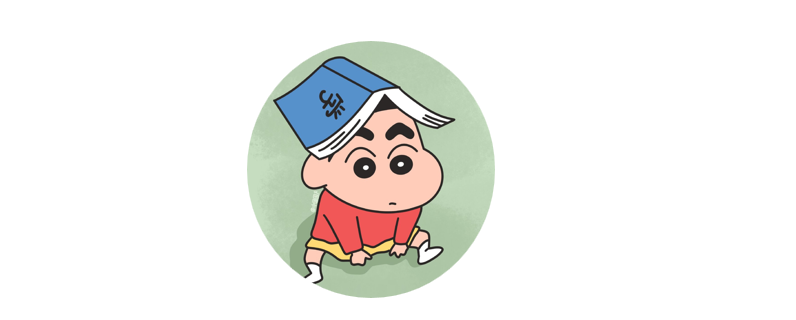
📝个人主页🌹:Eternity._
🌹🌹期待您的关注 🌹🌹
❀ 农作物病害分类
概述
农作物病害是国家粮食安全的一个主要威胁,是决定农作物产量和质量的主要因素。 由于传统方法缺乏必要的基础设施,并且极大程度依赖于人工经验,故诸多地区难以迅速高效地防治病害,从而影响农业的发展。因此,精确诊断农作物病害对于促进农业可持续发展至关重要。针对传统的农作物病害识别方法具有主观性并且极大程度依赖于人工经验的不足,利用卷积神经网络对农作物病害进行识别与分类。首先,利用数据增强技术扩充农作物病害原始数据集,增加数据的多样性和数量,同时可以提高训练网络的泛化能力和识别精度;然后搭建卷积神经网络对农作物图像进行病虫害的特征提取和分类,实现对农作物病害的准确识别和分类。最后通过搭建本地Web实现识别分类的可视化,可以详见视频。值得注意的是作者本人使用的是PlantVillage数据集进行训练、验证以及测试的。
Plant Village数据集共包含14中植物类别,分别为苹果、蓝莓、樱桃、玉米、葡萄、柑橘、桃、胡椒、马铃薯、树莓、大豆、南瓜、草莓和番茄。
本文所涉及的所有资源的获取方式:这里
演示效果
这里我只放核心服务的图像
识别界面

核心逻辑
这个是flask的路由设置代码
python
import os
from flask import Flask, redirect, render_template, request
from PIL import Image
import torchvision.transforms.functional as tf
import CNN
import numpy as np
import torch
import pandas as pd
import smtplib
from email.mime.text import MIMEText
disease_info = pd.read_csv('static/materials/disease_infov1.csv', encoding='utf-8')
supplement_info = pd.read_csv('static/materials/supplement_info.csv', encoding='utf-8')
model = CNN.CNN(38)
model.load_state_dict(torch.load("static/save_model/plant_disease_model_xhh500.pt"))
model.eval()
def prediction(image_path):
image = Image.open(image_path)
image = image.resize((224, 224))
input_data = tf.to_tensor(image)
input_data = input_data.view((-1, 3, 224, 224))
output = model(input_data)
output = output.detach().numpy()
index = np.argmax(output)
return index
app = Flask(__name__)
@app.route('/', methods=['GET', 'POST'])
def home_page():
return render_template('home.html')
@app.route('/contact')
def contact():
return render_template('contact.html')
@app.route('/services')
def services():
return render_template('services.html')
@app.route('/about')
def about():
return render_template('about.html')
@app.route('/typo')
def buchong():
return render_template('typo.html')
@app.route('/submit', methods=['GET', 'POST'])
def submit():
if request.method == 'POST':
image = request.files['image']
filename = image.filename
file_path = os.path.join('static/uploads', filename)
image.save(file_path)
print(file_path)
pred = prediction(file_path)
title = disease_info['disease_name'][pred]
description = disease_info['description'][pred]
prevent = disease_info['Possible Steps'][pred]
image_url = disease_info['image_url'][pred]
print(f"image_url: {image_url}")
supplement_name = supplement_info['supplement name'][pred]
supplement_image_url = supplement_info['supplement image'][pred]
supplement_buy_link = supplement_info['buy link'][pred]
return render_template('submit.html', title=title, desc=description, prevent=prevent,
image_url=image_url, pred=pred, sname=supplement_name, simage=supplement_image_url,
buy_link=supplement_buy_link)
@app.route('/market', methods=['GET', 'POST'])
def market():
return render_template('market.html', supplement_image=list(supplement_info['supplement image']),
supplement_name=list(supplement_info['supplement name']),
disease=list(disease_info['disease_name']), buy=list(supplement_info['buy link']))
if __name__ == '__main__':
app.config['JSON_AS_ASCII'] = False
app.run(debug=True)
这个是模型训练的代码
python
import pandas as pd
import torch.nn as nn
class CNN(nn.Module):
def __init__(self, K):
super(CNN, self).__init__()
self.conv_layers = nn.Sequential(
# conv1
nn.Conv2d(in_channels=3, out_channels=32, kernel_size=3, padding=1),
nn.ReLU(),
nn.BatchNorm2d(32),
nn.Conv2d(in_channels=32, out_channels=32, kernel_size=3, padding=1),
nn.ReLU(),
nn.BatchNorm2d(32),
nn.MaxPool2d(2),
# conv2
nn.Conv2d(in_channels=32, out_channels=64, kernel_size=3, padding=1),
nn.ReLU(),
nn.BatchNorm2d(64),
nn.Conv2d(in_channels=64, out_channels=64, kernel_size=3, padding=1),
nn.ReLU(),
nn.BatchNorm2d(64),
nn.MaxPool2d(2),
# conv3
nn.Conv2d(in_channels=64, out_channels=128, kernel_size=3, padding=1),
nn.ReLU(),
nn.BatchNorm2d(128),
nn.Conv2d(in_channels=128, out_channels=128, kernel_size=3, padding=1),
nn.ReLU(),
nn.BatchNorm2d(128),
nn.MaxPool2d(2),
# conv4
nn.Conv2d(in_channels=128, out_channels=256, kernel_size=3, padding=1),
nn.ReLU(),
nn.BatchNorm2d(256),
nn.Conv2d(in_channels=256, out_channels=256, kernel_size=3, padding=1),
nn.ReLU(),
nn.BatchNorm2d(256),
nn.MaxPool2d(2),
)
self.dense_layers = nn.Sequential(
nn.Dropout(0.4),
nn.Linear(50176, 1024),
nn.ReLU(),
nn.Dropout(0.4),
nn.Linear(1024, K),
)
def forward(self, X):
out = self.conv_layers(X)
# Flatten
out = out.view(-1, 50176)
# Fully connected
out = self.dense_layers(out)
return out
idx_to_classes = {0: 'Apple___Apple_scab',
1: 'Apple___Black_rot',
2: 'Apple___Cedar_apple_rust',
3: 'Apple___healthy',
4: 'Blueberry___healthy',
5: 'Cherry___healthy',
6: 'Cherry___Powdery_mildew',
7: 'Corn___Cercospora_leaf_spot Gray_leaf_spot',
8: 'Corn___Common_rust',
9: 'Corn___Northern_Leaf_Blight',
10: 'Corn___healthy',
11: 'Grape___Black_rot',
12: 'Grape___Esca_(Black_Measles)',
13: 'Grape___Leaf_blight_(Isariopsis_Leaf_Spot)',
14: 'Grape___healthy',
15: 'Orange___Haunglongbing_(Citrus_greening)',
16: 'Peach___Bacterial_spot',
17: 'Peach___healthy',
18: 'Pepper,_bell___Bacterial_spot',
19: 'Pepper,_bell___healthy',
20: 'Potato___Early_blight',
21: 'Potato___Late_blight',
22: 'Potato___healthy',
23: 'Raspberry___healthy',
24: 'Soybean___healthy',
25: 'Squash___Powdery_mildew',
26: 'Strawberry___Leaf_scorch',
27: 'Strawberry___healthy',
28: 'Tomato___Bacterial_spot',
29: 'Tomato___Early_blight',
30: 'Tomato___Late_blight',
31: 'Tomato___Leaf_Mold',
32: 'Tomato___Septoria_leaf_spot',
33: 'Tomato___Spider_mites Two-spotted_spider_mite',
34: 'Tomato___Target_Spot',
35: 'Tomato___Tomato_Yellow_Leaf_Curl_Virus',
36: 'Tomato___Tomato_mosaic_virus',
37: 'Tomato___healthy'}
使用方式
下载附件即可。
部署方式
Pycharm+Flask+Pytorch,安装成功之后即可在本地运行。
编程未来,从这里启航!解锁无限创意,让每一行代码都成为你通往成功的阶梯,帮助更多人欣赏与学习!
更多内容详见:这里