(self.norm1(self.rewrite(x)), dim=1)
else:
y = x
if self.dconv:
if self.freq:
B, C, Fr, T = y.shape
y = y.permute(0, 2, 1, 3).reshape(-1, C, T)
y = self.dconv(y)
if self.freq:
y = y.view(B, Fr, C, T).permute(0, 2, 1, 3)
else:
y = x
assert skip is None
z = self.norm2(self.conv_tr(y))
print('self.pad,self.last:', self.pad,self.last)
if self.freq:
if self.pad:
z = z[..., self.pad:-self.pad, :]
else:
z = z[..., self.pad:self.pad + length]
assert z.shape[-1] == length, (z.shape[-1], length)
if not self.last:
z = F.gelu(z)
return z, y
频域解码模块的核心代码如上所示。在上一篇频域编码模块的基础上,继续贴出完善之后的频域编解码模块全景图。
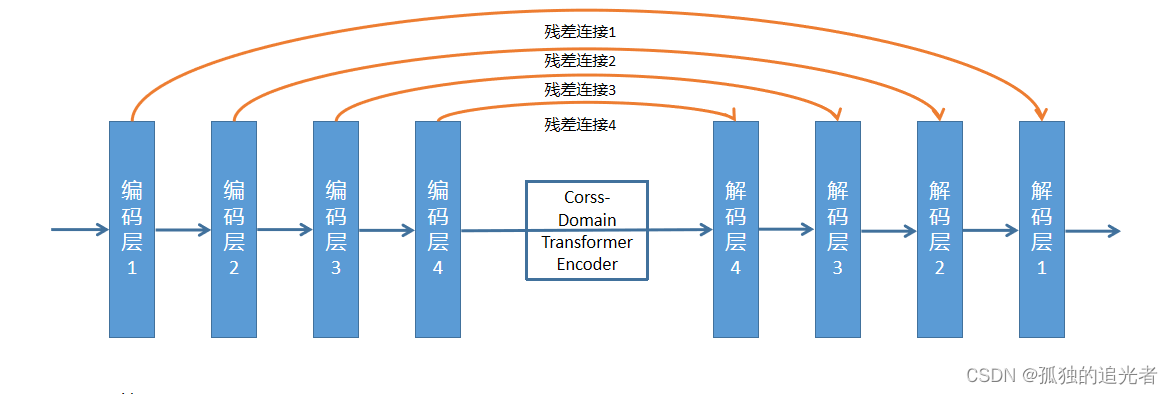
编码层:Conv2d+Norm1+GELU, Norm1:Identity()
解码层:(Conv2d+Norm1+GLU)+(ConvTranspose2d+Norm2+倒数第二个维度裁剪+GELU), Norm1\Norm2:Identity()
残差连接:(Conv1d+GroupNorm+GELU +Conv1d+GroupNorm+GLU+LayerScale())+(Conv2d+Norm2+GLU),Norm2:Identity() ,备注:Identity可以理解成直通
python
#频域编码层1-4的Conv2d分别是:
Conv2d(4, 48, kernel_size=(8, 1), stride=(4, 1), padding=(2, 0))
Conv2d(48, 96, kernel_size=(8, 1), stride=(4, 1), padding=(2, 0))
Conv2d(96, 192, kernel_size=(8, 1), stride=(4, 1), padding=(2, 0))
Conv2d(192, 384, kernel_size=(8, 1), stride=(4, 1), padding=(2, 0))
#频域解码层4-1的Conv2d和ConvTranspose2d
Conv2d(384, 768, kernel_size=(3, 3), stride=(1, 1), padding=(1, 1))
ConvTranspose2d(384, 192, kernel_size=(8, 1), stride=(4, 1))
Conv2d(192, 384, kernel_size=(3, 3), stride=(1, 1), padding=(1, 1))
ConvTranspose2d(192, 96, kernel_size=(8, 1), stride=(4, 1))
Conv2d(96, 192, kernel_size=(3, 3), stride=(1, 1), padding=(1, 1))
ConvTranspose2d(96, 48, kernel_size=(8, 1), stride=(4, 1))
Conv2d(48, 96, kernel_size=(3, 3), stride=(1, 1), padding=(1, 1))
ConvTranspose2d(48, 16, kernel_size=(8, 1), stride=(4, 1))
残差连接模块如下所示。
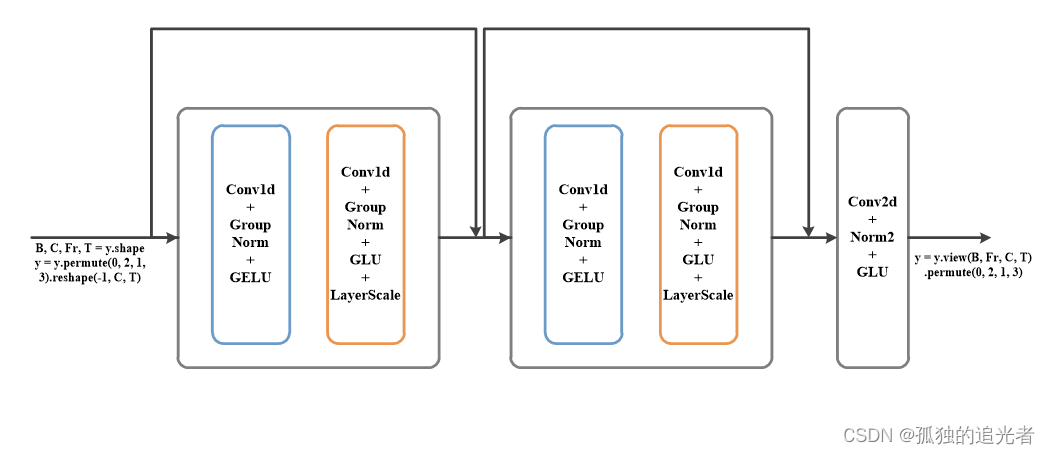
python
#残差连接1
DConv(
(layers): ModuleList(
(0): Sequential(
(0): Conv1d(48, 6, kernel_size=(3,), stride=(1,), padding=(1,))
(1): GroupNorm(1, 6, eps=1e-05, affine=True)
(2): GELU(approximate=none)
(3): Conv1d(6, 96, kernel_size=(1,), stride=(1,))
(4): GroupNorm(1, 96, eps=1e-05, affine=True)
(5): GLU(dim=1)
(6): LayerScale()
)
(1): Sequential(
(0): Conv1d(48, 6, kernel_size=(3,), stride=(1,), padding=(2,), dilation=(2,))
(1): GroupNorm(1, 6, eps=1e-05, affine=True)
(2): GELU(approximate=none)
(3): Conv1d(6, 96, kernel_size=(1,), stride=(1,))
(4): GroupNorm(1, 96, eps=1e-05, affine=True)
(5): GLU(dim=1)
(6): LayerScale()
)
)
)
Conv2d(48, 96, kernel_size=(1, 1), stride=(1, 1))
#残差连接2
DConv(
(layers): ModuleList(
(0): Sequential(
(0): Conv1d(96, 12, kernel_size=(3,), stride=(1,), padding=(1,))
(1): GroupNorm(1, 12, eps=1e-05, affine=True)
(2): GELU(approximate=none)
(3): Conv1d(12, 192, kernel_size=(1,), stride=(1,))
(4): GroupNorm(1, 192, eps=1e-05, affine=True)
(5): GLU(dim=1)
(6): LayerScale()
)
(1): Sequential(
(0): Conv1d(96, 12, kernel_size=(3,), stride=(1,), padding=(2,), dilation=(2,))
(1): GroupNorm(1, 12, eps=1e-05, affine=True)
(2): GELU(approximate=none)
(3): Conv1d(12, 192, kernel_size=(1,), stride=(1,))
(4): GroupNorm(1, 192, eps=1e-05, affine=True)
(5): GLU(dim=1)
(6): LayerScale()
)
)
)
Conv2d(96, 192, kernel_size=(1, 1), stride=(1, 1))
#残差连接3
DConv(
(layers): ModuleList(
(0): Sequential(
(0): Conv1d(192, 24, kernel_size=(3,), stride=(1,), padding=(1,))
(1): GroupNorm(1, 24, eps=1e-05, affine=True)
(2): GELU(approximate=none)
(3): Conv1d(24, 384, kernel_size=(1,), stride=(1,))
(4): GroupNorm(1, 384, eps=1e-05, affine=True)
(5): GLU(dim=1)
(6): LayerScale()
)
(1): Sequential(
(0): Conv1d(192, 24, kernel_size=(3,), stride=(1,), padding=(2,), dilation=(2,))
(1): GroupNorm(1, 24, eps=1e-05, affine=True)
(2): GELU(approximate=none)
(3): Conv1d(24, 384, kernel_size=(1,), stride=(1,))
(4): GroupNorm(1, 384, eps=1e-05, affine=True)
(5): GLU(dim=1)
(6): LayerScale()
)
)
)
Conv2d(192, 384, kernel_size=(1, 1), stride=(1, 1))
#残差连接4
DConv(
(layers): ModuleList(
(0): Sequential(
(0): Conv1d(384, 48, kernel_size=(3,), stride=(1,), padding=(1,))
(1): GroupNorm(1, 48, eps=1e-05, affine=True)
(2): GELU(approximate=none)
(3): Conv1d(48, 768, kernel_size=(1,), stride=(1,))
(4): GroupNorm(1, 768, eps=1e-05, affine=True)
(5): GLU(dim=1)
(6): LayerScale()
)
(1): Sequential(
(0): Conv1d(384, 48, kernel_size=(3,), stride=(1,), padding=(2,), dilation=(2,))
(1): GroupNorm(1, 48, eps=1e-05, affine=True)
(2): GELU(approximate=none)
(3): Conv1d(48, 768, kernel_size=(1,), stride=(1,))
(4): GroupNorm(1, 768, eps=1e-05, affine=True)
(5): GLU(dim=1)
(6): LayerScale()
)
)
)
Conv2d(384, 768, kernel_size=(1, 1), stride=(1, 1))
三、ISTFT模块
ISTFT模块的核心代码如下所示。
python
import torch as th
def ispectro(z, hop_length=None, length=None, pad=0):
*other, freqs, frames = z.shape
n_fft = 2 * freqs - 2
z = z.view(-1, freqs, frames)
win_length = n_fft // (1 + pad)
is_mps = z.device.type == 'mps'
if is_mps:
z = z.cpu()
x = th.istft(z,
n_fft,
hop_length,
window=th.hann_window(win_length).to(z.real),
win_length=win_length,
normalized=True,
length=length,
center=True)
_, length = x.shape
return x.view(*other, length)
其中,torch.istft【逆短时傅里叶变换(Inverse Short Time Fourier Transform,ISTFT)】,该函数期望是torch.stft函数的逆过程。它具有相同的参数(加上一个可选参数length),并且应该返回原始信号的最小二乘估计。算法将根据NOLA条件(非零重叠)进行检查。
torch.istft接口参数####
input (Tensor): 输入张量,期望是`torch.stft`的输出,可以是复数形式(`channel`, `fft_size`, `n_frame`),或者是实数形式(`channel`, `fft_size`, `n_frame`, 2),其中`channel`维度是可选的。
deprecated:: 1.8.0
实数输入已废弃,请使用`stft(..., return_complex=True)`返回的复数输入代替。
n_fft (int) : 傅里叶变换的大小。
hop_length (Optional[int]) : 相邻滑动窗口帧之间的距离。(默认:`n_fft // 4`)
win_length (Optional[int]) : 窗口帧和STFT滤波器的大小。(默认:`n_fft`)
window (Optional[torch.Tensor]) : 可选的窗函数。(默认:`torch.ones(win_length)`)
center (bool) : 指示输入是否在两边进行了填充,使得第`t`帧位于时间`t × hop_length`处居中。(默认:`True`)
normalized (bool) : 指示STFT是否被标准化。(默认:`False`)
onesided (Optional[bool]) : 指示STFT是否为单边谱。(默认:如果输入尺寸中的`n_fft != fft_size`则为`True`)
length (Optional[int]) : 修剪信号的长度,即原始信号的长度。(默认:整个信号)
return_complex (Optional[bool]):指示输出是否应为复数,或者输入是否应假定源自实信号和窗函数。注意,这与`onesided=True`不兼容。(默认:`False`)
频域解码模块和ISTFT模块解读完毕。还剩一个Cross-Domain Transformer Encoder模块没有解读。后面又来新的活了,希望能把demucs落地~。
感谢阅读,最近开始写公众号(分享好用的AI工具),欢迎大家一起见证我的成长(桂圆学AI)