文章目录
-
-
- 引言
- [第一章 半监督学习的基本概念](#第一章 半监督学习的基本概念)
-
- [1.1 什么是半监督学习](#1.1 什么是半监督学习)
- [1.2 半监督学习的优势](#1.2 半监督学习的优势)
- [第二章 半监督学习的核心算法](#第二章 半监督学习的核心算法)
-
- [2.1 自训练(Self-Training)](#2.1 自训练(Self-Training))
- [2.2 协同训练(Co-Training)](#2.2 协同训练(Co-Training))
- [2.3 图半监督学习(Graph-Based Semi-Supervised Learning)](#2.3 图半监督学习(Graph-Based Semi-Supervised Learning))
- [第三章 半监督学习的应用实例](#第三章 半监督学习的应用实例)
-
- [3.1 图像分类](#3.1 图像分类)
- [3.2 文本分类](#3.2 文本分类)
- [第四章 半监督学习的未来发展与挑战](#第四章 半监督学习的未来发展与挑战)
-
- [4.1 标签质量与模型鲁棒性](#4.1 标签质量与模型鲁棒性)
- [4.2 多视角与多模态学习](#4.2 多视角与多模态学习)
- [4.3 标注策略与主动学习](#4.3 标注策略与主动学习)
- 结论
-
引言
半监督学习(Semi-Supervised Learning)是一类机器学习方法,通过结合少量有标签数据和大量无标签数据来进行学习。相比于纯监督学习,半监督学习在标签数据稀缺的情况下能更有效地利用无标签数据,提高模型的泛化能力和预测准确性。本文将深入探讨半监督学习的基本原理、核心算法及其在实际中的应用,并提供代码示例以帮助读者更好地理解和掌握这一技术。
第一章 半监督学习的基本概念
1.1 什么是半监督学习
半监督学习是一种介于监督学习和无监督学习之间的方法,通过同时利用有标签和无标签数据进行训练。在许多实际应用中,获取大量有标签数据的成本高昂,而无标签数据通常比较丰富。半监督学习方法能够在这样的环境中有效发挥作用。
1.2 半监督学习的优势
半监督学习相比于纯监督学习具有以下优势:
- 减少标注成本:通过利用大量无标签数据,可以显著减少对有标签数据的依赖,从而降低数据标注成本。
- 提高模型性能:在有标签数据稀缺的情况下,通过引入无标签数据,可以提高模型的泛化能力和预测准确性。
- 更好地利用数据:充分利用已有的无标签数据,避免数据浪费,提升模型的整体表现。
第二章 半监督学习的核心算法
2.1 自训练(Self-Training)
自训练是一种简单但有效的半监督学习方法,通过使用有标签数据训练初始模型,然后利用该模型对无标签数据进行预测,将预测结果置信度高的无标签数据作为新的有标签数据,加入训练集中,反复迭代直到模型收敛。
python
import numpy as np
from sklearn.datasets import load_iris
from sklearn.model_selection import train_test_split
from sklearn.ensemble import RandomForestClassifier
from sklearn.metrics import accuracy_score
# 加载数据集
iris = load_iris()
X, y = iris.data, iris.target
# 划分有标签数据和无标签数据
X_train, X_unlabeled, y_train, _ = train_test_split(X, y, test_size=0.7, random_state=42)
X_unlabeled, X_test, _, y_test = train_test_split(X_unlabeled, _, test_size=0.5, random_state=42)
# 初始模型训练
model = RandomForestClassifier(n_estimators=100, random_state=42)
model.fit(X_train, y_train)
# 自训练过程
for i in range(10):
y_pred_unlabeled = model.predict(X_unlabeled)
X_train = np.vstack((X_train, X_unlabeled))
y_train = np.concatenate((y_train, y_pred_unlabeled))
model.fit(X_train, y_train)
# 评估模型
y_pred = model.predict(X_test)
accuracy = accuracy_score(y_test, y_pred)
print(f'自训练模型准确率: {accuracy}')
2.2 协同训练(Co-Training)
协同训练是一种基于多视图的半监督学习方法,通过训练两个或多个不同视角的分类器,分别对无标签数据进行预测,并将一个分类器高置信度的预测结果作为有标签数据供另一个分类器使用,迭代进行训练。
python
from sklearn.linear_model import LogisticRegression
from sklearn.tree import DecisionTreeClassifier
# 定义两个不同视角的分类器
model1 = LogisticRegression(random_state=42)
model2 = DecisionTreeClassifier(random_state=42)
# 初始模型训练
model1.fit(X_train, y_train)
model2.fit(X_train, y_train)
# 协同训练过程
for i in range(10):
y_pred_unlabeled1 = model1.predict(X_unlabeled)
y_pred_unlabeled2 = model2.predict(X_unlabeled)
high_confidence_idx1 = np.where(model1.predict_proba(X_unlabeled).max(axis=1) > 0.9)[0]
high_confidence_idx2 = np.where(model2.predict_proba(X_unlabeled).max(axis=1) > 0.9)[0]
X_train1 = np.vstack((X_train, X_unlabeled[high_confidence_idx2]))
y_train1 = np.concatenate((y_train, y_pred_unlabeled2[high_confidence_idx2]))
X_train2 = np.vstack((X_train, X_unlabeled[high_confidence_idx1]))
y_train2 = np.concatenate((y_train, y_pred_unlabeled1[high_confidence_idx1]))
model1.fit(X_train1, y_train1)
model2.fit(X_train2, y_train2)
# 评估模型
y_pred1 = model1.predict(X_test)
y_pred2 = model2.predict(X_test)
accuracy1 = accuracy_score(y_test, y_pred1)
accuracy2 = accuracy_score(y_test, y_pred2)
print(f'协同训练模型1准确率: {accuracy1}')
print(f'协同训练模型2准确率: {accuracy2}')
2.3 图半监督学习(Graph-Based Semi-Supervised Learning)
图半监督学习通过构建图结构,将数据点视为图中的节点,利用节点之间的相似性传播标签信息,从而实现无标签数据的标注。图半监督学习方法包括标签传播(Label Propagation)和图正则化(Graph Regularization)等。
python
from sklearn.semi_supervised import LabelPropagation
# 构建有标签数据和无标签数据
X_labeled, X_unlabeled, y_labeled, y_unlabeled = train_test_split(X, y, test_size=0.7, random_state=42)
y_unlabeled[:] = -1 # 将无标签数据的标签设为-1
# 合并有标签和无标签数据
X_combined = np.vstack((X_labeled, X_unlabeled))
y_combined = np.concatenate((y_labeled, y_unlabeled))
# 训练标签传播模型
label_propagation = LabelPropagation()
label_propagation.fit(X_combined, y_combined)
# 预测并评估模型
y_pred = label_propagation.predict(X_test)
accuracy = accuracy_score(y_test, y_pred)
print(f'标签传播模型准确率: {accuracy}')
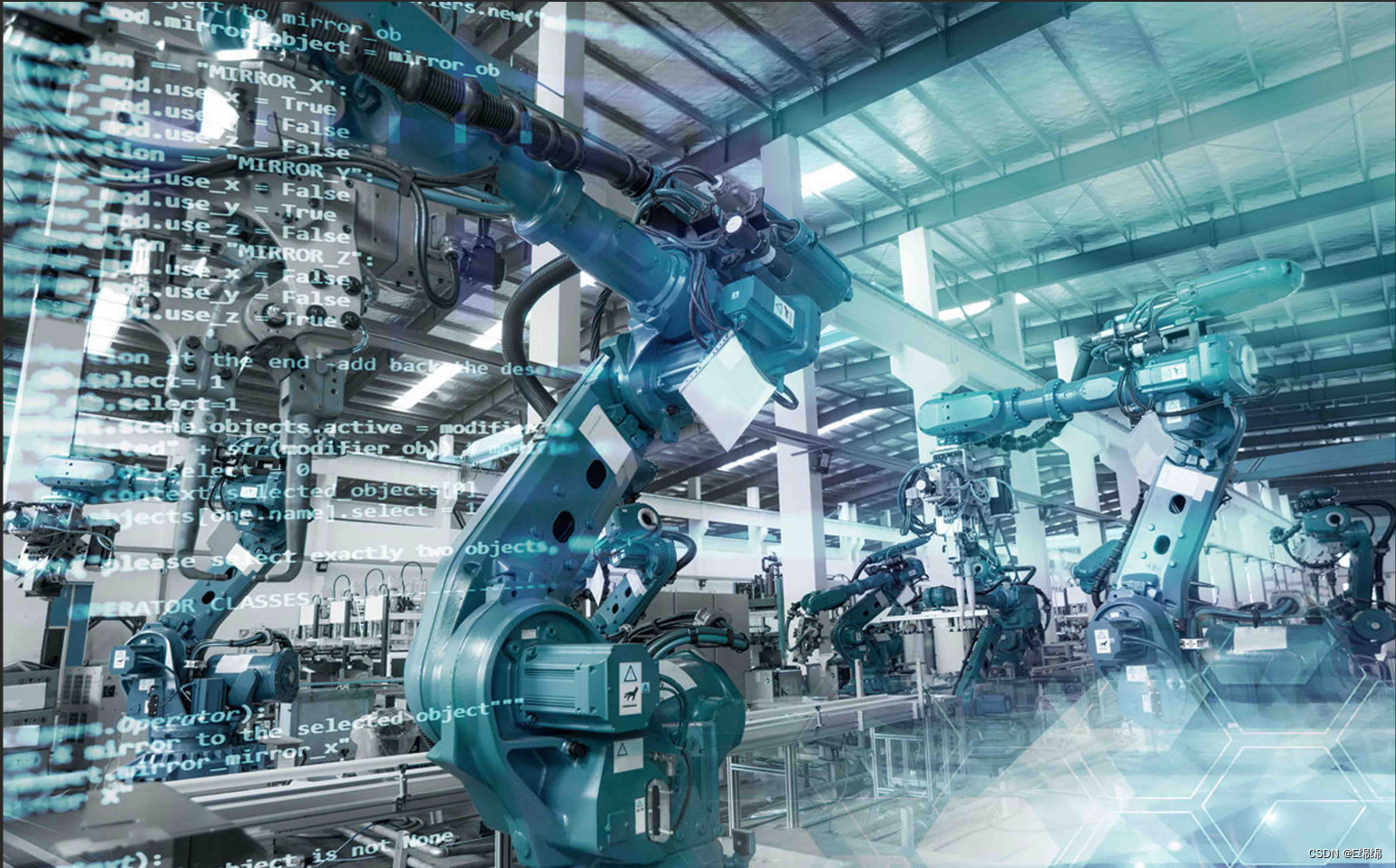
第三章 半监督学习的应用实例
3.1 图像分类
在图像分类任务中,半监督学习方法通过结合有标签和无标签图像数据,可以显著提高分类精度。以下是一个在MNIST数据集上使用自训练进行图像分类的示例。
python
import tensorflow as tf
from tensorflow.keras.datasets import mnist
from tensorflow.keras.models import Sequential
from tensorflow.keras.layers import Dense, Flatten
# 加载数据集
(x_train, y_train), (x_test, y_test) = mnist.load_data()
x_train, x_test = x_train / 255.0, x_test / 255.0
# 划分有标签数据和无标签数据
x_labeled, x_unlabeled = x_train[:1000], x_train[1000:]
y_labeled = y_train[:1000]
# 定义模型
model = Sequential([
Flatten(input_shape=(28, 28)),
Dense(128, activation='relu'),
Dense(10, activation='softmax')
])
model.compile(optimizer='adam', loss='sparse_categorical_crossentropy', metrics=['accuracy'])
# 初始模型训练
model.fit(x_labeled, y_labeled, epochs=10, validation_data=(x_test, y_test), verbose=2)
# 自训练过程
for i in range(10):
y_pred_unlabeled = np.argmax(model.predict(x_unlabeled), axis=1)
x_labeled = np.vstack((x_labeled, x_unlabeled))
y_labeled = np.concatenate((y_labeled, y_pred_unlabeled))
model.fit(x_labeled, y_labeled, epochs=10, validation_data=(x_test, y_test), verbose=2)
# 评估模型
test_loss, test_acc = model.evaluate(x_test, y_test, verbose=2)
print(f'自训练模型准确率: {test_acc}')
3.2 文本分类
在文本分类任务中,半监督学习方法通过结合有标签和无标签文本数据,可以提高分类效果。以下是一个在IMDB情感分析数据集上使用协同训练进行文本分类的示例。
python
from tensorflow.keras.preprocessing.text import Tokenizer
from tensorflow.keras.preprocessing.sequence import pad_sequences
from tensorflow.keras.layers import Embedding, LSTM, Dense, Bidirectional
from tensorflow.keras.models import Sequential
# 加载数据集
(x_train, y_train), (x_test, y_test) =
tf.keras.datasets.imdb.load_data(num_words=10000)
# 数据预处理
maxlen = 100
x_train = pad_sequences(x_train, maxlen=maxlen)
x_test = pad_sequences(x_test, maxlen=maxlen)
# 划分有标签数据和无标签数据
x_labeled, x_unlabeled = x_train[:1000], x_train[1000:]
y_labeled = y_train[:1000]
# 定义LSTM模型
def create_lstm_model():
model = Sequential([
Embedding(10000, 128, input_length=maxlen),
Bidirectional(LSTM(64)),
Dense(1, activation='sigmoid')
])
model.compile(optimizer='adam', loss='binary_crossentropy', metrics=['accuracy'])
return model
# 训练两个LSTM模型
model1 = create_lstm_model()
model2 = create_lstm_model()
model1.fit(x_labeled, y_labeled, epochs=5, validation_data=(x_test, y_test), verbose=2)
model2.fit(x_labeled, y_labeled, epochs=5, validation_data=(x_test, y_test), verbose=2)
# 协同训练过程
for i in range(5):
y_pred_unlabeled1 = (model1.predict(x_unlabeled) > 0.5).astype(int)
y_pred_unlabeled2 = (model2.predict(x_unlabeled) > 0.5).astype(int)
high_confidence_idx1 = np.where(np.abs(model1.predict(x_unlabeled) - 0.5) > 0.4)[0]
high_confidence_idx2 = np.where(np.abs(model2.predict(x_unlabeled) - 0.5) > 0.4)[0]
x_train1 = np.vstack((x_labeled, x_unlabeled[high_confidence_idx2]))
y_train1 = np.concatenate((y_labeled, y_pred_unlabeled2[high_confidence_idx2]))
x_train2 = np.vstack((x_labeled, x_unlabeled[high_confidence_idx1]))
y_train2 = np.concatenate((y_labeled, y_pred_unlabeled1[high_confidence_idx1]))
model1.fit(x_train1, y_train1, epochs=5, validation_data=(x_test, y_test), verbose=2)
model2.fit(x_train2, y_train2, epochs=5, validation_data=(x_test, y_test), verbose=2)
# 评估模型
test_loss1, test_acc1 = model1.evaluate(x_test, y_test, verbose=2)
test_loss2, test_acc2 = model2.evaluate(x_test, y_test, verbose=2)
print(f'协同训练模型1准确率: {test_acc1}')
print(f'协同训练模型2准确率: {test_acc2}')
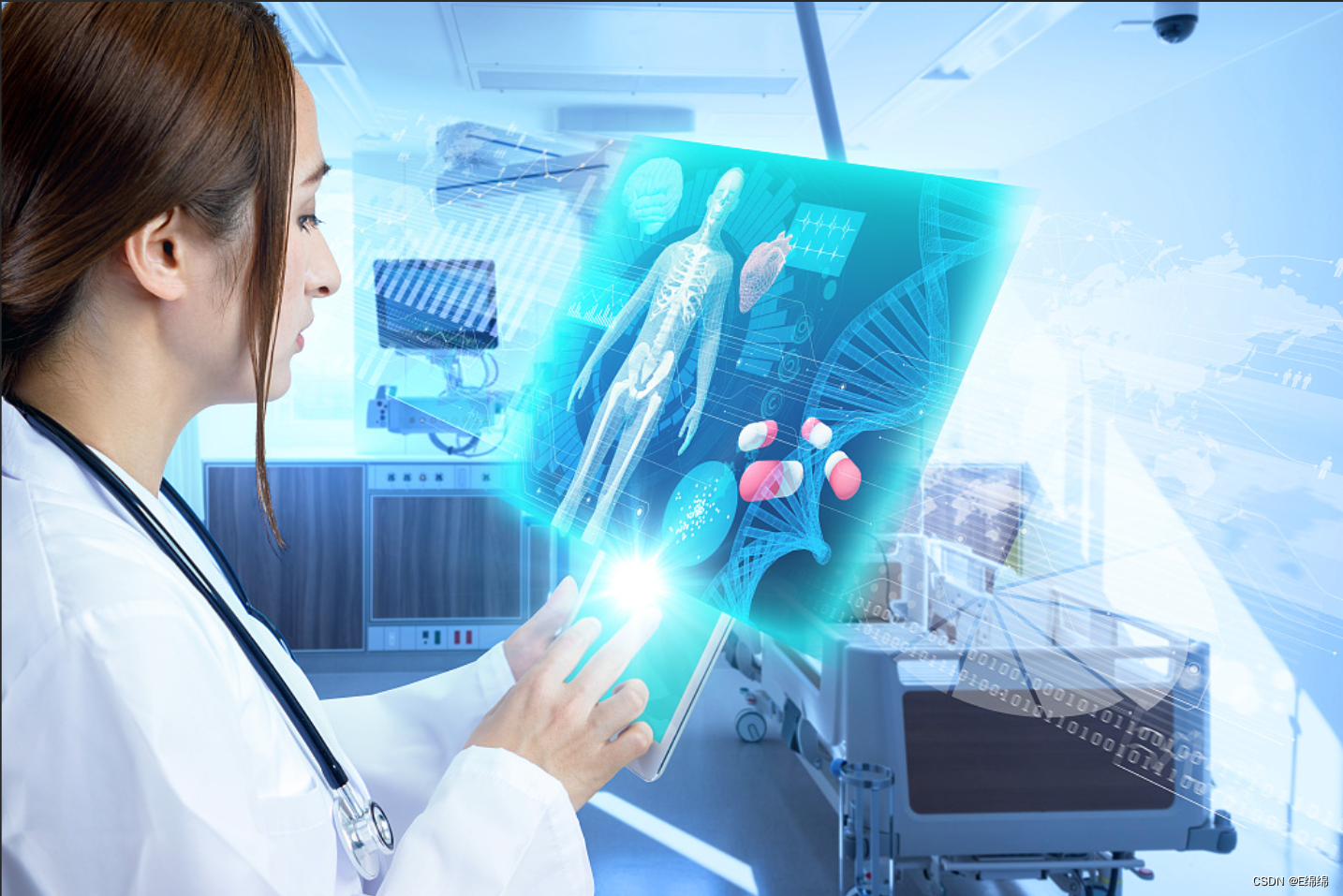
第四章 半监督学习的未来发展与挑战
4.1 标签质量与模型鲁棒性
在半监督学习中,标签数据的质量对模型性能有着至关重要的影响。研究如何保证标签数据的质量,以及在存在噪声标签的情况下提高模型的鲁棒性,是一个重要的研究方向。
4.2 多视角与多模态学习
多视角与多模态学习是半监督学习的一个重要方向,通过结合来自不同视角或不同模态的数据,可以提高模型的泛化能力和预测准确性。研究如何有效融合多视角和多模态数据,是半监督学习的一个关键挑战。
4.3 标注策略与主动学习
在实际应用中,通过主动学习策略,可以有效选择最有价值的样本进行标注,从而最大化利用有限的标注资源,提高半监督学习模型的性能。研究如何设计高效的主动学习策略,是半监督学习的一个重要研究课题。
结论
半监督学习作为一种有效的机器学习方法,通过结合少量有标签数据和大量无标签数据,在标签数据稀缺的情况下能够显著提高模型的泛化能力和预测准确性。本文详细介绍了半监督学习的基本概念、核心算法及其在实际中的应用,并提供了具体的代码示例,帮助读者深入理解和掌握这一技术。希望本文能够为您进一步探索和应用半监督学习提供有价值的参考。
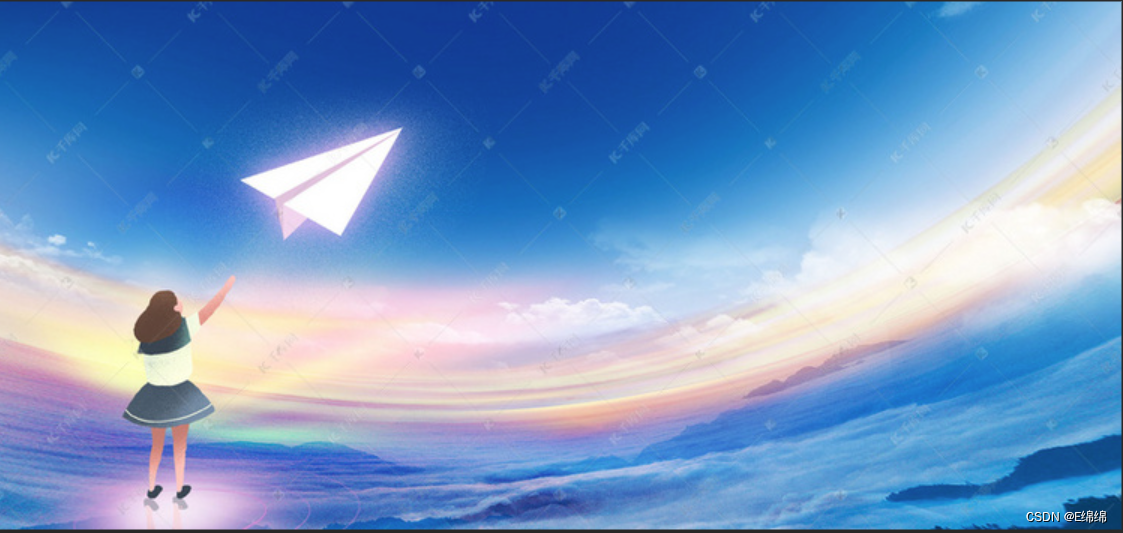