零、概述
Apache Flink 是一个高性能的开源分布式流处理框架,专注于实时数据流的处理。
它设计用于处理无界和有界数据流,在内存级速度下提供高效的有状态计算。
Flink 凭借其独特的Checkpoint机制和Exactly-Once语义,确保数据处理的准确性和一致性,同时支持高吞吐量和低延迟。
通过灵活的窗口操作和丰富的状态管理功能,Flink 能够应对复杂的实时数据处理需求,是大数据处理领域的重要技术之一。
其强大的DataStream API和Table API为开发者提供了高效、简洁的数据处理手段。
一、添加依赖 pom.xml
xml
<?xml version="1.0" encoding="UTF-8"?>
<project xmlns="http://maven.apache.org/POM/4.0.0"
xmlns:xsi="http://www.w3.org/2001/XMLSchema-instance"
xsi:schemaLocation="http://maven.apache.org/POM/4.0.0 http://maven.apache.org/xsd/maven-4.0.0.xsd">
<modelVersion>4.0.0</modelVersion>
<groupId>com.xch</groupId>
<artifactId>java-flink</artifactId>
<version>1.0-SNAPSHOT</version>
<properties>
<encoding>UTF-8</encoding>
<project.build.sourceEncoding>UTF-8</project.build.sourceEncoding>
<maven.compiler.source>1.8</maven.compiler.source>
<maven.compiler.target>1.8</maven.compiler.target>
<java.version>1.8</java.version>
<flink.version>1.12.2</flink.version>
</properties>
<dependencies>
<dependency>
<groupId>org.apache.flink</groupId>
<artifactId>flink-clients_2.12</artifactId>
<version>${flink.version}</version>
</dependency>
<dependency>
<groupId>org.apache.flink</groupId>
<artifactId>flink-java</artifactId>
<version>${flink.version}</version>
</dependency>
<dependency>
<groupId>org.apache.flink</groupId>
<artifactId>flink-streaming-java_2.12</artifactId>
<version>${flink.version}</version>
</dependency>
<dependency>
<groupId>org.apache.flink</groupId>
<artifactId>flink-table-api-java-bridge_2.12</artifactId>
<version>${flink.version}</version>
</dependency>
</dependencies>
</project>
二、map()
filter()
flatMap()
方法示例
2.1 map()方法示例
简单处理,和java8的stream的map()类似,不过只能进行简单的处理,返回:数组元素自身的和
java
public static List<Integer> mapDemo(DataSource<Integer> dataSteam) throws Exception {
return dataSteam.map(x -> x + x).collect();
}
2.2 filter()方法示例
过滤方法,返回偶数,
java
public static List<Integer> filterDemo(DataSource<Integer> dataSteam) throws Exception {
return dataSteam.filter(x -> x % 2 == 0).collect();
}
2.3 flatMap()方法示例
flatMap方法可以处理复杂、定制化的逻辑,返回元素的类型也可以是复杂的;
- 第一个简单处理的示例
java
public static List<Object> flatMapDemo(DataSource<Integer> dataSteam) throws Exception {
return dataSteam.flatMap(new FlatMapFunction<Integer, Object>() {
@Override
public void flatMap(Integer integer, Collector<Object> collector) throws Exception {
collector.collect(integer);
collector.collect(integer * integer);
}
}).collect();
}
- 第二个复杂的示例
java
public static List<Map<Integer, Object>> flatMapDemo1(DataSource<Integer> dataSteam) throws Exception {
return dataSteam.flatMap(new FlatMapFunction<Integer, Map<Integer, Object>>() {
@Override
public void flatMap(Integer integer, Collector<Map<Integer, Object>> collector) throws Exception{
Map<Integer, Object> hashMap = new HashMap<>();
hashMap.put(integer, integer * integer);
collector.collect(hashMap);
}
}).collect();
}
2.4 示例演示
java
import org.apache.flink.api.common.functions.FlatMapFunction;
import org.apache.flink.api.java.ExecutionEnvironment;
import org.apache.flink.api.java.operators.DataSource;
import org.apache.flink.util.Collector;
import java.util.HashMap;
import java.util.List;
import java.util.Map;
public class FlinkDemo {
public static void main(String[] args) throws Exception {
ExecutionEnvironment env = ExecutionEnvironment.getExecutionEnvironment();
DataSource<Integer> dataSteam = env.fromElements(1, 2, 3, 4, 5, 6, 7, 8, 9, 10);
System.out.println("mapDemo:" + mapDemo(dataSteam));
System.out.println("filterDemo:" + filterDemo(dataSteam));
System.out.println("flatMapDemo:" + flatMapDemo(dataSteam));
System.out.println("flatMapDemo1:" + flatMapDemo1(dataSteam));
}
}
输出内容:
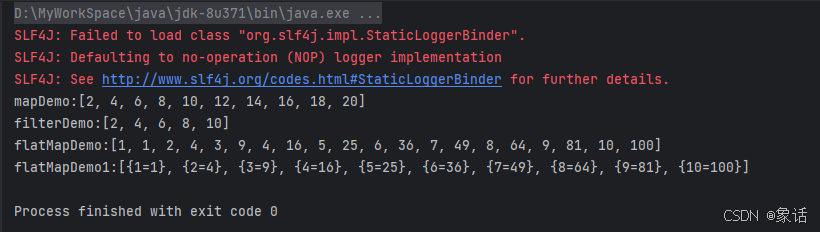