rockchip的yolov5 rknn推理分析
对于rockchip给出的这个yolov5后处理代码的分析,本人能力十分有限,可能有的地方描述的很不好,欢迎大家和我一起讨论,指出我的错误!!!
RKNN模型输出
将官方的YOLOv5 7.0模型转换成RKNN模型后,使用Netron观察网络的输入和输出
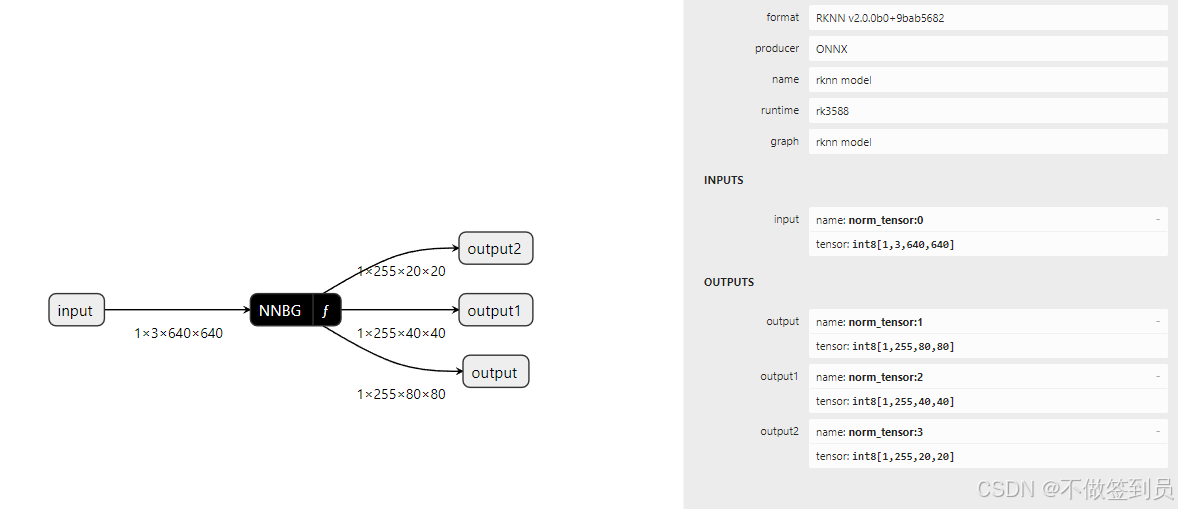
由于我们使用的是原始的COCO数据集(80分类)模型,因此这里YOLOv5的输入为:1 x 3 x 640 x 640 (NCHW)
- H:图片的高度:640
- W:图片的宽度:640
- C:图片的通道数:3
- N:图片的数量,通常为1
三个检测头最后分别输出为1 x 255 x 80 x 80、1 x 255 x 40 x 40、1 x 255 x 20 x 20,其中模型输出的
- 1表示batch_size,batch size表示批量大小,即一次处理的数据样本数量,批量大小为1,意味着这个张量只包含一个数据样本。
- 20 x 20、40 x 40、80 x 80分别为3个特征层的形状大小,
- 255 =3 x 85 前面的3表示每个特征点对应的3个先验框,后面的85可以拆分成4+1+80。
- 前4个参数用于判断每一个特征点的回归参数,回归参数调整后可以获得预测框;
- 第5个参数用于判断每一个特征点是否包含物体,即先验框是否包含物体的概率大小。
- 最后80个参数用于判断每一个特征点所包含的各个物体的类别概率。
1 x 255 x 80 x 80、1 x 255 x 40 x 40、1 x 255 x 20 x 20即有一下拆分
- (4 + 1 + 80) x 3 x 80 x 80 = 1 x 255 x 80 x 80
- (4 + 1 + 80) x 3 x 40 x 40 = 1 x 255 x 40 x 40
- (4 + 1 + 80) x 3 x 20 x 20 = 1 x 255 x 20 x 20
所以总的输出为:85 x 3 x (20 x 20 + 40 x 40 + 80 x 80) = 85 x 25200,即一共有25200个先验框。
模型推理前处理
尺寸处理
由于模型的输入数据为NCHW格式,即1 x 3 x 640 x 640,而我们推理一张图像的时候,绝大对数的图像数据其w和h尺寸不一定完全满足640 x 640,通常我们利用opencv的resize操纵将图像的尺寸resize成640 x 640,但是这样常常会导致图像变形失真进而可能会有图像特征的丢失;
在rockchip提供的后处理代码中,对于图像的输入使用了letterbox,即将输入图像调整到指定的新尺寸,同时保持图像内容的宽高比例,通过在图像周围添加边框(通常是黑色)来实现,由此便可以实现将不同尺寸的图像变为640 x 640;letterbox:常用于模型输入的图片尺寸为正方形的情况,它可以将为长方形的图像,在保持图片的长宽比例的情况下,剩下的部分采用灰色填充。
letterbox的代码如下所示:
python
def letterbox(im, new_shape=(640, 640), color=(0, 0, 0)):
shape = im.shape[:2] # current shape [height, width]
if isinstance(new_shape, int): # 是否是一个整数
new_shape = (new_shape, new_shape)
# 比例 (new / old)
r = min(new_shape[0] / shape[0], new_shape[1] / shape[1])
# 计算填充
ratio = r # ratios
new_unpad = int(round(shape[1] * r)), int(round(shape[0] * r))
dw, dh = new_shape[1] - new_unpad[0], new_shape[0] - new_unpad[1] # wh padding
dw /= 2 # divide padding into 2 sides
dh /= 2
if shape[::-1] != new_unpad: # resize
im = cv2.resize(im, new_unpad, interpolation=cv2.INTER_LINEAR)
top, bottom = int(round(dh - 0.1)), int(round(dh + 0.1))
left, right = int(round(dw - 0.1)), int(round(dw + 0.1))
im = cv2.copyMakeBorder(im, top, bottom, left, right, cv2.BORDER_CONSTANT, value=color) # add border
return im, ratio, (dw, dh)
letterbox的代码流程为:先获取当前图像的维度-高度和宽度,接着取目标维度的宽比上当前图像的宽和目标维度的高比上当前图像的高的最小值,即为新尺寸和当前尺寸之间的最小缩放比例,根据缩放比例 计算新的未填充图像尺寸 new_unpad
,计算新尺寸和目标图像尺寸之间的差异,得到水平和垂直方向上的边框尺寸 dw
和 dh
,将 dw
和 dh
除以 2,以便在图像的两侧平均分配边框;如果原始图像尺寸与计算出的未填充尺寸不匹配,使用 cv2.resize
对图像进行缩放。使用 cv2.copyMakeBorder
函数在图像周围添加边框,这里使用的是常数边框,其颜色由 color
参数指定。返回调整尺寸并添加边框后的图像 im
,缩放比例 ratio
,以及边框尺寸 (dw, dh)
。
色彩空间转换
在对输入数据的尺寸进行处理完后,由于模型推理的图像色彩空间为RGB,而opnecv读取的图像其色彩空间为BGR,所以需要进行色彩空间转换
python
img = cv2.cvtColor(img, cv2.COLOR_BGR2RGB)
cvtColor指定cv2.COLOR_BGR2RGB:将颜色空间由BGR图像转为RGB
尺度扩充
由于模型的输入数据为神经网络容易识别的NCHW格式 ,所以要将图片数据HWC转换(transpose)为NCHW格式
所以要进行
python
img2 = np.expand_dims(img, 0)
使用NumPy库时,np.expand_dims
函数用于增加数组的维度。对于图像处理,这通常意味着将一个形状为 (height, width, channels)
的三维数组增加一个新的轴,变为 (batch_size, height, width, channels)
形状的四维数组。
这里是 np.expand_dims(img, 0)
的详细解释:
img
: 这是一个三维数组,代表一张图像,其形状可能是(height, width, channels)
0
: 这是np.expand_dims
的第二个参数,表示要在数组的第0维(即最外层)增加一个新的轴。
执行 img2 = np.expand_dims(img, 0)
后,img2
将具有一个新的维度,形状变为 (1, height, width, channels)
。这个操作在深度学习中非常常见,因为大多数深度学习框架都期望输入数据具有批量维度(batch_size
),即使批量大小为1。
模型推理
python
outputs = rknn.inference(inputs=[img2], data_format=['nhwc'])
data_format:输入数据的 layout 列表,"nchw"或"nhwc",只对 4 维的输入有效。默认值为 None,表示所有输入的 layout 都为 NHWC。
这里存在一个问题:观察模型的输入格式为NCHW,这里指定了NHWC的时候也可以成功推理,在咨询了相关技术群的大佬,普遍认为当设置data_format为NHWC时,在输入数据为NCHW的时候,inference内部存在一个transpose会自行转换,但是实际情况是否是这样不得而知,希望有懂的大佬解释一下(在下跪了)!!
输出的outputs是一个列表,里面是三个数组,这三个数组的维度分别为(1, 255, 80, 80)、(1, 255, 40, 40)、(1, 255, 20, 20),
python
input0_data = outputs[0] # (1, 255, 80, 80)
input1_data = outputs[1] # (1, 255, 40, 40)
input2_data = outputs[2] # (1, 255, 20, 20)
这三个数组的尺寸即是使用Netron查看到的网络输出尺寸;
在进行后处理前,将输出维度的顺序由 1 * 255 * h * w 变换为 3 * 85 * h * w,然后再调整顺序为:h * w * 3 * 85,并均保存到input_data列表中
python
input0_data = input0_data.reshape([3, -1]+list(input0_data.shape[-2:])) # (1, 255, 80, 80) -> (3, 85, 80, 80)
input1_data = input1_data.reshape([3, -1]+list(input1_data.shape[-2:])) # (1, 255, 40, 40) -> (3, 85, 40, 40)
input2_data = input2_data.reshape([3, -1]+list(input2_data.shape[-2:])) # (1, 255, 20, 20) -> (3, 85, 20, 20)
input_data = list()
input_data.append(np.transpose(input0_data, (2, 3, 0, 1))) # (3, 85, 80, 80) -> (80, 80, 3, 85)
input_data.append(np.transpose(input1_data, (2, 3, 0, 1))) # (3, 85, 40, 40) -> (40, 40, 3, 85)
input_data.append(np.transpose(input2_data, (2, 3, 0, 1))) # (3, 85, 20, 20) -> (20, 20, 3, 85)
模型推理后处理
后处理流程
将input_data列表传递给yolov5_post_process后处理函数
python
boxes, classes, scores = yolov5_post_process(input_data)
最终得到的是对于640 x 640图像的三个信息:
-
boxes:检测框信息,对于每一个检测框的信息呈现为左上角坐标和右下角坐标
-
classes:检测框检测出来的物体类别
-
scores:检测出来的物体的置信度
yolov5_post_process函数接受的参数为:包含了三个输出特征顺序为h * w * 3 * 85的列表 - input_data
首先指定掩码masks和锚框anchors
- 锚框(anchors)为目标检测的预定义框,它们是在训练之前设定的先验框,在训练开始前根据数据集自动计算或手动设置的,以适应不同大小的目标。由于我们使用的模型权重是YOLOv5推理COCO数据集的权重,所以锚框直接使用配置文件(在
yolov5s.yaml
配置文件中)中预设的针对COCO数据集的锚定框尺寸,预设了640×640图像大小下的锚定框尺寸[10,13, 16,30, 33,23]
、[30,61, 62,45, 59,119]
和[116,90, 156,198, 373,326]
,分别对应于不同特征图上的锚框 ,用于在特征图上预测目标的位置和尺寸。
python
anchors = [[10, 13], [16, 30], [33, 23], [30, 61], [62, 45], [59, 119], [116, 90], [156, 198], [373, 326]]
- 掩码(masks)能够在不同尺度的特征图上检测不同大小的目标,其中大特征图用于检测小目标,小特征图用于检测大目标 。
python
masks = [[0, 1, 2], [3, 4, 5], [6, 7, 8]]
创建boxes, classes, scores三个列表,用来存储3个维度特征图经过解码和低置信度过滤得到的剩余预测框的位置信息、预测框预测的物品类别、预测的概率值
python
boxes, classes, scores = [], [], []
for input, mask in zip(input_data, masks):
b, c, s = process(input, mask, anchors)
b, c, s = filter_boxes(b, c, s)
boxes.append(b)
classes.append(c)
scores.append(s)
for循环遍历的input和mask只有三个值,因为zip
函数通常用于将多个可迭代对象中对应的元素打包成一个个元组,然后返回由这些元组组成的迭代器。在(input_data, masks):中,input_data
和 masks
都是列表,且它们拥有相同数量的元素,那么 zip
函数将它们对应元素配对,形成元组 (input, mask)
,其中 input
是来自 input_data
的一个元素,mask
是来自 masks
的一个元素, 然后,这个元组被用来在 for
循环中迭代。
例如下面依次是遍历出来的input的维度(input完全打印显示不下)和mask的值:
input的维度 | mask值 |
---|---|
(80, 80, 3, 85) | [0, 1, 2] |
(40, 40, 3, 85) | [3, 4, 5] |
(20, 20, 3, 85) | [6, 7, 8] |
将每一个特征图,及对应的掩码和所有的anchors传递给process函数
python
b, c, s = process(input, mask, anchors)
process函数为解码函数,他返回不同特征图的预测框的尺寸信息、预测框是否含有物体的概率、预测框在含有物体的条件下对于COCO数据集80个物体类别的条件概率;
在将上面得到的结果进行两次低置信度过滤,得到b过滤后的预测框的信息, c过滤后的预测框预测的物体类别, s过滤后的预测框预测的物体类别的概率值;
python
b, c, s = filter_boxes(b, c, s)
我们将维度在下面依次展示:
b的维度 | c的维度 | s的维度 |
---|---|---|
(0, 4) | (0,) | (0,) |
(15, 4) | (15,) | (15,) |
(39, 4) | (39,) | (39,) |
将三个特征图得到的结果列表boxes, classes, scores,使用 NumPy 的 concatenate
函数将三个列表中的所有数组连接成一个单独的数组,
python
# 三个特征图各自的结果合并到一起
boxes = np.concatenate(boxes)
boxes = xywh2xyxy(boxes)
classes = np.concatenate(classes)
scores = np.concatenate(scores)
'''
打印出boxes、classes和scores的维度:
boxes.shape : (54, 4)
classes.shape : (54,)
scores.shape : (54,)
54 = 0 + 15 + 39
'''
即最终boxes, classes, scores合并为的数组尺寸为:
- boxes.shape : (*, 4) 4为预测框的信息,将其转化成为了用左上角坐标点和右下角坐标点表示
- classes.shape : (*,) 预测框的预测的物体的类别
- scores.shape : (*, ) 预测框预测物体的置信度得分
上面 *为三个特征图剩余的预测框的个数
同时将预测框的boxes信息由中心点的x,y和框的w,h值转化为预测框的左上角x1,y1和右下角的x2,y2表示
python
def xywh2xyxy(x):
# Convert [x, y, w, h] to [x1, y1, x2, y2]
y = np.copy(x)
y[:, 0] = x[:, 0] - x[:, 2] / 2 # top left x 原始的 x 坐标是中心点的 x 坐标,减去宽的一半得到左上角的 x 坐标。
y[:, 1] = x[:, 1] - x[:, 3] / 2 # top left y 原始的 y 坐标是中心点的 y 坐标,减去高的一半得到左上角的 y 坐标。
y[:, 2] = x[:, 0] + x[:, 2] / 2 # bottom right x 中心点的 x 坐标加上宽的一半得到右下角的 x 坐标。
y[:, 3] = x[:, 1] + x[:, 3] / 2 # bottom right y 中心点的 y 坐标加上高的一半得到右下角的 y 坐标。
return y
至此,所有的预测框存在着预测的物体是相同类别的,此时分为两种情况:1. 图像数据中此类物体有多个,此时预测框预测的没有问题;2. 一个物体有多个预测框这便是有问题的;对于第二个情况,我们利用NMS非极大值抑制来解决;
创建三个nboxes, nclasses, nscores列表用于存储非极大值抑制的结果;
python
# nms
nboxes, nclasses, nscores = [], [], []
不重复(set自动去重)的遍历classes列表中的所有类别,c为classes列表中不重复的类别
python
for c in set(classes):
'''
打印set(classes):
{0, 5}
'''
说明在classes中只有两个类别
使用np.where的条件判断返回classes类别中类别为c的相同类别的索引,然后取出相同类别的预测框的信息、类别和置信度
python
# 找到某一类别的所有索引
inds = np.where(classes == c)
# 取出相同类别的预测框 类别 和 置信度
b = boxes[inds]
c = classes[inds]
s = scores[inds]
将相同类别的预测框的信息、置信度传递给非极大值抑制函数,对相同类别进行非极大值抑制
python
# 对相同类别进行非极大值抑制
keep = nms_boxes(b, s)
最后经过非极大值抑制后,第0类剩余的预测框的索引为:[21, 8, 27, 40],第5类剩余的预测框的索引为[9],即目前仅仅剩下五个预测框,我们将剩下的预测框拼接到一起
python
boxes = np.concatenate(nboxes)
classes = np.concatenate(nclasses)
scores = np.concatenate(nscores)
最后得到的boxes维度为(5, 4),classes维度为(5,),scores的维度为(5,),将结果返回,最终后处理完成;
yolov5_post_process的代码为:
python
def yolov5_post_process(input_data):
masks = [[0, 1, 2], [3, 4, 5], [6, 7, 8]]
anchors = [[10, 13], [16, 30], [33, 23], [30, 61], [62, 45],
[59, 119], [116, 90], [156, 198], [373, 326]]
boxes, classes, scores = [], [], []
for input, mask in zip(input_data, masks):
b, c, s = process(input, mask, anchors)
b, c, s = filter_boxes(b, c, s)
boxes.append(b)
classes.append(c)
scores.append(s)
# 三个结果合并到一起
boxes = np.concatenate(boxes)
boxes = xywh2xyxy(boxes)
classes = np.concatenate(classes)
scores = np.concatenate(scores)
# nms
nboxes, nclasses, nscores = [], [], []
for c in set(classes): # 遍历所有不同的类别
# 找到某一类别的所有索引
inds = np.where(classes == c)
# 取出相同类别的检测框 类别 和 置信度
b = boxes[inds]
c = classes[inds]
s = scores[inds]
# 对相同类别进行非极大值抑制
keep = nms_boxes(b, s)
if len(keep) != 0:
nboxes.append(b[keep])
nclasses.append(c[keep])
nscores.append(s[keep])
if not nclasses and not nscores:
return None, None, None
boxes = np.concatenate(nboxes)
classes = np.concatenate(nclasses)
scores = np.concatenate(nscores)
return boxes, classes, scores
解码
解码函数process每次接收的参数为特征图input,及对应的掩码mask和所有的anchors,下面表格从上到下依次为process接收的参数(input太长仅展示尺寸)
python
b, c, s = process(input, mask, anchors)
input的尺寸 | mask | anchors |
---|---|---|
(80, 80, 3, 85) | [0, 1, 2] | [[10, 13], [16, 30], [33, 23], [30, 61], [62, 45], [59, 119], [116, 90], [156, 198], [373, 326]] |
(40, 40, 3, 85) | [3, 4, 5] | [[10, 13], [16, 30], [33, 23], [30, 61], [62, 45], [59, 119], [116, 90], [156, 198], [373, 326]] |
(20, 20, 3, 85) | [6, 7, 8] | [[10, 13], [16, 30], [33, 23], [30, 61], [62, 45], [59, 119], [116, 90], [156, 198], [373, 326]] |
对于每一个特征图,先利用mask提取对应特征图的anchors
python
anchors = [anchors[i] for i in mask]
三次调用process时不同尺寸特征图对应的anchors为:
(80, 80, 3, 85) 对应的anchors为:[[10, 13], [16, 30], [33, 23]]
(40, 40, 3, 85) 对应的anchors为:[[30, 61], [62, 45], [59, 119]]
(20, 20, 3, 85) 对应的anchors为:[[116, 90], [156, 198], [373, 326]]
这是因为:
80 x 80为浅层的特征图,包含较多的低层级信息,适合用于检测小目标,所以这一特征图所用的anchors尺度较小;
20 x 20为深层的特征图,包含更多高层级的信息,如轮廓、结构等信息,适合用于大目标的检测,所以这一特征图所用的anchors尺度较大。
40 x 40特征图介于上面两个尺度之间的anchors用来检测中等大小的目标
接着读取每个输出特征图的尺寸:
python
grid_h, grid_w = map(int, input.shape[0:2])
三次调用process时grid_h, grid_w的值为:(80, 80)、(40, 40)、(20, 20)
根据grid_h, grid_w获取特征图的尺寸,我们便可以创建一个grid_h * grid_w * 3 * 2的坐标格,创建了一个包含每个参与预测的网格单元格中心点所在格子的左上角坐标数组。举个例子:以(80, 80, 3, 85) 其中80 x 80为一张图划分的单元格的数量,但是每个单元格有三个anchors,即参与预测的网格单元格的数量为80 x 80 x 3
python
col = np.tile(np.arange(0, grid_w), grid_w).reshape(-1, grid_w)
row = np.tile(np.arange(0, grid_h).reshape(-1, 1), grid_h)
col = col.reshape(grid_h, grid_w, 1, 1).repeat(3, axis=-2)
row = row.reshape(grid_h, grid_w, 1, 1).repeat(3, axis=-2)
grid = np.concatenate((col, row), axis=-1)
三次调用process时,这里我们生成的grid的尺寸以此为:(80, 80, 3, 2)、(40, 40, 3, 2)、(20, 20, 3, 2) 后面的2为每个单元格的的左上角坐标。
预测框的中心点坐标的解码公式为:
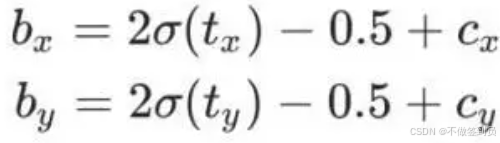
tx和ty为模型预测的格子的中心点相对坐标,cx和cy每个单元格的的左上角坐标;对于每个预测框中心点的解码,在YOLOv3中是使用sigmoid函数将预测的中心点的值约束在0到1之间,然后加上网格grid的左上角的点的坐标,但是在YOLOv5中作者又考虑到如果预测点落在网格线上是需要取到0或者1,但是sigmoid则需要取到负无穷或正无穷,因此已经使用2*sigmoid(x)-0.5代替sigmoid(x)了。
由于在rockchip在yolov5模型导出为onnx的时候,修改了yolo.py代码,将其中的forward函数修改为了下面的代码
python
def forward(self, x):
z = [] # inference output
for i in range(self.nl):
z.append(torch.sigmoid(self.m[i](x[i])))
return z
所以输出的特征图已经被sigmoid作用,所以在解码公式中的sigmoid作用便可以省去
python
# yolov5/models/yolo.py Detect forward
# 预测框的x或y = (x或y * 2. - 0.5 + grid) * 格子的实际尺寸 # xy
box_xy = input[..., :2]*2 - 0.5
box_xy += grid
box_xy *= int(IMG_SIZE/grid_h)
因此参照前面的公式,最后利用实际的图像尺寸与划分的格子的尺寸比值,得到在实际图像中的格子尺寸便得到了真正的检测框的中心点值
预测框的尺寸的解码公式为:
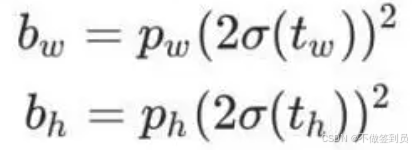
tw和th模型预测的格子尺寸的相对值,pw和ph为对应的anchors值
python
# wh = (wh.sigmoid() * 2) ** 2 * self.anchor_grid[i]
box_wh = pow(input[..., 2:4]*2, 2) * anchors
上面的pow(..., 2): 这是一个内置函数,用于计算上述乘法结果的平方。pow(x, y)相当于x**y。
python
box = np.concatenate((box_xy, box_wh), axis=-1)
将解码出来的预测框的中心点坐标和框的尺寸拼接到一起,则三次执行process函数输出的box的维度为:(80, 80, 3, 4)、(40, 40, 3, 4)、(20, 20, 3, 4)
获取每次调用process函数时的是否含有物体的概率,以(80, 80, 3, 85)的特征图为例,只取最后一个维度的一个值相当于现在只有了(80, 80, 3)个值,新增一个维度
python
box_confidence = input[..., 4]
box_confidence = np.expand_dims(box_confidence, axis=-1)
即三次执行process函数输出的box_confidence依次为(80, 80, 3, 1)、(40, 40, 3, 1)、(20, 20, 3, 1)
接着取每个特征图在有物体的条件下80个类别各自的条件概率
python
box_class_probs = input[..., 5:]
即三次执行process函数输出的box_class_probs依次为(80, 80, 3, 80)、(40, 40, 3, 80)、(20, 20, 3, 80)
最后返回以下结果
- box:该特征图检测框的信息(中心点坐标和框的大小)
- box_confidence:该特征图的预测框是否含有物体的概率
- box_class_probs:该特征图的预测框在有物体的条件下80个类别各自的条件概率
process的代码如下所示:
python
def process(input, mask, anchors):
anchors = [anchors[i] for i in mask]
grid_h, grid_w = map(int, input.shape[0:2])
col = np.tile(np.arange(0, grid_w), grid_w).reshape(-1, grid_w)
row = np.tile(np.arange(0, grid_h).reshape(-1, 1), grid_h)
col = col.reshape(grid_h, grid_w, 1, 1).repeat(3, axis=-2)
row = row.reshape(grid_h, grid_w, 1, 1).repeat(3, axis=-2)
grid = np.concatenate((col, row), axis=-1)
box_xy = input[..., :2]*2 - 0.5
box_xy += grid
box_xy *= int(IMG_SIZE/grid_h)
box_wh = pow(input[..., 2:4]*2, 2) * anchors
box = np.concatenate((box_xy, box_wh), axis=-1)
box_confidence = input[..., 4]
box_confidence = np.expand_dims(box_confidence, axis=-1)
box_class_probs = input[..., 5:]
return box, box_confidence, box_class_probs
低阈值过滤
filter_boxes低置信度过滤接受的参数为process的返回值,使用下面的表格展示为三次process的输出的boxes, box_confidences, box_class_probs的各个维度;
前面提到过将85分成了 4(检测框的信息) + 1(包含物体的置信度) + 80(80个类别的条件概率),在这里更进一步有体现
boxes的维度 | box_confidences的维度 | box_class_probs的维度 |
---|---|---|
(80, 80, 3, 4) | (80, 80, 3, 1) | (80, 80, 3, 80) |
(40, 40, 3, 4) | (40, 40, 3, 1) | (40, 40, 3, 80) |
(20, 20, 3, 4) | (20, 20, 3, 1) | (20, 20, 3, 80) |
首先将boxes, box_confidences, box_class_probs进行维度变换,将前三个维度合并;举个例子:(80, 80, 3, 4),最后一个维度的4为预测框的信息,预测框的个数为80 x 80 x 3 = 19200个,将前三个维度合并即是将(80, 80, 3, 4)给reshape成(19200, 4)(是NumPy库中的reshape
方法通常用于对一个多维数组或张量进行重塑)
python
boxes = boxes.reshape(-1, 4)
box_confidences = box_confidences.reshape(-1)
box_class_probs = box_class_probs.reshape(-1, box_class_probs.shape[-1])
这里boxes和box_confidences以及box_class_probs使用reshape的时候第一个维度指定了-1
表示自动计算这个维度的大小,以确保总元素数量保持不变;
boxes的第二个维度指定为固定值4,他是预测框的中心点和框的尺寸信息,而box_class_probs的第二个维度并没有指定固定的值,因为默认权重使用的是COCO数据集的80分类,但是其他数据集可能并不是80分类,随意需要因数据集情况而变;
最终执行三次filter_boxes函数依次得到的boxes, box_confidences, box_class_probs维度变换的结果如下所示:
boxes reshape | box_confidences reshape | box_class_probs reshape |
---|---|---|
(19200, 4) | (19200,) | (19200, 80) |
(4800, 4) | (4800,) | (4800, 80) |
(1200, 4) | (1200,) | (1200, 80) |
其中19200 = 80 x 80 x 3 、4800 = 40 x 40 x 3、1200 = 20 x 20 x 3
前面提到过:box_confidences为预测框所包含物体的置信度,利用numpy的条件索引where
函数,当预测框所包含的物体置信度大于OBJ_THRESH(0.25)的时候返回预测框的索引
python
_box_pos = np.where(box_confidences >= OBJ_THRESH)
不同特征图对预测框包含物体的置信度进行过滤后,得到的_box_pos的结果如下所示:
-
(array([], dtype=int64),)
-
(array([2687, 2789, 2792, 2807, 2810, 2858, 2861, 2909, 2912, 2927, 2978, 3138, 3140, 3258, 3260]),)
-
(array([508, 509, 511, 512, 567, 568, 569, 570, 571, 572, 672, 673, 675, 676, 677, 681, 682, 684, 685, 705, 706, 708, 709, 729, 730, 732, 733, 734, 735, 736, 737, 741, 742, 768, 769, 786, 787, 789, 790]),)
根据对预测框包含物体的置信度的过滤后得到的预测框索引,提取出余下的预测框的有效信息
python
boxes = boxes[_box_pos]
box_confidences = box_confidences[_box_pos]
box_class_probs = box_class_probs[_box_pos]
下面为经过过滤后余下的预测框的维度变化表:
boxes 过滤后的shape | box_confidences 过滤后的shape | box_class_probs 过滤后的shape |
---|---|---|
(19200, 4) -> (0, 4) | (19200,) -> (0,) | (19200, 80) -> (0, 80) |
(4800, 4) -> (15, 4) | (4800,) -> (15,) | (4800, 80) -> (15, 80) |
(1200, 4) -> (39, 4) | (1200,) -> (39,) | (1200, 80) -> (39, 80) |
可以发现第一个尺寸的特征图的预测框经过过滤后,由19200个变成了0个,第二个尺寸的特征图的预测框经过过滤后,由4800个变成了15个,第三个尺寸的特征图的预测框经过过滤后,由1200个变成了39个
上面使用了预测框是否包含物体的置信度进行了过滤,得到了余下的框,下面将根据每个预测框的所有类别的条件概率值进行第二次过滤
python
class_max_score = np.max(box_class_probs, axis=-1)
'''
0个:
[]
15个:
[0.9792088 0.9948762 0.99095935 0.97137517 0.951791 0.9792088
0.98704255 0.998793 0.998793 0.90870583 0.9948762 0.8656206
0.92437315 0.90870583 0.9635415 ]
39个:
[0.9794845 0.9834025 0.9794845 0.9834025 0.9363872 0.97164863
0.9834025 0.95989484 0.9834025 0.9873204 0.9951563 0.9951563
0.9951563 0.9951563 0.9990742 0.9403051 0.95989484 0.9834025
0.99123836 0.97164863 0.99123836 0.9794845 0.99123836 0.9167975
0.96381277 0.9990742 0.9990742 0.9990742 0.9990742 0.9990742
0.9990742 0.93246925 0.97164863 0.99123836 0.9951563 0.9677307
0.9834025 0.9559769 0.97556657]
'''
_class_pos = np.where(class_max_score >= OBJ_THRESH)
'''
(array([], dtype=int64),)
(array([ 0, 1, 2, 3, 4, 5, 6, 7, 8, 9, 10, 11, 12, 13, 14]),) 15个
(array([ 0, 1, 2, 3, 4, 5, 6, 7, 8, 9, 10, 11, 12, 13, 14, 15, 16,
17, 18, 19, 20, 21, 22, 23, 24, 25, 26, 27, 28, 29, 30, 31, 32, 33, 34, 35, 36, 37, 38]),) 39个
'''
box_class_probs 是一个形状为 [n, c] 的数组,其中 n 是预测框的数量,c 是所有类别的条件概率值,那么 axis=-1 (axis=-1: 指定了沿着数组的最后一个轴进行操作。)将计算每个预测框中所有类别的最大的条件概率值。当该预测框的所有类别的最大条件概率值概率大于OBJ_THRESH(0.25)的时候 ,返回框的索引;从上面的_class_pos返回的索引结果上来看,第二次过滤没有过滤掉预测框;
由于我们最终需要返回预测框预测物体的类别,所以使用 NumPy 的 argmax 函数来确定 box_class_probs 数组中每个边界框最可能的类别索引,数组 classes 的形状将是 [n, ],其中每个元素是对应边界框在 box_class_probs 中概率最高类别的索引。axis=-1: 这个参数指定了 argmax 函数沿着数组的最后一个轴(即80个类别的概率分布)进行操作。这意味着对于每个边界框(不考虑其他维度),argmax 将找到所有类别概率最高的类别索引。然后在根据第二次过滤预测框的结果保留余下的预测框的类别;
python
classes = np.argmax(box_class_probs, axis=-1)
'''
打印classes的值为:
[]
[0 0 0 0 0 0 0 0 0 0 0 0 0 0 0]
[5 5 5 5 5 5 5 5 5 5 0 0 0 0 0 0 0 0 0 0 0 0 0 0 0 0 0 0 0 0 0 0 0 0 0 0 0 0 0]
打印classes的维度为:
(0,)
(15,)
(39,)
'''
classes = classes[_class_pos]
'''
打印classes的维度为: 由于前面我们发现第二次过滤时没有过滤掉人何框,所以classes的维度没有变
(0,)
(15,)
(39,)
'''
根据第二次过滤得到的索引保存余下的预测框
python
boxes = boxes[_class_pos]
'''
boxes的维度:
(0, 4)
(15, 4)
(39, 4)
'''
计算预测框预测的概率最大的类别的得分,box_confidences为预测框是否有物体的概率值,class_max_score为在预测框有物体的情况下是80个类别的最大的一个类别的条件概率,故class_max_score * box_confidences为预测框预测为最大概率物品的全概率值;
python
scores = (class_max_score * box_confidences)[_class_pos]
'''
scores的维度为:
(0,)
(15,)
(39,)
'''
最终我们将boxes过滤后的预测框的信息, classes过滤后的预测框预测的物体类别, scores过滤后的预测框预测的物体类别的概率值返回;
filter_boxes的代码如下所示:
python
def filter_boxes(boxes, box_confidences, box_class_probs):
boxes = boxes.reshape(-1, 4)
box_confidences = box_confidences.reshape(-1)
box_class_probs = box_class_probs.reshape(-1, box_class_probs.shape[-1])
_box_pos = np.where(box_confidences >= OBJ_THRESH)
boxes = boxes[_box_pos]
box_confidences = box_confidences[_box_pos]
box_class_probs = box_class_probs[_box_pos]
class_max_score = np.max(box_class_probs, axis=-1)
_class_pos = np.where(class_max_score >= OBJ_THRESH)
classes = np.argmax(box_class_probs, axis=-1)
classes = classes[_class_pos]
boxes = boxes[_class_pos]
scores = (class_max_score* box_confidences)[_class_pos]
return boxes, classes, scores
NMS非极大值抑制
nms_boxes进行非极大值抑制,接受的参数为相同类别的预测框的信息、置信度
前面我们讲过在进行非极大值抑制之前,预测框的box由中心点坐标和尺寸信息的形式变成了用左上角坐标和右下角坐标表示的形式,在进行非极大值抑制的时候
需要计算预测框的尺寸,使用左上角坐标和右下角坐标的横坐标和纵坐标差值便可以求出所有预测框的尺寸
python
# 现在的坐标形式为 x1,y1 框的左上角坐标 x2,y2 框的右下角坐标
x = boxes[:, 0]
y = boxes[:, 1]
w = boxes[:, 2] - boxes[:, 0]
h = boxes[:, 3] - boxes[:, 1]
此时x和y为所有预测框的左上角坐标的横纵坐标值,w和h为所有预测框的宽高值,接着利用所有预测框的宽高值求出所有预测框的面积
python
areas = w * h # 所有框的面积
对所有预测框的置信度值按从大到小的方式进行排序,并返回索引
python
order = scores.argsort()[::-1]
argsort 函数返回的是数组 scores 中元素从小到大的索引值 [::-1]: 这是 Python 中的切片操作,用于对数组或列表进行逆序。order 将包含 scores 数组中元素从大到小的索引。
新建一个列表keep用于存储进行非极大值抑制后得到的索引结果
使用while循环,只要预测框的置信度值的索引排序表不是空的就一直遍历
python
while order.size > 0:
由于置信度是按照从大到小排序的,排序的第一个值便是置信度最大的预测框的索引,直接将其加入到keep数组
python
i = order[0] # 选择置信度最大的框
keep.append(i)
利用当前选中的置信度最大的索引取出概况的左上角坐标和后面其他框的索引的左上角坐标进行比较选出值最大的即为xx1和yy1,同理右下角坐标的比较,计算出所有的xx2和yy2
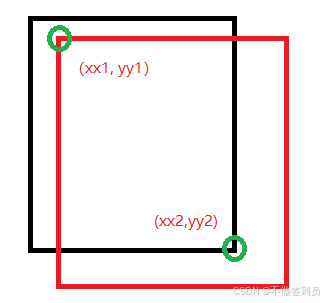
python
xx1 = np.maximum(x[i], x[order[1:]]) # 取当前边界框和排序后其他边界框的较大值。
yy1 = np.maximum(y[i], y[order[1:]])
xx2 = np.minimum(x[i] + w[i], x[order[1:]] + w[order[1:]]) # 取当前边界框和排序后其他边界框的较小值。
yy2 = np.minimum(y[i] + h[i], y[order[1:]] + h[order[1:]])
计算出当前置信度最大值的预测框和后面框的交集区域的面积
python
# 此时 xx1 xx2 以及 yy1 yy2夹的便是两个框的交的部分
w1 = np.maximum(0.0, xx2 - xx1 + 0.00001)
h1 = np.maximum(0.0, yy2 - yy1 + 0.00001)
# inter: 计算所有的交集区域的面积,即宽度和高度的乘积。
inter = w1 * h1
计算交并比(IoU),这是两个边界框交集面积与并集面积的比值,其中交集的面积在上面的inter已经计算出来,并集面积是两个边界框面积之和减去交集面积。
python
ovr = inter / (areas[i] + areas[order[1:]] - inter)
计算交并比后,根据使用 np.where 函数找到交并比小于等于阈值 NMS_THRESH 的索引,更新 order 数组,只保留交并比小于等于阈值的边界框的索引。
python
inds = np.where(ovr <= NMS_THRESH)[0]
这里要注意的是inds的到的索引值是除去第一个预测框后,剩余满足要求的预测框的索引值,所以如果要在列表中取保存这些满足要求的预测框需要+1+,因为 是因为order列表中包含第一个边界框,而第一个预测框已经被收录进了keep列表,在nms的时候(即置信度最高的边界框)不会被抑制,所以不需要包括在内。
python
order = order[inds + 1]
即接着再用次大的框去抑制后面的框,最后循环结束,将keep列表转换成为数组的各式并返回;
举一个完整的流程来描述这个NMS的实现过程,在前面打印set(classes)的值为{0, 5},说明只有这两个类需要进行非极大值抑制,以第0类为例:
刚开始循环的时候,属于第0类的keep、order和order[1:]的情况如下所示:
keep = []
order = [21 8 27 20 34 26 7 33 35 37 23 22 25 36 5 18 3 24 17 39 38 19 10 1 31 30 9 32 40 42 6 15 16 28 0 43 4 2 41 12 29 14 11 13]
i = order[0] = 21
keep = [21]
order[1:] = [ 8 27 20 34 26 7 33 35 37 23 22 25 36 5 18 3 24 17 39 38 19 10 1 31 30 9 32 40 42 6 15 16 28 0 43 4 2 41 12 29 14 11 13]
执行了nms过滤后inds的值为:
inds = [ 0 1 3 4 5 6 7 11 13 14 16 17 18 19 20 21 22 23 24 26 27 28 29 30 31 32 34 36 37 38 39 40 41 42]
inds + 1 = [ 1 2 4 5 6 7 8 12 14 15 17 18 19 20 21 22 23 24 25 27 28 29 30 31 32 33 35 37 38 39 40 41 42 43]
执行order = order[inds + 1]后,开始新一轮循环:
新二轮循环的时候,属于第0类的keep、order和order[1:]的情况如下所示:
keep = [21]
order = [ 8 27 34 26 7 33 35 25 5 18 24 17 39 38 19 10 1 31 30 32 40 42 6 15 16 28 43 2 41 12 29 14 11 13]
i = order[0] = 8
keep = [21, 8]
order[1:] = [ 27 20 34 26 7 33 35 37 23 22 25 36 5 18 3 24 17 39 38 19 10 1 31 30 9 32 40 42 6 15 16 28 0 43 4 2 41 12 29 14 11 13]
执行了nms过滤后inds的值为:
inds = [ 0 2 6 7 9 11 12 14 19 20 21 24 25 27 28 29 30 31 32]
inds + 1 = [ 1 3 7 8 10 12 13 15 20 21 22 25 26 28 29 30 31 32 33]
执行order = order[inds + 1]后,开始新一轮循环:
新三轮循环的时候,属于第0类的keep、order和order[1:]的情况如下所示:
keep = [21, 8]
order = [27 26 25 5 24 39 38 10 40 42 6 28 43 41 12 29 14 11 13]
i = order[0] = 27
keep = [21, 8, 27]
order[1:] = [26 25 5 24 39 38 10 40 42 6 28 43 41 12 29 14 11 13]
执行了nms过滤后inds的值为:
inds = [ 7 8 10 11 12 13 14 15 16 17]
inds + 1 = [ 8 9 11 12 13 14 15 16 17 18]
执行order = order[inds + 1]后,开始新一轮循环:
新四轮循环的时候,属于第0类的keep、order和order[1:]的情况如下所示:
keep = [21, 8, 27]
order = [40 42 28 43 41 12 29 14 11 13]
i = order[0] = 40
keep = [21, 8, 27, 40]
order[1:] = [42 28 43 41 12 29 14 11 13]
执行了nms过滤后inds的值为:
inds = []
inds + 1 = []
执行order = order[inds + 1]后,此时order = [],此时满足循环终止条件,循环终止,此时对于第0类,经过非极大值抑制后剩余的预测框的索引为keep = [21, 8, 27,40]
nms_boxes的函数实现为:
python
def nms_boxes(boxes, scores):
# 现在的坐标形式为 x1,y1 框的左上角坐标 x2,y2 框的右下角坐标
x = boxes[:, 0]
y = boxes[:, 1]
w = boxes[:, 2] - boxes[:, 0]
h = boxes[:, 3] - boxes[:, 1]
areas = w * h # 所有框的面积
order = scores.argsort()[::-1]
keep = []
while order.size > 0:
i = order[0] # 选择置信度最大的框
keep.append(i)
xx1 = np.maximum(x[i], x[order[1:]]) # 取当前边界框和排序后其他边界框的较大值。
yy1 = np.maximum(y[i], y[order[1:]])
xx2 = np.minimum(x[i] + w[i], x[order[1:]] + w[order[1:]]) # 取当前边界框和排序后其他边界框的较小值。
yy2 = np.minimum(y[i] + h[i], y[order[1:]] + h[order[1:]])
# 此时 xx1 xx2 以及 yy1 yy2夹的便是两个框的交的部分
w1 = np.maximum(0.0, xx2 - xx1 + 0.00001)
h1 = np.maximum(0.0, yy2 - yy1 + 0.00001)
# inter: 计算所有的交集区域的面积,即宽度和高度的乘积。
inter = w1 * h1
# ovr: 计算交并比(IoU,Intersection over Union),这是两个边界框交集面积与并集面积的比值。
# 并集面积是两个边界框面积之和减去交集面积。
ovr = inter / (areas[i] + areas[order[1:]] - inter)
# 使用 np.where 函数找到交并比小于等于阈值 NMS_THRESH 的索引。
inds = np.where(ovr <= NMS_THRESH)[0]
# 更新 order 数组,只保留交并比小于等于阈值的边界框的索引。
# + 1 是因为通常在 NMS 过程中,第一个边界框(即置信度最高的边界框)不会被抑制,所以不需要包括在内。
# 即接着再用次大的框去抑制后面的框
order = order[inds + 1]
keep = np.array(keep)
return keep
结果绘制
对模型推理出来的特征进行后处理后得到预测框boxes维度为(5, 4),预测框预测的物体类别classes维度为(5,),以及预测框预测的物体类别的置信度得分scores的维度为(5,)
python
boxes, classes, scores = yolov5_post_process(input_data)
将结果绘制到图像数据上,由于我们的输入数据可能不是640 x 640但是我们在推理的时候将其转化成640 x 640尺寸的图像进行推理,得到的结果也均与640 x 640有关,如要想绘制到原输入图像上,需要进行获取预测框在原始图像上的尺寸
python
img_src = cv2.imread(IMG_PATH)
src_shape = img_src.shape[:2]
下面为结果绘制的代码
python
if boxes is not None:
boxes = get_real_box(src_shape, boxes, dw, dh, ratio)
draw(img_src, boxes, scores, classes)
cv2.imwrite('result.jpg', img_src)
print('Save results to result.jpg!')
get_real_box为计算原始图像填充为640 x 640图像后得到的box,在原始图像上的box尺寸,它根据输入的原始图像、缩放宽度(dw
)、缩放高度(dh
)和缩放比例(ratio
)来调整预测框在原始图像上的位置和大小,确保它们在原始图像的尺寸范围内。下面是代码的大致流程为:
-
首先,每个坐标减去
dw
或dh
,这可能是为了调整边界框的位置。 -
然后,坐标除以
ratio
,这可能是为了根据缩放比例调整边界框的大小。 -
最后,使用
np.clip
函数将调整后的坐标限制在原始图像的尺寸范围内,确保边界框不会超出图像边界。
python
def get_real_box(src_shape, box, dw, dh, ratio):
bbox = copy(box) # 创建了输入边界框 box 的一个副本,以避免直接修改原始数据。
# unletter_box result
bbox[:,0] -= dw
bbox[:,0] /= ratio
bbox[:,0] = np.clip(bbox[:,0], 0, src_shape[1])
bbox[:,1] -= dh
bbox[:,1] /= ratio
bbox[:,1] = np.clip(bbox[:,1], 0, src_shape[0])
bbox[:,2] -= dw
bbox[:,2] /= ratio
bbox[:,2] = np.clip(bbox[:,2], 0, src_shape[1])
bbox[:,3] -= dh
bbox[:,3] /= ratio
bbox[:,3] = np.clip(bbox[:,3], 0, src_shape[0])
return bbox
最后将原始输入图像以及在原始输入图像上的预测框的尺寸、预测的物体置信度得分、预测的物体传入进行检测结果的绘制
python
def draw(image, boxes, scores, classes):
print("{:^12} {:^12} {}".format('class', 'score', 'xmin, ymin, xmax, ymax'))
print('-' * 50)
for box, score, cl in zip(boxes, scores, classes):
top, left, right, bottom = box
top = int(top)
left = int(left)
right = int(right)
bottom = int(bottom)
cv2.rectangle(image, (top, left), (right, bottom), (255, 0, 0), 2)
cv2.putText(image, '{0} {1:.2f}'.format(CLASSES[cl], score),
(top, left - 6),
cv2.FONT_HERSHEY_SIMPLEX,
0.6, (0, 0, 255), 2)
print("{:^12} {:^12.3f} [{:>4}, {:>4}, {:>4}, {:>4}]".format(CLASSES[cl], score, top, left, right, bottom))
print("{:^12} {:^12.3f} [{:>4}, {:>4}, {:>4}, {:>4}]".format(CLASSES[cl], score, top, left, right, bottom))打印的结果如下所示:
class score xmin, ymin, xmax, ymax
--------------------------------------------------
person 0.820 [ 210, 241, 284, 518]
person 0.806 [ 114, 233, 208, 546]
person 0.804 [ 474, 230, 560, 522]
person 0.436 [ 79, 336, 121, 515]
bus 0.774 [ 88, 129, 556, 467]
参考: