目录
前言
随机森林是一种集成学习方法,主要用于分类和回归任务。它通过构建多个决策树并将其结果结合起来,提高模型的准确性。每棵树在训练时使用数据的随机子集和特征的随机子集,从而降低过拟合风险,并增强模型的泛化能力。最终预测是通过对所有树的预测结果进行投票(分类)或平均(回归)来实现的。
一、集成学习
1.集成学习的含义
集成学习是将多个基础学习器进行组合,来实现比单一学习器显著优越的学习性能
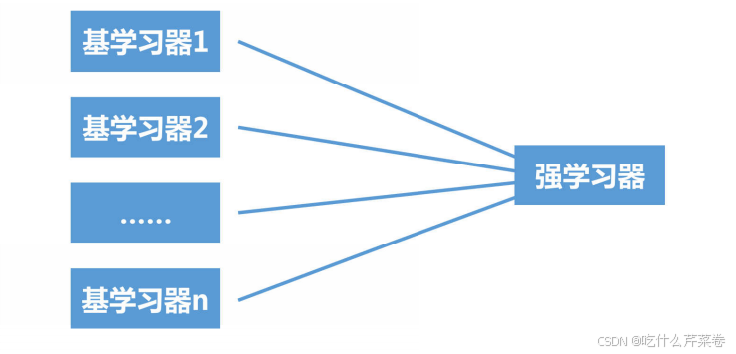
2.集成学习的代表
- bagging 方法:典型的是随机森林
- boosting 方法:典型的是Xgboost
- stacking 方法:堆叠模型
3.集成学习的应用
- 分类问题集成
- 回归问题集成
- 特征选取集成
二、随机森林
1.随机森林的特点
- 数据采样随机:随机从训练集中选取自定百分比的数据
- 特征选取随机:随机从特征中选取自定百分比的特征
- 森林:很多树
- 基分类器为决策树
2.随机森林生成步骤
- 生成多个决策树 :
- 从原始数据集中通过Bootstrap抽样生成多个子集,每个子集用于训练一棵决策树。
- 在每棵树的训练过程中,随机选择特征子集进行节点分裂,增加树的多样性。
- 预测与投票 :
- 对于分类 任务,通过对所有决策树的预测结果进行投票,选择票数最多的类别作为最终预测。
- 对于回归 任务,通过对所有决策树的预测结果进行平均,得到最终的预测值。
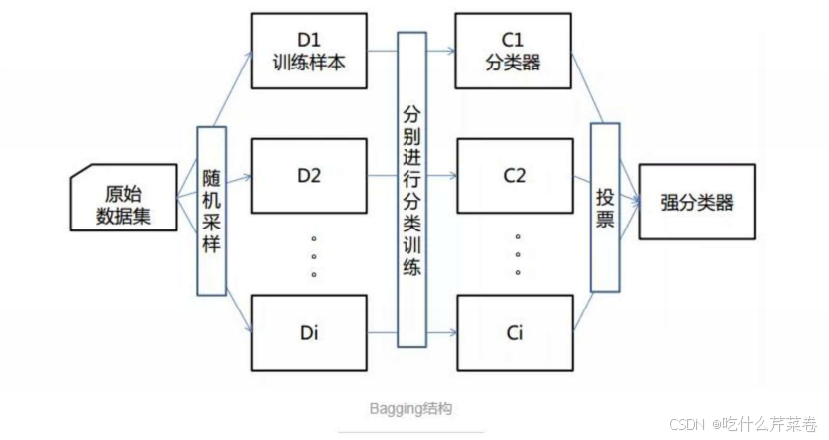
3.随机森林优点
- 具有极高的准确率。
- 随机性的引入,使得随机森林的抗噪声能力很强。
- 随机性的引入,使得随机森林不容易过拟合。
- 能够处理很高维度的数据,不用做特征选择。
- 容易实现并行化计算。
4.随机森林的缺点
- 当随机森林中的决策树个数很多时,训练时需要的空间和时间会较大。
- 随机森林模型还有许多不好解释的地方,有点算个黑盒模型,
三、代码实现
- 本次使用的是多特征二分类数据
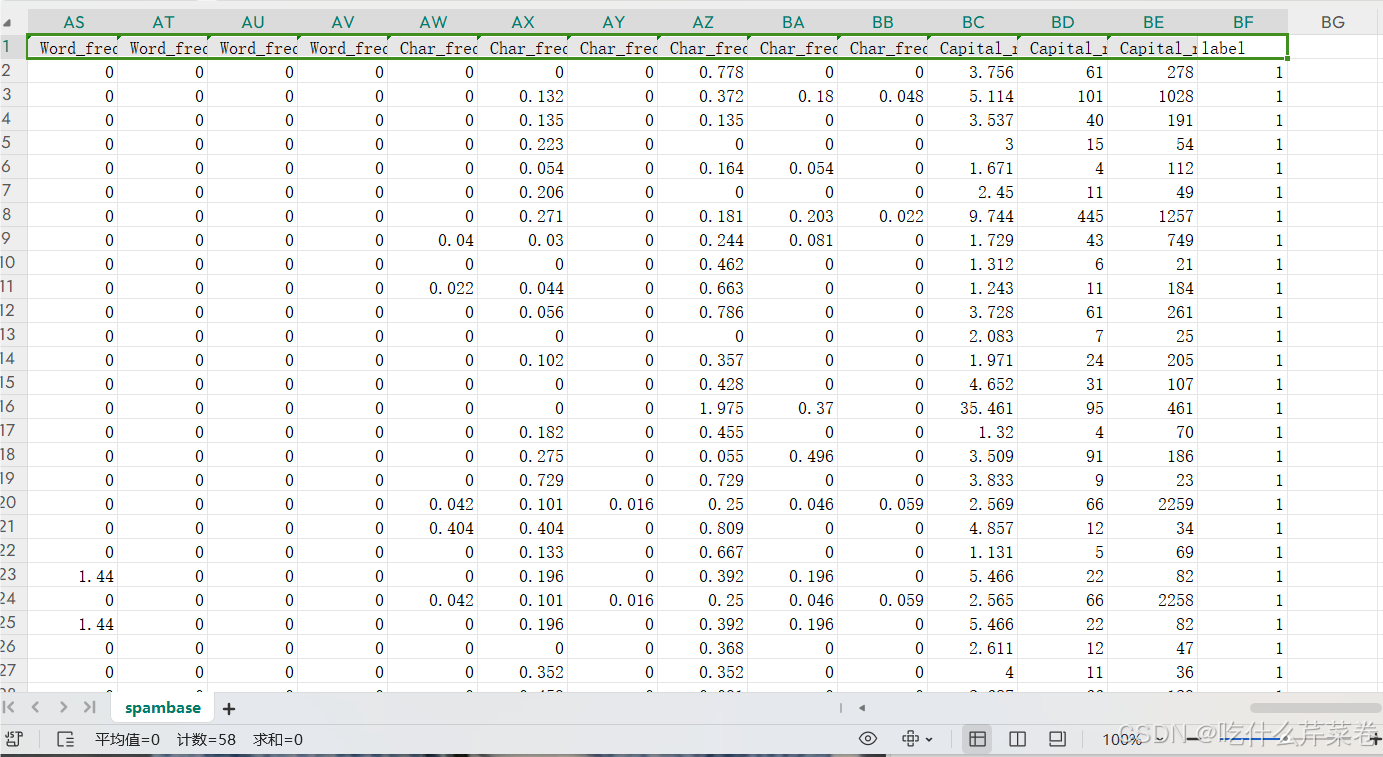
1.完整代码
python
import pandas as pd
from sklearn.model_selection import train_test_split
# 可视化混淆矩阵
def cm_plot(y, yp):
from sklearn.metrics import confusion_matrix
import matplotlib.pyplot as plt
cm = confusion_matrix(y, yp)
plt.matshow(cm, cmap=plt.cm.Blues)
plt.colorbar()
for x in range(len(cm)):
for y in range(len(cm)):
plt.annotate(cm[x, y], xy=(y, x), horizontalalignment='center',
verticalalignment='center')
plt.ylabel('True label')
plt.xlabel('Predicted label')
return plt
data = pd.read_csv('spambase.csv')
x = data.iloc[:, :-1] # 取出特征数据
y = data.iloc[:, -1] # 取出标签
x_train, x_test, y_train, y_test = \
train_test_split(x, y, test_size=0.3, random_state=0)
"""
n_estimators:决策树的个数
max_feature:特征的个数
"""
from sklearn.ensemble import RandomForestClassifier
rf = RandomForestClassifier(
n_estimators=100
, max_features=0.8 # 80%的特征
, random_state=0
)
rf.fit(x_train, y_train)
from sklearn import metrics
train_predict = rf.predict(x_train)
print(metrics.classification_report(y_train, train_predict))
test_predict = rf.predict(x_test)
print(metrics.classification_report(y_test, test_predict))
cm_plot(y_test, test_predict).show()
输出:
- 可视化混淆矩阵------测试集
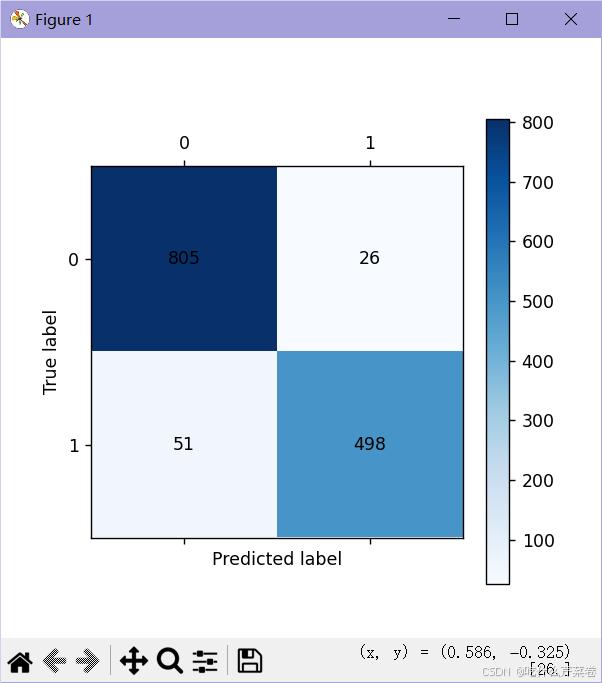
- 混淆矩阵
python
precision recall f1-score support
0 1.00 1.00 1.00 1954
1 1.00 1.00 1.00 1263
accuracy 1.00 3217
macro avg 1.00 1.00 1.00 3217
weighted avg 1.00 1.00 1.00 3217
precision recall f1-score support
0 0.94 0.97 0.95 831
1 0.95 0.91 0.93 549
accuracy 0.94 1380
macro avg 0.95 0.94 0.94 1380
weighted avg 0.94 0.94 0.94 1380
2.数据预处理
- 取出训练集,测试集的特征数据和标签
python
import pandas as pd
from sklearn.model_selection import train_test_split
data = pd.read_csv('spambase.csv')
x = data.iloc[:, :-1]
y = data.iloc[:, -1]
x_train, x_test, y_train, y_test = \
train_test_split(x, y, test_size=0.3, random_state=0)
3.创建并训练模型
- 创建一个100个决策树的随机森林,每棵树选取80%的特征进行训练
python
"""
n_estimators:决策树的个数
max_feature:特征的个数
"""
from sklearn.ensemble import RandomForestClassifier
rf = RandomForestClassifier(
n_estimators=100
, max_features=0.8 # 80%的特征
, random_state=0
)
rf.fit(x_train, y_train)
4.测试模型
- 使用训练集数据和测试集数据进行测试,得到结果
python
from sklearn import metrics
train_predict = rf.predict(x_train)
print(metrics.classification_report(y_train, train_predict))
test_predict = rf.predict(x_test)
print(metrics.classification_report(y_test, test_predict))
cm_plot(y_test, test_predict).show()
输出:
- 虽然训练集数据进行测试时正确率非常高,看起来像过拟合
- 但是不用担心,测试集正确率并没有下降多少
- 说明该模型并没有过拟合
- 可以看出随机森林不仅正确率高,还不容易过拟合
python
precision recall f1-score support
0 1.00 1.00 1.00 1954
1 1.00 1.00 1.00 1263
accuracy 1.00 3217
macro avg 1.00 1.00 1.00 3217
weighted avg 1.00 1.00 1.00 3217
precision recall f1-score support
0 0.94 0.97 0.95 831
1 0.95 0.91 0.93 549
accuracy 0.94 1380
macro avg 0.95 0.94 0.94 1380
weighted avg 0.94 0.94 0.94 1380
总结
本篇讲述了集成学习的概念,随机森林的概念,特点,步骤和优缺点,最后使用代码实例演示了随机森林的使用。