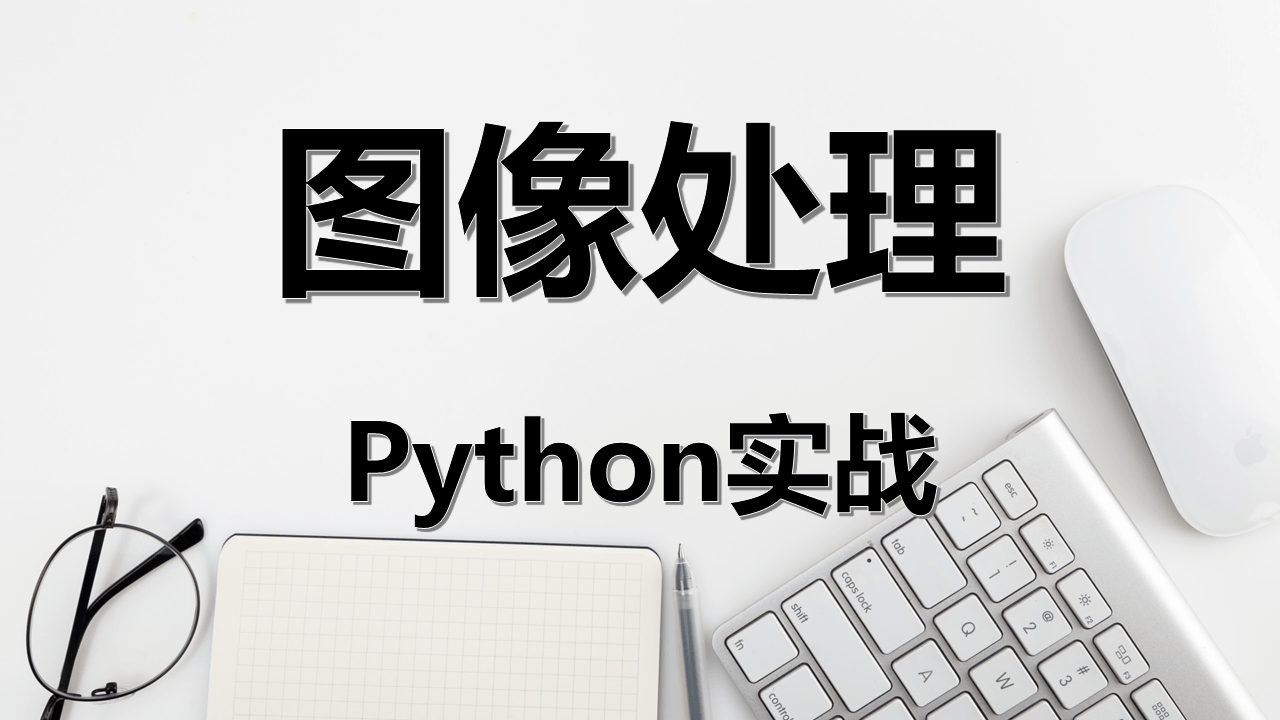
在掌握了Python图像处理的基础和进阶技巧后,本篇将继续深入探讨更多图像处理技术,并通过具体的实战案例帮助读者理解和掌握这些技术的应用场景和实现方法。本文将涵盖图像分割与语义分割、视频处理与分析、三维重建以及图像增强与预处理等主题。
1. 图像分割与语义分割
图像分割是指将数字图像细分为多个区域或捕捉到图像中的对象或边界的任务。它可以分为二值分割、多区域分割等类型。而语义分割不仅分割图像,还为每个像素分配一个类别标签,从而确定该像素属于哪个对象或区域。
1.1 基于深度学习的语义分割
语义分割是图像分割的一种形式,它为图像中的每个像素分配一个类别标签。常用的深度学习模型包括U-Net、Mask R-CNN、DeepLab等。这里以U-Net为例,展示如何使用深度学习模型进行语义分割:
python
import torch
import torch.nn as nn
import torch.optim as optim
import torch.nn.functional as F
from torch.utils.data import DataLoader
from torchvision import datasets, transforms
from torchvision.transforms import ToTensor
from PIL import Image
import matplotlib.pyplot as plt
import numpy as np
# U-Net定义
class UNet(nn.Module):
def __init__(self, n_channels, n_classes):
super(UNet, self).__init__()
self.inc = DoubleConv(n_channels, 64)
self.down1 = Down(64, 128)
self.down2 = Down(128, 256)
self.down3 = Down(256, 512)
self.down4 = Down(512, 512)
self.up1 = Up(1024, 256)
self.up2 = Up(512, 128)
self.up3 = Up(256, 64)
self.up4 = Up(128, 64)
self.outc = OutConv(64, n_classes)
def forward(self, x):
x1 = self.inc(x)
x2 = self.down1(x1)
x3 = self.down2(x2)
x4 = self.down3(x3)
x5 = self.down4(x4)
x = self.up1(x5, x4)
x = self.up2(x, x3)
x = self.up3(x, x2)
x = self.up4(x, x1)
logits = self.outc(x)
return logits
# 卷积层
class DoubleConv(nn.Module):
def __init__(self, in_channels, out_channels):
super(DoubleConv, self).__init__()
self.double_conv = nn.Sequential(
nn.Conv2d(in_channels, out_channels, kernel_size=3, padding=1),
nn.BatchNorm2d(out_channels),
nn.ReLU(inplace=True),
nn.Conv2d(out_channels, out_channels, kernel_size=3, padding=1),
nn.BatchNorm2d(out_channels),
nn.ReLU(inplace=True)
)
def forward(self, x):
return self.double_conv(x)
# 下采样层
class Down(nn.Module):
def __init__(self, in_channels, out_channels):
super(Down, self).__init__()
self.maxpool_conv = nn.Sequential(
nn.MaxPool2d(2),
DoubleConv(in_channels, out_channels)
)
def forward(self, x):
return self.maxpool_conv(x)
# 上采样层
class Up(nn.Module):
def __init__(self, in_channels, out_channels, bilinear=True):
super(Up, self).__init__()
if bilinear:
self.up = nn.Upsample(scale_factor=2, mode='bilinear', align_corners=True)
self.conv = DoubleConv(in_channels, out_channels)
else:
self.up = nn.ConvTranspose2d(in_channels, in_channels // 2, kernel_size=2, stride=2)
self.conv = DoubleConv(in_channels, out_channels)
def forward(self, x1, x2):
x1 = self.up(x1)
diffY = x2.size()[2] - x1.size()[2]
diffX = x2.size()[3] - x1.size()[3]
x1 = F.pad(x1, [diffX // 2, diffX - diffX // 2, diffY // 2, diffY - diffY // 2])
x = torch.cat([x2, x1], dim=1)
return self.conv(x)
# 输出层
class OutConv(nn.Module):
def __init__(self, in_channels, out_channels):
super(OutConv, self).__init__()
self.conv = nn.Conv2d(in_channels, out_channels, kernel_size=1)
def forward(self, x):
return self.conv(x)
# 加载预训练模型
model = UNet(n_channels=3, n_classes=2)
model.load_state_dict(torch.load('path/to/unet_model.pth', map_location=torch.device('cpu')))
model.eval()
# 加载图像
image = Image.open('path/to/image.jpg')
transform = transforms.Compose([
transforms.Resize((256, 256)),
transforms.ToTensor()
])
image_tensor = transform(image).unsqueeze(0)
# 进行语义分割
with torch.no_grad():
output = model(image_tensor)
# 处理输出图像
seg_image = torch.argmax(output, dim=1).squeeze().numpy()
plt.imshow(seg_image, cmap='gray')
plt.axis('off')
plt.show()
2. 视频处理与分析
视频处理涉及从视频中提取有用的信息,如动作识别、场景理解等。这里介绍如何使用3D CNN进行动作识别:
2.1 动作识别
使用深度学习模型(如3D CNN)进行动作识别:
python
import torch
import torch.nn as nn
from torch.utils.data import DataLoader
from torchvision import datasets, transforms
import numpy as np
import cv2
# 3D CNN定义
class Simple3DNet(nn.Module):
def __init__(self):
super(Simple3DNet, self).__init__()
self.conv1 = nn.Conv3d(3, 16, kernel_size=(3, 3, 3), padding=1)
self.pool = nn.MaxPool3d(kernel_size=(1, 2, 2), stride=(1, 2, 2))
self.conv2 = nn.Conv3d(16, 32, kernel_size=(3, 3, 3), padding=1)
self.fc = nn.Linear(32 * 16 * 16, 10)
def forward(self, x):
x = F.relu(self.conv1(x))
x = self.pool(x)
x = F.relu(self.conv2(x))
x = self.pool(x)
x = x.view(-1, 32 * 16 * 16)
x = self.fc(x)
return x
# 加载预训练模型
model = Simple3DNet()
model.load_state_dict(torch.load('path/to/3d_cnn_model.pth', map_location=torch.device('cpu')))
model.eval()
# 读取视频
video_path = 'path/to/video.mp4'
video = cv2.VideoCapture(video_path)
frames = []
# 提取帧
while video.isOpened():
ret, frame = video.read()
if not ret:
break
frames.append(frame)
video.release()
# 将帧转换为Tensor
frames = np.array(frames)
frames = frames.transpose((3, 0, 1, 2))
frames = torch.from_numpy(frames).unsqueeze(0).float()
# 进行动作识别
with torch.no_grad():
outputs = model(frames)
# 处理输出
predicted_class = torch.argmax(outputs, dim=1).item()
print(f"Predicted class: {predicted_class}")
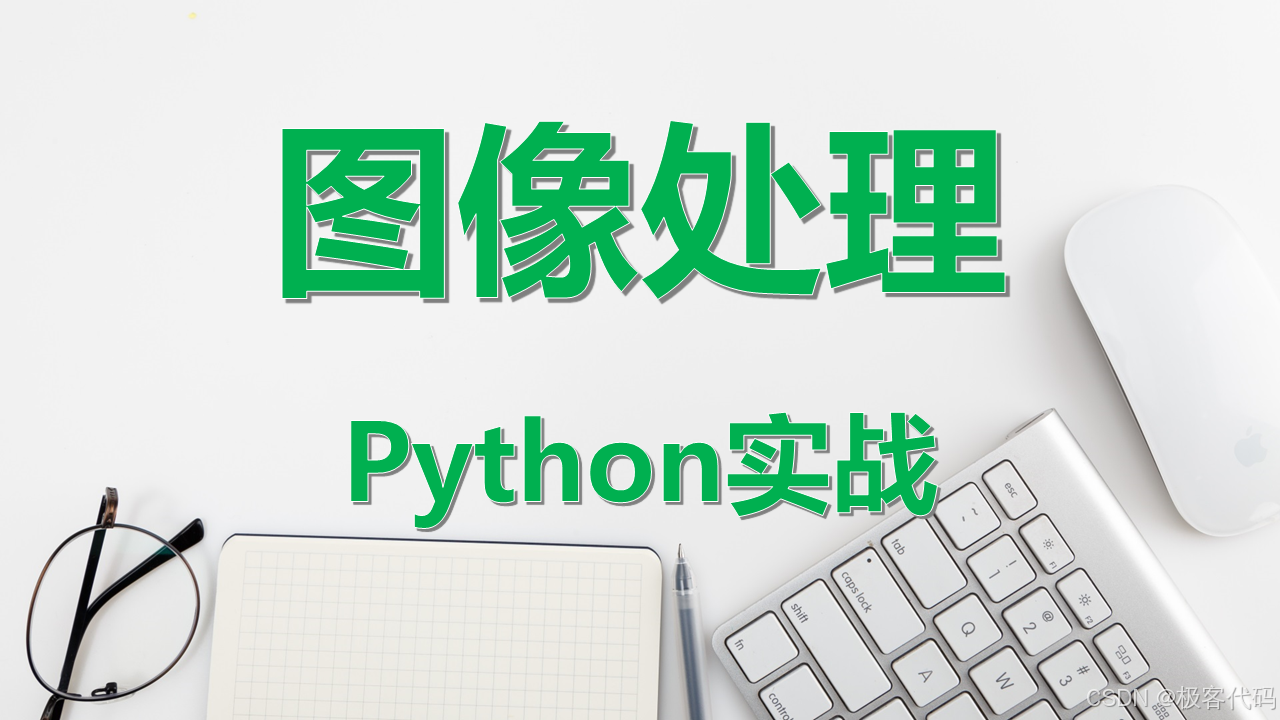
3. 三维重建
三维重建是指从二维图像中恢复物体或场景三维几何形状的过程。常见的三维重建方法包括结构从运动(SfM)、光束法平差(BA)等。这里介绍如何使用OpenCV进行结构从运动的三维重建:
3.1 结构从运动(SfM)
使用OpenCV进行结构从运动的三维重建:
python
import cv2
import numpy as np
from matplotlib import pyplot as plt
# 读取视频
video_path = 'path/to/video.mp4'
video = cv2.VideoCapture(video_path)
# 初始化特征检测器
sift = cv2.SIFT_create()
# 创建特征匹配器
matcher = cv2.BFMatcher(cv2.NORM_L2, crossCheck=True)
# 存储特征点
features = []
# 逐帧处理
while video.isOpened():
ret, frame = video.read()
if not ret:
break
# 转换为灰度图像
gray = cv2.cvtColor(frame, cv2.COLOR_BGR2GRAY)
# 检测特征点
keypoints, descriptors = sift.detectAndCompute(gray, None)
# 存储特征点
features.append((keypoints, descriptors))
video.release()
# 构建稀疏点云
point_cloud = []
for i in range(len(features) - 1):
keypoints1, descriptors1 = features[i]
keypoints2, descriptors2 = features[i + 1]
# 特征匹配
matches = matcher.match(descriptors1, descriptors2)
matches = sorted(matches, key=lambda x: x.distance)
# 构建点云
points = []
for match in matches:
point1 = keypoints1[match.queryIdx].pt
point2 = keypoints2[match.trainIdx].pt
points.append((point1, point2))
point_cloud.extend(points)
# 可视化点云
plt.scatter(*zip(*point_cloud), s=1)
plt.show()
4. 图像增强与预处理
图像增强和预处理是提高图像质量的关键步骤。这里介绍如何使用OpenCV进行自适应直方图均衡化:
4.1 自适应直方图均衡化
使用OpenCV进行自适应直方图均衡化:
python
import cv2
import numpy as np
import matplotlib.pyplot as plt
# 读取图像
image = cv2.imread('path/to/image.jpg', cv2.IMREAD_GRAYSCALE)
# 应用自适应直方图均衡化
clahe = cv2.createCLAHE(clipLimit=2.0, tileGridSize=(8, 8))
enhanced_image = clahe.apply(image)
# 显示原始图像和增强后的图像
plt.figure(figsize=(10, 5))
plt.subplot(121)
plt.imshow(image, cmap='gray')
plt.title('Original Image')
plt.axis('off')
plt.subplot(122)
plt.imshow(enhanced_image, cmap='gray')
plt.title('Enhanced Image')
plt.axis('off')
plt.show()
总结
通过本篇的深入探讨,你应该已经掌握了Python在图像处理方面的一些进阶技术,包括语义分割、视频处理与分析、三维重建以及图像增强与预处理。这些技术在实际应用中有着广泛的需求,例如在医疗诊断、自动驾驶、虚拟现实等领域。随着技术的不断进步和发展,图像处理仍然是一个充满挑战和机遇的研究方向。希望这些实战案例能为你提供灵感和指导,在未来的工作中发挥重要作用。