24深度卷积神经网络AlexNet
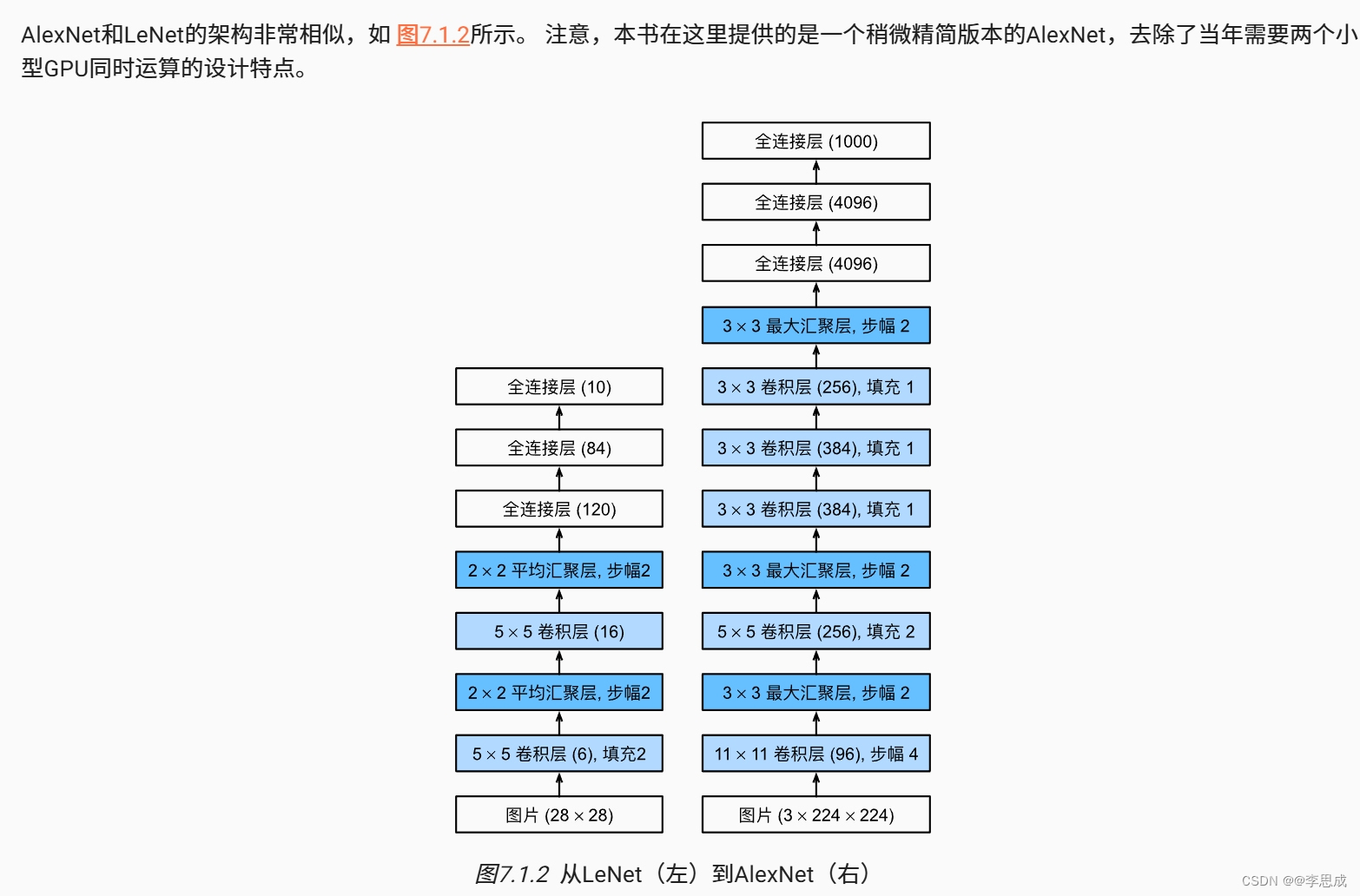
python
import torch
from torch import nn
import liliPytorch as lp
import liliPytorch as lp
import matplotlib.pyplot as plt
dropout1 = 0.5
#Alexnet架构
net = nn.Sequential(
nn.Conv2d(1, 96, kernel_size=11, stride=4, padding=1),
nn.ReLU(),
nn.MaxPool2d(kernel_size=3, stride=2),
nn.Conv2d(96, 256, kernel_size=5, padding=2),
nn.ReLU(),
nn.MaxPool2d(kernel_size=3, stride=2),
nn.Conv2d(256, 384, kernel_size=3, padding=1),
nn.ReLU(),
nn.Conv2d(384, 384, kernel_size=3, padding=1),
nn.ReLU(),
nn.Conv2d(384, 256, kernel_size=3, padding=1),
nn.ReLU(),
nn.MaxPool2d(kernel_size=3, stride=2),
nn.Flatten(),
nn.Linear(6400, 4096),
nn.ReLU(),
nn.Dropout(dropout1),
nn.Linear(4096, 4096),
nn.ReLU(),
nn.Dropout(dropout1),
nn.Linear(4096,10)
)
#魔改一下
lilinet = nn.Sequential(
nn.Conv2d(1, 96, kernel_size=11, stride=4, padding=1),
nn.ReLU(),
nn.MaxPool2d(kernel_size=3, stride=2),
nn.Conv2d(96, 256, kernel_size=5, padding=2),
nn.ReLU(),
nn.MaxPool2d(kernel_size=3, stride=2),
nn.MaxPool2d(kernel_size=3, stride=2),
nn.Flatten(),
nn.Linear(6400, 4096),
nn.ReLU(),
nn.Dropout(dropout1),
nn.Linear(4096, 4096),
nn.ReLU(),
nn.Dropout(dropout1),
nn.Linear(4096,10)
)
# 通过在每一层打印输出的形状,我们可以检查模型
X = torch.rand(size=(1, 1, 224, 224), dtype=torch.float32)
for layer in net:
X = layer(X) # 将输入依次通过每一层
print(layer.__class__.__name__, 'output shape: \t', X.shape) # 打印每一层的输出形状
"""
Conv2d output shape: torch.Size([1, 96, 54, 54])
ReLU output shape: torch.Size([1, 96, 54, 54])
MaxPool2d output shape: torch.Size([1, 96, 26, 26])
Conv2d output shape: torch.Size([1, 256, 26, 26])
ReLU output shape: torch.Size([1, 256, 26, 26])
MaxPool2d output shape: torch.Size([1, 256, 12, 12])
Conv2d output shape: torch.Size([1, 384, 12, 12])
ReLU output shape: torch.Size([1, 384, 12, 12])
Conv2d output shape: torch.Size([1, 384, 12, 12])
ReLU output shape: torch.Size([1, 384, 12, 12])
Conv2d output shape: torch.Size([1, 256, 12, 12])
ReLU output shape: torch.Size([1, 256, 12, 12])
MaxPool2d output shape: torch.Size([1, 256, 5, 5])
Flatten output shape: torch.Size([1, 6400])
Linear output shape: torch.Size([1, 4096])
ReLU output shape: torch.Size([1, 4096])
Dropout output shape: torch.Size([1, 4096])
Linear output shape: torch.Size([1, 4096])
ReLU output shape: torch.Size([1, 4096])
Dropout output shape: torch.Size([1, 4096])
Linear output shape: torch.Size([1, 10])
"""
#读取数据集
batch_size = 64
train_iter, test_iter = lp.loda_data_fashion_mnist(batch_size, resize=224) # 加载Fashion-MNIST数据集
#Alexnet架构
# lr, num_epochs = 0.01, 10
# batch_size = 128
# lp.train_ch6(net, train_iter, test_iter, num_epochs, lr, lp.try_gpu())
# loss 0.329, train acc 0.879, test acc 0.883
# 魔改
lr, num_epochs = 0.1, 10
lp.train_ch6(lilinet, train_iter, test_iter, num_epochs, lr, lp.try_gpu())
plt.show() # 显示训练曲线
#lr, num_epochs = 0.01, 10
#batch_size = 128
#loss 0.356, train acc 0.868, test acc 0.870
#lr, num_epochs = 0.1, 10
#batch_size = 64
#loss 0.212, train acc 0.920, test acc 0.903
运行结果: