Title
题目
CP-Net: Instance-aware part segmentation network for biological cell parsing
CP-Net:用于生物细胞解析的实例感知部分分割网络
01
文献速递介绍
实例分割是计算机视觉中的一个经典任务,用于识别图像中每个像素的对象类别(语义类型)并确定唯一的对象ID(实例索引)(Yi等,2019)。与仅将对象类别分配给每个像素的语义分割相比,实例分割能够区分同一对象类别内的不同实例(Zhou等,2022)。生物细胞的实例分割在显微图像(例如神经元细胞Upschulte等,2022,血细胞Ljosa等,2012和精子细胞Fraczek等,2022)中对于细胞分析至关重要,如病理分析(Jiang等,2021)、表型变化识别(Yang等,2017)和细胞迁移分析(Tsai等,2019)。
Abatract
摘要
Instance segmentation of biological cells is important in medical image analysis for identifying and segmentingindividual cells, and quantitative measurement of subcellular structures requires further cell-level subcellularpart segmentation. Subcellular structure measurements are critical for cell phenotyping and quality analysis.For these purposes, instance-aware part segmentation network is first introduced to distinguish individualcells and segment subcellular structures for each detected cell. This approach is demonstrated on humansperm cells since the World Health Organization has established quantitative standards for sperm qualityassessment. Specifically, a novel Cell Parsing Net (CP-Net) is proposed for accurate instance-level cell parsing.An attention-based feature fusion module is designed to alleviate contour misalignments for cells with anirregular shape by using instance masks as spatial cues instead of as strict constraints to differentiate variousinstances. A coarse-to-fine segmentation module is developed to effectively segment tiny subcellular structureswithin a cell through hierarchical segmentation from whole to part instead of directly segmenting each cellpart. Moreover, a sperm parsing dataset is built including 320 annotated sperm images with five semanticsubcellular part labels. Extensive experiments on the collected dataset demonstrate that the proposed CP-Netoutperforms state-of-the-art instance-aware part segmentation networks.
生物细胞的实例分割在医学图像分析中非常重要,用于识别和分割单个细胞,而细胞级别的亚细胞结构分割则需要进一步的定量测量。亚细胞结构的测量对细胞表型分析和质量分析至关重要。为此,首次引入了实例感知部分分割网络,以区分单个细胞并对每个检测到的细胞进行亚细胞结构的分割。此方法在人体精子细胞上得到了验证,因为世界卫生组织已制定了精子质量评估的定量标准。具体来说,提出了一种新的细胞解析网络(CP-Net),用于精确的实例级细胞解析。设计了一个基于注意力的特征融合模块,通过使用实例掩码作为空间提示而非严格约束来缓解不规则形状细胞的轮廓不对齐问题。开发了一个粗到细的分割模块,通过从整体到部分的分层分割,而不是直接分割每个细胞部分,有效地分割细胞内的微小亚细胞结构。此外,建立了一个包括320张带有五个语义亚细胞部分标签的注释精子图像的精子解析数据集。在收集的数据集上进行的大量实验表明,所提出的CP-Net优于最先进的实例感知部分分割网络。
Method
方法
Fig. 3 shows the overall architecture of our proposed CP-Net modelfor cell parsing. A convolutional backbone and Feature Pyramid Network (FPN) (Lin et al., 2017) firstly extract features from the inputimage and rescale extracted features, then the instance segmentationmodule and part segmentation module generate instance masks encoding instance-aware information and part segmentation features withcontour-preserved information based on extracted features, finally theattention-based feature fusion module merges and refines instancemasks and part segmentation features to generate instance-aware partparsing results for each instance. The rest part of this section describesthe design of each module.
图3显示了我们提出的用于细胞解析的CP-Net模型的整体架构。首先,通过卷积骨干网络和特征金字塔网络(FPN)(Lin等,2017)从输入图像中提取特征并重新调整提取的特征,然后实例分割模块和部分分割模块基于提取的特征生成编码实例感知信息的实例掩码和保留轮廓信息的部分分割特征,最后基于注意力的特征融合模块将实例掩码和部分分割特征合并和细化,以生成每个实例的实例感知部分解析结果。本节的其余部分将描述各个模块的设计。
Conclusion
结论
This paper reported a Cell Parsing Net (CP-Net) for the instancelevel sperm parsing task. State-of-the-art instance-aware part segmentation networks include top-down, bottom-up and two-stage top-downmethods. The bottom-up methods cannot effectively locate boundaryand separate adjacent instances due to the lack of bounding box locating procedure. In comparison, the top-down methods can betterseparate intersecting instances; however, they suffer from distortionand context loss due to imaging resizing in ROI Align, causing thesubcellular parts of a sperm outside of the bounding box to be croppedout. The two-stage top-down methods can achieve a higher accuracyby combining results from an instance segmentation network and aparallel semantic segmentation network. However, they still suffer frommisalignments because per-pixel matching induces error from inaccurate instance masks. Based on the structure of two-stage top-downmethods, the proposed CP-Net outperformed the classical top-downmethods and bottom-up methods by avoiding feature distortion andimproving instance distinction. This was evidenced by experimentallycomparing the proposed network and classical top-down methods suchas RP-R-CNN and bottom-up methods such as PGN.
本文介绍了一种用于实例级别精子解析任务的细胞解析网络(CP-Net)。当前最先进的实例感知部分分割网络包括自上而下、自下而上和两阶段自上而下的方法。自下而上的方法由于缺少边界框定位过程,无法有效地定位边界和分离相邻实例。相比之下,自上而下的方法能够更好地分离相交的实例;然而,它们由于在ROI Align中图像缩放而导致的变形和上下文丢失问题,可能会将边界框之外的精子亚细胞部分裁剪掉。两阶段自上而下的方法通过结合实例分割网络和并行语义分割网络的结果,可以实现更高的准确性。然而,它们仍然存在错位问题,因为逐像素匹配会由于实例掩码不准确而引入错误。基于两阶段自上而下方法的结构,所提出的CP-Net通过避免特征变形和提高实例区分度,优于传统的自上而下方法和自下而上方法。这一点通过实验比较所提出的网络与传统的自上而下方法(如RP-R-CNN)和自下而上方法(如PGN)得到了证明。
Figure
图
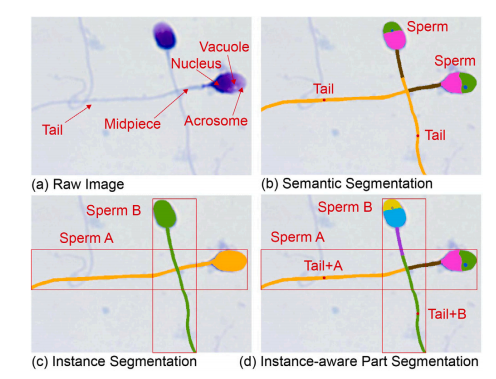
Fig. 1. Examples of sperm cell segmentation in different parsing levels. (a) Raw imageof stained sperm cells taken under 100× microscopy magnification. (b) Semantic segmentation networks cannot distinguish different sperm cells. (c) Instance segmentationnetworks cannot segment subcellular structures. (d) Instance-aware part segmentationcan not only distinguish different sperm cells, but also segment subcellular parts foreach sperm cell.
图1. 不同解析层次下的精子细胞分割示例。(a) 在100倍显微镜放大倍率下拍摄的染色精子细胞原始图像。(b) 语义分割网络无法区分不同的精子细胞。(c) 实例分割网络无法分割亚细胞结构。(d) 实例感知部分分割不仅能够区分不同的精子细胞,还能对每个精子细胞的亚细胞部分进行分割。
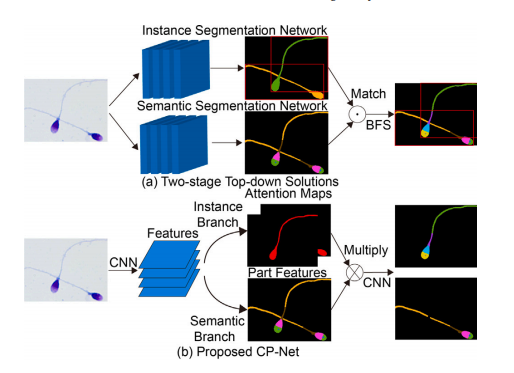
Fig. 2. (a) State-of-the-art two-stage top-down methods spatially per-pixel matchinstance masks and part segmentation maps, then refine the matched result usingBFS-based CCA. (b) Our proposed CP-Net fuses instance masks and part segmentationfeatures through attention mechanism to generate instance-aware part features, whichare then refined by CNN to produce instance-aware results with high quality contours.
图2. (a) 最先进的两阶段自上而下方法逐像素匹配实例掩码和部分分割图,然后使用基于BFS的CCA对匹配结果进行细化。(b) 我们提出的CP-Net通过注意力机制融合实例掩码和部分分割特征,以生成实例感知部分特征,然后通过CNN进行细化,生成具有高质量轮廓的实例感知结果。
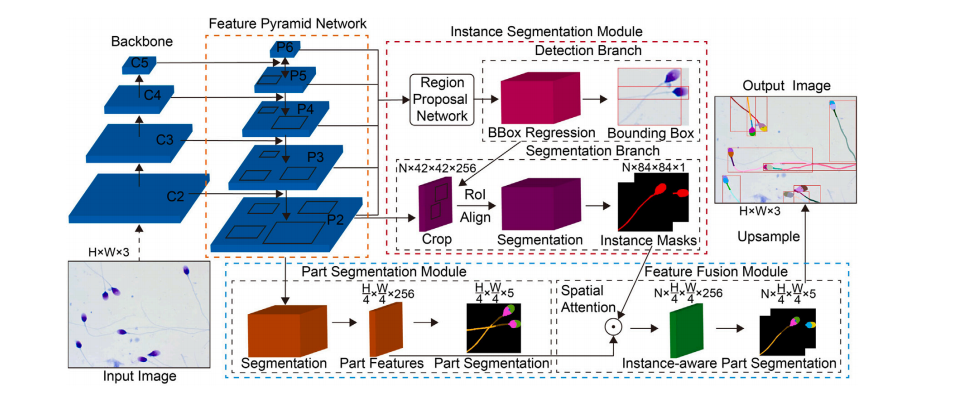
Fig. 3. The architecture of our proposed CP-Net model for instance-level cell parsing. The convolutional backbone first extracts features from the input image, and FPN rescalesextracted features to acquire multi-scale feature maps. Then the instance segmentation module generates instance masks for each cell encoding instance-aware information, and thepart segmentation module produces part segmentation features with detailed contour information. Finally, the attention-based feature fusion module merges and refines instancemasks and part segmentation features to produce accurate part segmentation results for each cell.
图3. 我们提出的CP-Net模型用于实例级细胞解析的架构。卷积骨干网络首先从输入图像中提取特征,FPN对提取的特征进行重新调整以获取多尺度特征图。然后,实例分割模块为每个细胞生成编码实例感知信息的实例掩码,而部分分割模块生成具有详细轮廓信息的部分分割特征。最后,基于注意力的特征融合模块将实例掩码和部分分割特征合并和细化,以生成每个细胞的准确部分分割结果。
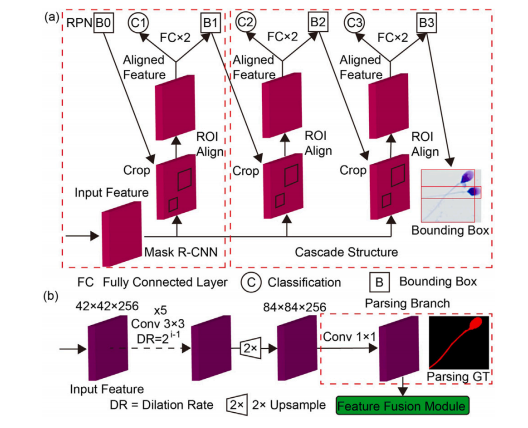
Fig. 4. (a) The structure of the detection branch in the instance segmentation module.A cascaded multi-regression detector is employed to suppress noisy predictions. 𝐵 isbounding box, 𝐶 classification. (b) The segmentation branch segments each instancebased on detection results. A cascaded dilated convolutional network is applied toexpand receptive fields.
图4. (a) 实例分割模块中检测分支的结构。采用级联多回归检测器来抑制噪声预测。𝐵 表示边界框,𝐶 表示分类。(b) 分割分支基于检测结果对每个实例进行分割。应用级联扩张卷积网络来扩展感受野。
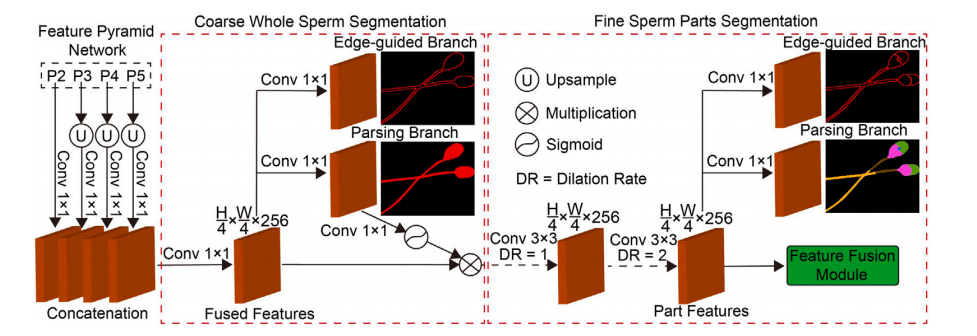
Fig. 5. The structure of our proposed coarse-to-fine part segmentation module. Multi-scale features from FPN are first merged to generate fused features, and a coarse segmentationfor the whole cell is performed to provide a more concentrated subregion where the target occupies a larger fraction. Then a fine segmentation for each part of the cell is performedon this concentrated subregion to better segment subcellular structures.
图5. 我们提出的粗到细部分分割模块的结构。首先,将来自FPN的多尺度特征合并生成融合特征,然后对整个细胞进行粗略分割,以提供一个更集中的子区域,使目标占据更大的比例。然后,在这个集中子区域内对细胞的每个部分进行细致分割,以更好地分割亚细胞结构。
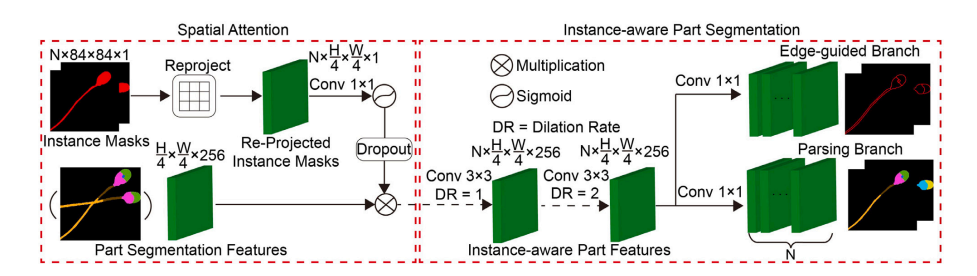
Fig. 6. The structure of our proposed attention-based feature fusion module. Instance masks from the instance segmentation module are first reshaped and re-projected to theiroriginal positions in the image, then merged with part segmentation features from the part segmentation module through the attention mechanism. The generated instance-awarepart features are then refined by cascaded dilated convolution layers to generate part segmentation results with better contours. Further, an edge-guided branch is employed tohelp reconstruct cell contours using edge information.
图6. 我们提出的基于注意力的特征融合模块的结构。实例分割模块生成的实例掩码首先被重塑并重新投影到图像中的原始位置,然后通过注意力机制与部分分割模块生成的部分分割特征进行融合。生成的实例感知部分特征随后通过级联扩张卷积层进行细化,以生成具有更好轮廓的部分分割结果。此外,采用了一个边缘引导分支来利用边缘信息帮助重建细胞轮廓。
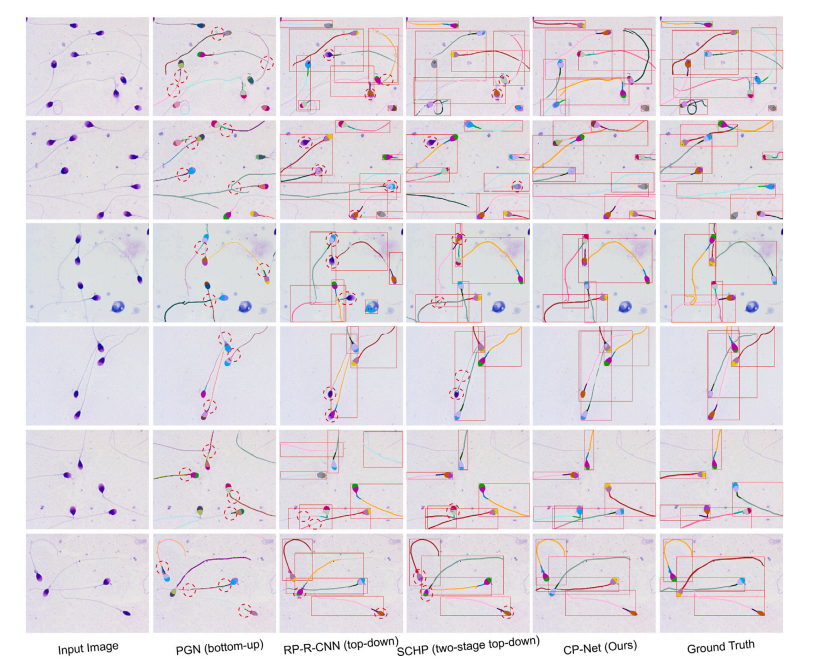
Fig. 7. Qualitative comparison results of instance-level cell parsing on the validation set of our collected Sperm Parsing Dataset. PGN (bottom-up solution) has lower instancedistinction, especially for intersected subcellular parts. RP-R-CNN (top-down solution) has distorted sperm cell mask, and sperm's subcellular parts out of bounding box are neglected.SCHP (two-stage top-down solution) has better mask contours, but still suffers from misalignments. Failure cases are labeled using red dashed circles. In comparison, our proposedCP-Net outperformed the other networks by avoiding contour misalignments and better segmenting tiny subcellular structures.
图7. 我们收集的精子解析数据集验证集上实例级细胞解析的定性比较结果。PGN(自下而上的解决方案)实例区分度较低,特别是在相交的亚细胞部分。RP-R-CNN(自上而下的解决方案)存在精子细胞掩码变形的问题,并且忽略了边界框之外的精子亚细胞部分。SCHP(两阶段自上而下的解决方案)掩码轮廓更好,但仍然存在对齐错误。失败案例用红色虚线圆圈标出。相比之下,我们提出的CP-Net通过避免轮廓错位并更好地分割微小的亚细胞结构,表现优于其他网络。
Table
表
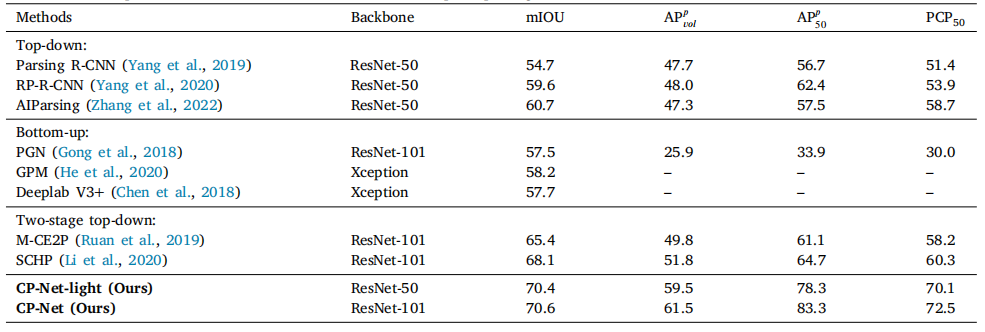
Table 1Quantitative comparisons with state-of-the-art methods on our sperm parsing dataset.
表1 我们的精子解析数据集上与最先进方法的定量比较。
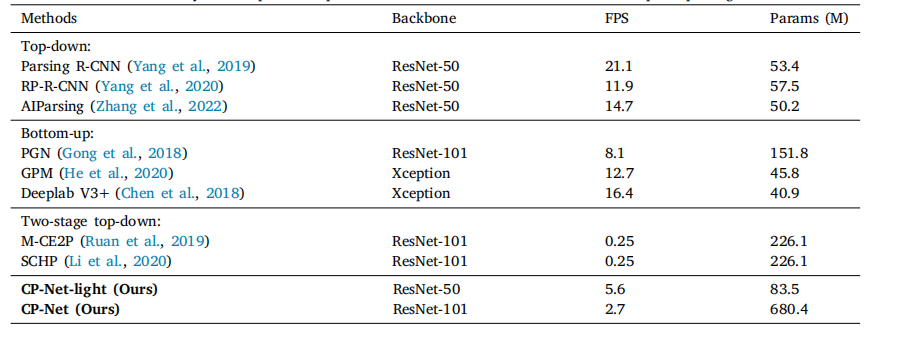
Table 2Inference time and memory consumption comparisons with state-of-the-art methods on our sperm parsing dataset.
表2 我们的精子解析数据集上与最先进方法的推理时间和内存消耗比较。

Table 3Ablation studies of the proposed CP-Net on our sperm parsing dataset
表3 我们在精子解析数据集上对所提出的CP-Net进行的消融研究。