一、张量
在PyTorch中,张量是PyTorch中最基本的数据结构。张量可以看作是一个多维数组,可以在GPU上加速运算。PyTorch的张量和Numpy的数组非常类似,但是与Numpy不同的是,PyTorch的张量可以自动地在GPU上进行加速计算。
PyTorch中的张量是深度学习模型中最常用的数据结构,它可以存储模型的输入、输出以及模型的参数,并且可以通过自动求导来计算梯度,方便进行反向传播算法的实现。
1、张量的数据类型
torch.float32
或torch.float
: 默认的浮点数类型,32位浮点数。torch.float64
或torch.double
: 64位浮点数。torch.float16
或torch.half
: 半精度浮点数。适用于GPU计算,可以节省内存和计算资源。torch.int8
: 8位整数。torch.int16
或torch.short
: 16位整数。torch.int32
或torch.int
: 32位整数。torch.int64
或torch.long
: 64位整数。torch.bool
: 布尔类型,只能是True或False。
2、张量的创建
在PyTorch中,可以使用多种方式来创建张量。下面是一些常用的方法:
2.1、使用torch.tensor()
函数:
可以使用torch.tensor()
函数从Python列表、元组、NumPy数组等数据结构中创建一个新的张量。例如:
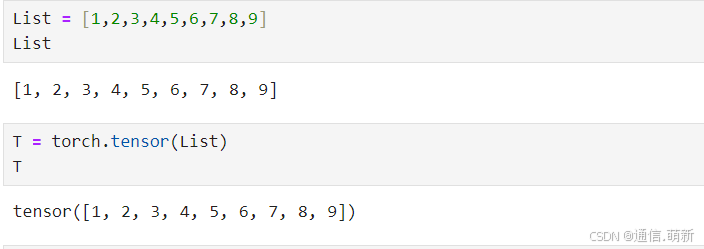
张量的维度可以通过.shape查看,并可使用.size0方法计算张量的形状大小,使用.numel0方法计算张量中包含元素的数量,例如:
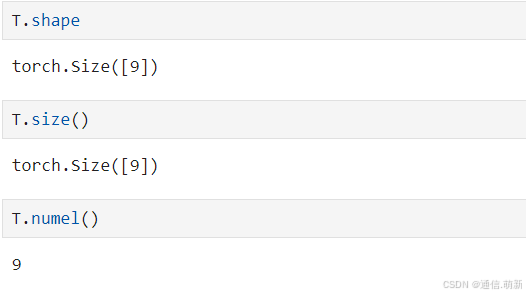
还可以使用参数dtype来指定张量的数据类型,使用参数requires_grad来指定张量是否需要计算梯度(只有浮点数才能计算梯度)。例如:
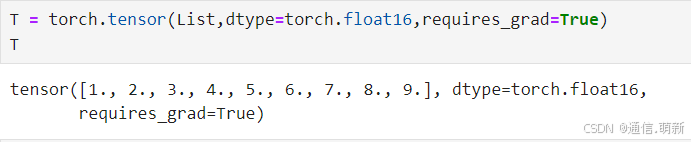
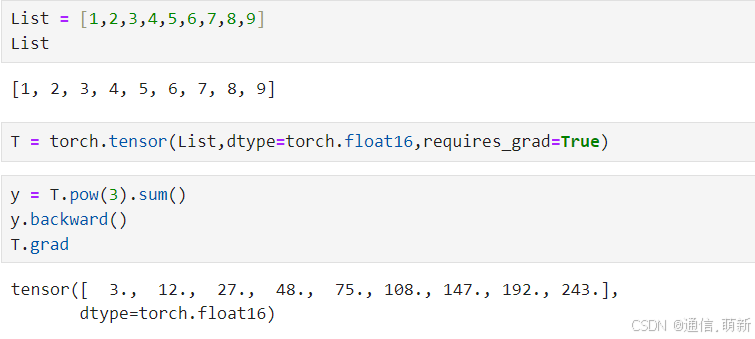
2.2、使用torch.Tensor()
函数:
在PyToreh中也可使用loreh.Tensor()函数来生成张量,而且可以根据指定的形状生成张量。
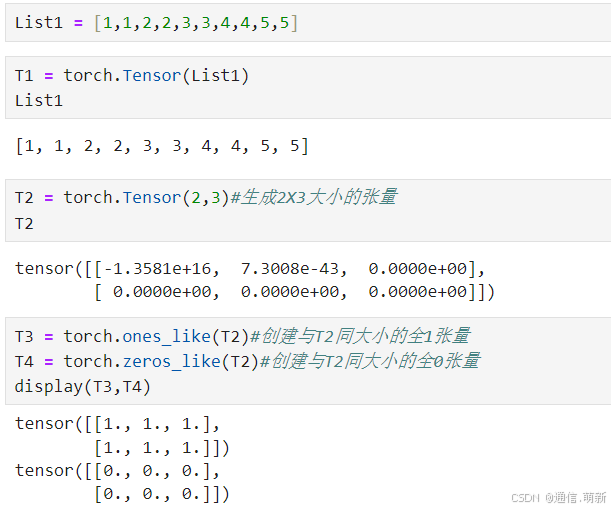
2.3、使用numpy数组转化为张量
将Numpy数组转化为PyTorch张量,可以使用torch.as_tensor()兩数和torehrom_numpy()函数,例如:
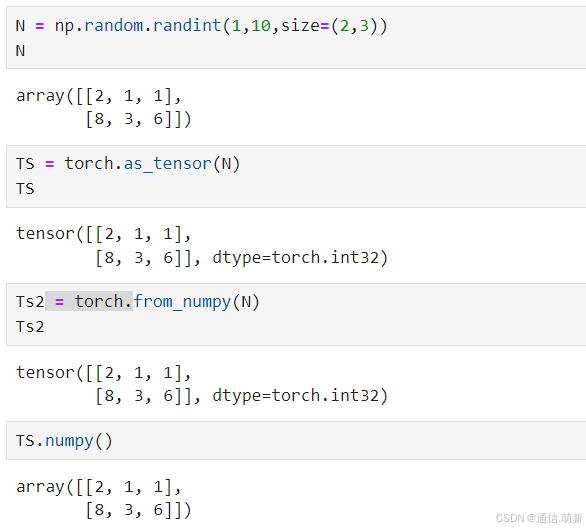
2.4、随机数生成张量
在PyToreh中还可以通过相关随机数来生成张量,并且可以指定生成随机数的分布函数等。在生成随机数之前,可以使用torch.manual_seed()函数,指定生成随机数的种子,用于保证生成的随机数是可重复出现的。
-
torch.randn():从标准正态分布中生成随机数。
-
torch.rand():从0到1之间的均匀分布中生成随机数。
-
torch.randint():在给定的范围内生成随机整数。
4.torch.normal():生成服从正态分布的随机数
2.5、其他
torch.zeros():全0张量
torch.ones():全1张量
torch.eye():单位张量
torch.full(():填充张量
torch.empty():空张量
3、张量操作
3.1、改变张量大小
改变张量的形状在深度学习的使用过程中经常会遇到,而且针对不同的情况对张量形状尺寸的改变有多种函数和方法可以使用,如tensor.reshape()方法可以设置张量的形状大小。
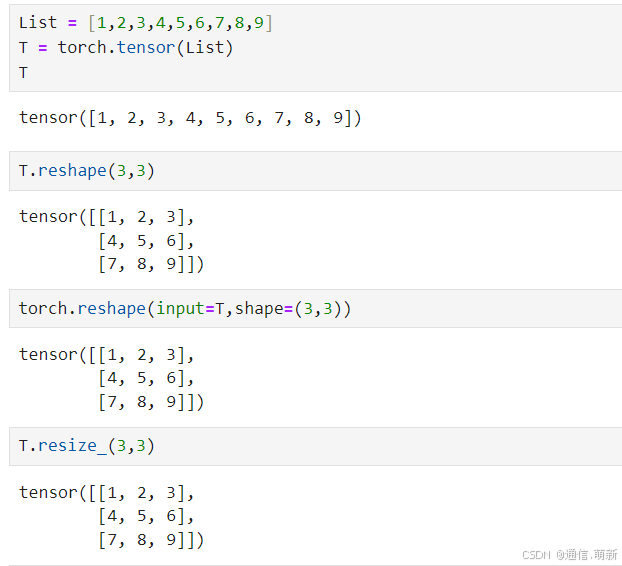
3.2、获取张量元素
该操作类似于python中数组的访问和切片
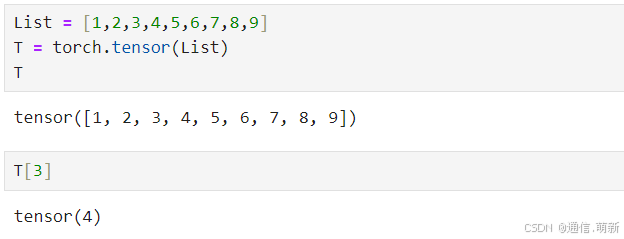
3.3、拼接和拆分张量
在PyTorch中,可以使用torch.cat()或torch.stack()
函数来拼接张量,使用torch.split()
或torch.chunk()
函数来拆分张量。
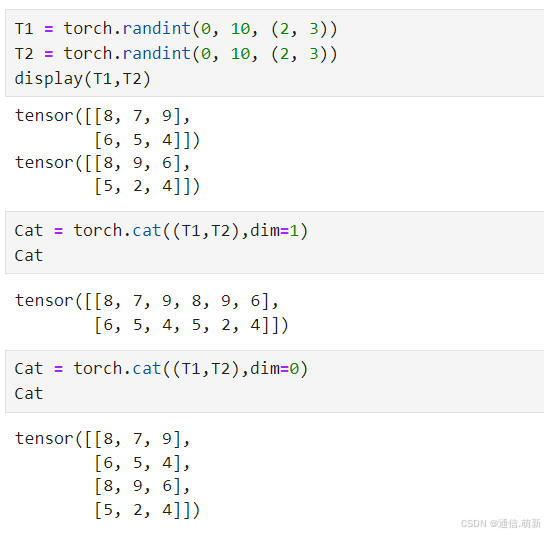
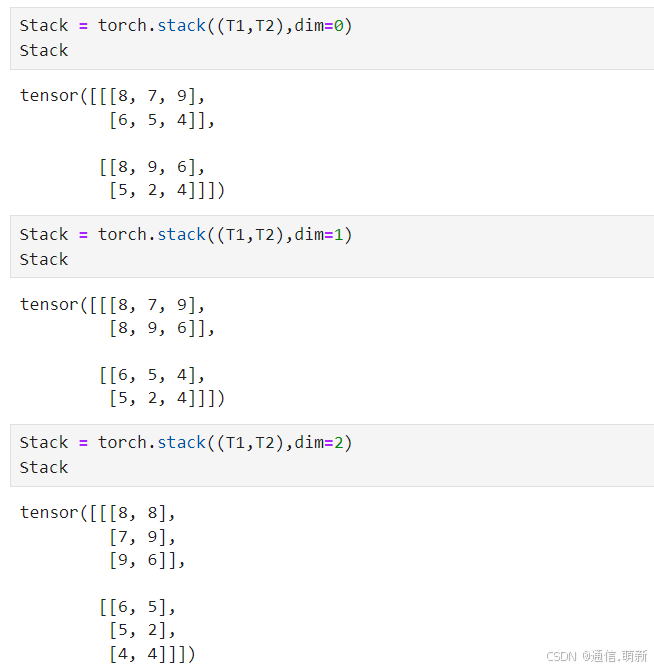
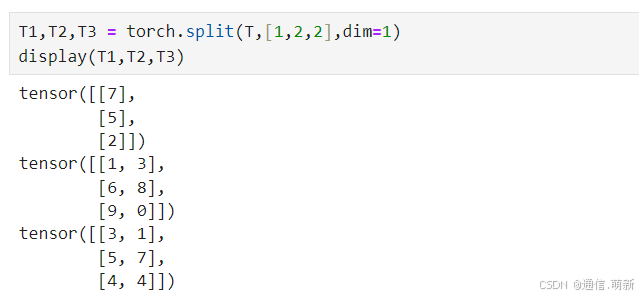
二、