打卡
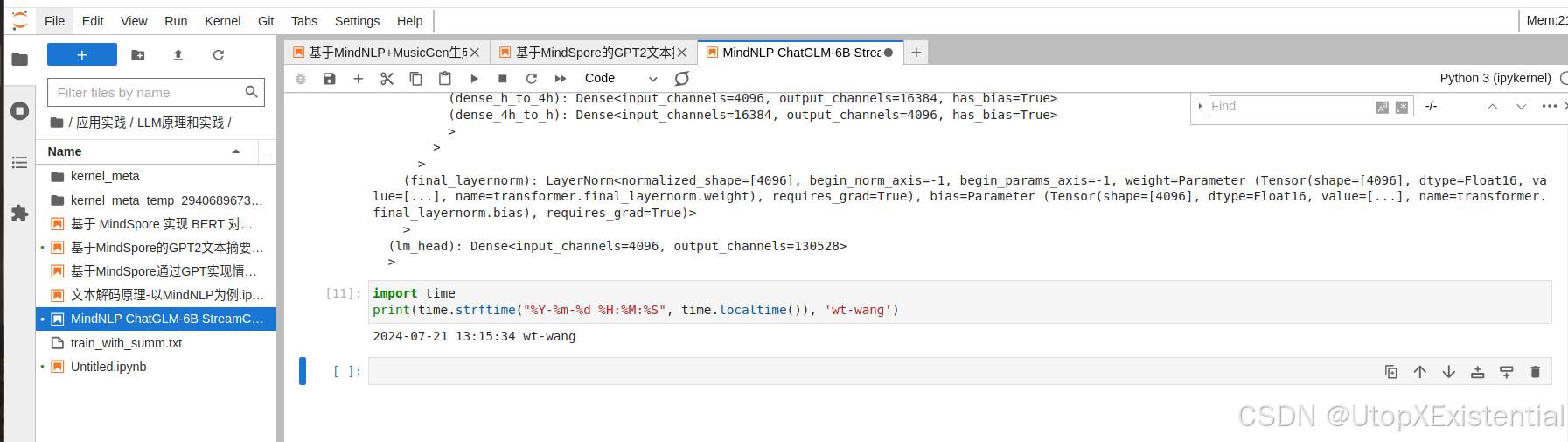
目录
[ChatGLM-6B 体验截图示例](#ChatGLM-6B 体验截图示例)
[ChatGLM-6B 模型结构解析如下](#ChatGLM-6B 模型结构解析如下)
[ChatGLM2-6B 模型结构解析如下](#ChatGLM2-6B 模型结构解析如下)
任务说明
加载智谱清言的chatglm模型权重文件(目前有4个版本),本次主要尝试了chatglm-6b。
chatglm 6b 在提供的本次实验npu卡中可以正常加载,加载2和3版本时加载tokenizer出错了。但是可以看到model的打印结果,看到chatglm2 和 chatglm3 的模型结构相比1版本,词表扩充了2w+。而我们知道chatglm3-6b 还具有了 functional calling 功能。
环境配置
- 欧拉操作系统2.0(SP8);
- python3.9;
- 华为 NPU 910A;
- mindnlp, mindspore, mindvision 安装部署。
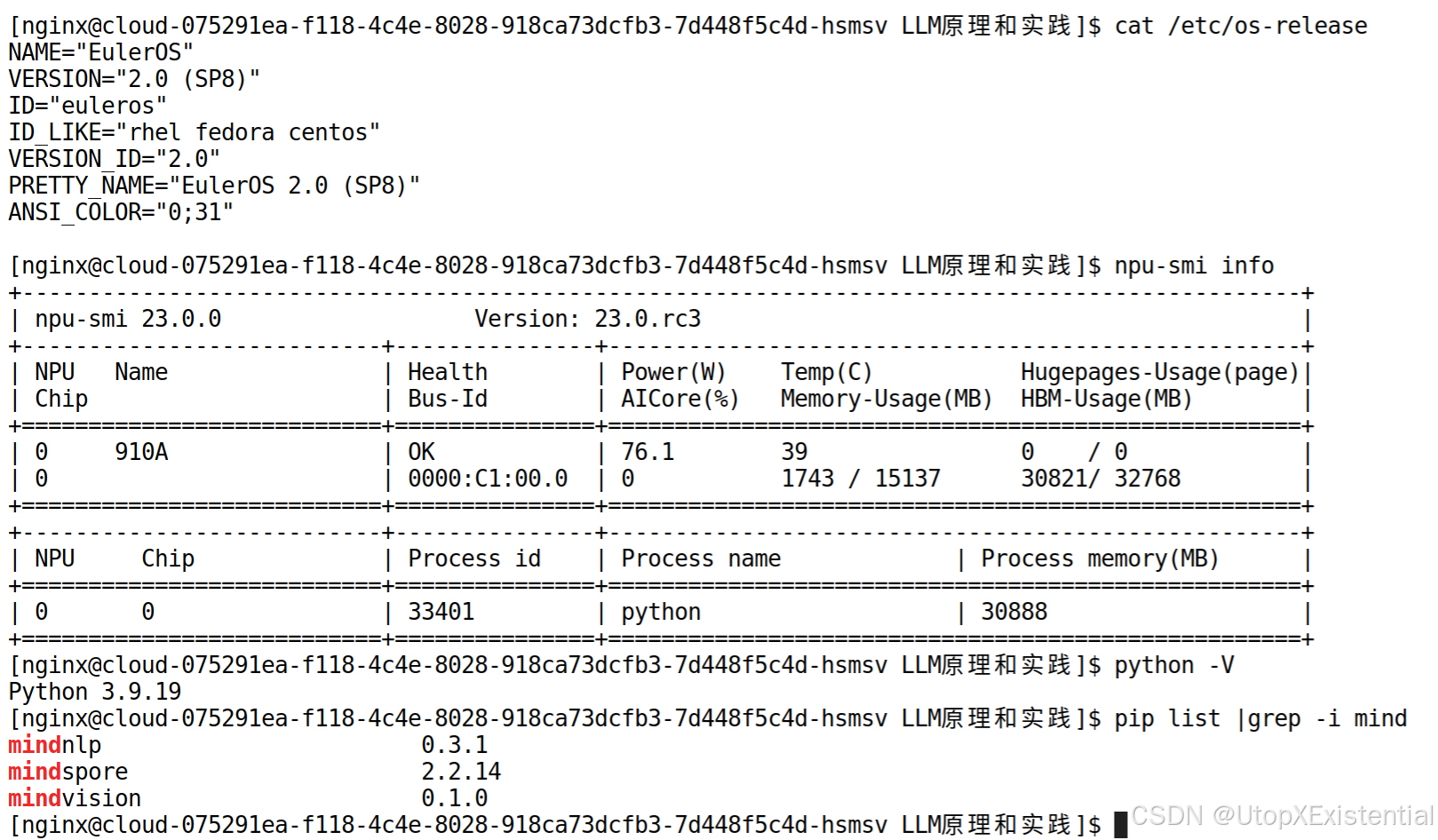
- 其余安装:
python
!pip install mdtex2html
!pip install -i https://pypi.mirrors.ustc.edu.cn/simple mindspore==2.2.14
- 变量设置:HF_ENDPOINT=https://hf-mirror.com
部署方式
python
from mindnlp.transformers import AutoModelForSeq2SeqLM, AutoTokenizer
import gradio as gr
import mdtex2html
### 下载 && 加载模型权重
model = AutoModelForSeq2SeqLM.from_pretrained(
'ZhipuAI/ChatGLM-6B',
mirror="modelscope").half()
model.set_train(False)
tokenizer = AutoTokenizer.from_pretrained(
'ZhipuAI/ChatGLM-6B',
mirror="modelscope")
### 修改参数和prompt体验模型
prompt = '你好'
history = []
response, _ = model.chat(tokenizer, prompt, history=history, max_length=20)
response
ChatGLM-6B体验截图示例
如上图,ChatGLM-6B tokenzier 的词表大小是 13w+,有5种特殊的 token。
如下为模型打印结果。
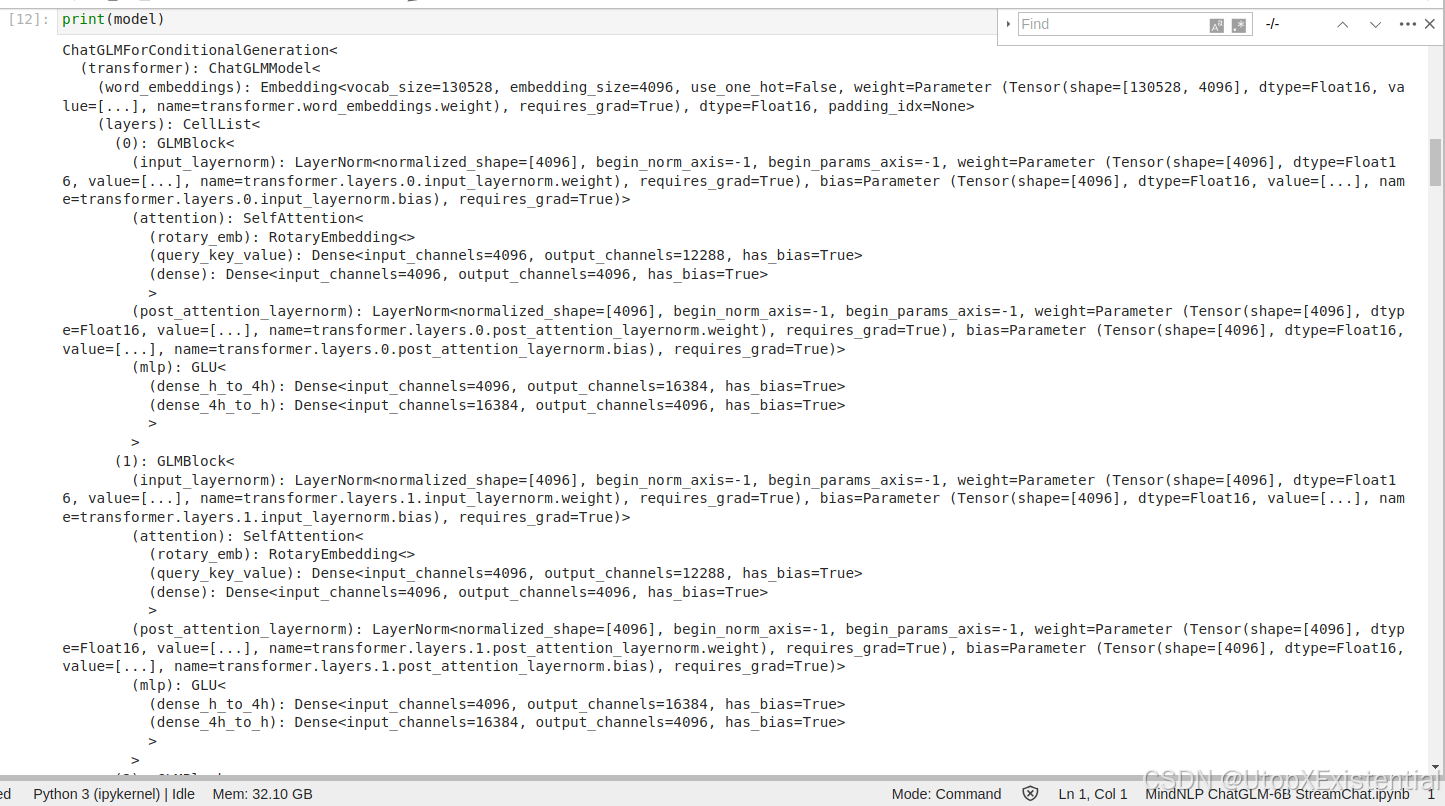
ChatGLM-6B 模型结构解析如下
1. 1级主类: ChatGLMForConditionalGeneration 生成式Transformer模型,用于条件文本生成。
2. 2级类: ChatGLMModel 层,是transformer 结构,是模型的核心部分。
3. 2级类: lm_head 结构的 Dense 全连接层。dim[in, out]=[4096, 130528]
4. ChatGLMModel 结构下的3级类组件分三层:
>> word_embeddings 嵌入层:dim[in, out]=[130528, 4096] ,即使用了 130528 个词汇,每个词汇映射到一个4096维的向量。
>> layers 网络结构层 :Transformer模型的主体,包含 28 个GLMBlock。
>> final_layernorm最后的层归一化。
5. GLMBlock 的结构:
》》1 input_layernorm层,层归一化,用于在注意力机制之前对输入进行归一化。
》》2 SelfAttention层,自注意力机制,用于计算输入序列中不同位置的注意力权重。共包括3层:RotaryEmbedding 旋转嵌入,用于增强模型对位置信息的处理能力; Dense(query_key_value)用于生成查询(Q)、键(K)和值(V)向量;Dense(Dense)用于自注意力机制的输出。。
》》3 post_attention_layernorm层,用于自注意力之后的归一化。
》》4 mlp 层 ,多层感知机,用于对自注意力层的输出进行进一步的非线性变换。这里的MLP使用的是GLU(门控线性单元)激活函数。dense_h_to_4h
线性变换将输入维度扩大4倍。dense_4h_to_h
将扩大后的维度还原。
如下图,chatglm-6b model 的打印结果。
python
$ print(model)
ChatGLMForConditionalGeneration<
(transformer): ChatGLMModel<
(word_embeddings): Embedding<vocab_size=130528, embedding_size=4096, use_one_hot=False, weight=Parameter (Tensor(shape=[130528, 4096], dtype=Float16, value=[...], name=transformer.word_embeddings.weight), requires_grad=True), dtype=Float16, padding_idx=None>
(layers): CellList<
(0): GLMBlock<
(input_layernorm): LayerNorm<normalized_shape=[4096], begin_norm_axis=-1, begin_params_axis=-1, weight=Parameter (Tensor(shape=[4096], dtype=Float16, value=[...], name=transformer.layers.0.input_layernorm.weight), requires_grad=True), bias=Parameter (Tensor(shape=[4096], dtype=Float16, value=[...], name=transformer.layers.0.input_layernorm.bias), requires_grad=True)>
(attention): SelfAttention<
(rotary_emb): RotaryEmbedding<>
(query_key_value): Dense<input_channels=4096, output_channels=12288, has_bias=True>
(dense): Dense<input_channels=4096, output_channels=4096, has_bias=True>
>
(post_attention_layernorm): LayerNorm<normalized_shape=[4096], begin_norm_axis=-1, begin_params_axis=-1, weight=Parameter (Tensor(shape=[4096], dtype=Float16, value=[...], name=transformer.layers.0.post_attention_layernorm.weight), requires_grad=True), bias=Parameter (Tensor(shape=[4096], dtype=Float16, value=[...], name=transformer.layers.0.post_attention_layernorm.bias), requires_grad=True)>
(mlp): GLU<
(dense_h_to_4h): Dense<input_channels=4096, output_channels=16384, has_bias=True>
(dense_4h_to_h): Dense<input_channels=16384, output_channels=4096, has_bias=True>
>
>
(1): GLMBlock<
(input_layernorm): LayerNorm<normalized_shape=[4096], begin_norm_axis=-1, begin_params_axis=-1, weight=Parameter (Tensor(shape=[4096], dtype=Float16, value=[...], name=transformer.layers.1.input_layernorm.weight), requires_grad=True), bias=Parameter (Tensor(shape=[4096], dtype=Float16, value=[...], name=transformer.layers.1.input_layernorm.bias), requires_grad=True)>
(attention): SelfAttention<
(rotary_emb): RotaryEmbedding<>
(query_key_value): Dense<input_channels=4096, output_channels=12288, has_bias=True>
(dense): Dense<input_channels=4096, output_channels=4096, has_bias=True>
>
(post_attention_layernorm): LayerNorm<normalized_shape=[4096], begin_norm_axis=-1, begin_params_axis=-1, weight=Parameter (Tensor(shape=[4096], dtype=Float16, value=[...], name=transformer.layers.1.post_attention_layernorm.weight), requires_grad=True), bias=Parameter (Tensor(shape=[4096], dtype=Float16, value=[...], name=transformer.layers.1.post_attention_layernorm.bias), requires_grad=True)>
(mlp): GLU<
(dense_h_to_4h): Dense<input_channels=4096, output_channels=16384, has_bias=True>
(dense_4h_to_h): Dense<input_channels=16384, output_channels=4096, has_bias=True>
>
>
(2): GLMBlock<
(input_layernorm): LayerNorm<normalized_shape=[4096], begin_norm_axis=-1, begin_params_axis=-1, weight=Parameter (Tensor(shape=[4096], dtype=Float16, value=[...], name=transformer.layers.2.input_layernorm.weight), requires_grad=True), bias=Parameter (Tensor(shape=[4096], dtype=Float16, value=[...], name=transformer.layers.2.input_layernorm.bias), requires_grad=True)>
(attention): SelfAttention<
(rotary_emb): RotaryEmbedding<>
(query_key_value): Dense<input_channels=4096, output_channels=12288, has_bias=True>
(dense): Dense<input_channels=4096, output_channels=4096, has_bias=True>
>
(post_attention_layernorm): LayerNorm<normalized_shape=[4096], begin_norm_axis=-1, begin_params_axis=-1, weight=Parameter (Tensor(shape=[4096], dtype=Float16, value=[...], name=transformer.layers.2.post_attention_layernorm.weight), requires_grad=True), bias=Parameter (Tensor(shape=[4096], dtype=Float16, value=[...], name=transformer.layers.2.post_attention_layernorm.bias), requires_grad=True)>
(mlp): GLU<
(dense_h_to_4h): Dense<input_channels=4096, output_channels=16384, has_bias=True>
(dense_4h_to_h): Dense<input_channels=16384, output_channels=4096, has_bias=True>
>
>
(3): GLMBlock<
(input_layernorm): LayerNorm<normalized_shape=[4096], begin_norm_axis=-1, begin_params_axis=-1, weight=Parameter (Tensor(shape=[4096], dtype=Float16, value=[...], name=transformer.layers.3.input_layernorm.weight), requires_grad=True), bias=Parameter (Tensor(shape=[4096], dtype=Float16, value=[...], name=transformer.layers.3.input_layernorm.bias), requires_grad=True)>
(attention): SelfAttention<
(rotary_emb): RotaryEmbedding<>
(query_key_value): Dense<input_channels=4096, output_channels=12288, has_bias=True>
(dense): Dense<input_channels=4096, output_channels=4096, has_bias=True>
>
(post_attention_layernorm): LayerNorm<normalized_shape=[4096], begin_norm_axis=-1, begin_params_axis=-1, weight=Parameter (Tensor(shape=[4096], dtype=Float16, value=[...], name=transformer.layers.3.post_attention_layernorm.weight), requires_grad=True), bias=Parameter (Tensor(shape=[4096], dtype=Float16, value=[...], name=transformer.layers.3.post_attention_layernorm.bias), requires_grad=True)>
(mlp): GLU<
(dense_h_to_4h): Dense<input_channels=4096, output_channels=16384, has_bias=True>
(dense_4h_to_h): Dense<input_channels=16384, output_channels=4096, has_bias=True>
>
>
(4): GLMBlock<
(input_layernorm): LayerNorm<normalized_shape=[4096], begin_norm_axis=-1, begin_params_axis=-1, weight=Parameter (Tensor(shape=[4096], dtype=Float16, value=[...], name=transformer.layers.4.input_layernorm.weight), requires_grad=True), bias=Parameter (Tensor(shape=[4096], dtype=Float16, value=[...], name=transformer.layers.4.input_layernorm.bias), requires_grad=True)>
(attention): SelfAttention<
(rotary_emb): RotaryEmbedding<>
(query_key_value): Dense<input_channels=4096, output_channels=12288, has_bias=True>
(dense): Dense<input_channels=4096, output_channels=4096, has_bias=True>
>
(post_attention_layernorm): LayerNorm<normalized_shape=[4096], begin_norm_axis=-1, begin_params_axis=-1, weight=Parameter (Tensor(shape=[4096], dtype=Float16, value=[...], name=transformer.layers.4.post_attention_layernorm.weight), requires_grad=True), bias=Parameter (Tensor(shape=[4096], dtype=Float16, value=[...], name=transformer.layers.4.post_attention_layernorm.bias), requires_grad=True)>
(mlp): GLU<
(dense_h_to_4h): Dense<input_channels=4096, output_channels=16384, has_bias=True>
(dense_4h_to_h): Dense<input_channels=16384, output_channels=4096, has_bias=True>
>
>
(5): GLMBlock<
(input_layernorm): LayerNorm<normalized_shape=[4096], begin_norm_axis=-1, begin_params_axis=-1, weight=Parameter (Tensor(shape=[4096], dtype=Float16, value=[...], name=transformer.layers.5.input_layernorm.weight), requires_grad=True), bias=Parameter (Tensor(shape=[4096], dtype=Float16, value=[...], name=transformer.layers.5.input_layernorm.bias), requires_grad=True)>
(attention): SelfAttention<
(rotary_emb): RotaryEmbedding<>
(query_key_value): Dense<input_channels=4096, output_channels=12288, has_bias=True>
(dense): Dense<input_channels=4096, output_channels=4096, has_bias=True>
>
(post_attention_layernorm): LayerNorm<normalized_shape=[4096], begin_norm_axis=-1, begin_params_axis=-1, weight=Parameter (Tensor(shape=[4096], dtype=Float16, value=[...], name=transformer.layers.5.post_attention_layernorm.weight), requires_grad=True), bias=Parameter (Tensor(shape=[4096], dtype=Float16, value=[...], name=transformer.layers.5.post_attention_layernorm.bias), requires_grad=True)>
(mlp): GLU<
(dense_h_to_4h): Dense<input_channels=4096, output_channels=16384, has_bias=True>
(dense_4h_to_h): Dense<input_channels=16384, output_channels=4096, has_bias=True>
>
>
(6): GLMBlock<
(input_layernorm): LayerNorm<normalized_shape=[4096], begin_norm_axis=-1, begin_params_axis=-1, weight=Parameter (Tensor(shape=[4096], dtype=Float16, value=[...], name=transformer.layers.6.input_layernorm.weight), requires_grad=True), bias=Parameter (Tensor(shape=[4096], dtype=Float16, value=[...], name=transformer.layers.6.input_layernorm.bias), requires_grad=True)>
(attention): SelfAttention<
(rotary_emb): RotaryEmbedding<>
(query_key_value): Dense<input_channels=4096, output_channels=12288, has_bias=True>
(dense): Dense<input_channels=4096, output_channels=4096, has_bias=True>
>
(post_attention_layernorm): LayerNorm<normalized_shape=[4096], begin_norm_axis=-1, begin_params_axis=-1, weight=Parameter (Tensor(shape=[4096], dtype=Float16, value=[...], name=transformer.layers.6.post_attention_layernorm.weight), requires_grad=True), bias=Parameter (Tensor(shape=[4096], dtype=Float16, value=[...], name=transformer.layers.6.post_attention_layernorm.bias), requires_grad=True)>
(mlp): GLU<
(dense_h_to_4h): Dense<input_channels=4096, output_channels=16384, has_bias=True>
(dense_4h_to_h): Dense<input_channels=16384, output_channels=4096, has_bias=True>
>
>
(7): GLMBlock<
(input_layernorm): LayerNorm<normalized_shape=[4096], begin_norm_axis=-1, begin_params_axis=-1, weight=Parameter (Tensor(shape=[4096], dtype=Float16, value=[...], name=transformer.layers.7.input_layernorm.weight), requires_grad=True), bias=Parameter (Tensor(shape=[4096], dtype=Float16, value=[...], name=transformer.layers.7.input_layernorm.bias), requires_grad=True)>
(attention): SelfAttention<
(rotary_emb): RotaryEmbedding<>
(query_key_value): Dense<input_channels=4096, output_channels=12288, has_bias=True>
(dense): Dense<input_channels=4096, output_channels=4096, has_bias=True>
>
(post_attention_layernorm): LayerNorm<normalized_shape=[4096], begin_norm_axis=-1, begin_params_axis=-1, weight=Parameter (Tensor(shape=[4096], dtype=Float16, value=[...], name=transformer.layers.7.post_attention_layernorm.weight), requires_grad=True), bias=Parameter (Tensor(shape=[4096], dtype=Float16, value=[...], name=transformer.layers.7.post_attention_layernorm.bias), requires_grad=True)>
(mlp): GLU<
(dense_h_to_4h): Dense<input_channels=4096, output_channels=16384, has_bias=True>
(dense_4h_to_h): Dense<input_channels=16384, output_channels=4096, has_bias=True>
>
>
(8): GLMBlock<
(input_layernorm): LayerNorm<normalized_shape=[4096], begin_norm_axis=-1, begin_params_axis=-1, weight=Parameter (Tensor(shape=[4096], dtype=Float16, value=[...], name=transformer.layers.8.input_layernorm.weight), requires_grad=True), bias=Parameter (Tensor(shape=[4096], dtype=Float16, value=[...], name=transformer.layers.8.input_layernorm.bias), requires_grad=True)>
(attention): SelfAttention<
(rotary_emb): RotaryEmbedding<>
(query_key_value): Dense<input_channels=4096, output_channels=12288, has_bias=True>
(dense): Dense<input_channels=4096, output_channels=4096, has_bias=True>
>
(post_attention_layernorm): LayerNorm<normalized_shape=[4096], begin_norm_axis=-1, begin_params_axis=-1, weight=Parameter (Tensor(shape=[4096], dtype=Float16, value=[...], name=transformer.layers.8.post_attention_layernorm.weight), requires_grad=True), bias=Parameter (Tensor(shape=[4096], dtype=Float16, value=[...], name=transformer.layers.8.post_attention_layernorm.bias), requires_grad=True)>
(mlp): GLU<
(dense_h_to_4h): Dense<input_channels=4096, output_channels=16384, has_bias=True>
(dense_4h_to_h): Dense<input_channels=16384, output_channels=4096, has_bias=True>
>
>
(9): GLMBlock<
(input_layernorm): LayerNorm<normalized_shape=[4096], begin_norm_axis=-1, begin_params_axis=-1, weight=Parameter (Tensor(shape=[4096], dtype=Float16, value=[...], name=transformer.layers.9.input_layernorm.weight), requires_grad=True), bias=Parameter (Tensor(shape=[4096], dtype=Float16, value=[...], name=transformer.layers.9.input_layernorm.bias), requires_grad=True)>
(attention): SelfAttention<
(rotary_emb): RotaryEmbedding<>
(query_key_value): Dense<input_channels=4096, output_channels=12288, has_bias=True>
(dense): Dense<input_channels=4096, output_channels=4096, has_bias=True>
>
(post_attention_layernorm): LayerNorm<normalized_shape=[4096], begin_norm_axis=-1, begin_params_axis=-1, weight=Parameter (Tensor(shape=[4096], dtype=Float16, value=[...], name=transformer.layers.9.post_attention_layernorm.weight), requires_grad=True), bias=Parameter (Tensor(shape=[4096], dtype=Float16, value=[...], name=transformer.layers.9.post_attention_layernorm.bias), requires_grad=True)>
(mlp): GLU<
(dense_h_to_4h): Dense<input_channels=4096, output_channels=16384, has_bias=True>
(dense_4h_to_h): Dense<input_channels=16384, output_channels=4096, has_bias=True>
>
>
(10): GLMBlock<
(input_layernorm): LayerNorm<normalized_shape=[4096], begin_norm_axis=-1, begin_params_axis=-1, weight=Parameter (Tensor(shape=[4096], dtype=Float16, value=[...], name=transformer.layers.10.input_layernorm.weight), requires_grad=True), bias=Parameter (Tensor(shape=[4096], dtype=Float16, value=[...], name=transformer.layers.10.input_layernorm.bias), requires_grad=True)>
(attention): SelfAttention<
(rotary_emb): RotaryEmbedding<>
(query_key_value): Dense<input_channels=4096, output_channels=12288, has_bias=True>
(dense): Dense<input_channels=4096, output_channels=4096, has_bias=True>
>
(post_attention_layernorm): LayerNorm<normalized_shape=[4096], begin_norm_axis=-1, begin_params_axis=-1, weight=Parameter (Tensor(shape=[4096], dtype=Float16, value=[...], name=transformer.layers.10.post_attention_layernorm.weight), requires_grad=True), bias=Parameter (Tensor(shape=[4096], dtype=Float16, value=[...], name=transformer.layers.10.post_attention_layernorm.bias), requires_grad=True)>
(mlp): GLU<
(dense_h_to_4h): Dense<input_channels=4096, output_channels=16384, has_bias=True>
(dense_4h_to_h): Dense<input_channels=16384, output_channels=4096, has_bias=True>
>
>
(11): GLMBlock<
(input_layernorm): LayerNorm<normalized_shape=[4096], begin_norm_axis=-1, begin_params_axis=-1, weight=Parameter (Tensor(shape=[4096], dtype=Float16, value=[...], name=transformer.layers.11.input_layernorm.weight), requires_grad=True), bias=Parameter (Tensor(shape=[4096], dtype=Float16, value=[...], name=transformer.layers.11.input_layernorm.bias), requires_grad=True)>
(attention): SelfAttention<
(rotary_emb): RotaryEmbedding<>
(query_key_value): Dense<input_channels=4096, output_channels=12288, has_bias=True>
(dense): Dense<input_channels=4096, output_channels=4096, has_bias=True>
>
(post_attention_layernorm): LayerNorm<normalized_shape=[4096], begin_norm_axis=-1, begin_params_axis=-1, weight=Parameter (Tensor(shape=[4096], dtype=Float16, value=[...], name=transformer.layers.11.post_attention_layernorm.weight), requires_grad=True), bias=Parameter (Tensor(shape=[4096], dtype=Float16, value=[...], name=transformer.layers.11.post_attention_layernorm.bias), requires_grad=True)>
(mlp): GLU<
(dense_h_to_4h): Dense<input_channels=4096, output_channels=16384, has_bias=True>
(dense_4h_to_h): Dense<input_channels=16384, output_channels=4096, has_bias=True>
>
>
(12): GLMBlock<
(input_layernorm): LayerNorm<normalized_shape=[4096], begin_norm_axis=-1, begin_params_axis=-1, weight=Parameter (Tensor(shape=[4096], dtype=Float16, value=[...], name=transformer.layers.12.input_layernorm.weight), requires_grad=True), bias=Parameter (Tensor(shape=[4096], dtype=Float16, value=[...], name=transformer.layers.12.input_layernorm.bias), requires_grad=True)>
(attention): SelfAttention<
(rotary_emb): RotaryEmbedding<>
(query_key_value): Dense<input_channels=4096, output_channels=12288, has_bias=True>
(dense): Dense<input_channels=4096, output_channels=4096, has_bias=True>
>
(post_attention_layernorm): LayerNorm<normalized_shape=[4096], begin_norm_axis=-1, begin_params_axis=-1, weight=Parameter (Tensor(shape=[4096], dtype=Float16, value=[...], name=transformer.layers.12.post_attention_layernorm.weight), requires_grad=True), bias=Parameter (Tensor(shape=[4096], dtype=Float16, value=[...], name=transformer.layers.12.post_attention_layernorm.bias), requires_grad=True)>
(mlp): GLU<
(dense_h_to_4h): Dense<input_channels=4096, output_channels=16384, has_bias=True>
(dense_4h_to_h): Dense<input_channels=16384, output_channels=4096, has_bias=True>
>
>
(13): GLMBlock<
(input_layernorm): LayerNorm<normalized_shape=[4096], begin_norm_axis=-1, begin_params_axis=-1, weight=Parameter (Tensor(shape=[4096], dtype=Float16, value=[...], name=transformer.layers.13.input_layernorm.weight), requires_grad=True), bias=Parameter (Tensor(shape=[4096], dtype=Float16, value=[...], name=transformer.layers.13.input_layernorm.bias), requires_grad=True)>
(attention): SelfAttention<
(rotary_emb): RotaryEmbedding<>
(query_key_value): Dense<input_channels=4096, output_channels=12288, has_bias=True>
(dense): Dense<input_channels=4096, output_channels=4096, has_bias=True>
>
(post_attention_layernorm): LayerNorm<normalized_shape=[4096], begin_norm_axis=-1, begin_params_axis=-1, weight=Parameter (Tensor(shape=[4096], dtype=Float16, value=[...], name=transformer.layers.13.post_attention_layernorm.weight), requires_grad=True), bias=Parameter (Tensor(shape=[4096], dtype=Float16, value=[...], name=transformer.layers.13.post_attention_layernorm.bias), requires_grad=True)>
(mlp): GLU<
(dense_h_to_4h): Dense<input_channels=4096, output_channels=16384, has_bias=True>
(dense_4h_to_h): Dense<input_channels=16384, output_channels=4096, has_bias=True>
>
>
(14): GLMBlock<
(input_layernorm): LayerNorm<normalized_shape=[4096], begin_norm_axis=-1, begin_params_axis=-1, weight=Parameter (Tensor(shape=[4096], dtype=Float16, value=[...], name=transformer.layers.14.input_layernorm.weight), requires_grad=True), bias=Parameter (Tensor(shape=[4096], dtype=Float16, value=[...], name=transformer.layers.14.input_layernorm.bias), requires_grad=True)>
(attention): SelfAttention<
(rotary_emb): RotaryEmbedding<>
(query_key_value): Dense<input_channels=4096, output_channels=12288, has_bias=True>
(dense): Dense<input_channels=4096, output_channels=4096, has_bias=True>
>
(post_attention_layernorm): LayerNorm<normalized_shape=[4096], begin_norm_axis=-1, begin_params_axis=-1, weight=Parameter (Tensor(shape=[4096], dtype=Float16, value=[...], name=transformer.layers.14.post_attention_layernorm.weight), requires_grad=True), bias=Parameter (Tensor(shape=[4096], dtype=Float16, value=[...], name=transformer.layers.14.post_attention_layernorm.bias), requires_grad=True)>
(mlp): GLU<
(dense_h_to_4h): Dense<input_channels=4096, output_channels=16384, has_bias=True>
(dense_4h_to_h): Dense<input_channels=16384, output_channels=4096, has_bias=True>
>
>
(15): GLMBlock<
(input_layernorm): LayerNorm<normalized_shape=[4096], begin_norm_axis=-1, begin_params_axis=-1, weight=Parameter (Tensor(shape=[4096], dtype=Float16, value=[...], name=transformer.layers.15.input_layernorm.weight), requires_grad=True), bias=Parameter (Tensor(shape=[4096], dtype=Float16, value=[...], name=transformer.layers.15.input_layernorm.bias), requires_grad=True)>
(attention): SelfAttention<
(rotary_emb): RotaryEmbedding<>
(query_key_value): Dense<input_channels=4096, output_channels=12288, has_bias=True>
(dense): Dense<input_channels=4096, output_channels=4096, has_bias=True>
>
(post_attention_layernorm): LayerNorm<normalized_shape=[4096], begin_norm_axis=-1, begin_params_axis=-1, weight=Parameter (Tensor(shape=[4096], dtype=Float16, value=[...], name=transformer.layers.15.post_attention_layernorm.weight), requires_grad=True), bias=Parameter (Tensor(shape=[4096], dtype=Float16, value=[...], name=transformer.layers.15.post_attention_layernorm.bias), requires_grad=True)>
(mlp): GLU<
(dense_h_to_4h): Dense<input_channels=4096, output_channels=16384, has_bias=True>
(dense_4h_to_h): Dense<input_channels=16384, output_channels=4096, has_bias=True>
>
>
(16): GLMBlock<
(input_layernorm): LayerNorm<normalized_shape=[4096], begin_norm_axis=-1, begin_params_axis=-1, weight=Parameter (Tensor(shape=[4096], dtype=Float16, value=[...], name=transformer.layers.16.input_layernorm.weight), requires_grad=True), bias=Parameter (Tensor(shape=[4096], dtype=Float16, value=[...], name=transformer.layers.16.input_layernorm.bias), requires_grad=True)>
(attention): SelfAttention<
(rotary_emb): RotaryEmbedding<>
(query_key_value): Dense<input_channels=4096, output_channels=12288, has_bias=True>
(dense): Dense<input_channels=4096, output_channels=4096, has_bias=True>
>
(post_attention_layernorm): LayerNorm<normalized_shape=[4096], begin_norm_axis=-1, begin_params_axis=-1, weight=Parameter (Tensor(shape=[4096], dtype=Float16, value=[...], name=transformer.layers.16.post_attention_layernorm.weight), requires_grad=True), bias=Parameter (Tensor(shape=[4096], dtype=Float16, value=[...], name=transformer.layers.16.post_attention_layernorm.bias), requires_grad=True)>
(mlp): GLU<
(dense_h_to_4h): Dense<input_channels=4096, output_channels=16384, has_bias=True>
(dense_4h_to_h): Dense<input_channels=16384, output_channels=4096, has_bias=True>
>
>
(17): GLMBlock<
(input_layernorm): LayerNorm<normalized_shape=[4096], begin_norm_axis=-1, begin_params_axis=-1, weight=Parameter (Tensor(shape=[4096], dtype=Float16, value=[...], name=transformer.layers.17.input_layernorm.weight), requires_grad=True), bias=Parameter (Tensor(shape=[4096], dtype=Float16, value=[...], name=transformer.layers.17.input_layernorm.bias), requires_grad=True)>
(attention): SelfAttention<
(rotary_emb): RotaryEmbedding<>
(query_key_value): Dense<input_channels=4096, output_channels=12288, has_bias=True>
(dense): Dense<input_channels=4096, output_channels=4096, has_bias=True>
>
(post_attention_layernorm): LayerNorm<normalized_shape=[4096], begin_norm_axis=-1, begin_params_axis=-1, weight=Parameter (Tensor(shape=[4096], dtype=Float16, value=[...], name=transformer.layers.17.post_attention_layernorm.weight), requires_grad=True), bias=Parameter (Tensor(shape=[4096], dtype=Float16, value=[...], name=transformer.layers.17.post_attention_layernorm.bias), requires_grad=True)>
(mlp): GLU<
(dense_h_to_4h): Dense<input_channels=4096, output_channels=16384, has_bias=True>
(dense_4h_to_h): Dense<input_channels=16384, output_channels=4096, has_bias=True>
>
>
(18): GLMBlock<
(input_layernorm): LayerNorm<normalized_shape=[4096], begin_norm_axis=-1, begin_params_axis=-1, weight=Parameter (Tensor(shape=[4096], dtype=Float16, value=[...], name=transformer.layers.18.input_layernorm.weight), requires_grad=True), bias=Parameter (Tensor(shape=[4096], dtype=Float16, value=[...], name=transformer.layers.18.input_layernorm.bias), requires_grad=True)>
(attention): SelfAttention<
(rotary_emb): RotaryEmbedding<>
(query_key_value): Dense<input_channels=4096, output_channels=12288, has_bias=True>
(dense): Dense<input_channels=4096, output_channels=4096, has_bias=True>
>
(post_attention_layernorm): LayerNorm<normalized_shape=[4096], begin_norm_axis=-1, begin_params_axis=-1, weight=Parameter (Tensor(shape=[4096], dtype=Float16, value=[...], name=transformer.layers.18.post_attention_layernorm.weight), requires_grad=True), bias=Parameter (Tensor(shape=[4096], dtype=Float16, value=[...], name=transformer.layers.18.post_attention_layernorm.bias), requires_grad=True)>
(mlp): GLU<
(dense_h_to_4h): Dense<input_channels=4096, output_channels=16384, has_bias=True>
(dense_4h_to_h): Dense<input_channels=16384, output_channels=4096, has_bias=True>
>
>
(19): GLMBlock<
(input_layernorm): LayerNorm<normalized_shape=[4096], begin_norm_axis=-1, begin_params_axis=-1, weight=Parameter (Tensor(shape=[4096], dtype=Float16, value=[...], name=transformer.layers.19.input_layernorm.weight), requires_grad=True), bias=Parameter (Tensor(shape=[4096], dtype=Float16, value=[...], name=transformer.layers.19.input_layernorm.bias), requires_grad=True)>
(attention): SelfAttention<
(rotary_emb): RotaryEmbedding<>
(query_key_value): Dense<input_channels=4096, output_channels=12288, has_bias=True>
(dense): Dense<input_channels=4096, output_channels=4096, has_bias=True>
>
(post_attention_layernorm): LayerNorm<normalized_shape=[4096], begin_norm_axis=-1, begin_params_axis=-1, weight=Parameter (Tensor(shape=[4096], dtype=Float16, value=[...], name=transformer.layers.19.post_attention_layernorm.weight), requires_grad=True), bias=Parameter (Tensor(shape=[4096], dtype=Float16, value=[...], name=transformer.layers.19.post_attention_layernorm.bias), requires_grad=True)>
(mlp): GLU<
(dense_h_to_4h): Dense<input_channels=4096, output_channels=16384, has_bias=True>
(dense_4h_to_h): Dense<input_channels=16384, output_channels=4096, has_bias=True>
>
>
(20): GLMBlock<
(input_layernorm): LayerNorm<normalized_shape=[4096], begin_norm_axis=-1, begin_params_axis=-1, weight=Parameter (Tensor(shape=[4096], dtype=Float16, value=[...], name=transformer.layers.20.input_layernorm.weight), requires_grad=True), bias=Parameter (Tensor(shape=[4096], dtype=Float16, value=[...], name=transformer.layers.20.input_layernorm.bias), requires_grad=True)>
(attention): SelfAttention<
(rotary_emb): RotaryEmbedding<>
(query_key_value): Dense<input_channels=4096, output_channels=12288, has_bias=True>
(dense): Dense<input_channels=4096, output_channels=4096, has_bias=True>
>
(post_attention_layernorm): LayerNorm<normalized_shape=[4096], begin_norm_axis=-1, begin_params_axis=-1, weight=Parameter (Tensor(shape=[4096], dtype=Float16, value=[...], name=transformer.layers.20.post_attention_layernorm.weight), requires_grad=True), bias=Parameter (Tensor(shape=[4096], dtype=Float16, value=[...], name=transformer.layers.20.post_attention_layernorm.bias), requires_grad=True)>
(mlp): GLU<
(dense_h_to_4h): Dense<input_channels=4096, output_channels=16384, has_bias=True>
(dense_4h_to_h): Dense<input_channels=16384, output_channels=4096, has_bias=True>
>
>
(21): GLMBlock<
(input_layernorm): LayerNorm<normalized_shape=[4096], begin_norm_axis=-1, begin_params_axis=-1, weight=Parameter (Tensor(shape=[4096], dtype=Float16, value=[...], name=transformer.layers.21.input_layernorm.weight), requires_grad=True), bias=Parameter (Tensor(shape=[4096], dtype=Float16, value=[...], name=transformer.layers.21.input_layernorm.bias), requires_grad=True)>
(attention): SelfAttention<
(rotary_emb): RotaryEmbedding<>
(query_key_value): Dense<input_channels=4096, output_channels=12288, has_bias=True>
(dense): Dense<input_channels=4096, output_channels=4096, has_bias=True>
>
(post_attention_layernorm): LayerNorm<normalized_shape=[4096], begin_norm_axis=-1, begin_params_axis=-1, weight=Parameter (Tensor(shape=[4096], dtype=Float16, value=[...], name=transformer.layers.21.post_attention_layernorm.weight), requires_grad=True), bias=Parameter (Tensor(shape=[4096], dtype=Float16, value=[...], name=transformer.layers.21.post_attention_layernorm.bias), requires_grad=True)>
(mlp): GLU<
(dense_h_to_4h): Dense<input_channels=4096, output_channels=16384, has_bias=True>
(dense_4h_to_h): Dense<input_channels=16384, output_channels=4096, has_bias=True>
>
>
(22): GLMBlock<
(input_layernorm): LayerNorm<normalized_shape=[4096], begin_norm_axis=-1, begin_params_axis=-1, weight=Parameter (Tensor(shape=[4096], dtype=Float16, value=[...], name=transformer.layers.22.input_layernorm.weight), requires_grad=True), bias=Parameter (Tensor(shape=[4096], dtype=Float16, value=[...], name=transformer.layers.22.input_layernorm.bias), requires_grad=True)>
(attention): SelfAttention<
(rotary_emb): RotaryEmbedding<>
(query_key_value): Dense<input_channels=4096, output_channels=12288, has_bias=True>
(dense): Dense<input_channels=4096, output_channels=4096, has_bias=True>
>
(post_attention_layernorm): LayerNorm<normalized_shape=[4096], begin_norm_axis=-1, begin_params_axis=-1, weight=Parameter (Tensor(shape=[4096], dtype=Float16, value=[...], name=transformer.layers.22.post_attention_layernorm.weight), requires_grad=True), bias=Parameter (Tensor(shape=[4096], dtype=Float16, value=[...], name=transformer.layers.22.post_attention_layernorm.bias), requires_grad=True)>
(mlp): GLU<
(dense_h_to_4h): Dense<input_channels=4096, output_channels=16384, has_bias=True>
(dense_4h_to_h): Dense<input_channels=16384, output_channels=4096, has_bias=True>
>
>
(23): GLMBlock<
(input_layernorm): LayerNorm<normalized_shape=[4096], begin_norm_axis=-1, begin_params_axis=-1, weight=Parameter (Tensor(shape=[4096], dtype=Float16, value=[...], name=transformer.layers.23.input_layernorm.weight), requires_grad=True), bias=Parameter (Tensor(shape=[4096], dtype=Float16, value=[...], name=transformer.layers.23.input_layernorm.bias), requires_grad=True)>
(attention): SelfAttention<
(rotary_emb): RotaryEmbedding<>
(query_key_value): Dense<input_channels=4096, output_channels=12288, has_bias=True>
(dense): Dense<input_channels=4096, output_channels=4096, has_bias=True>
>
(post_attention_layernorm): LayerNorm<normalized_shape=[4096], begin_norm_axis=-1, begin_params_axis=-1, weight=Parameter (Tensor(shape=[4096], dtype=Float16, value=[...], name=transformer.layers.23.post_attention_layernorm.weight), requires_grad=True), bias=Parameter (Tensor(shape=[4096], dtype=Float16, value=[...], name=transformer.layers.23.post_attention_layernorm.bias), requires_grad=True)>
(mlp): GLU<
(dense_h_to_4h): Dense<input_channels=4096, output_channels=16384, has_bias=True>
(dense_4h_to_h): Dense<input_channels=16384, output_channels=4096, has_bias=True>
>
>
(24): GLMBlock<
(input_layernorm): LayerNorm<normalized_shape=[4096], begin_norm_axis=-1, begin_params_axis=-1, weight=Parameter (Tensor(shape=[4096], dtype=Float16, value=[...], name=transformer.layers.24.input_layernorm.weight), requires_grad=True), bias=Parameter (Tensor(shape=[4096], dtype=Float16, value=[...], name=transformer.layers.24.input_layernorm.bias), requires_grad=True)>
(attention): SelfAttention<
(rotary_emb): RotaryEmbedding<>
(query_key_value): Dense<input_channels=4096, output_channels=12288, has_bias=True>
(dense): Dense<input_channels=4096, output_channels=4096, has_bias=True>
>
(post_attention_layernorm): LayerNorm<normalized_shape=[4096], begin_norm_axis=-1, begin_params_axis=-1, weight=Parameter (Tensor(shape=[4096], dtype=Float16, value=[...], name=transformer.layers.24.post_attention_layernorm.weight), requires_grad=True), bias=Parameter (Tensor(shape=[4096], dtype=Float16, value=[...], name=transformer.layers.24.post_attention_layernorm.bias), requires_grad=True)>
(mlp): GLU<
(dense_h_to_4h): Dense<input_channels=4096, output_channels=16384, has_bias=True>
(dense_4h_to_h): Dense<input_channels=16384, output_channels=4096, has_bias=True>
>
>
(25): GLMBlock<
(input_layernorm): LayerNorm<normalized_shape=[4096], begin_norm_axis=-1, begin_params_axis=-1, weight=Parameter (Tensor(shape=[4096], dtype=Float16, value=[...], name=transformer.layers.25.input_layernorm.weight), requires_grad=True), bias=Parameter (Tensor(shape=[4096], dtype=Float16, value=[...], name=transformer.layers.25.input_layernorm.bias), requires_grad=True)>
(attention): SelfAttention<
(rotary_emb): RotaryEmbedding<>
(query_key_value): Dense<input_channels=4096, output_channels=12288, has_bias=True>
(dense): Dense<input_channels=4096, output_channels=4096, has_bias=True>
>
(post_attention_layernorm): LayerNorm<normalized_shape=[4096], begin_norm_axis=-1, begin_params_axis=-1, weight=Parameter (Tensor(shape=[4096], dtype=Float16, value=[...], name=transformer.layers.25.post_attention_layernorm.weight), requires_grad=True), bias=Parameter (Tensor(shape=[4096], dtype=Float16, value=[...], name=transformer.layers.25.post_attention_layernorm.bias), requires_grad=True)>
(mlp): GLU<
(dense_h_to_4h): Dense<input_channels=4096, output_channels=16384, has_bias=True>
(dense_4h_to_h): Dense<input_channels=16384, output_channels=4096, has_bias=True>
>
>
(26): GLMBlock<
(input_layernorm): LayerNorm<normalized_shape=[4096], begin_norm_axis=-1, begin_params_axis=-1, weight=Parameter (Tensor(shape=[4096], dtype=Float16, value=[...], name=transformer.layers.26.input_layernorm.weight), requires_grad=True), bias=Parameter (Tensor(shape=[4096], dtype=Float16, value=[...], name=transformer.layers.26.input_layernorm.bias), requires_grad=True)>
(attention): SelfAttention<
(rotary_emb): RotaryEmbedding<>
(query_key_value): Dense<input_channels=4096, output_channels=12288, has_bias=True>
(dense): Dense<input_channels=4096, output_channels=4096, has_bias=True>
>
(post_attention_layernorm): LayerNorm<normalized_shape=[4096], begin_norm_axis=-1, begin_params_axis=-1, weight=Parameter (Tensor(shape=[4096], dtype=Float16, value=[...], name=transformer.layers.26.post_attention_layernorm.weight), requires_grad=True), bias=Parameter (Tensor(shape=[4096], dtype=Float16, value=[...], name=transformer.layers.26.post_attention_layernorm.bias), requires_grad=True)>
(mlp): GLU<
(dense_h_to_4h): Dense<input_channels=4096, output_channels=16384, has_bias=True>
(dense_4h_to_h): Dense<input_channels=16384, output_channels=4096, has_bias=True>
>
>
(27): GLMBlock<
(input_layernorm): LayerNorm<normalized_shape=[4096], begin_norm_axis=-1, begin_params_axis=-1, weight=Parameter (Tensor(shape=[4096], dtype=Float16, value=[...], name=transformer.layers.27.input_layernorm.weight), requires_grad=True), bias=Parameter (Tensor(shape=[4096], dtype=Float16, value=[...], name=transformer.layers.27.input_layernorm.bias), requires_grad=True)>
(attention): SelfAttention<
(rotary_emb): RotaryEmbedding<>
(query_key_value): Dense<input_channels=4096, output_channels=12288, has_bias=True>
(dense): Dense<input_channels=4096, output_channels=4096, has_bias=True>
>
(post_attention_layernorm): LayerNorm<normalized_shape=[4096], begin_norm_axis=-1, begin_params_axis=-1, weight=Parameter (Tensor(shape=[4096], dtype=Float16, value=[...], name=transformer.layers.27.post_attention_layernorm.weight), requires_grad=True), bias=Parameter (Tensor(shape=[4096], dtype=Float16, value=[...], name=transformer.layers.27.post_attention_layernorm.bias), requires_grad=True)>
(mlp): GLU<
(dense_h_to_4h): Dense<input_channels=4096, output_channels=16384, has_bias=True>
(dense_4h_to_h): Dense<input_channels=16384, output_channels=4096, has_bias=True>
>
>
>
(final_layernorm): LayerNorm<normalized_shape=[4096], begin_norm_axis=-1, begin_params_axis=-1, weight=Parameter (Tensor(shape=[4096], dtype=Float16, value=[...], name=transformer.final_layernorm.weight), requires_grad=True), bias=Parameter (Tensor(shape=[4096], dtype=Float16, value=[...], name=transformer.final_layernorm.bias), requires_grad=True)>
>
(lm_head): Dense<input_channels=4096, output_channels=130528>
>
ChatGLM2-6B 模型结构解析如下
如下图,chatglm2-6b model 的打印结果。相比于1版本,模型结构没有变化,只是vocab_size词表扩充成了15w+。
python
$ print(model)
ChatGLMForConditionalGeneration<
(transformer): ChatGLMModel<
(word_embeddings): Embedding<vocab_size=150528, embedding_size=4096, use_one_hot=False, weight=Parameter (Tensor(shape=[150528, 4096], dtype=Float16, value=[...], name=transformer.word_embeddings.weight), requires_grad=True), dtype=Float16, padding_idx=None>
(layers): CellList<
(0): GLMBlock<
(input_layernorm): LayerNorm<normalized_shape=[4096], begin_norm_axis=-1, begin_params_axis=-1, weight=Parameter (Tensor(shape=[4096], dtype=Float16, value=[...], name=transformer.layers.0.input_layernorm.weight), requires_grad=True), bias=Parameter (Tensor(shape=[4096], dtype=Float16, value=[...], name=transformer.layers.0.input_layernorm.bias), requires_grad=True)>
(attention): SelfAttention<
(rotary_emb): RotaryEmbedding<>
(query_key_value): Dense<input_channels=4096, output_channels=12288, has_bias=True>
(dense): Dense<input_channels=4096, output_channels=4096, has_bias=True>
>
(post_attention_layernorm): LayerNorm<normalized_shape=[4096], begin_norm_axis=-1, begin_params_axis=-1, weight=Parameter (Tensor(shape=[4096], dtype=Float16, value=[...], name=transformer.layers.0.post_attention_layernorm.weight), requires_grad=True), bias=Parameter (Tensor(shape=[4096], dtype=Float16, value=[...], name=transformer.layers.0.post_attention_layernorm.bias), requires_grad=True)>
(mlp): GLU<
(dense_h_to_4h): Dense<input_channels=4096, output_channels=16384, has_bias=True>
(dense_4h_to_h): Dense<input_channels=16384, output_channels=4096, has_bias=True>
>
>
(1): GLMBlock<
(input_layernorm): LayerNorm<normalized_shape=[4096], begin_norm_axis=-1, begin_params_axis=-1, weight=Parameter (Tensor(shape=[4096], dtype=Float16, value=[...], name=transformer.layers.1.input_layernorm.weight), requires_grad=True), bias=Parameter (Tensor(shape=[4096], dtype=Float16, value=[...], name=transformer.layers.1.input_layernorm.bias), requires_grad=True)>
(attention): SelfAttention<
(rotary_emb): RotaryEmbedding<>
(query_key_value): Dense<input_channels=4096, output_channels=12288, has_bias=True>
(dense): Dense<input_channels=4096, output_channels=4096, has_bias=True>
>
(post_attention_layernorm): LayerNorm<normalized_shape=[4096], begin_norm_axis=-1, begin_params_axis=-1, weight=Parameter (Tensor(shape=[4096], dtype=Float16, value=[...], name=transformer.layers.1.post_attention_layernorm.weight), requires_grad=True), bias=Parameter (Tensor(shape=[4096], dtype=Float16, value=[...], name=transformer.layers.1.post_attention_layernorm.bias), requires_grad=True)>
(mlp): GLU<
(dense_h_to_4h): Dense<input_channels=4096, output_channels=16384, has_bias=True>
(dense_4h_to_h): Dense<input_channels=16384, output_channels=4096, has_bias=True>
>
>
(2): GLMBlock<
(input_layernorm): LayerNorm<normalized_shape=[4096], begin_norm_axis=-1, begin_params_axis=-1, weight=Parameter (Tensor(shape=[4096], dtype=Float16, value=[...], name=transformer.layers.2.input_layernorm.weight), requires_grad=True), bias=Parameter (Tensor(shape=[4096], dtype=Float16, value=[...], name=transformer.layers.2.input_layernorm.bias), requires_grad=True)>
(attention): SelfAttention<
(rotary_emb): RotaryEmbedding<>
(query_key_value): Dense<input_channels=4096, output_channels=12288, has_bias=True>
(dense): Dense<input_channels=4096, output_channels=4096, has_bias=True>
>
(post_attention_layernorm): LayerNorm<normalized_shape=[4096], begin_norm_axis=-1, begin_params_axis=-1, weight=Parameter (Tensor(shape=[4096], dtype=Float16, value=[...], name=transformer.layers.2.post_attention_layernorm.weight), requires_grad=True), bias=Parameter (Tensor(shape=[4096], dtype=Float16, value=[...], name=transformer.layers.2.post_attention_layernorm.bias), requires_grad=True)>
(mlp): GLU<
(dense_h_to_4h): Dense<input_channels=4096, output_channels=16384, has_bias=True>
(dense_4h_to_h): Dense<input_channels=16384, output_channels=4096, has_bias=True>
>
>
(3): GLMBlock<
(input_layernorm): LayerNorm<normalized_shape=[4096], begin_norm_axis=-1, begin_params_axis=-1, weight=Parameter (Tensor(shape=[4096], dtype=Float16, value=[...], name=transformer.layers.3.input_layernorm.weight), requires_grad=True), bias=Parameter (Tensor(shape=[4096], dtype=Float16, value=[...], name=transformer.layers.3.input_layernorm.bias), requires_grad=True)>
(attention): SelfAttention<
(rotary_emb): RotaryEmbedding<>
(query_key_value): Dense<input_channels=4096, output_channels=12288, has_bias=True>
(dense): Dense<input_channels=4096, output_channels=4096, has_bias=True>
>
(post_attention_layernorm): LayerNorm<normalized_shape=[4096], begin_norm_axis=-1, begin_params_axis=-1, weight=Parameter (Tensor(shape=[4096], dtype=Float16, value=[...], name=transformer.layers.3.post_attention_layernorm.weight), requires_grad=True), bias=Parameter (Tensor(shape=[4096], dtype=Float16, value=[...], name=transformer.layers.3.post_attention_layernorm.bias), requires_grad=True)>
(mlp): GLU<
(dense_h_to_4h): Dense<input_channels=4096, output_channels=16384, has_bias=True>
(dense_4h_to_h): Dense<input_channels=16384, output_channels=4096, has_bias=True>
>
>
(4): GLMBlock<
(input_layernorm): LayerNorm<normalized_shape=[4096], begin_norm_axis=-1, begin_params_axis=-1, weight=Parameter (Tensor(shape=[4096], dtype=Float16, value=[...], name=transformer.layers.4.input_layernorm.weight), requires_grad=True), bias=Parameter (Tensor(shape=[4096], dtype=Float16, value=[...], name=transformer.layers.4.input_layernorm.bias), requires_grad=True)>
(attention): SelfAttention<
(rotary_emb): RotaryEmbedding<>
(query_key_value): Dense<input_channels=4096, output_channels=12288, has_bias=True>
(dense): Dense<input_channels=4096, output_channels=4096, has_bias=True>
>
(post_attention_layernorm): LayerNorm<normalized_shape=[4096], begin_norm_axis=-1, begin_params_axis=-1, weight=Parameter (Tensor(shape=[4096], dtype=Float16, value=[...], name=transformer.layers.4.post_attention_layernorm.weight), requires_grad=True), bias=Parameter (Tensor(shape=[4096], dtype=Float16, value=[...], name=transformer.layers.4.post_attention_layernorm.bias), requires_grad=True)>
(mlp): GLU<
(dense_h_to_4h): Dense<input_channels=4096, output_channels=16384, has_bias=True>
(dense_4h_to_h): Dense<input_channels=16384, output_channels=4096, has_bias=True>
>
>
(5): GLMBlock<
(input_layernorm): LayerNorm<normalized_shape=[4096], begin_norm_axis=-1, begin_params_axis=-1, weight=Parameter (Tensor(shape=[4096], dtype=Float16, value=[...], name=transformer.layers.5.input_layernorm.weight), requires_grad=True), bias=Parameter (Tensor(shape=[4096], dtype=Float16, value=[...], name=transformer.layers.5.input_layernorm.bias), requires_grad=True)>
(attention): SelfAttention<
(rotary_emb): RotaryEmbedding<>
(query_key_value): Dense<input_channels=4096, output_channels=12288, has_bias=True>
(dense): Dense<input_channels=4096, output_channels=4096, has_bias=True>
>
(post_attention_layernorm): LayerNorm<normalized_shape=[4096], begin_norm_axis=-1, begin_params_axis=-1, weight=Parameter (Tensor(shape=[4096], dtype=Float16, value=[...], name=transformer.layers.5.post_attention_layernorm.weight), requires_grad=True), bias=Parameter (Tensor(shape=[4096], dtype=Float16, value=[...], name=transformer.layers.5.post_attention_layernorm.bias), requires_grad=True)>
(mlp): GLU<
(dense_h_to_4h): Dense<input_channels=4096, output_channels=16384, has_bias=True>
(dense_4h_to_h): Dense<input_channels=16384, output_channels=4096, has_bias=True>
>
>
(6): GLMBlock<
(input_layernorm): LayerNorm<normalized_shape=[4096], begin_norm_axis=-1, begin_params_axis=-1, weight=Parameter (Tensor(shape=[4096], dtype=Float16, value=[...], name=transformer.layers.6.input_layernorm.weight), requires_grad=True), bias=Parameter (Tensor(shape=[4096], dtype=Float16, value=[...], name=transformer.layers.6.input_layernorm.bias), requires_grad=True)>
(attention): SelfAttention<
(rotary_emb): RotaryEmbedding<>
(query_key_value): Dense<input_channels=4096, output_channels=12288, has_bias=True>
(dense): Dense<input_channels=4096, output_channels=4096, has_bias=True>
>
(post_attention_layernorm): LayerNorm<normalized_shape=[4096], begin_norm_axis=-1, begin_params_axis=-1, weight=Parameter (Tensor(shape=[4096], dtype=Float16, value=[...], name=transformer.layers.6.post_attention_layernorm.weight), requires_grad=True), bias=Parameter (Tensor(shape=[4096], dtype=Float16, value=[...], name=transformer.layers.6.post_attention_layernorm.bias), requires_grad=True)>
(mlp): GLU<
(dense_h_to_4h): Dense<input_channels=4096, output_channels=16384, has_bias=True>
(dense_4h_to_h): Dense<input_channels=16384, output_channels=4096, has_bias=True>
>
>
(7): GLMBlock<
(input_layernorm): LayerNorm<normalized_shape=[4096], begin_norm_axis=-1, begin_params_axis=-1, weight=Parameter (Tensor(shape=[4096], dtype=Float16, value=[...], name=transformer.layers.7.input_layernorm.weight), requires_grad=True), bias=Parameter (Tensor(shape=[4096], dtype=Float16, value=[...], name=transformer.layers.7.input_layernorm.bias), requires_grad=True)>
(attention): SelfAttention<
(rotary_emb): RotaryEmbedding<>
(query_key_value): Dense<input_channels=4096, output_channels=12288, has_bias=True>
(dense): Dense<input_channels=4096, output_channels=4096, has_bias=True>
>
(post_attention_layernorm): LayerNorm<normalized_shape=[4096], begin_norm_axis=-1, begin_params_axis=-1, weight=Parameter (Tensor(shape=[4096], dtype=Float16, value=[...], name=transformer.layers.7.post_attention_layernorm.weight), requires_grad=True), bias=Parameter (Tensor(shape=[4096], dtype=Float16, value=[...], name=transformer.layers.7.post_attention_layernorm.bias), requires_grad=True)>
(mlp): GLU<
(dense_h_to_4h): Dense<input_channels=4096, output_channels=16384, has_bias=True>
(dense_4h_to_h): Dense<input_channels=16384, output_channels=4096, has_bias=True>
>
>
(8): GLMBlock<
(input_layernorm): LayerNorm<normalized_shape=[4096], begin_norm_axis=-1, begin_params_axis=-1, weight=Parameter (Tensor(shape=[4096], dtype=Float16, value=[...], name=transformer.layers.8.input_layernorm.weight), requires_grad=True), bias=Parameter (Tensor(shape=[4096], dtype=Float16, value=[...], name=transformer.layers.8.input_layernorm.bias), requires_grad=True)>
(attention): SelfAttention<
(rotary_emb): RotaryEmbedding<>
(query_key_value): Dense<input_channels=4096, output_channels=12288, has_bias=True>
(dense): Dense<input_channels=4096, output_channels=4096, has_bias=True>
>
(post_attention_layernorm): LayerNorm<normalized_shape=[4096], begin_norm_axis=-1, begin_params_axis=-1, weight=Parameter (Tensor(shape=[4096], dtype=Float16, value=[...], name=transformer.layers.8.post_attention_layernorm.weight), requires_grad=True), bias=Parameter (Tensor(shape=[4096], dtype=Float16, value=[...], name=transformer.layers.8.post_attention_layernorm.bias), requires_grad=True)>
(mlp): GLU<
(dense_h_to_4h): Dense<input_channels=4096, output_channels=16384, has_bias=True>
(dense_4h_to_h): Dense<input_channels=16384, output_channels=4096, has_bias=True>
>
>
(9): GLMBlock<
(input_layernorm): LayerNorm<normalized_shape=[4096], begin_norm_axis=-1, begin_params_axis=-1, weight=Parameter (Tensor(shape=[4096], dtype=Float16, value=[...], name=transformer.layers.9.input_layernorm.weight), requires_grad=True), bias=Parameter (Tensor(shape=[4096], dtype=Float16, value=[...], name=transformer.layers.9.input_layernorm.bias), requires_grad=True)>
(attention): SelfAttention<
(rotary_emb): RotaryEmbedding<>
(query_key_value): Dense<input_channels=4096, output_channels=12288, has_bias=True>
(dense): Dense<input_channels=4096, output_channels=4096, has_bias=True>
>
(post_attention_layernorm): LayerNorm<normalized_shape=[4096], begin_norm_axis=-1, begin_params_axis=-1, weight=Parameter (Tensor(shape=[4096], dtype=Float16, value=[...], name=transformer.layers.9.post_attention_layernorm.weight), requires_grad=True), bias=Parameter (Tensor(shape=[4096], dtype=Float16, value=[...], name=transformer.layers.9.post_attention_layernorm.bias), requires_grad=True)>
(mlp): GLU<
(dense_h_to_4h): Dense<input_channels=4096, output_channels=16384, has_bias=True>
(dense_4h_to_h): Dense<input_channels=16384, output_channels=4096, has_bias=True>
>
>
(10): GLMBlock<
(input_layernorm): LayerNorm<normalized_shape=[4096], begin_norm_axis=-1, begin_params_axis=-1, weight=Parameter (Tensor(shape=[4096], dtype=Float16, value=[...], name=transformer.layers.10.input_layernorm.weight), requires_grad=True), bias=Parameter (Tensor(shape=[4096], dtype=Float16, value=[...], name=transformer.layers.10.input_layernorm.bias), requires_grad=True)>
(attention): SelfAttention<
(rotary_emb): RotaryEmbedding<>
(query_key_value): Dense<input_channels=4096, output_channels=12288, has_bias=True>
(dense): Dense<input_channels=4096, output_channels=4096, has_bias=True>
>
(post_attention_layernorm): LayerNorm<normalized_shape=[4096], begin_norm_axis=-1, begin_params_axis=-1, weight=Parameter (Tensor(shape=[4096], dtype=Float16, value=[...], name=transformer.layers.10.post_attention_layernorm.weight), requires_grad=True), bias=Parameter (Tensor(shape=[4096], dtype=Float16, value=[...], name=transformer.layers.10.post_attention_layernorm.bias), requires_grad=True)>
(mlp): GLU<
(dense_h_to_4h): Dense<input_channels=4096, output_channels=16384, has_bias=True>
(dense_4h_to_h): Dense<input_channels=16384, output_channels=4096, has_bias=True>
>
>
(11): GLMBlock<
(input_layernorm): LayerNorm<normalized_shape=[4096], begin_norm_axis=-1, begin_params_axis=-1, weight=Parameter (Tensor(shape=[4096], dtype=Float16, value=[...], name=transformer.layers.11.input_layernorm.weight), requires_grad=True), bias=Parameter (Tensor(shape=[4096], dtype=Float16, value=[...], name=transformer.layers.11.input_layernorm.bias), requires_grad=True)>
(attention): SelfAttention<
(rotary_emb): RotaryEmbedding<>
(query_key_value): Dense<input_channels=4096, output_channels=12288, has_bias=True>
(dense): Dense<input_channels=4096, output_channels=4096, has_bias=True>
>
(post_attention_layernorm): LayerNorm<normalized_shape=[4096], begin_norm_axis=-1, begin_params_axis=-1, weight=Parameter (Tensor(shape=[4096], dtype=Float16, value=[...], name=transformer.layers.11.post_attention_layernorm.weight), requires_grad=True), bias=Parameter (Tensor(shape=[4096], dtype=Float16, value=[...], name=transformer.layers.11.post_attention_layernorm.bias), requires_grad=True)>
(mlp): GLU<
(dense_h_to_4h): Dense<input_channels=4096, output_channels=16384, has_bias=True>
(dense_4h_to_h): Dense<input_channels=16384, output_channels=4096, has_bias=True>
>
>
(12): GLMBlock<
(input_layernorm): LayerNorm<normalized_shape=[4096], begin_norm_axis=-1, begin_params_axis=-1, weight=Parameter (Tensor(shape=[4096], dtype=Float16, value=[...], name=transformer.layers.12.input_layernorm.weight), requires_grad=True), bias=Parameter (Tensor(shape=[4096], dtype=Float16, value=[...], name=transformer.layers.12.input_layernorm.bias), requires_grad=True)>
(attention): SelfAttention<
(rotary_emb): RotaryEmbedding<>
(query_key_value): Dense<input_channels=4096, output_channels=12288, has_bias=True>
(dense): Dense<input_channels=4096, output_channels=4096, has_bias=True>
>
(post_attention_layernorm): LayerNorm<normalized_shape=[4096], begin_norm_axis=-1, begin_params_axis=-1, weight=Parameter (Tensor(shape=[4096], dtype=Float16, value=[...], name=transformer.layers.12.post_attention_layernorm.weight), requires_grad=True), bias=Parameter (Tensor(shape=[4096], dtype=Float16, value=[...], name=transformer.layers.12.post_attention_layernorm.bias), requires_grad=True)>
(mlp): GLU<
(dense_h_to_4h): Dense<input_channels=4096, output_channels=16384, has_bias=True>
(dense_4h_to_h): Dense<input_channels=16384, output_channels=4096, has_bias=True>
>
>
(13): GLMBlock<
(input_layernorm): LayerNorm<normalized_shape=[4096], begin_norm_axis=-1, begin_params_axis=-1, weight=Parameter (Tensor(shape=[4096], dtype=Float16, value=[...], name=transformer.layers.13.input_layernorm.weight), requires_grad=True), bias=Parameter (Tensor(shape=[4096], dtype=Float16, value=[...], name=transformer.layers.13.input_layernorm.bias), requires_grad=True)>
(attention): SelfAttention<
(rotary_emb): RotaryEmbedding<>
(query_key_value): Dense<input_channels=4096, output_channels=12288, has_bias=True>
(dense): Dense<input_channels=4096, output_channels=4096, has_bias=True>
>
(post_attention_layernorm): LayerNorm<normalized_shape=[4096], begin_norm_axis=-1, begin_params_axis=-1, weight=Parameter (Tensor(shape=[4096], dtype=Float16, value=[...], name=transformer.layers.13.post_attention_layernorm.weight), requires_grad=True), bias=Parameter (Tensor(shape=[4096], dtype=Float16, value=[...], name=transformer.layers.13.post_attention_layernorm.bias), requires_grad=True)>
(mlp): GLU<
(dense_h_to_4h): Dense<input_channels=4096, output_channels=16384, has_bias=True>
(dense_4h_to_h): Dense<input_channels=16384, output_channels=4096, has_bias=True>
>
>
(14): GLMBlock<
(input_layernorm): LayerNorm<normalized_shape=[4096], begin_norm_axis=-1, begin_params_axis=-1, weight=Parameter (Tensor(shape=[4096], dtype=Float16, value=[...], name=transformer.layers.14.input_layernorm.weight), requires_grad=True), bias=Parameter (Tensor(shape=[4096], dtype=Float16, value=[...], name=transformer.layers.14.input_layernorm.bias), requires_grad=True)>
(attention): SelfAttention<
(rotary_emb): RotaryEmbedding<>
(query_key_value): Dense<input_channels=4096, output_channels=12288, has_bias=True>
(dense): Dense<input_channels=4096, output_channels=4096, has_bias=True>
>
(post_attention_layernorm): LayerNorm<normalized_shape=[4096], begin_norm_axis=-1, begin_params_axis=-1, weight=Parameter (Tensor(shape=[4096], dtype=Float16, value=[...], name=transformer.layers.14.post_attention_layernorm.weight), requires_grad=True), bias=Parameter (Tensor(shape=[4096], dtype=Float16, value=[...], name=transformer.layers.14.post_attention_layernorm.bias), requires_grad=True)>
(mlp): GLU<
(dense_h_to_4h): Dense<input_channels=4096, output_channels=16384, has_bias=True>
(dense_4h_to_h): Dense<input_channels=16384, output_channels=4096, has_bias=True>
>
>
(15): GLMBlock<
(input_layernorm): LayerNorm<normalized_shape=[4096], begin_norm_axis=-1, begin_params_axis=-1, weight=Parameter (Tensor(shape=[4096], dtype=Float16, value=[...], name=transformer.layers.15.input_layernorm.weight), requires_grad=True), bias=Parameter (Tensor(shape=[4096], dtype=Float16, value=[...], name=transformer.layers.15.input_layernorm.bias), requires_grad=True)>
(attention): SelfAttention<
(rotary_emb): RotaryEmbedding<>
(query_key_value): Dense<input_channels=4096, output_channels=12288, has_bias=True>
(dense): Dense<input_channels=4096, output_channels=4096, has_bias=True>
>
(post_attention_layernorm): LayerNorm<normalized_shape=[4096], begin_norm_axis=-1, begin_params_axis=-1, weight=Parameter (Tensor(shape=[4096], dtype=Float16, value=[...], name=transformer.layers.15.post_attention_layernorm.weight), requires_grad=True), bias=Parameter (Tensor(shape=[4096], dtype=Float16, value=[...], name=transformer.layers.15.post_attention_layernorm.bias), requires_grad=True)>
(mlp): GLU<
(dense_h_to_4h): Dense<input_channels=4096, output_channels=16384, has_bias=True>
(dense_4h_to_h): Dense<input_channels=16384, output_channels=4096, has_bias=True>
>
>
(16): GLMBlock<
(input_layernorm): LayerNorm<normalized_shape=[4096], begin_norm_axis=-1, begin_params_axis=-1, weight=Parameter (Tensor(shape=[4096], dtype=Float16, value=[...], name=transformer.layers.16.input_layernorm.weight), requires_grad=True), bias=Parameter (Tensor(shape=[4096], dtype=Float16, value=[...], name=transformer.layers.16.input_layernorm.bias), requires_grad=True)>
(attention): SelfAttention<
(rotary_emb): RotaryEmbedding<>
(query_key_value): Dense<input_channels=4096, output_channels=12288, has_bias=True>
(dense): Dense<input_channels=4096, output_channels=4096, has_bias=True>
>
(post_attention_layernorm): LayerNorm<normalized_shape=[4096], begin_norm_axis=-1, begin_params_axis=-1, weight=Parameter (Tensor(shape=[4096], dtype=Float16, value=[...], name=transformer.layers.16.post_attention_layernorm.weight), requires_grad=True), bias=Parameter (Tensor(shape=[4096], dtype=Float16, value=[...], name=transformer.layers.16.post_attention_layernorm.bias), requires_grad=True)>
(mlp): GLU<
(dense_h_to_4h): Dense<input_channels=4096, output_channels=16384, has_bias=True>
(dense_4h_to_h): Dense<input_channels=16384, output_channels=4096, has_bias=True>
>
>
(17): GLMBlock<
(input_layernorm): LayerNorm<normalized_shape=[4096], begin_norm_axis=-1, begin_params_axis=-1, weight=Parameter (Tensor(shape=[4096], dtype=Float16, value=[...], name=transformer.layers.17.input_layernorm.weight), requires_grad=True), bias=Parameter (Tensor(shape=[4096], dtype=Float16, value=[...], name=transformer.layers.17.input_layernorm.bias), requires_grad=True)>
(attention): SelfAttention<
(rotary_emb): RotaryEmbedding<>
(query_key_value): Dense<input_channels=4096, output_channels=12288, has_bias=True>
(dense): Dense<input_channels=4096, output_channels=4096, has_bias=True>
>
(post_attention_layernorm): LayerNorm<normalized_shape=[4096], begin_norm_axis=-1, begin_params_axis=-1, weight=Parameter (Tensor(shape=[4096], dtype=Float16, value=[...], name=transformer.layers.17.post_attention_layernorm.weight), requires_grad=True), bias=Parameter (Tensor(shape=[4096], dtype=Float16, value=[...], name=transformer.layers.17.post_attention_layernorm.bias), requires_grad=True)>
(mlp): GLU<
(dense_h_to_4h): Dense<input_channels=4096, output_channels=16384, has_bias=True>
(dense_4h_to_h): Dense<input_channels=16384, output_channels=4096, has_bias=True>
>
>
(18): GLMBlock<
(input_layernorm): LayerNorm<normalized_shape=[4096], begin_norm_axis=-1, begin_params_axis=-1, weight=Parameter (Tensor(shape=[4096], dtype=Float16, value=[...], name=transformer.layers.18.input_layernorm.weight), requires_grad=True), bias=Parameter (Tensor(shape=[4096], dtype=Float16, value=[...], name=transformer.layers.18.input_layernorm.bias), requires_grad=True)>
(attention): SelfAttention<
(rotary_emb): RotaryEmbedding<>
(query_key_value): Dense<input_channels=4096, output_channels=12288, has_bias=True>
(dense): Dense<input_channels=4096, output_channels=4096, has_bias=True>
>
(post_attention_layernorm): LayerNorm<normalized_shape=[4096], begin_norm_axis=-1, begin_params_axis=-1, weight=Parameter (Tensor(shape=[4096], dtype=Float16, value=[...], name=transformer.layers.18.post_attention_layernorm.weight), requires_grad=True), bias=Parameter (Tensor(shape=[4096], dtype=Float16, value=[...], name=transformer.layers.18.post_attention_layernorm.bias), requires_grad=True)>
(mlp): GLU<
(dense_h_to_4h): Dense<input_channels=4096, output_channels=16384, has_bias=True>
(dense_4h_to_h): Dense<input_channels=16384, output_channels=4096, has_bias=True>
>
>
(19): GLMBlock<
(input_layernorm): LayerNorm<normalized_shape=[4096], begin_norm_axis=-1, begin_params_axis=-1, weight=Parameter (Tensor(shape=[4096], dtype=Float16, value=[...], name=transformer.layers.19.input_layernorm.weight), requires_grad=True), bias=Parameter (Tensor(shape=[4096], dtype=Float16, value=[...], name=transformer.layers.19.input_layernorm.bias), requires_grad=True)>
(attention): SelfAttention<
(rotary_emb): RotaryEmbedding<>
(query_key_value): Dense<input_channels=4096, output_channels=12288, has_bias=True>
(dense): Dense<input_channels=4096, output_channels=4096, has_bias=True>
>
(post_attention_layernorm): LayerNorm<normalized_shape=[4096], begin_norm_axis=-1, begin_params_axis=-1, weight=Parameter (Tensor(shape=[4096], dtype=Float16, value=[...], name=transformer.layers.19.post_attention_layernorm.weight), requires_grad=True), bias=Parameter (Tensor(shape=[4096], dtype=Float16, value=[...], name=transformer.layers.19.post_attention_layernorm.bias), requires_grad=True)>
(mlp): GLU<
(dense_h_to_4h): Dense<input_channels=4096, output_channels=16384, has_bias=True>
(dense_4h_to_h): Dense<input_channels=16384, output_channels=4096, has_bias=True>
>
>
(20): GLMBlock<
(input_layernorm): LayerNorm<normalized_shape=[4096], begin_norm_axis=-1, begin_params_axis=-1, weight=Parameter (Tensor(shape=[4096], dtype=Float16, value=[...], name=transformer.layers.20.input_layernorm.weight), requires_grad=True), bias=Parameter (Tensor(shape=[4096], dtype=Float16, value=[...], name=transformer.layers.20.input_layernorm.bias), requires_grad=True)>
(attention): SelfAttention<
(rotary_emb): RotaryEmbedding<>
(query_key_value): Dense<input_channels=4096, output_channels=12288, has_bias=True>
(dense): Dense<input_channels=4096, output_channels=4096, has_bias=True>
>
(post_attention_layernorm): LayerNorm<normalized_shape=[4096], begin_norm_axis=-1, begin_params_axis=-1, weight=Parameter (Tensor(shape=[4096], dtype=Float16, value=[...], name=transformer.layers.20.post_attention_layernorm.weight), requires_grad=True), bias=Parameter (Tensor(shape=[4096], dtype=Float16, value=[...], name=transformer.layers.20.post_attention_layernorm.bias), requires_grad=True)>
(mlp): GLU<
(dense_h_to_4h): Dense<input_channels=4096, output_channels=16384, has_bias=True>
(dense_4h_to_h): Dense<input_channels=16384, output_channels=4096, has_bias=True>
>
>
(21): GLMBlock<
(input_layernorm): LayerNorm<normalized_shape=[4096], begin_norm_axis=-1, begin_params_axis=-1, weight=Parameter (Tensor(shape=[4096], dtype=Float16, value=[...], name=transformer.layers.21.input_layernorm.weight), requires_grad=True), bias=Parameter (Tensor(shape=[4096], dtype=Float16, value=[...], name=transformer.layers.21.input_layernorm.bias), requires_grad=True)>
(attention): SelfAttention<
(rotary_emb): RotaryEmbedding<>
(query_key_value): Dense<input_channels=4096, output_channels=12288, has_bias=True>
(dense): Dense<input_channels=4096, output_channels=4096, has_bias=True>
>
(post_attention_layernorm): LayerNorm<normalized_shape=[4096], begin_norm_axis=-1, begin_params_axis=-1, weight=Parameter (Tensor(shape=[4096], dtype=Float16, value=[...], name=transformer.layers.21.post_attention_layernorm.weight), requires_grad=True), bias=Parameter (Tensor(shape=[4096], dtype=Float16, value=[...], name=transformer.layers.21.post_attention_layernorm.bias), requires_grad=True)>
(mlp): GLU<
(dense_h_to_4h): Dense<input_channels=4096, output_channels=16384, has_bias=True>
(dense_4h_to_h): Dense<input_channels=16384, output_channels=4096, has_bias=True>
>
>
(22): GLMBlock<
(input_layernorm): LayerNorm<normalized_shape=[4096], begin_norm_axis=-1, begin_params_axis=-1, weight=Parameter (Tensor(shape=[4096], dtype=Float16, value=[...], name=transformer.layers.22.input_layernorm.weight), requires_grad=True), bias=Parameter (Tensor(shape=[4096], dtype=Float16, value=[...], name=transformer.layers.22.input_layernorm.bias), requires_grad=True)>
(attention): SelfAttention<
(rotary_emb): RotaryEmbedding<>
(query_key_value): Dense<input_channels=4096, output_channels=12288, has_bias=True>
(dense): Dense<input_channels=4096, output_channels=4096, has_bias=True>
>
(post_attention_layernorm): LayerNorm<normalized_shape=[4096], begin_norm_axis=-1, begin_params_axis=-1, weight=Parameter (Tensor(shape=[4096], dtype=Float16, value=[...], name=transformer.layers.22.post_attention_layernorm.weight), requires_grad=True), bias=Parameter (Tensor(shape=[4096], dtype=Float16, value=[...], name=transformer.layers.22.post_attention_layernorm.bias), requires_grad=True)>
(mlp): GLU<
(dense_h_to_4h): Dense<input_channels=4096, output_channels=16384, has_bias=True>
(dense_4h_to_h): Dense<input_channels=16384, output_channels=4096, has_bias=True>
>
>
(23): GLMBlock<
(input_layernorm): LayerNorm<normalized_shape=[4096], begin_norm_axis=-1, begin_params_axis=-1, weight=Parameter (Tensor(shape=[4096], dtype=Float16, value=[...], name=transformer.layers.23.input_layernorm.weight), requires_grad=True), bias=Parameter (Tensor(shape=[4096], dtype=Float16, value=[...], name=transformer.layers.23.input_layernorm.bias), requires_grad=True)>
(attention): SelfAttention<
(rotary_emb): RotaryEmbedding<>
(query_key_value): Dense<input_channels=4096, output_channels=12288, has_bias=True>
(dense): Dense<input_channels=4096, output_channels=4096, has_bias=True>
>
(post_attention_layernorm): LayerNorm<normalized_shape=[4096], begin_norm_axis=-1, begin_params_axis=-1, weight=Parameter (Tensor(shape=[4096], dtype=Float16, value=[...], name=transformer.layers.23.post_attention_layernorm.weight), requires_grad=True), bias=Parameter (Tensor(shape=[4096], dtype=Float16, value=[...], name=transformer.layers.23.post_attention_layernorm.bias), requires_grad=True)>
(mlp): GLU<
(dense_h_to_4h): Dense<input_channels=4096, output_channels=16384, has_bias=True>
(dense_4h_to_h): Dense<input_channels=16384, output_channels=4096, has_bias=True>
>
>
(24): GLMBlock<
(input_layernorm): LayerNorm<normalized_shape=[4096], begin_norm_axis=-1, begin_params_axis=-1, weight=Parameter (Tensor(shape=[4096], dtype=Float16, value=[...], name=transformer.layers.24.input_layernorm.weight), requires_grad=True), bias=Parameter (Tensor(shape=[4096], dtype=Float16, value=[...], name=transformer.layers.24.input_layernorm.bias), requires_grad=True)>
(attention): SelfAttention<
(rotary_emb): RotaryEmbedding<>
(query_key_value): Dense<input_channels=4096, output_channels=12288, has_bias=True>
(dense): Dense<input_channels=4096, output_channels=4096, has_bias=True>
>
(post_attention_layernorm): LayerNorm<normalized_shape=[4096], begin_norm_axis=-1, begin_params_axis=-1, weight=Parameter (Tensor(shape=[4096], dtype=Float16, value=[...], name=transformer.layers.24.post_attention_layernorm.weight), requires_grad=True), bias=Parameter (Tensor(shape=[4096], dtype=Float16, value=[...], name=transformer.layers.24.post_attention_layernorm.bias), requires_grad=True)>
(mlp): GLU<
(dense_h_to_4h): Dense<input_channels=4096, output_channels=16384, has_bias=True>
(dense_4h_to_h): Dense<input_channels=16384, output_channels=4096, has_bias=True>
>
>
(25): GLMBlock<
(input_layernorm): LayerNorm<normalized_shape=[4096], begin_norm_axis=-1, begin_params_axis=-1, weight=Parameter (Tensor(shape=[4096], dtype=Float16, value=[...], name=transformer.layers.25.input_layernorm.weight), requires_grad=True), bias=Parameter (Tensor(shape=[4096], dtype=Float16, value=[...], name=transformer.layers.25.input_layernorm.bias), requires_grad=True)>
(attention): SelfAttention<
(rotary_emb): RotaryEmbedding<>
(query_key_value): Dense<input_channels=4096, output_channels=12288, has_bias=True>
(dense): Dense<input_channels=4096, output_channels=4096, has_bias=True>
>
(post_attention_layernorm): LayerNorm<normalized_shape=[4096], begin_norm_axis=-1, begin_params_axis=-1, weight=Parameter (Tensor(shape=[4096], dtype=Float16, value=[...], name=transformer.layers.25.post_attention_layernorm.weight), requires_grad=True), bias=Parameter (Tensor(shape=[4096], dtype=Float16, value=[...], name=transformer.layers.25.post_attention_layernorm.bias), requires_grad=True)>
(mlp): GLU<
(dense_h_to_4h): Dense<input_channels=4096, output_channels=16384, has_bias=True>
(dense_4h_to_h): Dense<input_channels=16384, output_channels=4096, has_bias=True>
>
>
(26): GLMBlock<
(input_layernorm): LayerNorm<normalized_shape=[4096], begin_norm_axis=-1, begin_params_axis=-1, weight=Parameter (Tensor(shape=[4096], dtype=Float16, value=[...], name=transformer.layers.26.input_layernorm.weight), requires_grad=True), bias=Parameter (Tensor(shape=[4096], dtype=Float16, value=[...], name=transformer.layers.26.input_layernorm.bias), requires_grad=True)>
(attention): SelfAttention<
(rotary_emb): RotaryEmbedding<>
(query_key_value): Dense<input_channels=4096, output_channels=12288, has_bias=True>
(dense): Dense<input_channels=4096, output_channels=4096, has_bias=True>
>
(post_attention_layernorm): LayerNorm<normalized_shape=[4096], begin_norm_axis=-1, begin_params_axis=-1, weight=Parameter (Tensor(shape=[4096], dtype=Float16, value=[...], name=transformer.layers.26.post_attention_layernorm.weight), requires_grad=True), bias=Parameter (Tensor(shape=[4096], dtype=Float16, value=[...], name=transformer.layers.26.post_attention_layernorm.bias), requires_grad=True)>
(mlp): GLU<
(dense_h_to_4h): Dense<input_channels=4096, output_channels=16384, has_bias=True>
(dense_4h_to_h): Dense<input_channels=16384, output_channels=4096, has_bias=True>
>
>
(27): GLMBlock<
(input_layernorm): LayerNorm<normalized_shape=[4096], begin_norm_axis=-1, begin_params_axis=-1, weight=Parameter (Tensor(shape=[4096], dtype=Float16, value=[...], name=transformer.layers.27.input_layernorm.weight), requires_grad=True), bias=Parameter (Tensor(shape=[4096], dtype=Float16, value=[...], name=transformer.layers.27.input_layernorm.bias), requires_grad=True)>
(attention): SelfAttention<
(rotary_emb): RotaryEmbedding<>
(query_key_value): Dense<input_channels=4096, output_channels=12288, has_bias=True>
(dense): Dense<input_channels=4096, output_channels=4096, has_bias=True>
>
(post_attention_layernorm): LayerNorm<normalized_shape=[4096], begin_norm_axis=-1, begin_params_axis=-1, weight=Parameter (Tensor(shape=[4096], dtype=Float16, value=[...], name=transformer.layers.27.post_attention_layernorm.weight), requires_grad=True), bias=Parameter (Tensor(shape=[4096], dtype=Float16, value=[...], name=transformer.layers.27.post_attention_layernorm.bias), requires_grad=True)>
(mlp): GLU<
(dense_h_to_4h): Dense<input_channels=4096, output_channels=16384, has_bias=True>
(dense_4h_to_h): Dense<input_channels=16384, output_channels=4096, has_bias=True>
>
>
>
(final_layernorm): LayerNorm<normalized_shape=[4096], begin_norm_axis=-1, begin_params_axis=-1, weight=Parameter (Tensor(shape=[4096], dtype=Float16, value=[...], name=transformer.final_layernorm.weight), requires_grad=True), bias=Parameter (Tensor(shape=[4096], dtype=Float16, value=[...], name=transformer.final_layernorm.bias), requires_grad=True)>
>
(lm_head): Dense<input_channels=4096, output_channels=150528>
>