文章目录
前言
💫你好,我是辰chen,本文旨在准备考研复试或就业
💫本篇博客内容来自:Machine Learning 2022 Spring
💫更多和本篇博客相关内容详见专栏:Machine Learning(李宏毅2022春) 以及 机器学习(Machine Learning)
💫欢迎大家的关注,我的博客主要关注于算法讲解、LLM大模型、通信感知等
以下的几个专栏是本人比较满意的专栏(大部分专栏仍在持续更新),欢迎大家的关注:
💥考研
代码一
python3
import torch
import torch.nn as nn
import torch.nn.functional as F
import numpy as np
import pandas as pd
import math
seed = 15
torch.backends.cudnn.deterministic = True # 使用确定性算法,确保可重复性
torch.backends.cudnn.benkmark = False # 关闭 cuDNN 的自动优化机制,确保可重复性
np.random.seed(seed)
torch.manual_seed(seed) # 设置 PyTorch 的随机种子
if torch.cuda.is_available():
torch.cuda.manual_seed(seed) # 设置所有 GPU 设备的随机种子,确保在多 GPU 环境下的结果一致
print(True)
python3
import os
# 查看当前工作目录
current_path = os.getcwd()
print(f"当前路径:{current_path}")
python3
df = pd.read_csv('data/kaggle/ml2021spring-hw1/covid.train.csv')
test_df = pd.read_csv('data/kaggle/ml2021spring-hw1/covid.test.csv')
df.head()

python3
df.shape
python3
test_df.shape
python3
# combined_df 仍会保留 95 列,test_df 缺失的第 95 列将被填充为 NaN
combined_df = pd.concat([df, test_df])
x = df[df.columns[1:94]]
y = df[df.columns[94]]
python3
from sklearn.feature_selection import SelectKBest # 用于特征选择,选择得分最高的 k 个特征
from sklearn.feature_selection import f_regression # 用于计算每个特征与目标变量之间的相关性评分
"""
score_func=f_regression : 使用 F 检验(方差分析)来计算每个特征的相关性评分
k=14 : 选择得分最高的 14 个特征
Q:为什么选取的是 14 个特征?
A:下一个代码块运行的结果,即查看所有的得分,最高的 14 个特征得分明显高于第 15 个特征的得分
"""
bestfeatures = SelectKBest(score_func = f_regression, k = 14)
fit = bestfeatures.fit(x, y)
cols = bestfeatures.get_support(indices = True)
"""
使用 iloc 按列索引筛选原数据框 df 中的列,只保留得分最高的 14 个特征
选中列索引为 cols 的列,并更新 df
"""
df = df.iloc[:, cols]
df.head()

python3
df.shape
python3
# fit.scores_ 是 SelectKBest 拟合后的属性,它存储每个特征与目标变量之间的 F 值
dfscores = pd.DataFrame(fit.scores_)
dfcolumns = pd.DataFrame(x.columns)
featureScores = pd.concat([dfcolumns, dfscores], axis=1)
featureScores.columns = ['Space', 'Score']
print(featureScores.nlargest(15, 'Score'))
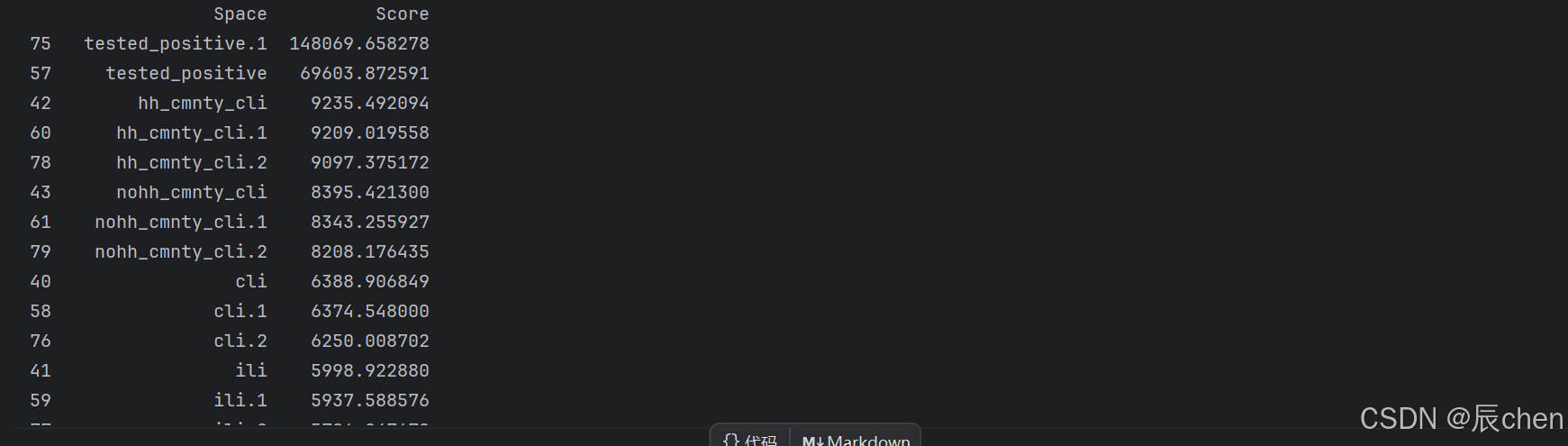
python3
for col in df.columns:
print(col)
标准化(Standardization)是机器学习中的一种常见预处理步骤,它通过对特征进行变换,使得每个特征的数值范围相似。这在一些算法中尤为重要,例如线性回归、支持向量机和神经网络等,它们对数据的尺度非常敏感。
python3
"""
对数据进行标准化处理
将 pandas 数据框中的数据转换为 PyTorch 的 CUDA 张量
便于在 GPU 上进行训练
"""
mean_std = {}
for col in df.columns: # 遍历数据框 df 中的每一列,对每个特征进行标准化
mean_std[col] = (combined_df[col].mean(), combined_df[col].std())
df[col] = (df[col] - mean_std[col][0]) / mean_std[col][1]
x = df[df.columns].values
y = y.values
x = torch.Tensor(x).cuda()
y = torch.Tensor(y).cuda()
标准化后的值 = 原始值 − 均值 标准差 标准化后的值=\frac{原始值-均值}{标准差} 标准化后的值=标准差原始值−均值
在这段代码中,使用 combined_df
的均值和标准差对 df
进行标准化,这种做法是因为数据集需要在训练和测试数据的同一分布下进行标准化。这是常见的实践方法。
保持训练和测试数据的同一分布:
- 在实际的机器学习中,标准化通常基于整个数据集的统计信息(即训练集和测试集的组合),而不是仅基于训练集或测试集。
- 如果训练集和测试集分别使用自己的均值和标准差进行标准化,那么标准化后的分布可能会有偏差,导致模型在测试时表现不佳。
- 通过使用合并后的数据集(
combined_df
)来计算均值和标准差,可以确保训练和测试数据在同一分布下被缩放,从而更好地泛化模型。
避免数据泄露:
- 在训练模型时,我们不能使用测试数据来计算均值和标准差,因为这可能导致数据泄露。
- 但在预处理的标准化阶段,如果是基于交叉验证或模型评估的场景下,使用整个数据集的统计信息来标准化数据是可以的,因为模型本身并没有在测试集上进行训练。
后续代码中同样会对 test_df
的列进行标准化,目前是为了训练模型,故代码不放在这里
python3
df.head()

python3
class Net(nn.Module):
def __init__(self):
super(Net, self).__init__()
self.fc1 = nn.Linear(14, 32)
self.fc2 = nn.Linear(32, 1)
def forward(self, x):
x = self.fc1(x)
x = F.relu(x)
x = self.fc2(x)
return x
python3
network = Net()
network.cuda()
# weight_decay=7e-3:设置权重衰减(L2 正则化),可以防止过拟合
optimizer = torch.optim.RAdam(network.parameters(), lr=7.5e-5, weight_decay=7e-3)
python3
epochs = 10000
batch_size = 128
train_losses = []
test_losses = []
# # Early Stopping parameters
ES_patience = 500 # 当在连续 500 轮中模型在验证集上的损失不再下降时,训练会提前终止
ES_counter = 0 # 记录连续损失未下降的次数
best_epoch = 0 # 记录当前最好的轮数
best_loss = 1000 # 记录训练过程中的最小损失
python3
def train(epoch):
network.train()
for i in range(len(x_train) // batch_size):
# 在每个小批次训练开始前,先将模型的梯度缓存清零,以免前一次反向传播的梯度影响当前的更新
optimizer.zero_grad()
pred = network(x_train[batch_size * i : batch_size * (i + 1)])
"""
pred.view(-1):将预测值展平为一维,以便与目标值形状匹配
"""
loss = F.mse_loss(pred.view(-1), y_train[batch_size * i : batch_size * (i + 1)])
loss.backward()
optimizer.step()
train_losses.append(loss.item())
python3
def test(epoch):
global best_epoch, best_loss, ES_counter
network.eval()
with torch.no_grad():
pred = network(x_test)
loss = F.mse_loss(pred.view(-1), y_test)
# Early Stopping
if best_loss > loss:
ES_counter, best_epoch, best_loss = 0, epoch, loss
torch.save(network.state_dict(), 'data/kaggle/ml2021spring-hw1/model.pth')
print('Saving model (epoch = {:4d}, MSE loss = {:.4f})'.format(epoch, loss))
else:
ES_counter += 1
if ES_counter == ES_patience:
print('Early Stopping (Best epoch = {:4d}, Best MSE loss = {:4f})'.format(best_epoch, best_loss))
test_losses.append(loss.item())
python3
from sklearn.model_selection import train_test_split # 用于将数据集拆分为训练集和测试集
for epoch in range(1, epochs + 1):
x_train, x_test, y_train, y_test = train_test_split(x, y, test_size=0.3, random_state=epoch % 10)
train(epoch)
test(epoch)
if ES_counter == ES_patience:
break
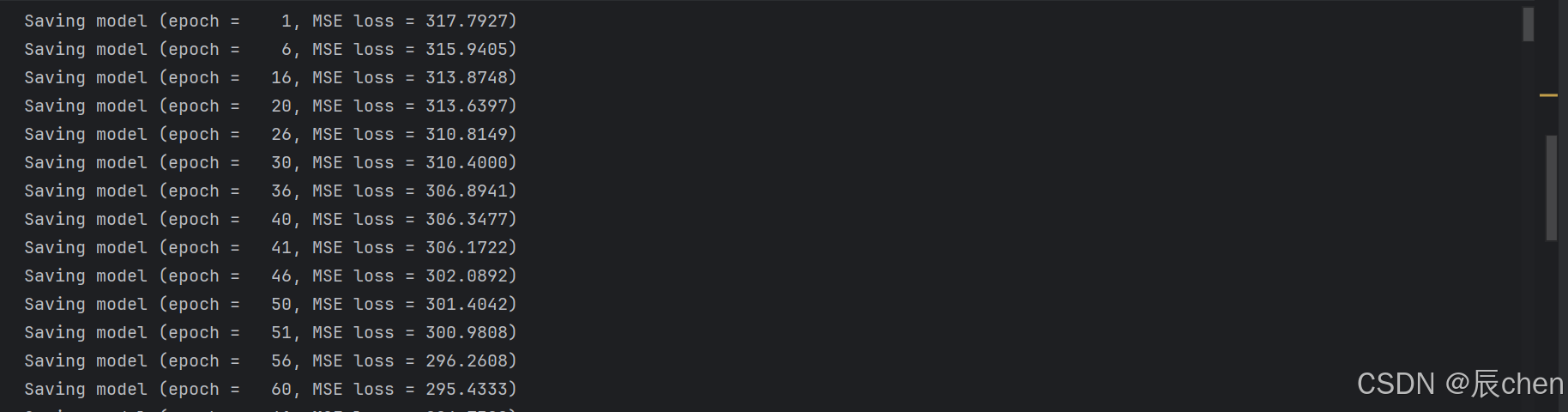
python3
import matplotlib.pyplot as plt
fig = plt.figure()
plt.plot(range(len(train_losses)), train_losses, color='red')
plt.plot(range(len(train_losses)), test_losses, color='blue')
plt.legend(['Train Loss', 'Test Loss'], loc='upper right')
plt.xlabel('Epoch')
plt.ylabel('Loss')
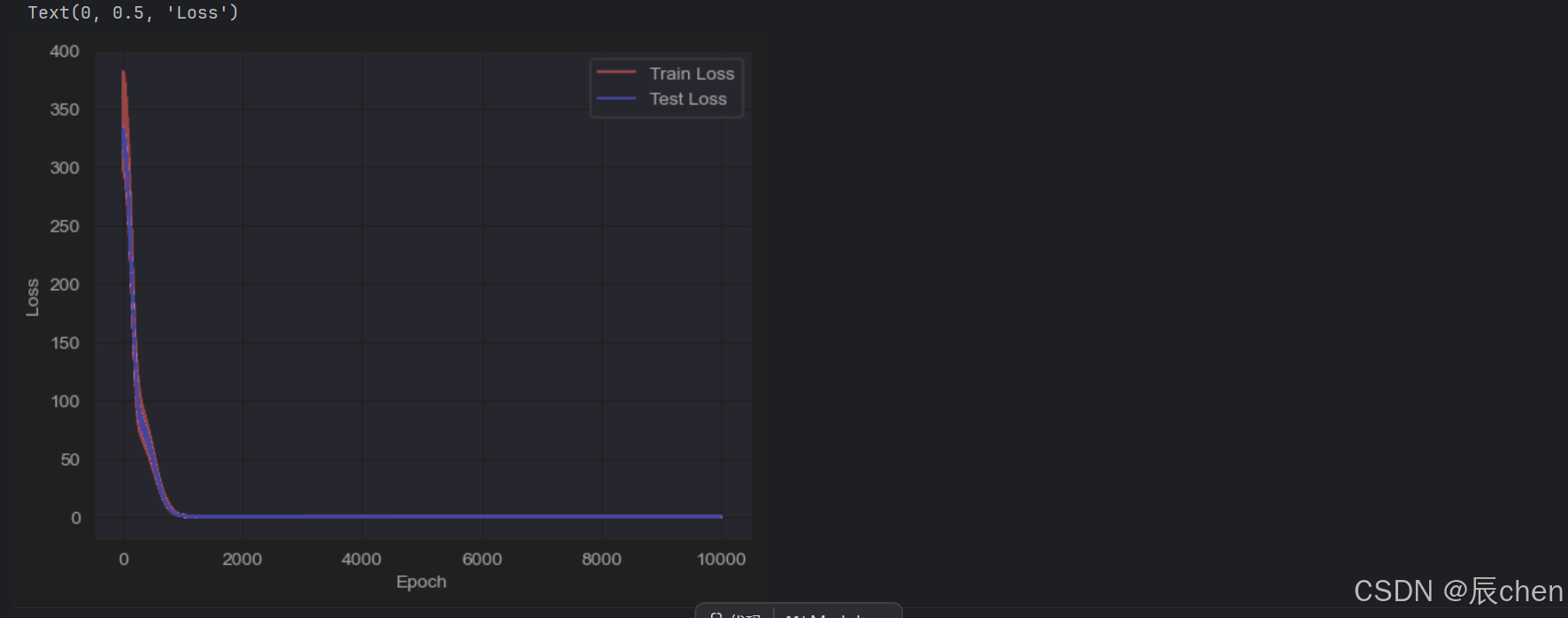
python3
best_network = Net().cuda()
network_state_dict = torch.load('data/kaggle/ml2021spring-hw1/model.pth')
best_network.load_state_dict(network_state_dict)
python3
best_network.eval()
test_df = test_df.iloc[:, cols]
for col in test_df.columns:
test_df[col] = (test_df[col] - mean_std[col][0]) / mean_std[col][1]
test_X = test_df.values # Pandas to Numpy
test_X = torch.Tensor(test_X).cuda() # Numpy to Tensor
pred = best_network(test_X)
python3
import csv
print('Saving Results')
with open('data/kaggle/ml2021spring-hw1/my_submission.csv', "w", newline='') as fp:
writer = csv.writer(fp)
writer.writerow(['id', 'tested_positive'])
for i, p in enumerate(pred):
writer.writerow([i, p.item()])
代码二
python3
import numpy as np
import pandas as pd
from tqdm import tqdm
import matplotlib.pyplot as plt
import seaborn as sns
from sklearn.preprocessing import MinMaxScaler
from sklearn.feature_selection import mutual_info_regression as mir
from sklearn.metrics import mean_squared_error as mse
from sklearn.model_selection import KFold
# 下面三个包都是进行梯度提升决策树(GBDT)的机器学习框架,适合于分类、回归和排序等任务
from sklearn import linear_model
import xgboost as xgb
import lightgbm as lgbm
import catboost as cb
python3
train = pd.read_csv('data/kaggle/ml2021spring-hw1/covid.train.csv', index_col='id')
test = pd.read_csv('data/kaggle/ml2021spring-hw1/covid.test.csv', index_col='id')
sample = pd.read_csv('data/kaggle/ml2021spring-hw1/sampleSubmission.csv', index_col='id')
python3
train.shape
train.csv 结构 :
- 前40列是 One Hot 编码
- 后54列被分为三天的数据 (18 * 3)
- 4列 : 类似 COVID 疾病
- 8列 : 行为指标
- 5列 : 心理健康指标
- 1列 : 检测呈阳性的病例(目标预测)
python3
train[train.columns[:40]] # 展示了前 40 列的数据
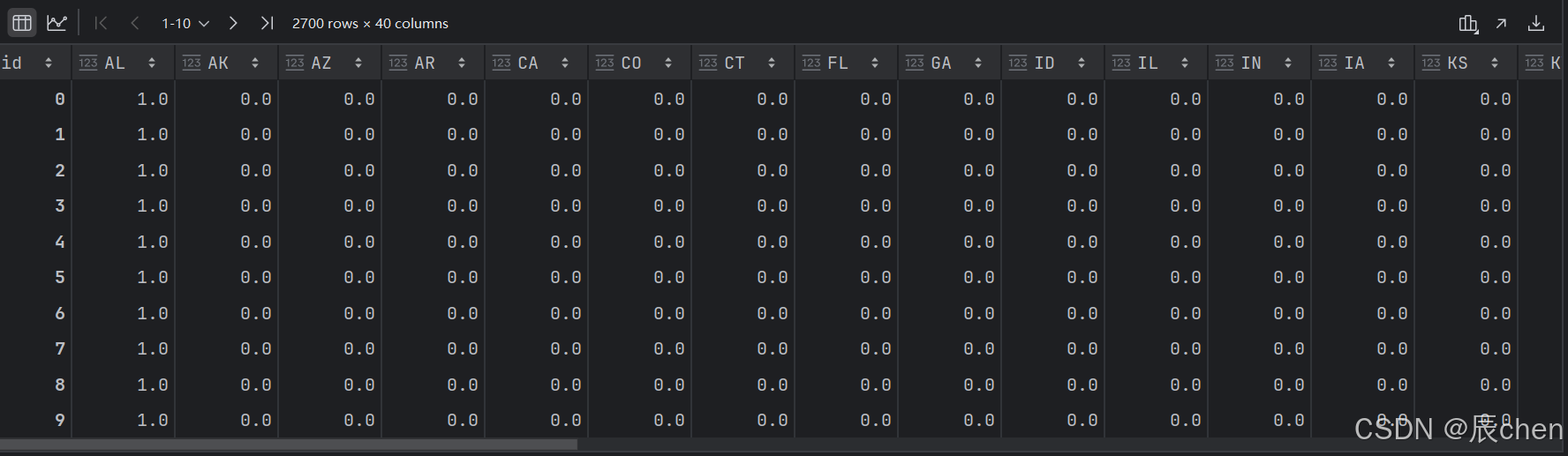
python3
day_one = train.columns[40:58]
day_two = train.columns[58:76]
day_three = train.columns[76:]
python3
train[day_one].head()

python3
train[day_two].head()

python3
train[day_three].head()

python3
def kfold_cv(model, X, y):
kf = KFold(n_splits=5)
scores = []
for train_index, test_index in kf.split(X, y):
"""
X_train 和 X_test:从 X 中提取训练和测试数据,使用 .iloc 根据索引进行切分
y 只有一列,所以可以直接使用 y[train_idx] 和 y[test_idx] 来根据索引进行切分
"""
X_train, X_test = X.iloc[train_index], X.iloc[test_index]
y_train, y_test = y[train_index], y[test_index]
model.fit(X_train, y_train)
scores.append(mse(y_test, model.predict(X_test), squared=True)) # squared=True 表示计算的是均方误差(MSE),而非均方根误差(RMSE)
return np.mean(scores) # 返回所有折的 MSE 均值,即模型在 5 折交叉验证中的平均误差
python3
"""
由于是三天的数据,所以会有三个tested_positive
当 CSV 文件被读取时,如果存在重复的列名,Pandas 会自动给这些列名加上后缀,后缀通常是 .1、.2
假设原始 CSV 文件中有以下列 :
id, tested_positive, tested_positive, tested_positive
在这种情况下,Pandas 读取后会自动重命名这些列为 :
id, tested_positive, tested_positive.1, tested_positive.2
我们最终的目标也是根据前两天去估计test中第三天的tested_positive
"""
X = train.drop(columns=['tested_positive.2'])
y = train['tested_positive.2']
python3
"""
mir(X, y) 是一个函数,用于计算每个特征与目标变量 y 之间的相关性得分
index=X.columns:将 DataFrame 的索引设置为 X 的列名
.sort_values():用于对 DataFrame 的值进行排序
by=0:表示按照第 0 列(也就是互信息得分列)进行排序
ascending=False:表示按照降序排序,这样得分高的特征会排在上面
"""
top_features = pd.DataFrame(mir(X, y), index=X.columns).sort_values(by=0, ascending=False)
python3
top_features.head(20)
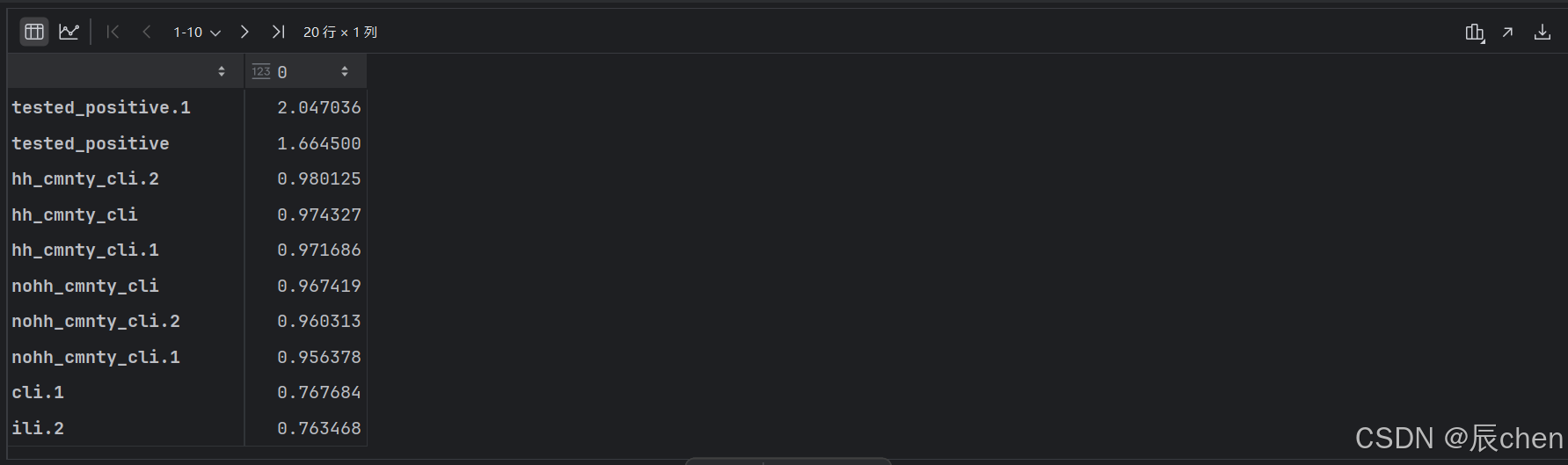
python3
# 从运行结果可以看出前14个特征值分数明显高于后面的分数,故仅选取前14个特征值
selected_features = top_features.index[:14]
X_selected = X[selected_features]
test_selected = test[selected_features]
python3
# 从运行结果可以看出前14个特征值分数明显高于后面的分数,故仅选取前14个特征值
selected_features = top_features.index[:14]
X_selected = X[selected_features]
test_selected = test[selected_features]
python3
"""
"xgboost": xgb.XGBRegressor(n_jobs=-1):
XGBoost 是一种高效的梯度提升决策树模型。
n_jobs=-1:使用所有可用的 CPU 核心进行并行计算(此模型被注释掉了)。
"catboost": cb.CatBoostRegressor(thread_count=-1, verbose=0):
CatBoost 是一种高效的梯度提升模型,擅长处理类别特征(此模型也被注释掉了)。
thread_count=-1:使用所有可用线程。
verbose=0:不打印训练过程的详细信息。
"lightgbm": lgbm.LGBMRegressor(n_jobs=-1):
LightGBM 是一种高效的梯度提升模型。
n_jobs=-1:使用所有 CPU 核心进行并行计算。
"linear_regression": linear_model.LinearRegression():
线性回归模型,用于线性关系的预测。
"ridgecv": linear_model.RidgeCV():
带有交叉验证的 Ridge 回归模型。
使用 L2 正则化来防止过拟合。
"lasso": linear_model.LassoCV():
带有交叉验证的 Lasso 回归模型。
使用 L1 正则化,自动选择最重要的特征。
"elasticnet": linear_model.ElasticNetCV():
带有交叉验证的弹性网络回归模型。
结合了 L1 和 L2 正则化的优点。
"ardregression": linear_model.ARDRegression():
ARD 回归模型,自动相关确定性回归,属于贝叶斯回归的一种。
"lassolarscv": linear_model.LassoLarsCV():
带有交叉验证的 LassoLars(最小角回归)模型,用于稀疏特征的选择。
"lassolarsic": linear_model.LassoLarsIC():
基于信息准则(如 AIC 或 BIC)选择特征的 LassoLars 回归模型。
"bayesianridge": linear_model.BayesianRidge():
贝叶斯 Ridge 回归模型,使用贝叶斯方法估计回归系数。
"""
model_list = {
# "xgboost": xgb.XGBRegressor(n_jobs=-1),
# "catboost": cb.CatBoostRegressor(thread_count=-1, verbose=0),
"lightgbm": lgbm.LGBMRegressor(n_jobs=-1),
"linear_regression": linear_model.LinearRegression(),
"ridgecv": linear_model.RidgeCV(),
"lasso": linear_model.LassoCV(),
"elasticnet": linear_model.ElasticNetCV(),
"ardregression": linear_model.ARDRegression(),
"lassolarscv": linear_model.LassoLarsCV(),
"lassolarsic": linear_model.LassoLarsIC(),
"bayesianridge": linear_model.BayesianRidge(),
}
python3
import warnings
warnings.filterwarnings('ignore')
python3
# 通过交叉验证评估模型性能
for name, model in model_list.items():
print(f'{name}: {kfold_cv(model, X_selected, y)}')
"""
对测试集进行预测和集成
把每个模型预测出的predictions数组的对应位置的对应元素直接相加
然后取平均作为最终的输出
这种取多模型预测平均值的方法是一种合理的集成学习策略,特别是当模型的性能相近时
在一些 Kaggle 比赛或回归问题中,简单平均常常能够提高成绩
可以根据实际情况尝试加权平均、Stacking 等方法来进一步提升性能
"""
predictions = np.zeros(893,)
for name, model in model_list.items():
predictions += model.predict(test[selected_features])
predictions = predictions / len(model_list)

python3
submission = pd.DataFrame({
'id': test.index,
'tested_positive': predictions
})
save_path = 'data/kaggle/ml2021spring-hw1/try_model_submission.csv'
submission.to_csv(save_path, index=False)
对比
baseline
的设定如下图:
如果使用老师提供的未经优化的代码,分数如图:
使用代码一优化,分数如图:
使用代码二优化,分数如图: